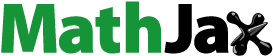
Abstract
Vaccines suppress the increased spread of infectious diseases or the number of severe cases. Considerable research and development yielded several vaccines against coronavirus disease 2019 (COVID-19). To contain the disease, governments have run vaccination campaigns and implemented restrictions depending on vaccination status. Ensuring the stability of vaccine supply with increased and/or seasonal demand has thus become critical. However, determination of the required vaccine supply is difficult due to variations in virus mutations, their impacts on the disease, and vaccine efficacy. In this work, we developed a mathematical model to consider the effect of vaccination on the infection numbers, taking COVID-19 as a case study. We have conducted a sensitivity analysis to identify the key variables affecting the required vaccine supply. We have conducted a scenario analysis to assess the impact of changes in vaccine supply parameters on the number of severe cases in Japan. Production of different products at dual-use facilities has been suggested to tackle the variability in vaccine demand. The analysis provided insights into the feasibility and adequate timing for product-switch at dual-use facilities. The proposed method can be applied to other infectious diseases and can be expanded to support various aspects for decision-making for policy building.
1. Introduction
Vaccination is a countermeasure for suppressing the spread of infectious diseases or the number of severe cases, such as for coronavirus disease 2019 (COVID-19). Other interventions, such as mobility restrictions and physical distancing, can mitigate the risk of outbreaks, but at the expense of economic and social disruptions (Wouters et al. Citation2021). Immunity attained by vaccination and the reduction in number of severe cases requiring hospitalization can allow individuals to lead normal lives (Mallory et al. Citation2018; Frederiksen et al. Citation2020). Thus, vaccination can resolve the dilemma, i.e., the choice between saving lives and preserving the economy.
Several COVID-19 vaccines have now been authorized or approved around the world, with many more under development (Wouters et al. Citation2021). The vaccines authorized by the U.S. Food and Drug administration are Comirnaty and Pfizer-BioNTech, Spikevax and Moderna, Janssen, and Novavax COVID-19 vaccines (Food and Drug Administration Citationn.d.) with more than a dozen already being distributed (Hossain et al. Citation2021). In addition, hundreds of COVID-19 vaccines are under pre-clinical and clinical development to deal with new variants (World Health Organization Citationn.d.).
The focus has now shifted to expanding production capacity of vaccines to meet the expected high demand for COVID-19 after extensive research and development (Wouters et al. Citation2021). Governments around the world have tried to attain the vaccines and have run large vaccination campaigns. Policies were instituted to restrict people’s behavior according to their vaccination status. The constant evolution of the virus necessitates running further campaigns to ensure sufficient protection form new variants. At the same time, some developing countries are still struggling to attain enough vaccine supplies (So and Woo Citation2020; Castillo et al. Citation2021; Economist Intelligence Citation2021).
Biopharmaceutical production facilities needed for vaccine production require large capital investments (Ministry of Economy Trade and Industry Citationn.d.). Yet, vaccine production is typically regarded as unprofitable. Vaccines are preventatives rather than treatments. Generally, they also have long-lasting effects as opposed to products being periodically administered (Xue and Ouellette Citation2020). However, in the case of fast evolving viruses such as those associated with COVID-19, the need for several booster campaigns can be anticipated. To secure the sufficient production capacity, governments have been making policies related to vaccine production and supply, especially given the fluctuating or seasonal nature of the rise in demand following the initial outbreak. For example, the concept of “dual-use” facilities was conceived in Japan, where both vaccines and non-vaccine biopharmaceuticals can be produced in the same facility (Ministry of Economy Trade and Industry Citationn.d.). Thereby, the facility would produce biopharmaceuticals in normal times with no outbreaks of infectious diseases and would switch to vaccine production if the need arises. Such facilities are expected to reduce the investment risk, by ensuring the efficient use of the production capacity even in the absence of demand for vaccines. However, in such a case, balancing the switch between manufacturing different products would be critical to avoid delays in vaccine supply.
The required vaccine supply can be difficult to estimate as it will depend on the infectious disease considered, the available vaccines, and the evolution of variants. In the case of SARS-Cov-2, the evolution of new variants with different features affects how quickly the virus spreads, and the efficacy of the vaccines. In an effort to control infection rates and the probability of severe symptoms, there is a race to develop new boosters and vaccines (World Health Organization Citationn.d.). Therefore, there is a need for models that track infection rates and methods to support decision-making by analysing the impact of vaccine-related decisions on infection numbers given the different characteristics of infectious diseases and vaccine types.
This study aims to develop a method to gain insights into the impact of vaccine-related parameters on infection numbers under different conditions and their influence on vaccine production policies. The method has been applied to the case of COVID-19 in Japan as an example. Many mathematical models were developed before and after the outbreak of COVID-19 (Kaddar et al. Citation2011; Shu et al. Citation2012; Chen et al. Citation2020; Cooper et al. Citation2020; Fanelli and Piazza Citation2020; Jakhar et al. Citation2020; Linka et al. Citation2020; Ma Citation2020; Xu et al. Citation2020; Bubar et al. Citation2021; Buckner et al. Citation2021; Chang et al. Citation2021; Grimm et al. Citation2021; Han et al. Citation2021; Lee et al. Citation2021; Matrajt et al. Citation2021). These models are based on compartment models which are well established in the field of epidemiology. Compartment models categorize the population into distinct compartments, each representing a different health status. Among others, SEIR models are commonly used in the field, which consider four populations: susceptible (S), exposed (E), infected (I), and recovered (R). In previous work, we have developed a new SEIR model which utilizes mobility and environmental data to estimate the infection rate (Kim et al. Citation2021). The data-driven component in this model allows for tracking and continuously learning the changes affecting the time-dependent infection rate. In this work, we have extended this model to consider the additional effects of vaccination. The study has three components: (1) development of a mathematical model of infectious diseases to incorporate the effects of vaccination, (2) a sensitivity analysis to identify key variables regarding vaccine supply, and (3) a scenario analysis to assess and gain insights into the impact of the decision making in the production process on the infection numbers. Insights from the scenario analysis are used to assess the feasibility of operating a dual-use facility for vaccine production and to identify critical timing for product switch at such facilities. The proposed method can be also extended to other infectious diseases and could be used to examine different aspects of vaccine-related policies.
2. Methods
The Methods include sections on Datasets, Mathematical models, Sensitivity analysis and Scenario analysis.
2.1. Datasets
The datasets used in our study included COVID-19 cases, number of PCR tests, vaccination rate, mobility, and environment. We used a simple moving average of the last 7 days to reduce the variability between days of the week. For example, the number of newly infected people on 21st February 2020 was calculated using the data from 15th to 21st February 2020.
2.1.1. COVID-19 cases and number of PCR tests
We used the open data about the COVID-19 cases in Japan released by the Ministry of Health, Labour and Welfare (MHLW) (Citationn.d.). The number of newly infected people, the cumulative numbers of recovered patients, the COVID-19 related deaths, and the number of PCR tests were obtained.
2.1.2. Vaccination rate
We used the data about vaccination rate in Japan released by the Prime Minister’s Office of Japan (Citationn.d.).
2.1.3. Mobility
We used the mobility data for Japan released by Google (Citationn.d.). Changes in mobility in the six categories of retail and recreation, groceries and pharmacies, parks, transit stations, workplaces, and residential were included in the data. According to Google (Citationn.d.), the data show “how visits and length of stay at different places change compared to a baseline.” Here, the baseline day represents “the median value, for the corresponding day of the week, during the 5-week period Jan 3–Feb 6, 2020”.
2.1.4. Environment
We used the data about temperature and relative humidity released by the Japan Meteorological Agency (Citationn.d.) were used. The values in Tokyo were used for this study.
2.2. Mathematical model of infectious diseases
The present study investigates a comprehensive set of compartments representing distinct population groups. These compartments include susceptible (), vaccinated (
), exposed (
), infected (
), and recovered (
) populations, with their sub-compartments. The previous work (Kim et al. Citation2021) primarily focused on the fundamental transitions between compartments: from
to
from
to
from
to
and finally from
to
In this work, the model was expanded to incorporate the dynamics of vaccinated population (
), which aligns with the vaccination practiced in Japan during the specified time frame.
Within our study framework, we extended our analysis to account for the effects of 1st, 2nd, and 3rd shot of vaccinations. As a result, the vaccinated population () was categorized into three distinct sub-compartments (
). This approach recognized that vaccinated populations, though exhibiting a lower susceptibility to infectious diseases compared to their non-vaccinated counterparts, still retain a certain level of vulnerability. Consequently, the model encompassed sub-compartments of exposed (
) and infected populations (
). Moreover, to account for the finite duration of vaccine-induced immunization, transitions from
to
were introduced in the model.
The overview of the compartments is shown in , and the mathematical models are shown in EquationEqs. (1)–(13). The calculations for the derivative of the susceptible population () considered transitions from the vaccinated and recovered compartments (
) due to loss of antibodies, as well as transitions into the vaccinated compartments (
), and the exposed compartments (
). The derivatives of vaccinated populations (
) were determined by the number of people receiving 1st, 2nd, and 3rd vaccinations (
), the number of people waning immunity from the recovered and vaccinated compartments (
), and transitions to the exposed compartments (
). The derivatives of the exposed populations (
) were calculated based on transitions from the susceptible/vaccinated compartments (
) and transitions to the infected compartments (
). The derivatives of the infected populations (
) were calculated based on transitions from the exposed compartments (
) and transitions to the recovered compartment (
). Finally, the derivative of the recovered population (
) was determined by transitions from the infected compartments (
) and the number of people waning immunity (
).
Figure 1. Overview of the proposed mathematical model of infectious diseases. a. The compartments in the proposed model are classified by vaccination status in addition to infection status. b. Results of fitting the proposed model to the cases in Japan. The number of new COVID-19 cases in Japan was simulated from January 22, 2020 until 29 November, 2021.

The definition of other parameters in EquationEqs. (1)–(13) are as follows: are the incidence rates,
are the infection rates,
are the recovery rates, and
are the vaccine efficacies of 1st, 2nd, and 3rd shots of vaccination. In this study, it was assumed that antibody loss happens after 150 days. We considered the incidence rates (
) and the recovery rates (
) as disease-specific parameters and assumed that they were constant, using the same values with the previous work of the authors’ research group (Kim et al. Citation2021). We set the total population
which is the sum of all compartments, to be constant, and the population of Japan was used as the value of
(1)
(1)
(2)
(2)
(3)
(3)
(4)
(4)
(5)
(5)
(6)
(6)
(7)
(7)
(8)
(8)
(9)
(9)
(10)
(10)
(11)
(11)
(12)
(12)
(13)
(13)
For the calculations in and , the same approach of the random forest regression from the work of Kim et al. (Citation2021) was used to estimate the value of applying 6 days of time delay between explanatory variables (e.g., mobility on April 1st, 2020) and objective variables (e.g., number of infected people on April 7th, 2020). The number of PCR tests and vaccination rate were newly added to the data used in the random forest regression in this work. The scikit-learn package from the Python library was used for the calculations.
Figure 2. The results of sensitivity analysis to find critical parameters of vaccinations. a. Global sensitivity analysis using Sobol’ method. The vertical and horizontal axes represent contribution to the total variance, and vaccine-related parameters, respectively. The error bars show the 95% confidence interval. b. Contour line plot showing the impact of vaccination campaign start date and vaccine supply rate (daily number of vaccinations) on the total number of cases of COVID-19 in Japan. The maximum percentage of population vaccinated and the vaccine efficacy were fixed in this simulation. The star mark shows the result when all four parameters varied in the sensitivity analysis were fixed at their base values.

2.3. Sensitivity analysis
The sensitivity of the cumulative number of infections (from January 22nd, 2020 to November 29th, 2021) to four vaccine-related parameters was tested, where the parameters consist of start date of vaccine supply, vaccine supply rate, maximum percentage of population vaccinated, and vaccine efficacy changed. The Sobol’ method was used (I. M. Sobol’ Citation1990). The start date of vaccine supply was May 9th, 2021, and the time lag between vaccine production and vaccination was not considered in this study. The vaccine efficacy referred to the efficacy of 2nd vaccine, while the efficacy of 1st vaccine shot was fixed as 0.53. These values were taken as an average representing the different types of administered vaccines in Japan. Only two shots were administered in Japan within the time period of this analysis. The ranges of the parameters are shown in . The base values for each parameter are: May 9th, 2021 (start date of vaccine supply), 6.32 × 105 day−1 (vaccine supply rate), 0.80 (maximum percentage of population vaccinated), and 0.87 (vaccine efficacy). These values were determined based on the statistics reporting the actual case in Japan (National Institute of Infectious Diseases Citation2021).
Table 1. Ranges of start date of vaccine supply, vaccine supply rate, maximum percentage of population vaccinated, and vaccine efficacy values used in the sensitivity analysis.
2.4. Scenario analysis
The scenario analysis was performed to calculate the number of severe cases assuming that the spread of COVID-19 due to a new variant like the Omicron started on January 1st, 2022. The calculation was conducted until June 29th, 2022. The number of severe cases was defined as the product of the number of cases and the severity rate, and the severity rate was set for each compartment based on their vaccination status. For example, the number of severe cases on a given day is calculated as
The detailed calculation conditions were summarized as follows.
Table
The base scenarios in are summarized in .
Figure 3. Scenario analysis of the impact of the 3rd vaccine shot on the number of severe cases of COVID-19 in Japan. a. Base scenario 1 and sub-scenarios varying start date of vaccine supply, b. Base scenario 2 and sub-scenarios varying start date of vaccine supply under a slower vaccine supply rate (daily number of vaccinations), and c. Base scenario 3 and sub-scenarios varying start date of vaccine supply with a higher infection rate.

Table 2. Base scenarios description.
3. Results
3.1. Mathematical model of infectious diseases including vaccinated populations
The model developed in this work was used to calculate COVID-19 infection numbers in Japan from January 22nd, 2020 to November 29th, 2021. The newly developed model takes into account vaccination status in addition to infection status. The compartments of the proposed mathematical model are presented in . Previous models (Kim et al. Citation2021) considered susceptible (), exposed (
), infected (
), and removed (
) (including recovered and dead population) compartments. In this work,
included only the recovered population because the fatality rate of COVID-19 is much lower than the recovery rate. The vaccinated population against COVID-19 was further classified into three groups (
and
) according to the number of vaccination shots taken. The model considered vaccine efficacy per dose and corresponding compartments for exposed (
and
) and infected (
and
) were also added. The incidence rate
and the recovery rate
are specific to the infectious disease, whereas the efficacy
is determined by the vaccine as described in Methods section. The infection rate
was taken as time-dependent and was calculated using a data-driven component in the model. A random forest model was used to calculate
as a function of mobility, temperature, and humidity. The number of polymerase chain reaction (PCR) tests taken was additionally taken into account to assess its impact on the reported infection numbers. The model results for the prediction of the COVID-19 infection numbers in Japan are presented in , where R2 value was 0.976. The high accuracy of the prediction confirms the importance of compartmentalizing the population in the model according to vaccination status. The model can be adapted for different infectious diseases and the numbers of compartments can also be modified for each investigated disease.
3.2. Sensitivity analysis
A global sensitivity analysis was performed to quantify the sensitivity of the cumulative number of infections (from January 22nd, 2020 to November 29th, 2021) to changes in four parameters: the start date of vaccination supply, vaccine supply rate (i.e., average number of daily vaccinations), maximum percentage of population vaccinated, and vaccine efficacy. In this time period in Japan, only two vaccine shots were administered, and thus in this analysis, the effects of only the first two shots were considered. shows the contributions to total variances of cumulative COVID-19 cases in Japan with the four vaccine-related parameters. The start date of vaccine supply showed the highest impact on the number of cases followed by vaccine supply rate. This result confirmed the importance of a fast and massive supply of vaccines. The impact of the start date and the vaccine supply rate on the COVID-19 cases was visualized by a contour line plot in . The cumulative number of infections varied from less than 200 thousand to more than nine million depending on the values of the start date and the vaccine supply rate.
3.3. Scenario analysis
The numbers of severe cases which require hospitalization in Japan were calculated with different scenarios of vaccines and compared with the hospitalization capacity in Japan, as shown in . We analysed three base scenarios about the 3rd shot of vaccine in Japan, by assuming that the spread of COVID-19 due to a new variant similar to the Omicron variant had occurred on January 1st, 2022. All base scenarios assumed that vaccine supply for the 3rd shot started at January 1st, 2022. Twelve sub-scenarios were also analysed, where for each base scenario, the start date of the vaccination campaign was varied. The start date of the vaccination supply was highlighted as the most influential parameter during the sensitivity analysis. Base scenario 1, and the associated group of sub-scenarios, used the same vaccine supply rate as that actually administered in Japan and assuming the same mobility restrictions that were implemented (). Base scenario 2 and its sub-scenarios assumed a vaccination campaign 10% slower than Base scenario 1 (). This group of scenarios tested the impact of supply rate, which was highlighted as the second most influential parameter during the sensitivity analysis. Base scenario 3 and its sub-scenarios assumed a higher infection rate () to simulate an outbreak of a more infectious variant and/or a situation without mobility restriction ().
As shown in , the number of severe cases exceeds the capacity in Japan if the start date of vaccine supply would have been delayed by more than 10 days compared to the base scenario (Base scenario 1 in ). A 30-day delay in the start of the vaccination campaign resulted in a 3.9 times increase in the number of severe cases. In addition, a slower supply rate than that of Base scenario 1 caused the number of severe cases to exceed the limit when keeping the same start date of vaccine supply (Base scenario 2 in ). The strongest impacts were observed with the scenarios of higher infection rate (). In this group of scenarios, even those with earlier start dates of vaccine supply, the health care system would be overwhelmed in terms of the number of severe cases.
4. Discussion
The proposed model captured the effects of vaccines as well as human mobility and environment conditions on the number of COVID-19 cases. This inclusion enhances the model’s ability in representing real-world dynamics and contributes to a more accurate representation of disease transmission and vaccine-related effects in the population under consideration. By considering the number of vaccine shots, the model can be used to support the decision making of vaccine supply. The model is applicable for the simulation of the COVID-19 cases, where numerous factors, e.g., vaccines and mobility, have a complex effect on the number of cases, as shown in .
The proposed model also enabled the quantitative determination of critical vaccine-related parameters through the sensitivity analysis. The start date of vaccine supply was the most critical factor affecting the number of cases (see ), followed by the supply rate of vaccines. Supplying vaccines as fast as possible is critical to suppress the pandemic, thereby prompt decisions are required for the governments and the pharmaceutical industry. These findings help to set scenarios that can be directly used by governments for decision-making purposes.
The scenario analysis provided an insight into the feasibility and the switchover timing of the dual-use facilities. For cases like the Omicron variant, the earlier start of vaccine supply can suppress the number of severe cases under the capacity as shown in . In reality, the Omicron was defined as Variant Under Monitoring (VUM) on 24th November, 2021 in the world (National Institute of Infectious Diseases Citation2021). If the decision was made to switch the production system of dual-use facilities on the same date, there would be 37 days until 1st of January, 2022, the initial day of spread assumed in the simulation above. This time frame needs to cover switchover, manufacturing, and distribution to public. Although the application of dual-use systems seems to be a reliable option for producing sufficient vaccines quantities (Ministry of Economy Trade and Industry Citationn.d.), switching the production system to vaccine production should be prompted to avoid shortages.
In case of a higher infection rate due to a more contagious variant or less mobility restrictions, the hospitals would likely be overwhelmed even if the supply had started 30 days earlier as shown in . There would not be enough time for product switchover of dual-use facilities. In the case of using the same vaccine for the third shot, vaccine stockpiles would have to be strategically maintained for immediate release following the identification of a new highly contagious variant. However, in the case of rolling out a new vaccine or in the absence of strategic stockpiles, dedicated vaccine production facilities will be required to mitigate the impact as fast as possible for highly contagious and fatal infectious diseases. In this case government incentives would still be needed to promote investments into dedicated vaccine production facilities given the diminished need for them once herd immunity is attained or a disease is contained.
The insights acquired through this study hold enduring significance even within a post-COVID-19 context, offering valuable guidance for the formulation and execution of policies aimed at proactive pandemic preparedness. The recognition of the pivotal role of the start date of vaccines supply underscores the importance of policies focused on expediting vaccine manufacturing timelines and optimizing the efficiency of vaccine distribution networks. Consequently, strategic policy decisions may extend to considerations surrounding the optimal geographic location for vaccine production facilities, with the aim of enhancing distribution effectiveness. Moreover, the findings related to switchable parameters provide a roadmap for the strategic implementation of dedicated facilities, poised to effectively address the demands of future pandemics. By leveraging these insights, policymakers can foster a proactive approach that supports global readiness for impending health crises. Furthermore, the model developed in this study considered the comprehensive set of compartments including three times of vaccination. The model can be easily modified and applied to other infectious diseases.
Model compartments can be continuously updated as more data becomes available. The accuracy of case number predictions can be improved with further understanding of the changes in the impact of vaccines, e.g., loss of antibodies. Despite the use of fixed values for vaccine efficacy and antibody loss, the data-driven component in the model would adjust the time-dependent infection rate and help easier tracking of further changes in the system, e.g., changes in virus spread and waning immunity. In addressing the operational dynamics of dual-use facilities, wherein regular production of other biopharmaceuticals takes place, the transition process is a critical in demonstrating the viability of this concept. The present study conducted a scenario analysis to systematically evaluate this dimension. Specifically, the analysis probes into the feasibility of incorporating timely vaccine production for infectious disease within the dual-use facilities, and it assessed optimal transition timings by manipulating variables such as the start date, supply rate, and infection rate. Leveraging the insights derived from this investigation, subsequent research can delve into process-specific inquiries. For instance, there is a potential avenue for exploring the optimal selection of equipment to facilitate seamless transitioning and identifying biopharmaceutical types that lend themselves to easier switches within such facilities. Furthermore, it is noteworthy that despite the provision of vaccine supplies, a temporal gap persists until these vaccines are administered to individuals. The implications drawn from this study offer valuable guidance in ascertaining the extent to which this interval should be minimized. This, in turn, presents an opportunity for further research endeavors to explore strategies for streamlining and hastening this critical period.
The developed model and method can be expanded to assess different scenarios, which can result in better understanding of the requirements and impacts of vaccine supply. The method is flexible in terms of application to different regions with different policies and conditions of vaccine supply. Vaccine-related model parameters can be easily updated, and the model can use different weather and mobility patterns for training. The model can also be used for different infectious diseases, which makes it a valuable tool for drawing conclusions in cases of potential future pandemics.
Code Availability Statement
The codes used in the work are available at https://github.com/PharmaPSE-Covid/COVID19.
Author Contributions
K.M., J.K., Y.I., K.O., S.B., and H.S. conceived the initial idea. K.M., J.K., Y.I., K.O., and H.S. designed the study. Y.I. performed the computations, and K.M., J.K., and K.O. verified the results. H.S. supervised the project. K.M. and J.K. took the lead in writing the manuscript with support from Y.I., K. O., S.B., and H.S.
Acknowledgements
The authors acknowledge industrial experts from the International Society of Pharmaceutical Engineering (ISPE) Japan community of practice “PharmaPSE COP” for useful discussions.
Disclosure statement
No potential conflict of interest was reported by the author(s).
Data availability statement
Data generated during the study are available in a public repository, https://github.com/PharmaPSE-Covid/COVID19.
Additional information
Funding
References
- Bubar KM, Reinholt K, Kissler SM, Lipsitch M, Cobey S, Grad YH, Larremore DB. 2021. Model-informed COVID-19 vaccine prioritization strategies by age and serostatus. Science. 371:916–921. doi: 10.1126/science.abe6959.
- Buckner JH, Chowell G, Springborn MR. 2021. Dynamic prioritization of covid-19 vaccines when social distancing is limited for essential workers. Proc Natl Acad Sci U S A. 118:e2025786118. doi: 10.1073/pnas.2025786118.
- Castillo JC, Ahuja A, Athey S, Baker A, Budish E, Chipty T, Glennerster R, Kominers SD, Kremer M, Larson G, et al. 2021. Market design to accelerate COVID-19 vaccine supply. Science. 371:1107–1109. doi: 10.1126/science.abg0889.
- Chang S, Pierson E, Koh PW, Gerardin J, Redbird B, Grusky D, Leskovec J. 2021. Mobility network models of COVID-19 explain inequities and inform reopening. Nature. 589:82–87. doi: 10.1038/s41586-020-2923-3.
- Chen X, Li M, Simchi-Levi D, Zhao T. 2020. Allocation of COVID-19 vaccines under limited supply. medRxiv. doi: 10.1101/2020.08.23.20179820.
- Cooper I, Mondal A, Antonopoulos CG. 2020. A SIR model assumption for the spread of COVID-19 in different communities. Chaos Solitons Fractals. 139:110057. doi: 10.1016/j.chaos.2020.110057.
- Economist Intelligence. 2021. Coronavirus vaccines: expect delays. [accessed 2022 Jul 2]. https://www.eiu.com/n/campaigns/q1-global-forecast-2021/.
- Fanelli D, Piazza F. 2020. Analysis and forecast of COVID-19 spreading in China, Italy and France. Chaos Solitons Fractals. 134:109761. doi: 10.1016/j.chaos.2020.109761.
- Food and Drug Administration. n.d. COVID-19 vaccines. [accessed 2022 Jul 2]. https://www.fda.gov/emergency-preparedness-and-response/coronavirus-disease-2019-covid-19/covid-19-vaccines#basics.
- Frederiksen LSF, Zhang Y, Foged C, Thakur A. 2020. The long road toward COVID-19 herd immunity: Vaccine platform technologies and mass immunization strategies. Front Immunol. 11:1817. doi: 10.3389/fimmu.2020.01817.
- Google. n.d. COVID-19 community mobility reports. [accessed 2022 Jul 2]. https://www.google.com/covid19/mobility/.
- Grimm V, Mengel F, Schmidt M. 2021. Extensions of the SEIR model for the analysis of tailored social distancing and tracing approaches to cope with COVID-19. Sci Rep. 11:4214. doi: 10.1038/s41598-021-83540-2.
- Han S, Cai J, Yang J, Zhang J, Wu Q, Zheng W, Shi H, Ajelli M, Zhou XH, Yu H. 2021. Time-varying optimization of COVID-19 vaccine prioritization in the context of limited vaccination capacity. Nat Commun. 12:4673. doi: 10.1038/s41467-021-24872-5.
- Hossain MK, Hassanzadeganroudsari M, Feehan J, Apostolopoulos V. 2021. The race for a COVID-19 vaccine: where are we up to? Expert Rev Vaccines. 21:355–376. doi: 10.1080/14760584.2022.2021074.
- Jakhar M, Ahluwalia PK, Kumar A. 2020. COVID-19 epidemic forecast in different states of India using SIR model. medRxiv. doi: 10.1101/2020.05.14.20101725.
- Japan Meteorological Agency. n.d. [accessed 2022 Jul 2]. https://www.jma.go.jp/jma/index.html.
- Kaddar A, Abta A, Alaoui HT. 2011. A comparison of delayed SIR and SEIR epidemic models. NAMC. 16:181–190. doi: 10.15388/NA.16.2.14104.
- Kim J, Matsunami K, Okamura K, Badr S, Sugiyama H. 2021. Determination of critical decision points for COVID-19 measures in Japan. Sci Rep. 11:16416. doi: 10.1038/s41598-021-95617-z.
- Lee EK, Li ZL, Liu YK, Leduc J. 2021. Strategies for vaccine prioritization and mass dispensing. Vaccines (Basel). 9:506. doi: 10.3390/vaccines9050506.
- Linka K, Peirlinck M, Kuhl E. 2020. The reproduction number of COVID-19 and its correlation with public health interventions. Comput Mech. 66:1035–1050. doi: 10.1007/s00466-020-01880-8.
- Ma J. 2020. Estimating epidemic exponential growth rate and basic reproduction number. Infect Dis Model. 5:129–141. doi: 10.1016/j.idm.2019.12.009.
- Mallory ML, Lindesmith LC, Baric RS. 2018. Vaccination-induced herd immunity: Successes and challenges. J Allergy Clin Immunol. 142:64–66. doi: 10.1016/j.jaci.2018.05.007.
- Matrajt L, Eaton J, Leung T, Brown ER. 2021. Vaccine optimization for COVID-19: Who to vaccinate first? Sci Adv. 7. doi: 10.1126/sciadv.abf1374.
- Ministry of Economy, Trade and Industry. n.d. The strategy for strengthening the vaccine development and production system. [accessed 2023 May 12]. https://www.meti.go.jp/english/policy/mono_info_service/mono/bio/vaccine_development/index.html.
- Ministry of Health, Labour and Welfare, Open data. n.d. [accessed 2022 Jul 2]. https://www.mhlw.go.jp/stf/covid-19/open-data.html.
- National Institute of Infectious Diseases. 2021. The B.1.1.529 mutant strain of SARS-CoV-2 (Omicron strain) (5th Report); [accessed 2022 Jul 25]. https://www.niid.go.jp/niid/ja/2019-ncov/2551-cepr/10876-sars-cov-2-b-1-1-529.html.
- Prime Minister’s Office of Japan. n.d. COVID-19 vaccines. [accessed 2022 Jul 2]. https://www.kantei.go.jp/jp/headline/kansensho/vaccine.html.
- Shu H, Fan D, Wei J. 2012. Global stability of multi-group SEIR epidemic models with distributed delays and nonlinear transmission. Nonlinear Anal Real World Appl. 13:1581–1592. doi: 10.1016/j.nonrwa.2011.11.016.
- So AD, Woo J. 2020. Reserving coronavirus disease 2019 vaccines for global access: cross sectional analysis. BMJ. 371:m4750. doi: 10.1136/bmj.m4750.
- Sobol’ IM. 1990. On sensitivity estimation for nonlinear mathematical models. Matem Mod. 2:112–118.
- Word Health Organization. n.d. COVID-19 vaccine tracker and landscape. [accessed 2022 Jul 2]. https://www.who.int/publications/m/item/draft-landscape-of-covid-19-candidate-vaccines.
- Wouters OJ, Shadlen KC, Salcher-Konrad M, Pollard AJ, Larson HJ, Teerawattananon Y, Jit M. 2021. Challenges in ensuring global access to COVID-19 vaccines: production, affordability, allocation, and deployment. Lancet. 397:1023–1034. doi: 10.1016/S0140-6736(21)00306-8.
- Xu C, Yu Y, Chen YQ, Lu Z. 2020. Forecast analysis of the epidemics trend of COVID-19 in the USA by a generalized fractional-order SEIR model. Nonlinear Dyn. 101:1621–1634. doi: 10.1007/s11071-020-05946-3.
- Xue QC, Ouellette LL. 2020. Innovation policy and the market for vaccines. J Law Biosci. 7:lsaa026. doi: 10.1093/jlb/lsaa026.