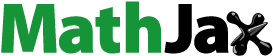
Abstract
In a cluster randomized trial clusters of persons, for instance, schools or health centers, are assigned to treatments, and all persons in the same cluster get the same treatment. Although less powerful than individual randomization, cluster randomization is a good alternative if individual randomization is impossible or leads to severe treatment contamination (carry-over). Focusing on cluster randomized trials with a pretest and post-test of a quantitative outcome, this paper shows the equivalence of four methods of analysis: a three-level mixed (multilevel) regression for repeated measures with as levels cluster, person, and time, and allowing for unstructured between-cluster and within-cluster covariance matrices; a two-level mixed regression with as levels cluster and person, using change from baseline as outcome; a two-level mixed regression with as levels cluster and time, using cluster means as data; a one-level analysis of cluster means of change from baseline. Subsequently, similar equivalences are shown between a constrained mixed model and methods using the pretest as covariate. All methods are also compared on a cluster randomized trial on mental health in children. From these equivalences follows a simple method to calculate the sample size for a cluster randomized trial with baseline measurement, which is demonstrated step-by-step.
Introduction
The effect of a new treatment on some quantitative health or educational outcome, for instance, the total score on a clinical questionnaire for depression or on a mathematical skills test, is usually evaluated with a “pretest-posttest control group design.” So, the outcome is measured before and after treatment on each participant in the treated group and in the control group. Randomized treatment assignment, also known as the randomized clinical trial (RCT), is seen as the golden standard for causal inference, but it is not always feasible. For instance, to compare different methods of classroom teaching, classes, but not individual students, can be randomized. Similarly, the effect of a health promotion program for smoking prevention or healthy food can be assessed by randomizing communities (towns, schools), but usually not individuals. Further, even if individual randomization is possible, it may lead to treatment contamination, that is, to carry-over of treatment components into the control group, by communication between treated and controls. A similar contamination risk exists when comparing two psychotherapies and randomizing individual patients instead of therapists or health centers. For these reasons, cluster randomized trials (Donner & Klar, Citation2000; Hayes & Moulton, Citation2009), also known as group randomized trials (Murray, Citation1998), are frequently encountered in psychology, education, and health research. In such trials, a large number of natural groups or clusters, such as schools, communities, and health centers, are randomly assigned to treatment or control, and all persons in the same cluster get the same treatment. Recent cluster randomized trials (CRTs) in psychology are found in, among others, the Journal of Consulting and Clinical Psychology (Conner et al., Citation2019; Crane et al., Citation2019; Felder et al., Citation2017; Haug et al., Citation2017; Morgan et al., Citation2018; Valente et al., Citation2018), the Journal of Educational Psychology (Herman et al., Citation2022; Olive et al., Citation2019; Savage et al., Citation2013), and Health Pychology (Donenberg et al., Citation2018; Ho et al., Citation2020).
A CRT is less powerful than an RCT due to outcome variation between clusters as expressed by the intraclass correlation (ICC), the proportion of the total (unexplained by predictors) outcome variance that is between as opposed to within clusters. Even a small ICC can make the sampling variance of the treatment effect twice as large as in an RCT, and this effect of the ICC is known as the design effect. On the other hand, treatment contamination in an RCT with individual randomization reduces the treatment effect and thereby also the power of the trial (for technical details and a discussion of when to prefer a CRT to an RCT, see e.g., Hemming et al., Citation2021; Moerbeek, Citation2005; Torgerson, Citation2001). Further, in most CRTs as in most RCTs, the outcome of interest is measured not only after treatment (posttest), but also before treatment (pretest, baseline). This further complicates the analysis and the sample size calculation of a CRT. The aim of this paper is therefore two-fold. The first aim is to show the equivalence of the state-of-the-art method of analyzing a CRT with a baseline measurement, which is a three-level mixed regression analysis, to some simple methods with respect to treatment effect estimation and testing in case of an equal sample size per cluster. The second aim is to show the practical implications of that equivalence for sample size calculation. Achieving these aims will also help researchers to understand (a) when a three-level mixed regression analysis is needed and when a simple method is acceptable, and (b) which specification of the random part of a mixed model (i.e., the variances and correlations) is safe and which specification can lead to underpowered studies and Type I errors, and (c) what the difference is between using the baseline as a repeated measure and using it as a covariate.
Throughout this paper we assume a CRT with two treatment arms and we label these as treated and control (where control may either be no treatment or treatment as usual), and a quantitative outcome that is measured before (baseline, pretest) and after (posttest) treatment. We initially assume an equal sample size per cluster, but this assumption is relaxed later.
Concerning the equivalence between new and old methods of treatment effect testing, analyzing a CRT without baseline measurement with a two-level mixed model (with as levels cluster and person) gives the same results as first computing the outcome mean per cluster and then analyzing cluster means with the two-sample t-test if the sample size is the same in each cluster (Moerbeek et al., Citation2003; Searle et al., Citation2006, p. 53, p. 415–416; Searle & Pukelsheim, Citation1986). Further, treatment effect testing in an RCT without nesting but with a baseline measurement, with a two-level mixed regression model with person and measurement as levels, and treatment, time, and their interaction as predictors, and an unstructured covariance matrix for the repeated measures, is equivalent to a two-sample t-test on the change from baseline (CHANGE = posttest minus pretest) (Van Breukelen, Citation2013). Finally, treatment effect testing with a constrained mixed model that assumes absence of a pretest group difference is equivalent to analysis of covariance with the posttest as dependent variable and the pretest as covariate (ANCOVA) with respect to the treatment effect, and nearly so with respect to its sampling variance (Liang & Zeger, Citation2000; Liu et al., Citation2009; Van Breukelen, Citation2013). The present paper shows similar equivalences for a CRT with baseline measurement, which gives rise to three-, two-, and one-level methods of analysis.
Concerning sample size calculation, Van Breukelen and Candel (Citation2012a) presented a simple formula for sample size calculation for a CRT without baseline measurement. Sample size formulae for CHANGE and ANCOVA in an classical RCT without nesting but with baseline follow from a combination of the sample size formula for the two-sample t-test (Cohen, Citation1988; Julious, Citation2010) with published equations for the sampling variance of the treatment effect when using CHANGE or ANCOVA instead of just the posttest (Porter & Raudenbush, Citation1987; Rausch et al., Citation2003; Senn, Citation1989). The present paper extends upon that work by presenting a sample size calculation method for CRTs with a baseline measurement that draws on the equivalences of the various methods of analysis for such a trial. There is more literature about sample size calculation for CRTs. Raudenbush (Citation1997), and Moerbeek et al. (Citation2000) derived optimal designs for cluster randomized trials, taking into account the study budget and the study cost per cluster and per person, but limited to trials without baseline measurement. Further, Heo and Leon (Citation2009), Heo et al. (Citation2013), and Teerenstra et al. (Citation2012) presented sample size methods for CRTs with a baseline measurement, but their statistical models will be seen to be more restrictive than the model in this paper. Finally, Cunningham and Johnson (Citation2016), Fazzari et al. (Citation2014), Hedges and Borenstein (Citation2014), Heo and Leon (Citation2008), and Teerenstra et al. (Citation2008) present sample size methods for two-, three-, and four-level CRTs without repeated measures, assuming a variance components model that is a special case of the model in the present paper. The present paper will show how the equivalences between complex and simple methods of treatment effect testing in a CRT with baseline measurement lead to a simple sample size calculation under weaker assumptions than any of the above papers.
The outline of this paper is as follows. The next section introduces a published CRT in mental health among primary school children (Kraag et al., Citation2009), of which the data will be reanalyzed after the theoretical part of the paper as an illustration of the theory. Subsequently, a general three-level regression model is presented for the analysis of a CRT with a baseline measurement and a quantitative outcome. It will be shown that this model includes as special cases various mixed models used in practice, specifically a variance components model and some random slope models. It is then shown that estimating and testing the treatment effect with the general model gives the same results as three more simple methods that reduce the model to a two- or even one-level model by aggregating repeated measures within persons to change scores, or aggregating person data to cluster means, or both. What then follows is the equivalence of a constrained three-level model that assumes absence of a treatment group difference at baseline with three more simple methods that reduce the model to a two- or even one-level model by treating the baseline as a covariate, or by aggregating person data to cluster means, or both. All methods are illustrated not only with simulated data, but also on the CRT in mental health. After that, it is shown how the aforementioned equivalences lead to a simple method for computing the sample size needed for a CRT with a baseline measurement. This method is then applied to the CRT on mental health and compared with the sample size according to the calculators Optimal Design Plus version 3.01 (Raudenbush et al., Citation2011; Spybrook et al., Citation2011) and Power and Precision version 4 (Borenstein et al., Citation2011), and an online calculator from the National Institutes of Health (Research Methods Resources: National Institutes of Health, Citation2023). The last section summarizes all results, points out study limitations that may inspire future research, and gives recommendations for sample size planning and data analysis.
A cluster randomized trial in mental health
Kraag et al. (Citation2009) reported a CRT to evaluate the effects of a stress management program on stress, coping, anxiety, and depression in school children with age 9-11 years at baseline. In total, 52 primary schools in the south-east of the Netherlands were randomly assigned to the program (26 schools) or control (26 schools), with one or two classes per school participating in the trial, but 3 schools (1 program, 2 control) withdrew before treatment. The program was implemented by the classroom teachers within a month after the pretest and consisted of lessons, booster sessions, homework assignments, daily exercises, and a teacher manual. The outcome variables of interest were measured before and after treatment, with a time interval of 7 months. The average number of responding children was about 28 per school. The data were analyzed with a three-level mixed linear regression model with school, child, and measurement as levels; treatment, time, and a treatment by time interaction as predictors; a random school effect; and an unstructured within-school covariance matrix for the repeated measures. This model was subsequently extended with covariates such as sex and ethnicity. A significant and beneficial though small effect was found on coping, but not on stress, depression, or anxiety. Two outcome variables of this trial will be reanalyzed later in this paper to illustrate the theory.
Three-level mixed model for a cluster randomized trial with baseline
To analyze a quantitative outcome variable Y measured before and after treatment in a CRT with k clusters of n persons each, the following model is assumed:
(1)
(1) with subscript i = 1,2,….n for person, j = 1,2,…k for cluster, and t = 1,2 for time point. Here, G indicates the treatment arm (0 for control, 1 for treated), and T indicates the time point (0 for baseline, 1 for posttest). Further,
is a random cluster effect with mean zero, and
is a residual with mean zero to capture a person effect and measurement error, at time point t.
Given this predictor coding, the fixed model part can be interpreted as follows: is the expected outcome at baseline in the control arm;
is the expected outcome difference at baseline between both treatment arms (which is zero in a CRT and in an RCT, and will be constrained to zero in a later section);
is the expected change from baseline in the control arm;
is the expected difference between both arms with respect to change from baseline, which is the parameter of interest for evaluating the treatment effect.
The random model part consists of a random cluster effect and a residual
capturing a person effect and measurement error, per time point t. The random effects
and
are assumed to be bivariate normal with zero mean and 2*2 between-cluster covariance matrix
for all j = 1,2,….k. The random effects of different clusters are assumed to be independent, that is,
and
are independent for all j ≠ j’ irrespective t = t’ or t ≠ t’. Likewise, the residuals
and
are assumed to be bivariate normal with zero mean and 2*2 within-cluster covariance matrix
for all i = 1,2,….n and independent for all i ≠ i’ irrespective j, j’, t, t’. The resulting covariance matrix for the two repeated measures of person i,
and
is then
the sum of the between-cluster and within-cluster covariance matrices. For the sequel, it is useful to identify the elements of each covariance matrix, and the following notation will be used for that:
(2)
(2)
The intraclass correlation (ICC) at time point t is defined as:
(3)
(3) which is the correlation between the outcomes
and
for any two different persons in the same cluster j at the same time point t. In its present general form in EquationEquation (2)
(2)
(2) , this mixed model can handle any kind of random effects model that preserves homogeneity of
and of
between treatment arms (heterogeneity is briefly discussed in the last section before the discussion). In particular, the following models are special cases of EquationEquation (2)
(2)
(2) :
the variance component or random intercept model, with a random cluster effect with variance
random person effect with variance
and measurement error with variance
This is equivalent to:
the random slope model, with a random cluster effect, random person effect, and random time effect
to allow individual differences in change. This gives
as in model 1, and
where
is the variance of the time effect, and
is the covariance between the person and time effects.
the random slope model, with a random cluster effect, random person effect, measurement error, and random time effect
to allow differences in change between clusters in the same arm. This gives
and
as in model 1, where
is the between-cluster variance of the time effect, and
is the covariance between the cluster and time effects.
the random slope model, with a random cluster effect, random person effect, and random time effect
to allow differences in change between clusters and between persons. This gives
as in model 3 and
as in model 2. However, model 4 has as many parameters as EquationEquation (2)
(2)
(2) and comes down to a reparametrization of it.
Of these four models, the random intercept model 1 was used to derive sample sizes by Heo and Leon (Citation2009), and model 2 was used by Heo et al. (Citation2013), assuming The random slope model 4 was used for sample size planning by Teerenstra et al. (Citation2012), assuming
and
Note that restricting the covariances to zero in the random slope models is not innocuous, as it obstructs fitting data where the pretest variance is larger than the posttest variance, unless the time coding is reversed or the software allows negative variances. Further, combining measurement error (as in model 1) with individual differences in change (as in model 2) gives an unidentifiable model if there are only two repeated measures. Model 2 is more flexible than model 1 by allowing for heterogeneity of variance between time points, but allowing the measurement error variance in model 1 to be time-dependent gives the same flexibility. Finally, the random intercept model 1 was also used for sample size planning in three-level designs without repeated measures by Cunningham and Johnson (Citation2016), Fazzari et al. (Citation2014), Hedges and Borenstein (Citation2014), Heo and Leon (Citation2008), Moerbeek et al. (Citation2000), and Teerenstra et al. (Citation2008).
Three-, two-, and one-level analyses of change from baseline
Theory and method
A quantitative outcome measured before and after treatment in a CRT with two treatment arms can be analyzed with mixed linear regression using the unconstrained model in EquationEquations (1)(1)
(1) and Equation(2)
(2)
(2) , or any of its special cases as listed in the previous section. This paper focuses on the general model, which can either be seen as a bivariate two-level model, involving as levels cluster and person, and as variables the pretest and posttest measurements, or as a three-level model, with the measurements as the first level. While the model of EquationEquations (1)
(1)
(1) and Equation(2)
(2)
(2) can be fitted to the raw data with standard software for mixed (multilevel) regression, the analysis can be simplified by summarizing data across persons per time point. For a CRT without baseline measurement and with an equal sample size per cluster it has been shown that two-level mixed regression of the individual data is equivalent to first aggregating the outcome to cluster means and then performing a two-sample t-test on the cluster means (Moerbeek et al., Citation2003; Searle et al., 2006, p. 53, p. 415–416; Searle & Pukelsheim, Citation1986). This equivalence extends to the CRT with a baseline measurement. Specifically, aggregating the individual baseline measurements to cluster means, and likewise aggregating the posttest measurements, we end up with a two-level (repeated measures) design with as levels cluster and time, which can be analyzed with the following two-level mixed regression model for repeated measures:
(4)
(4) with as covariance matrix
where:
(5)
(5) and n is the number of persons sampled per cluster.
An alternative approach to simplification of the three-level analysis is to summarize data not across persons, but across time points. As said before, the parameter of interest is in EquationEquation (1)
(1)
(1) , which is the difference between both arms with respect to change from baseline. This parameter can be estimated as follows. First, compute per person a summary measure called CHANGE, and defined as
the difference between the person’s posttest and pretest (baseline) value on the outcome of interest. Then, submit that summary measure to a two-level mixed regression, with as levels cluster and person, as the only predictor the treatment indicator
of EquationEquation (1)
(1)
(1) , and as random effects a cluster effect
and a person effect
:
(6)
(6) from which the intercept
and the expected baseline group difference
have canceled out, and in which
is as in EquationEquation (1)
(1)
(1) the expected change from baseline in the control arm, and
is as in EquationEquation (1)
(1)
(1) the expected difference between both arms with respect to change from baseline. Further, the random effects have the following variances and ICC, where the subscript cha means CHANGE:
(7a)
(7a)
(7b)
(7b)
(7c)
(7c)
(7d)
(7d)
For RCTs, the CHANGE summary method has already been shown to be equivalent to mixed regression of the repeated measures obtained before and after treatment (Van Breukelen, Citation2013), and the same equivalence will be seen to hold for CRTs. An example of this method of analysis is Olive et al. (Citation2019, p. 1336).
Finally, by summarizing data both across time points and across persons, we end up with a one-level (single outcome) analysis using the two-sample t-test for cluster means of CHANGE, or equivalently, fixed effects regression with the following model:
(8)
(8) where
with variance
and the sampling variance of the treatment effect estimator is simply
(9)
(9)
Here, and
are again cluster-level and person-level variance of CHANGE, as before, and
is the total variance of CHANGE for an arbitrary person from an arbitrary cluster, and k is the total number of clusters. The factor
in EquationEquation (9)
(9)
(9) is known as the design effect (DE, here
) and indicates the inflation of the sampling variance of the treatment effect due to the clustering. In the absence of a cluster effect, we have
and
= 1, and EquationEquation (9)
(9)
(9) then reduces to the sampling variance of the treatment effect estimator based on CHANGE in a classical RCT with a total sample size of nK persons.
The four methods of analysis, a three-level analysis of the measurements per person per time point using EquationEquations (1)(1)
(1) and Equation(2)
(2)
(2) , a two-level analysis of cluster means per time point using EquationEquation (4)
(4)
(4) , a two analysis of individual change scores using EquationEquation (6)
(6)
(6) , and a one-level analysis of cluster mean change scores using EquationEquation (8)
(8)
(8) , are shown in .
Illustration by simulation
The four methods will now be compared on five simulated CRTs of k = 40 clusters of n = 50 persons each to illustrate the equivalences shown by math in the preceding section with real numbers obtained by statistical data analysis. Only one replication is reported per parameter setting to show that the equivalences hold per replication and not just on the average across replications. The data are generated with EquationEquations (1)(1)
(1) and Equation(2)
(2)
(2) , with asfixed effects
= 100,
= 0,
= 20 and
= 10, implying a baseline outcome mean of 100 in both treatment arms, an average change from baseline of 20 in the control group, and an average change of 30 in the treated group, see EquationEquation (1)
(1)
(1) . Note, however, that the covariance matrix of fixed effects estimators in a linear mixed model does not depend on the true fixed effects (Verbeke & Molenberghs, Citation2000), and so the choice of fixed effects can be done without loss of generality. The first three simulations assumed an outcome variance of 10 at the cluster level and of 100 at the person level at each time point (
), implying an ICC of 0.09 at both time points, well within the range of ICCs found in health and educational research (Adams et al., Citation2004; Hedges & Hedberg, Citation2007). The three simulations differed in the pre-post correlations, however. The last two simulations allowed the outcome variance components and the ICC at posttest to differ from those at pretest at each design level (cluster, person). Details of the parameter choices and the results for all methods of analysis in all simulations are given in . All analyses were done with SPSS version 27. The SPSS syntaxes for one simulation are available as online supplement, with one syntax for all analyses of individual data, and one syntax for all analyses of cluster means. The other simulations differed in syntax from these two files only with respect to the parameter values as specified in step 1 of the syntax for individual data.
Table 1. Treatment effect estimate (SE) and variance component estimates from four methods of analysis of a CRT with a baseline measurement: Mixed regression for repeated measures on individual data and on cluster means, and CHANGE on individual data and on cluster means (k = 40 clusters, n = 50 persons per cluster, treatment effect =10). The treatment effect and its SE are the same for all four methods.
Within a simulation all four methods give the same treatment effect estimate and SE, which is therefore reported only once per simulation in . Between simulations the effect estimate varies due to sampling error as each simulation involved a new sample. The variance estimates are related as follows: Those of the two-level analysis of cluster means are obtained from those of the three-level analysis with EquationEquation (5)(5)
(5) and n = 50. Those of the two-level CHANGE analysis follow from the three-level analysis with EquationEquation (7)
(7a)
(7a) . The variance estimate of the one-level CHANGE analysis follows from EquationEquations (5)
(5)
(5) and Equation(7)
(7a)
(7a) .
Three-, two-, and one-level analyses of covariance
Theory and method
The preceding section showed the equivalence of a three-level mixed linear regression of a CRT with pre- and posttest outcome measurements to a two-level analysis of individual change from baseline scores, and to a one-level analysis of cluster means of change from baseline, as far as treatment effect estimation and testing is concerned. However, a baseline (pretest) measurement can also be included into the analysis as a covariate by regressing the posttest measurement on treatment and pretest (or, equivalently as far as the treatment effect is concerned, by regressing change from baseline on treatment and pretest, Laird, Citation1983; Liu et al., Citation2009). This again reduces the three-level analysis to a single measurement two-level analysis with as levels cluster and person. That analysis in turn can be reduced to a single measurement one-level analysis by aggregating individual data of both the outcome (posttest) and the covariate (pretest), and then regressing the posttest cluster mean on the treatment and on the pretest cluster mean. Further, in the context of a classical RCT without clustering, it has been shown that, apart from a small difference in the standard error of the treatment effect, analysis of covariance (ANCOVA) regressing posttest on treatment and pretest is equivalent to mixed linear regression following EquationEqs. (1)(1)
(1) and Equation(2)
(2)
(2) minus the cluster effects (i.e.,
) and with the baseline group difference constrained to be zero, that is,
= 0 (Liu et al., Citation2009; Van Breukelen, Citation2013), which is a valid constraint for an RCT. In the context of a CRT, the same equivalence between ANCOVA and mixed regression is obtained by noting that we can aggregate individual data to cluster means per time point, and then analyze these either with mixed linear regression using EquationEquation (1)
(1)
(1) with
= 0 and the same 2*2 covariance matrix as in EquationEquation (5)
(5)
(5) , or with the aforementioned one-level ANCOVA regressing the posttest cluster mean on treatment and on the pretest cluster mean. This section therefore compares four methods:
three-level analysis of individual data, using EquationEquation (1)
(1)
(1) with the constraint
= 0, and EquationEquation (2)
(2)
(2) ;
two-level analysis of cluster means per time point, using EquationEquation (1)
(1)
(1) with
= 0, and a covariance matrix of pretest and posttest cluster means following EquationEquation (5)
(5)
(5) ;
two-level ANCOVA regressing the individual posttest measure on treatment and on the individual pretest, taking clustering effects in posttest and in pretest into account;
one-level ANCOVA regressing the posttest cluster mean on treatment and on the pretest cluster mean.
Technical details of the last two methods will now be given. From EquationEquation (2)(2)
(2) and the independence between the cluster level random effects on the one hand and the person level random effects on the other hand it follows that regression of posttest on pretest within treatment groups involves two regressions, one at the cluster level (between-cluster regression), and one at the person level (within-cluster regression). Ignoring at first the fixed effects and focusing on the random model part, we have for the between-cluster regression of posttest on pretest:
(10a)
(10a) with
as unexplained posttest variance between clusters, and for the within-cluster regression of posttest on pretest:
(10b)
(10b) with
as unexplained posttest variance within clusters.
Inserting this into EquationEquation (1)(1)
(1) with the constraint
= 0 gives as equation for posttest
(11)
(11)
Replacing the unobservables and
with
and
respectively, gives the following identifiable approximation (Grilli & Rampichini, Citation2011, p. 124; Klar & Darlington, Citation2004, p. 2347; Shin & Raudenbush, Citation2010, p. 29; Snijders & Bosker, Citation1999, p. 30):
(12)
(12) with
which regresses the posttest on treatment group, the pretest cluster mean (a cluster level covariate), and the pretest individual deviation from the cluster mean (a person level covariate). Further,
and
if either of two conditions holds:
or
This can be verified by plugging either condition into EquationEquation (12)
(12)
(12) and then rewriting into EquationEquation (11)
(11)
(11) . The first condition holds approximately if the sample size per cluster, n, is large (for details, see the appendix). The second condition implies absence of a so-called contextual effect (Grilli & Rampichini, Citation2011; Shin & Raudenbush, Citation2010). Of importance for the sequel are the following properties of the predictors in EquationEquation (12)
(12)
(12) : Due to the cluster randomization,
is uncorrelated with treatment
if the number of clusters k is large. Further, both between-cluster predictors,
and
are uncorrelated with the within-cluster predictor
The theory above concerns the third method as defined early in this section, that is, a two-level mixed regression of individual posttest data on treatment and pretest data, with a random cluster effect. This method was used by Morgan et al. (Citation2018, p. 637 and Table 6) and Savage et al. (Citation2013, p. 318–319), but only the latter clearly allowed for a contexual effect.
Concerning now the fourth method, starting from EquationEquation (12)(12)
(12) and then aggregating (averaging) across individuals within the same cluster gives the following one-level model for regressing the posttest cluster mean on treatment and on the pretest cluster mean:
(13)
(13)
from which has dropped out as it is by definition on average zero in each cluster.
Illustration by simulation
The four methods presented in this section, that is, (a) three-level analysis following EquationEquation (1)(1)
(1) with the constraint
= 0, (b) two-level analysis of cluster means per time following EquationEquation (4)
(4)
(4) with
= 0, (c) two-level regression of individual posttest on treatment and pretest, and d) one-level regression of posttest cluster mean on treatment and pretest cluster mean, were applied to the same simulated data as in , and the results are given in . The purpose of this is again to illustrate the equivalences shown mathematically in the preceding section with statistical data analysis on real numbers, and each row in corresponds to a single replication to show that the equivalences hold per replication and not just on the average across a large number of replications.
Table 2. Treatment effect estimate (SE) and variance component estimates from four methods of analysis of a CRT with a baseline measurement: Mixed regression for repeated measures on individual data and on cluster means with the constraint of no group difference at baseline, and ANCOVA on individual data and on cluster means (k = 40 clusters, n = 50 persons per cluster, treatment effect = 10).
For method (c) based on EquationEquation (12)(12)
(12) , two versions were applied: with and without the within-cluster covariate
First and foremost, shows that the four methods give the same results, and they do so for each simulation, as expected given the equivalence between analysis of individual data and analysis of cluster means in a CRT with an equal sample size per cluster (see also Moerbeek et al., Citation2003), and given the equivalence between mixed regression for repeated measures with a zero baseline difference (
= 0) and classical regression of the posttest on treatment and the pretest (Van Breukelen, Citation2013). There is only a very small difference in SE between the two mixed regressions on the one hand and the two ANCOVA methods on the other hand. This is due to a subtle difference between the two methods that vanishes as the sample size, here the number of clusters, increases in case of randomized studies (for details, see Winkens et al., Citation2007, table 1). A further result is that the two-level ANCOVA gives the same standard error and thus precision with or without the within-subject covariate. The explanation for this is given in the appendix.
Just as in , the variance component estimates of one method can be inferred from those of another method. Specifically, those for the two-level mixed model on cluster means follow from those for the three-level mixed model on individual data by EquationEquation (5)(5)
(5) . Likewise, the only variance component estimate for the one-level ANCOVA model in EquationEquation (13)
(13)
(13) for cluster means follows from the estimates for the two-level ANCOVA model on individual data in EquationEquation (12)
(12)
(12) by EquationEquation (5)
(5)
(5) , where the variances are now residual posttest variances after adjusting for the pretest. The relation between the variance components of the three-level mixed model on the one hand and the two-level ANCOVA model on the other hand is more complicated, but follows from Equation (10). Specifically, the estimated residual person level posttest variance
in the two-level ANCOVA model (12) follows from the estimated person level covariance matrix
of the three-level mixed model by EquationEquation (10b)
(10b)
(10b) . The residual cluster level posttest variance
in the two-level ANCOVA model (12) follows from the cluster level covariance matrix
of the three-level mixed model by EquationEquation (10a)
(10a)
(10a) , with a deviation of about 5%, depending on the simulation and the ANCOVA model (with versus without within-subject covariate). As said before, the residuals in EquationEquations (11)
(11)
(11) and Equation(12)
(12)
(12) slightly differ unless the sample size per cluster, n, is so large that
As a last remark on , note that the SE of the treatment effect in ANCOVA is smaller than in for CHANGE in each simulation except the last, which only confirms the established fact that, in randomized experiments, ANCOVA has at least as much power and precision as CHANGE, and usually more (Porter & Raudenbush, Citation1987; Rausch et al., Citation2003; Senn, Citation1989; Van Breukelen, Citation2006, Citation2013). The almost equal SE for CHANGE and ANCOVA in the last simulation is due to the fact that, in terms of cluster means, the posttest variance is larger than the pretest variance in that simulation. For details, see the appendix.
Application to the cluster randomized trial in mental health
The preceding two sections showed the equivalence of four methods of change analysis, varying from a three-level mixed regression to a two-sample t-test on cluster means of CHANGE, and a similar equivalence between four further methods, of which two are constrained mixed models for repeated measures and two treat the pretest as a covariate (ANCOVA). This was shown under the conditions of an equal sample size n per cluster and absence of missing data. In practice, these conditions will rarely be met and this will induce some differences between the methods. A varying sample size per cluster induces heteroscedasticity of cluster means, see EquationEquation (5)(5)
(5) , and this calls for a weighted analysis of cluster means (Searle & Pukelsheim, Citation1986). Missingness of pretest or posttest leads to exclusion of that person from the analysis when analyzing change scores or when regressing the posttest on the pretest (unless multiple imputation is used or the pretest distribution is specified to allow maximum likelihood estimation), but not when using mixed regression for repeated measures. This induces some difference between CHANGE and ANCOVA on the one hand and mixed regression of repeated measures on the other hand. This section explores the similarity of all methods on the CRT in mental health among primary school children (Kraag et al., Citation2009) that was introduced earlier in this paper, and in which sample size variation and missing data did occur.
In the CRT, which served to evaluate the effects of a stress management program on stress, coping, anxiety, and depression in primary school children, 52 primary schools were randomly assigned to the program (26 schools) or control (26 schools), but 3 schools (1 program, 2 control) withdrew before treatment. The average sample size per school was 28 pupils, but this sample size varied from 8 to 61 across schools. The present analyses concern two outcomes, emotion-focused coping and stress (for details, see Kraag et al., Citation2009, p. 1188). The missingness rate was about 4% for coping and about 2% for stress at each time point in each treatment condition. The two outcomes were first analyzed with all four methods from the section on CHANGE analysis, and then with all four methods from the ANCOVA section. The results are shown in and . The last column of each table shows two methods, one that weights cluster means equally and one that weights them proportionally to their sample size. Because weighting by the inverse sampling variance of the cluster mean is optimal (Searle & Pukelsheim, Citation1986), it follows from EquationEquation (5)(5)
(5) that unweighted analysis is more appropriate for large sample sizes per clusters (because the sampling variance of a cluster mean then approaches
), and cluster size weighting is more appropriate if the ICC is close to zero (because the sampling variance of a cluster mean then approaches
), with the tipping point being an ICC of 1/(n + 1).
Table 3. Treatment effect estimate (SE) and variance component estimates from four methods of analysis of the CRT in Kraag et al. (Citation2009). Sample size: treated: 25 schools, 645 pupils (per school: mean 25, SD 7), control: 24 schools, 719 pupils (per school: mean 30, SD 12).
Table 4. Treatment effect estimate (SE) and variance component estimates from four methods of analysis of the CRT in Kraag et al. (Citation2009) (the mixed regression models assume absence of a group difference at baseline). Sample size: treated 25 schools, 645 pupils (per school: mean 25, SD 7), control: 24 schools, 719 pupils (per school: mean 30, SD 12).
Focusing on first, all four methods show a significant treatment effect on emotion-focused coping (all p ≤ 0.02), and no evidence for an effect on stress (all p > 0.70), noting that df ≈ 47 (nr of schools minus 2) for all methods. There are some differences in effect estimate and standard error between the methods, but these are not substantial. Looking next at , the four methods again agree in showing a significant treatment effect on emotion-focused coping (all p < 0.02), and no evidence for an effect on stress (all p > 0.60). There are again small differences in effect estimate and standard error between the methods.
It might also be useful to compare the results in terms of effect sizes. For a comparison between two treatments on a quantitative outcome, Cohen’s d is a logical candidate, and it is defined as the estimator of the ratio of the expected outcome difference between both treatments to the within-treatment outcome SD. Cohen’s d is obtained by replacing each parameter in
with its sample counterpart (Cohen, Citation1988, Citation1992). However, for a CRT with pre- and posttest measurement, it is not that obvious how to define d. The numerator is the treatment effect, so parameter
in EquationEquations (1)
(1)
(1) and Equation(12)
(12)
(12) . In case of a CHANGE analysis,
is the expected difference between both treatments with respect to change from baseline. The denominator should then be either the square root of the unexplained variance of individual change scores,
see EquationEquation (7)
(7a)
(7a) , or the square root of the unexplained variance of cluster mean change,
see EquationEquation (9)
(9)
(9) , depending on whether we analyze individual change or cluster mean change. The second definition corresponds to what is called the operational effect size by Hedges and Rhoads (Citation2010, p. 441). Clearly, these two definitions give quite different effect sizes even if the sample size is the same for all clusters, see the last two columns of . Similarly, with ANCOVA the denominator could either be the square root of the total unexplained variance in EquationEquation (12)
(12)
(12) for individual data, which is
or the square root of the unexplained variance in EquationEquation (13)
(13)
(13) for cluster means, which is
and these two give quite different effect sizes even if the sample size is the same for all clusters, see the last two columns of . In the CRT of Kraag et al., the sample size varied strongly between clusters, leading to some difference between methods with respect to the treatment effect estimate
which is the numerator of the effect size estimate d, on top of the aforementioned differences in denominator. To give an impression: In , for emotion-focused coping we find d = 0.55/√(0.19 + 5.86) = 0.22 based on individual change scores, but d = 0.48/√0.41 = 0.75 based on cluster mean change. Reporting effect sizes for a CRT with pre- and posttest is thus meaningful only if the effect size is defined unequivocally in terms of the precise method of analysis and the underlying model parameters. Depending on the choices made, a large or small effect size can result. Psychologists may prefer the definition in terms of individual change, here d = 0.22, the more so as the definition in terms of cluster mean change gives an effect size that depends on the sample size per cluster. In fact, there are multiple ways to define the effect size, depending on whether the denominator is the square root of the total unexplained variance, or of the within-cluster variance only, or of the between-cluster variance only (Hedges, Citation2007; Stapleton et al., Citation2015), and depending on whether the residual variance is, or is not, adjusted for covariates (Olejnik & Algina, Citation2000). However, a full discussion of effect size definitions is beyond the present scope. As an alternative to standardizing the treatment effect by dividing it by the residual standard deviation, one may also compare the treatment effect with the scale range of the measurement instrument. Emotion-focused coping ranged from 10 to 26, and stress symptoms ranged from 20 to 77, in this study. Compared to these ranges, the treatment effect estimates in and , which are always well below 1, may seem small.
Sample size (power) calculation
Sampling variance of the treatment effect
In the section on CHANGE methods, it was shown that three-level mixed regression of a quantitative outcome in a cluster randomized trial with a baseline recording and the same sample size per cluster is equivalent to one-level analysis of CHANGE applied to cluster means with respect to the treatment effect estimate and its standard error. In the section on ANCOVA methods, it was shown that three-level mixed regression with the constraint of no baseline group difference (due to randomization) is equivalent to one-level ANCOVA (regressing posttest on treatment and pretest) applied to cluster means with respect to the treatment effect and almost with respect to its standard error. A practical implication of these results is that the sample size needed for a CRT with baseline to have a pre-specified power and precision for treatment effect testing and estimation can be computed in a simple way based on analysis of cluster means. This section explains and demonstrates sample size calculation for CHANGE, assuming at first the same sample size per cluster and then correcting for unequal sample sizes. Sample size calculation for ANCOVA is a bit more complicated and is here briefly discussed, with technical details in the appendix. Since ANCOVA is more powerful than CHANGE (Porter & Raudenbush, Citation1987; Rausch et al., Citation2003; Senn, Citation1989; Van Breukelen, Citation2006, Citation2013), sample size calculation for CHANGE is a safe, albeit conservative, method if combined with data analysis using ANCOVA. Further, note that sample size calculation for CRTs is not new, but published work for CRTs with baseline measurement assumes a more restrictive covariance structure than this paper does. For details, see EquationEquation (2)(2)
(2) and the references in that section. Moreover, presenting the analysis of a CRT with repeated measures in terms of CHANGE strongly simplifies the methodology for sample size calculation, as will be seen below.
Throughout this section it is assumed that the sample size per cluster is fixed at n, either based on practical constraints (such as the class size in schools, or the typical size of a group in group therapy), or based on what is known as optimal design. The latter means that the sample size per cluster should be chosen as where
is the ICC of the outcome at hand (change from baseline, or posttest adjusted for pretest) and c and s are, respectively, the study cost per cluster and per study participant (Moerbeek et al., Citation2000; Raudenbush, Citation1997). Given a sample size n per cluster, the following equation holds for the sampling variance of the treatment effect estimator when using CHANGE of cluster means, as a function of the total number of clusters, k:
(14)
(14)
Here, k is the total number of clusters in the CRT, and all three variances and the covariance term concern cluster means and are given in EquationEquation (5)(5)
(5) . EquationEquation (14)
(14)
(14) is a rewriting of EquationEquation (9)
(9)
(9) , as may be verified by using EquationEquations (5)
(5)
(5) and Equation(7)
(7a)
(7a) .
EquationEquation (14)(14)
(14) is the same as for CHANGE analysis of a classical RCT as presented in, among others, Porter and Raudenbush (Citation1987), Rausch et al. (Citation2003), Senn (Citation1989), and Winkens et al. (Citation2007), but now applied to cluster means. Specifically, (14) is the sampling variance of the mean difference between two independent samples of k/2 units each when the dependent variable is the average change per cluster.
Using EquationEquations (5)(5)
(5) and Equation(7)
(7a)
(7a) , EquationEquation (14)
(14)
(14) can be rewritten into EquationEquation (9)
(9)
(9) to see how the sampling variance of the treatment effect depends on the total variance
and on the ICC
of individual change from baseline scores.
Sample size calculation
From EquationEquations (14)(14)
(14) and Equation(9)
(9)
(9) it can be derived that the total number of clusters needed for a power
to detect a treatment effect
when testing two-tailed with a Type I error
and sample size n per cluster, is (Julious, Citation2010; Van Breukelen & Candel, Citation2012a):
(15)
(15)
Here, is the design effect
is the ICC of change from baseline, see EquationEquations (7)
(7a)
(7a) and Equation(9)
(9)
(9) , and δ is the standardized effect size (estimated by Cohen’s d) applied to individual change data. Further,
is the 100(1-γ)-th percentile of the standard normal distribution (e.g., 1.28 for a power of 90%) and
is the 100(1-α/2)-th percentile (e.g., 1.96 if α = 0.05 two-tailed).
If there is no clustering effect, we have = 0,
= 1, and EquationEquation (15)
(15)
(15) then reduces to the total number of persons needed for a classical RCT, as may be verified by multiplying both sides of the equation by n. Stated differently, dividing the total sample size N = nk of a CRT by the design effect gives the effective sample size, that is, the sample size needed for a classical RCT to have the same power as the CRT has. As the ICC
increases, so do the number of clusters k and the total sample size N = nk that are needed. Note that increasing the sample size per cluster, n, increases the design effect, thereby canceling part of the power gain obtained by increasing n. The best way to increase the power and precision of a CRT is therefore to increase the number of clusters k. shows the total number of clusters needed according to EquationEquation (15)
(15)
(15) as a function of the sample size per cluster for three values of the ICC of change, assuming two-tailed testing with
= 5% and a power of 90%. As EquationEquation (15)
(15)
(15) shows, the number of clusters needed also depends strongly on the effect size. It is therefore important to choose an effect size that is neither unrealistically large (leading to an underpowered study), nor too small to be worthwile detecting (leading to a very large and possibly infeasible sample size). Further, the sample size as computed with EquationEquation (15)
(15)
(15) requires the application of some correction factors to account for (a) the fact that the test statistic used in the data analysis is a Student t-test instead of a z-test, and (b) the sample size n will usually vary between clusters in an unplanned way, and (c) persons or clusters may drop out from the study. The next subsection demonstrates sample size calculation for the mental health trial with these correction factors.
Step-by-step example
As an example, the sample size for the CRT of Kraag et al. (Citation2009) will be reconstructed step-by-step, using the information given in that publication. The authors assumed the posttest outcome as dependent variable, but here, change from baseline will be used instead. The consequences of that for the effect size, ICC, and power will be discussed after the example.
Step 1: Specification of the input parameters for EquationEquation (15)
(15)
(15)
The authors planned to test the treatment effect per outcome with two-tailed α = 0.01 instead of 0.05 to adjust for multiple outcome testing, which gives = 2.58. Further, they aimed at a power of 90%, so
= 1.28, to detect a medium sized effect, so δ = 0.50.
The authors planned to have a sample size of n = 30 pupils per school, which is roughly the average class size in the Netherlands. Further, they expected an ICC of 0.10, based on reviews of ICC values in CRTs in primary care (Adams et al., Citation2004) and in education (Hedges & Hedberg, Citation2007), lacking a similar review for mental health studies at that time. Note that gives an ICC below 0.05 for both outcomes and for posttest as dependent variable as well as for change.
Step 2: Calculation of the total number of clusters needed
The choices and assumptions in step 1 give as design effect:
and EquationEquation (15)
(15)
(15) then gives as the total number of clusters needed:
after rounding upward.
Step 3: Correction for the difference between a z-test and a t-test
EquationEquation (15)(15)
(15) is based on a z-test for the difference between two unpaired means, but the actual test will be a t-test because the outcome variance
is unknown and the factor
should then be replaced with
which is larger because the Student t-distribution has thicker tails than the standard normal distribution. As shown by Lemme et al. (Citation2015), the total number of clusters must be increased with 2 if
= 5% or with 4 if
= 1%, for a power of 80% as well as for a power op 90%. This gives k = 35 (instead of 31 as in step 2) in our example, which agrees very well with the results from two free power calculators for a CRT. Optimal Design Plus V3.01 (Raudenbush et al., Citation2011; Spybrook et al., Citation2011) gives a power of 90% for 35 clusters, based on α = 1%, ICC = 0.10, and δ = 0.50. Power and Precision V4.10 (Borenstein et al., Citation2011) gives a power of 0.895 for 34 clusters and a power of 0.916 for 36 clusters (it does not allow odd numbers). It also agrees well with an online calculator (Research Methods Resources: National Institutes of Health, Citation2023), which gives 18 clusters per treatment arm, so, 36 in total.
Step 4: Correction for sample size variation between clusters
EquationEquation (15)(15)
(15) and published sample size equations and software for CRTs assume that the sample size n is the same for each cluster. In practice, this sample size will vary between clusters, both because clusters (e.g., schools, classes, health centers) differ in total size and because not all cluster members may want to participate in the CRT. Given a total sample size
where
is the average sample size per cluster, the power of a CRT decreases as the variation in n between clusters increases. It has been shown (Van Breukelen et al., Citation2007) that this power loss can be compensated by multiplying the number of clusters k with a correction factor
where cv is the coefficient of variation (SD/mean) of the sample size per cluster. This cv depends on the distribution of n, but will rarely exceed 1.0 and is at most 0.50 for a normal distribution (to prevent negative cluster sizes) and up to 0.70 or so for skewed distributions. Assuming
= 0.70 gives as total number of clusters:
starting from k = 35 as obtained in step 3. The NIH online calculator gives k = 46. No details of this part of the NIH calculator could be found, but its result agrees with that given by a conservative method in Van Breukelen and Candel (Citation2012b) which uses as correction factor
starting from k = 36 clusters as given by the NIH calculator before correction.
Step 5: Correction for anticipated non-response and drop-out
Finally, Kraag et al. (Citation2009) increased the planned total number of schools in their CRT to 50 to compensate the power loss arising from 20% non-response or drop-out, noting that non-response/drop-out of individual children affects the power less than complete school non-response/drop-out, so that k = 50 is a safe correction. The actual sample size in the CRT was 52 schools (of which 3 dropped out before treatment) with an average sample size of 28 pupils. This correction of course only accounts for power loss incurred by non-response or drop-out that is at random, not for bias in treatment effect estimation that may arise when drop-out is related to unobserved variables. However, that is beyond the scope of this paper.
To this step-by-step example, five remarks must be added.
First, the effect size and the ICC, and thus also the sample size needed, will depend on whether the posttest measurement or change from baseline (CHANGE) is analyzed. The treatment effect itself is the same for both dependent variables, that is, in EquationEquations (1)
(1)
(1) and Equation(15)
(15)
(15) is the expected difference between both treatments at posttest because the expected pretest difference is zero due to the randomized treatment assignment. However,
and
in EquationEquation (15)
(15)
(15) will usually differ from
and
as EquationEquations (3)
(3)
(3) and Equation(7)
(7a)
(7a) show. One case in which
=
and
both hold, is when the posttest variance is equal to the pretest variance and the correlation between pretest and posttest is 0.50, at the person level and likewise at the cluster level. The effect size, ICC and sample size needed, are then the same for CHANGE as for posttest. If the pre-post correlation is larger (smaller) than 0.50 at either or both design levels, then change as dependent variable requires a smaller (larger) sample size than posttest analysis ignoring the pretest, at least if pre and post variance are equal at the individual level and also at the cluster level. If the pretest variance is larger (smaller) than the posttest variance at either or both design levels, then change requires a larger (smaller) sample size than posttest analysis, at least if the pre-post correlation is 0.50 at each design level. So, depending on the configuration of the covariance parameters in EquationEquation (2)
(2)
(2) and Equation(7)
(7a)
(7a) , CHANGE analysis can require a smaller or larger sample size than analyzing the posttest only. Lacking any prior knowledge from similar trials, a safe default assumption in the design phase may therefore be to assume that CHANGE and posttest analysis require the same sample size.
Secondly, and related to the first comment, the data can also be analyzed with ANCOVA (posttest as dependent, pretest as covariate). EquationEquation (15)(15)
(15) can then still be applied, but
and
must be replaced with the residual SD and residual ICC at posttest, respectively, with
in EquationEquation (12)
(12)
(12) as the residual. Details of the sampling variance of the treatment effect in ANCOVA are given in the appendix, showing that the sample size needed is usually smaller than that for CHANGE and posttest analysis. In the step-by-step example above, assuming the same outcome variance and ICC at pretest as at posttest, and a pretest-posttest correlation of 0.50 at each design level (cluster, person), CHANGE and posttest analysis both require 36 clusters, ignoring cluster size variation and drop-out (see step 3). In contrast, ANCOVA requires only 28 clusters then, both according to EquationEquations (A.2)
(A.2)
(A.2) and Equation(A.3)
(A.3)
(A.3) in the appendix and according to the online NIH calculator, but ignoring possible chance correlation between treatment indicator and covariate as expressed by the Variance Inflation Factor (VIF) in EquationEquation (A.2)
(A.2)
(A.2) . With a sample size of 28 clusters this chance correlation can increase the sampling variance of the treatment effect estimator of ANCOVA and the sample size needed with up to 19%, thus almost nullifying the advantage of ANCOVA compared to CHANGE. However, that requires the treatment-covariate correlation to be two standard errors away from zero (for details, see the appendix). More realistic would be a treatment-covariate correlation of one standard error, giving an increase of the sampling variance with 4%, so that ANCOVA would require 29 or 30 clusters in the example. Further, the difference in sample size needed by ANCOVA and CHANGE decreases as the covariate’s regression weight approaches one, see EquationEquations (12)
(12)
(12) and Equation(13)
(13)
(13) , which occurs if the pretest-posttest correlation is strong or if the posttest variance is much larger than the pretest variance, see EquationEquation (10)
(10a)
(10a) . Whether the sample size is best calculated for CHANGE, or ANCOVA, or posttest analysis ignoring the pretest, also depends on the availability of covariance parameter estimates from published trials. For ANCOVA, we either need estimates of the same parameters as for CHANGE minus that of the pretest variance, or estimates of the residual posttest variance at each design level (cluster, person) given the pretest covariate (see appendix). This availability may depend on the field of application (e.g., education or health).
Third, EquationEquation (7)(7a)
(7a) for CHANGE shows the risk of assuming a simple random intercept model for the school level as in models 1 and 2 below EquationEquation (2)
(2)
(2) , either in the design phase as in Heo and Leon (Citation2009), or in the analysis phase as in Kraag et al. (Citation2009) and Escriva-Boulley et al. (Citation2018). The random school effect drops out from the change from baseline score and thus from its sampling variance in EquationEquation (9)
(9)
(9) because
= 0 according to the model, leading to an underpowered study in the design phase and an underestimated standard error of the treatment in the analysis phase if the random school effect is not stable over time (i.e., if
in truth). A similar effect occurs in ANCOVA where the random intercept model implies a perfect pretest-posttest correlation at the cluster level, leading to underestimation of the sampling variance of the treatment effect (for details, see EquationEqs. (A.2)
(A.2)
(A.2) and Equation(A.3)
(A.3)
(A.3) in the appendix). Allowing for an unstructured covariance matrix at each design level as in EquationEquation (2)
(2)
(2) safeguards against this while still allowing model simplification if needed during data analysis (as done in and for stress symptoms).
As a fourth remark to the stepwise example, EquationEquation (15)(15)
(15) can also be used to compute the sample size needed for a pre-specified width of the confidence interval for the treatment effect, as follows: Assume a power of 50% so that
in EquationEquation (15)
(15)
(15) , and replace the true treatment effect
with half the pre-specified confidence interval width. This follows from the fact that half the confidence interval width is equal to
which can be rewritten into
by using EquationEquation (9)
(9)
(9) .
Last, we assumed homogeneity of the covariance matrices and
across treatment arms. Heterogeneity does not alter the equivalences between the four CHANGE methods, but the treatment effect test must then use the Satterthwaite-Welch degrees of freedom, and the sample size must be slightly larger (Lemme et al., Citation2015). For the ANCOVA methods, heterogeneity of
and
usually gives heterogeneity of the covariate’s regression weight and thus treatment by covariate interaction, see EquationEquation (10)
(10a)
(10a) , which is beyond our scope.
Discussion
This paper discussed the analysis of cluster randomized trials (CRTs) with a pretest and a posttest of a quantitative outcome variable. CRTs are run to evaluate the effects of an intervention administered at an organizational (e.g., school, health center, community) level, and they are frequently encountered in public health (lifestyle interventions), mental health (prevention of depression or bullying), family medicine (patient counseling), and education (teaching methods). CRTs are typically analyzed with three-level mixed regression of individual pre- and posttest data as in EquationEquation (1)(1)
(1) , taking clustering into account by one of the special cases of the covariance structure in EquationEquation (2)
(2)
(2) . These models range from a simple variance components model with a random cluster effect, a random person effect, and a random measurement effect, to the general model of EquationEquation (2)
(2)
(2) itself.
In the section on CHANGE it was shown that, with an equal sample size per cluster, treatment effect estimation and testing with the general three-level model is equivalent to, respectively, two-level mixed regression of pretest and posttest cluster means, two-level mixed regression of individual change (post-pre) scores, and one-level fixed regression of cluster mean change scores. In the section on ANCOVA, it was shown that three-level mixed regression following EquationEquations (1)(1)
(1) and Equation(2)
(2)
(2) but with the constraint
= 0 (implying absence of a baseline difference between treated and controls) is equivalent to, respectively, two-level mixed regression of pretest and posttest cluster means with the same constraint
= 0, two-level mixed regression of individual posttest scores on treatment and pretest scores (ANCOVA on individual data), and one-level fixed regression of cluster mean posttest scores on treatment and cluster mean pretest scores (ANCOVA on cluster means). All methods were furthermore applied to data from a CRT in mental health by Kraag et al. (Citation2009), suggesting that, even under strong sample size variation between clusters, the methods still give quite similar results. Subsequently, it was shown how the number of clusters needed for a CRT with baseline can be computed in a simple way for the CHANGE methods in , given specification of the Type I error risk α, power, effect size for change from baseline, ICC for change from baseline, and sample size per cluster. The appendix shows how this method can be used for the ANCOVA methods in .
Although the simulations used a fairly large sample with 40 clusters and 50 persons per cluster, the equivalences between the methods of analysis also hold for smaller sample sizes provided that restricted maximum likelihood (REML) estimation is used in mixed regression (for details on REML versus ML, see e.g. Searle et al., Citation2006; Verbeke & Molenberghs, Citation2000). However, the small difference in standard error of the treatment effect between ANCOVA and the constrained mixed model, visible in , becomes a bit larger if the number of clusters decreases (for details, see Van Breukelen, Citation2013, p. 920).
The results in this paper have practical implications for data analysis and sample size calculation for a CRT with baseline measurement. First, both the CHANGE and the ANCOVA methods are valid, but the latter have more power. For RCTs this was already known (Porter & Raudenbush, Citation1987; Rausch et al., Citation2003; Senn, Citation1989). For CRTs it follows from the equivalences shown in this paper between multilevel analyses on the one hand and a simple CHANGE or ANCOVA analysis of cluster means on the other hand. It is also illustrated by the smaller standard errors in compared to . Secondly, if the sample size is roughly the same in all clusters and there are not many missing data, then a simple analysis of cluster means is a good alternative to multilevel analysis for the purpose of treatment effect estimation (but not for estimating effects of covariates that vary within clusters). Third, the sample size for a CRT with a baseline measurement can be computed in a simple way without restrictive assumptions about the covariance structure (as made in most publications on sample size for CRTs), and without having to specify six different covariance parameters. Finally, the CHANGE EquationEquations (7)(7a)
(7a) and Equation(9)
(9)
(9) show that, in the random intercept model for cluster effects used in Heo and Leon (Citation2009), Kraag et al. (Citation2009), and Escriva-Boulley et al. (Citation2018), the random cluster effect cancels out from the sampling variance of the treatment effect, which leads to an increased Type I error risk and undercoverage of confidence intervals if the cluster effect is not stable over time. This model may also have been used in some trials mentioned in the introduction where the model was not reported in a clear way (Conner et al., Citation2019; Felder et al., Citation2017; Herman et al., Citation2022; Ho et al., Citation2020).
Just like any other paper, this one has its limitations. Here, we mention five.
First, the equivalences between the various methods only hold if the sample size is the same in all clusters and there are no missing data. Sample size variation between clusters increases the sampling variance of the treatment effect in case of analysis of individual data, and even more so in case of analysis of cluster means, whether weighted by cluster size or unweighted (Searle & Pukelsheim, Citation1986; Van Breukelen et al., Citation2007). Missingness at pretest or at posttest leads to a loss of power for all methods, but also to a difference between mixed regression for repeated measures, which can include all individuals with at least one measurement, and CHANGE and ANCOVA, which only include complete cases (unless the bivariate distribution of pretest and posttest is specified to allow multiple imputation or maximum likelihood estimation including incomplete cases). For these reasons, mixed regression of the individual pretest and posttest data remains the method of choice unless the sample size variation between clusters and the percentage of missingness are both small so that analysis of cluster means is nearly equivalent to mixed regression of individual data. However, as shown in the previous section, the equivalences between methods in case of an equal sample size per cluster simplify the sample size calculation for a CRT with repeated measures, and that sample size is then easily corrected for cluster size variation.
A second limitation is that effects of within-cluster covariates such as the individual’s age or years of education, are lost by aggregating individual data to cluster means, as shown by EquationEquations (12)(12)
(12) and Equation(13)
(13)
(13) . By categorizing covariates their effects can still be studied after aggregation, albeit with a loss of information due to the categorization. Specifically, the outcome mean can be computed per cluster per covariate category (e.g., separately for old and young individuals). The main effect of the covariate can then be tested with a paired t-test of old versus young persons, with clusters as units of analysis. The treatment by covariate cross-level interaction can be tested by the two-sample t-test of treated versus control clusters, with as dependent variable the mean outcome difference between old and young persons within the cluster. However, the problem that the outcome means are based on varying sample sizes may then be more pronounced than for testing the main effect of treatment because the covariate distribution will not be exactly the same in each cluster. It is therefore questionable whether aggregation is a viable alternative to the analysis of individual data for the study of within-cluster covariate effects.
Third, this paper is limited to CRTs with two repeated outcome measures. CRTs may include a follow-up measurement or an intermediate measurement between pre- and posttest. Literature on classical RCTs with more than two repeated measures suggests various methods of analysis, including an extension of EquationEquation (1)(1)
(1) with dummy indicators for all extra time points and with their interactions with treatment, or aggregation of repeated measures to a linear contrast or an area under the curve summary measure, which is then analyzed as a single measurement just like the change score (Frison & Pocock, Citation1992, Citation1997; Senn et al., Citation2000). For each of these methods, multilevel analysis accounting for intraclass correlation and cluster mean analysis can be expected to give the same results if the sample size is equal across clusters, and similar results if it varies mildly.
A fourth limitation of this paper is to quantitative outcomes. Categorical, especially binary, outcomes can occur in CRTs, for instance, smoking status in smoking prevention trials. Binary data are typically analyzed with mixed logistic regression or generalized estimating equations (GEE), but aggregation to the cluster level gives quantitative data (proportions or sums). This suggests various alternatives, among others binomial and Poisson regression, but also (weighted) linear regression with the log-odds ( at cluster level as dependent variable, where p is the proportion persons with outcome 1 in that cluster. Further, sample size calculation is more complicated for binary outcomes of a CRT as there are no closed form equations for the sampling variance of the treatment effect (Moerbeek et al., Citation2001; Teerenstra et al., Citation2010).
A last limitation to be mentioned is that to cluster randomized trials. Nonrandomized comparisons between two groups of clusters are abundant in psychology and education, such as a comparison between schools using textbook A and schools using textbook B for math with respect to the end-of-year math grades of their students, or a comparison between therapists treating depression with cognitive-behavioral therapy and therapists using interpersonal therapy. It is known from the literature on nonrandomized comparisons without clustering that CHANGE and ANCOVA can give opposite results, which is known as Lord’s paradox (see e.g., Maris, Citation1998; Van Breukelen, Citation2013). For nonrandomized comparisons, it thus first needs to be established which method is valid for treatment effect inference under which conditions (see e.g., Rubin, Citation2004, Citation2005; Schafer & Kang, Citation2008) before a meaningful comparison can be made between individual and aggregated data methods, or a sample size procedure can be proposed. The fact that CHANGE methods can only test treatment effects under the strong assumption that, without treatment, the two groups would have shown parallel change, is probably well-known. The equivalence between ANCOVA and a constrained mixed model that assumes absence of a baseline group difference is much less known, but it should be a warning against the use of ANCOVA for nonrandomized group comparisons with a baseline difference, regardless of whether the groups consist of individuals or of clusters.
In summary, based on the present work the following recommendations can be given for the data analysis and the sample size planning of a cluster randomized trial with a pretest and posttest of a quantitative outcome. First, if the sample size is nearly equal in all clusters and there are few missing data, then an analysis of cluster means is a simple alternative to mixed regression of individual data. Else, mixed regression of individual data is needed. Secondly, in both cases, ANCOVA or the nearly equivalent constrained mixed model can be expected to have more power than CHANGE respectively the unconstrained mixed model. However, the power gain depends on the pretest-posttest correlation, the ratio of pretest variance to posttest variance, and chance correlation between treatment and pretest (for details, see the Appendix). Third and last, sample size calculation is simplified by first assuming an equal sample size per cluster and a simple data analysis of cluster means with CHANGE or ANCOVA, and then correcting the number of clusters for the power loss arising from cluster size variation as expressed by the coefficient of variation of cluster size. These calculations can be done by hand with the present equations which show how each input parameter affects the sample size needed, or with the online NIH calculator which is quite user friendly, or with both as a double check. Whether the sample size calculation is based on CHANGE or ANCOVA depends on the planned method of data analysis and on the availability of covariance parameter estimates from similar trials in the field of application.
Article information
Conflict of interest disclosures: Each author signed a form for disclosure of potential conflicts of interest. No authors reported any financial or other conflicts of interest in relation to the work described.
Ethical principles: The authors affirm having followed professional ethical guidelines in preparing this work. These guidelines include obtaining informed consent from human participants, maintaining ethical treatment and respect for the rights of human or animal participants, and ensuring the privacy of participants and their data, such as ensuring that individual participants cannot be identified in reported results or from publicly available original or archival data.
Funding: This work was not supported by a grant.
Role of the funders/sponsors: None of the funders or sponsors of this research had any role in the design and conduct of the study; collection, management, analysis, and interpretation of data; preparation, review, or approval of the manuscript; or decision to submit the manuscript for publication.
Acknowledgments: The authors would like to thank the anonymous reviewers and associate editor for their comments on prior versions of this manuscript. The ideas and opinions expressed herein are those of the authors alone, and endorsement by the author’s institution is not intended and should not be inferred.
Open Scholarship
This article has earned the Center for Open Science badges for Open Data. The data are openly accessible at https://dataverse.nl/dataset.xhtml?persistentId=doi:10.34894/VHAFZL.
Additional information
Funding
References
- Adams, G., Gulliford, M. C., Ukoumunne, O. C., Eldridge, S., Chinn, S., & Campbell, M. J. (2004). Patterns of intracluster correlation from primary care research to inform study design and analysis. Journal of Clinical Epidemiology, 57(8), 785–794. https://doi.org/10.1016/j.jclinepi.2003.12.013
- Borenstein, M., Hedges, L., Rothstein, H., Cohen, J., Schoenfeld, D. (2011). Power and precision release 4.1. https://www.power-analysis.com/
- Cohen, J. (1988). Statistical power analysis for the behavioral sciences (2nd ed.). Erlbaum.
- Cohen, J. (1992). A power primer. Psychological Bulletin, 112(1), 155–159. https://doi.org/10.1037/0033-2909.112.1.155
- Conner, M., Grogan, S., West, R., Simms-Ellis, R., Scholtens, K., Sykes-Muskett, B., Cowap, L., Lawton, R., Armitage, C. J., Meads, D., Schmitt, L., Torgerson, C., & Siddiqi, K. (2019). Effectiveness and cost-effectiveness of repeated implementation intention formation on adolescent smoking initiation: A cluster randomized controlled trial. Journal of Consulting and Clinical Psychology, 87(5), 422–432. https://doi.org/10.1037/ccp000038710.1037/ccp0000387
- Crane, M. F., Boga, D., Karin, E., Gucciardi, D. F., Rapport, F., Callen, J., & Sinclair, L. (2019). Strengthening resilience in military officer cadets: A group-randomized controlled trial of coping and emotion-regulatory self-reflection training. Journal of Consulting and Clinical Psychology, 87(2), 125–140. https://doi.org/10.1037/ccp0000356
- Cunningham, T. D., & Johnson, R. E. (2016). Design effects for sample size computation in three-level designs. Statistical Methods in Medical Research, 25(2), 505–519. https://doi.org/10.1177/0962280212460443
- Donenberg, G., Emerson, E., & Kendall, A. D. (2018). HIV-risk reduction intervention for juvenile offenders on probation: The PHAT life group randomized controlled trial. Health Psychology, 37(4), 364–374. https://doi.org/10.1037/hea0000582
- Donner, A., & Klar, N. (2000). Design and analysis of cluster randomization trials in health research. Wiley.
- Escriva-Boulley, G., Tessier, D., Ntoumanis, N., & Sarrazin, P. (2018). Need-supportive professional development in elementary school physical education: Effects of a cluster randomized control trial on teachers’ motivating style and student physical activity. Sport, Exercise, and Performance Psychology, 7(2), 218–234. https://doi.org/10.1037/spy0000119
- Fazzari, M. J., Kim, M. Y., & Heo, M. (2014). Sample size determination for three-level randomized clinical trials with randomization at the first or second level. Journal of Biopharmaceutical Statistics, 24(3), 579–599. https://doi.org/10.1080/10543406.2014.888436
- Felder, J. N., Epel, E., Lewis, J. B., Cunningham, S. D., Tobin, J. N., Schindler Rising, S., Thomas, M., & Ickovics, J. R. (2017). Depressive symptoms and gestational length among pregnant adolescents: Cluster randomized controlled trial of CenterPregnancy plus group prenatal care. Journal of Consulting and Clinical Psychology, 85(6), 574–584. https://doi.org/10.1037/ccp0000191
- Frison, L., & Pocock, S. (1992). Repeated measures in clinical trials: Analysis using mean summary statistics and its implication for design. Statistics in Medicine, 11(13), 1685–1704. https://doi.org/10.1002/sim.4780111304
- Frison, L., & Pocock, S. (1997). Linearly divergent treatment effects in clinical trials with repeated measures: Efficient analysis using summary statistics. Statistics in Medicine, 16(24), 2855–2872. https://doi.org/10.1002/(SICI)1097-0258(19971230)16:24<2855::AID-SIM749>3.0.CO;2-Y
- Fuller, W. A. (1995). Estimation in the presence of measurement error. International Statistical Review / Revue Internationale de Statistique, 63(2), 121–147. https://doi.org/10.2307/1403606
- Fuller, W. A., & Hidiroglou, M. A. (1978). Regression estimation after correcting for attenuation. Journal of the American Statistical Association, 73(361), 99–104. https://doi.org/10.1080/01621459.1978.10480011
- Grilli, L., & Rampichini, C. (2011). The role of sample cluster means in multilevel models: A view on endogeneity and measurement error issues. Methodology, 7(4), 121–133. https://doi.org/10.1027/1614-2241/a000030
- Haug, S., Paz Castro, R., Kowatsch, T., Filler, A., Dey, M., & Schaub, M. P. (2017). Efficacy of a web- and text messaging-based intervention to reduce problematic drinking in adolescents: Results of a cluster-randomized controlled trial. Journal of Consulting and Clinical Psychology, 85(2), 147–159. https://doi.org/10.1037/ccp0000138
- Hayes, R. J., & Moulton, L. H. (2009). Cluster randomized trials. Chapman and Hall.
- Hedges, L. V. (2007). Effect sizes in cluster-randomized designs. Journal of Educational and Behavioral Statistics, 32(4), 341–370. https://doi.org/10.3102/1076998606298043
- Hedges, L. V., & Borenstein, M. (2014). Conditional optimal design in three- and four-level experiments. Journal of Educational and Behavioral Statistics, 39(4), 257–281. https://doi.org/10.3102/1076998614534897
- Hedges, L. V., & Hedberg, E. C. (2007). Intraclass correlation values for planning group-randomized trials in education. Educational Evaluation and Policy Analysis, 29(1), 60–87. https://doi.org/10.3102/0162373707299706
- Hedges, L. V., & Rhoads, C. (2010). Statistical power analysis. In International encyclopedia of education (pp. 436–443) Elsevier. https://doi.org/10.1016/B978-0-08-044894-7.01356-7
- Hemming, K., Taljaard, M., Moerbeek, M., & Forbes, A. (2021). Contamination: How much can an individually randomized trial tolerate? Statistics in Medicine, 40(14), 3329–3351. https://doi.org/10.1002/sim.8958
- Heo, M., & Leon, A. C. (2008). Statistical power and sample size requirements for three level hierarchical cluster randomized trials. Biometrics, 64(4), 1256–1262. https://doi.org/10.1111/j.1541-0420.2008.00993.x
- Heo, M., & Leon, A. C. (2009). Sample size requirements to detect an intervention by time interaction in longitudinal cluster randomized trials. Statistics in Medicine, 28(6), 1017–1027. https://doi.org/10.1002/sim.3527
- Heo, M., Xue, X., & Kim, M. Y. (2013). Sample size requirements to detect an intervention by time interaction in longitudinal cluster randomized trials with random slopes. Computational Statistics & Data Analysis, 60, 169–178. https://doi.org/10.1016/j.csda.2012.11.016
- Herman, K. C., Reinke, W. M., Dong, N., & Bradshaw, C. P. (2022). Can effective classroom behavior management increase student achievement in middle school? Findings from a group randomized trial. Journal of Educational Psychology, Online First, 114(1), 144–160. https://doi.org/10.1037/edu0000641
- Ho, H. C. Y., Mui, M. W.-K., Wan, A., Yew, C. W.-S., & Lam, T. H. (2020). A cluster randomized controlled trial of a positive physical activity intervention. Health Psychology, 39(8), 667–678. https://doi.org/10.1037/hea0000885
- Julious, S. A. (2010). Sample sizes for clinical trials. Chapman & Hall/CRC.
- Klar, N., & Darlington, G. (2004). Methods for analyzing change in cluster randomized trials. Statistics in Medicine, 23(15), 2341–2357. https://doi.org/10.1002/sim.1858
- Kraag, G., Van Breukelen, G. J. P., Kok, G., & Hosman, C. (2009). Learn young, learn fair’, a stress-management programme for 5th and 6th graders: Longitudinal results from an experimental study. Journal of Child Psychology and Psychiatry, and Allied Disciplines, 50(9), 1185–1195. https://doi.org/10.1111/j.1469-7610.2009.02088.x
- Lachin, J. M. (1981). Introduction to sample size calculation and power analysis for clinical trials. Controlled Clinical Trials, 2(2), 93–113. https://doi.org/10.1016/0197-2456(81)90001-5
- Laird, N. M. (1983). Further comparative analyses of pretest-posttest research designs. The American Statistician, 37(4a), 329–330. https://doi.org/10.1080/00031305.1983.10483133
- Lemme, F., Van Breukelen, G. J. P., Candel, M. J. J. M., & Berger, M. P. F. (2015). The effect of heterogeneous variance on efficiency and power of cluster randomized trials with a balanced 2x2 factorial design. Statistical Methods in Medical Research, 24(5), 574–593. https://doi.org/10.1177/0962280215583683
- Liang, K. Y., & Zeger, S. L. (2000). Longitudinal data analysis of continuous and discrete responses for pre-post designs. Sankhyā: The Indian Journal of Statistics, Series B, 62(1), 134–148. https://www.jstor.org/stable/25053123
- Liu, G. F., Lu, K., Mogg, R., Mallick, M., & Mehrotra, D. V. (2009). Should baseline be a covariate or dependent variable in analyses of change from baseline in clinical trials? Statistics in Medicine, 28(20), 2509–2530. https://doi.org/10.1002/sim.3639
- Maris, E. (1998). Covariance adjustment versus gain scores revisited. Psychological Methods, 3(3), 309–327. https://doi.org/10.1037/1082-989X.3.3.309
- Moerbeek, M. (2005). Randomization of clusters versus randomization of persons within clusters. Which is preferable? The American Statistician, 59(1), 72–78. https://doi.org/10.1198/000313005X20727
- Moerbeek, M., Van Breukelen, G. J. P., & Berger, M. P. F. (2000). Design issues for experiments in multilevel populations. Journal of Educational and Behavioral Statistics, 25(3), 271–284. https://doi.org/10.3102/10769986025003271
- Moerbeek, M., Van Breukelen, G. J. P., & Berger, M. P. F. (2001). Optimal experimental design for multilevel logistic models. Journal of the Royal Statistical Society: Series D (the Statistician), 50(1), 17–30. https://doi.org/10.1111/1467-9884.00257
- Moerbeek, M., Van Breukelen, G. J. P., & Berger, M. P. F. (2003). A comparison between traditional methods and multilevel regression for the analysis of multicenter intervention studies. Journal of Clinical Epidemiology, 56(4), 341–350. https://doi.org/10.1016/S0895-4356(03)00007-6
- Morgan, L., Hooker, J. L., Sparapani, N., Reinhardt, V. P., Schatschneider, C., & Wetherby, A. M. (2018). Cluster randomized trial of the classroom SCERTS intervention for elementary students with autism spectrum disorder. Journal of Consulting and Clinical Psychology, 86(7), 631–644. https://doi.org/10.1037/ccp0000314
- Murray, D. M. (1998). Design and analysis of group-randomized trials. Oxford University Press.
- Olejnik, S., & Algina, J. (2000). Measures of effect size for comparative studies: Applications, interpretations, and limitations. Contemporary Educational Psychology, 25(3), 241–286. https://doi.org/10.1006/ceps.2000.1040
- Olive, L. S., Byrne, D., Cunningham, R. B., Telford, R. M., & Telford, R. D. (2019). Can physical education improve the mental health of children? The LOOK study cluster-randomized controlled trial. Journal of Educational Psychology, 111(7), 1331–1340. https://doi.org/10.1037/edu0000338
- Porter, A. C., & Raudenbush, S. W. (1987). Analysis of covariance: Its model and use in psychological research. Journal of Counseling Psychology, 34(4), 383–392. https://doi.org/10.1037/0022-0167.34.4.383
- Raudenbush, S. W. (1997). Statistical analysis and optimal design for cluster randomized trials. Psychological Methods, 2(2), 173–185.https://doi.org/10.1037/1082-989X.2.2.173
- Raudenbush, S. W., Spybrook, J., Congdon, R., Liu, X., Martinez, A., Bloom, H., Hill, C. (2011). Optimal design plus empirical evidence (version 3.0). https://sites.google.com/site/optimaldesignsoftware/home
- Rausch, J. R., Maxwell, S. E., & Kelley, K. (2003). Analytic methods for questions pertaining to a randomized pretest, posttest, follow-up design. Journal of Clinical Child and Adolescent Psychology, 32(3), 467–486. https://doi.org/10.1207/S15374424JCCP3203_15
- Research Methods Resources: National Institutes of Health. (2023). https://researchmethodsresources.nih.gov/grt-calculator
- Rubin, D. B. (2004). Teaching statistical inference for causal effects in experiments and observational studies. Journal of Educational and Behavioral Statistics, 29(3), 343–367. https://doi.org/10.3102/10769986029003343
- Rubin, D. B. (2005). Causal inference using potential outcomes: Design, modeling, decisions. Journal of the American Statistical Association, 100(469), 322–331. https://doi.org/10.1198/016214504000001880
- Savage, R., Abrami, P. C., Piquette, N., Wood, C., Deleveau, G., Sanghera-Sidhu, S., & Burgos, G. (2013). A (Pan-Canadian) cluster randomized control effectiveness trial of the ABACADABRA web-based literacy program. Journal of Educational Psychology, 105(2), 310–328. https://doi.org/10.1037/a0031025
- Schafer, J. L., & Kang, J. (2008). Average causal effects from nonrandomized studies: A practical guide and simulated example. Psychological Methods, 13(4), 279–313. https://doi.org/10.1037/a0014268
- Searle, S., Casella, G., & McCulloch, C. (2006). Variance components. Wiley.
- Searle, S., & Pukelsheim, F. (1986). Effect of intraclass correlation on weighted averages. The American Statistician, 40(2), 103–105. https://doi.org/10.1080/00031305.1986.10475368
- Senn, S. J. (1989). Covariate imbalance and random allocation in clinical trials. Statistics in Medicine, 8(4), 467–475. https://doi.org/10.1002/sim.4780080410
- Senn, S. J., Stevens, L., & Chaturvedi, N. (2000). Repeated measures in clinical trials: Simple strategies for analysis using summary measures. Statistics in Medicine, 19(6), 861–877. https://doi.org/10.1002/(SICI)1097-0258(20000330)19:6<861::AID-SIM407>3.0.CO;2-F
- Shin, Y., & Raudenbush, S. W. (2010). A latent cluster-mean approach to the contextual effects model with missing data. Journal of Educational and Behavioral Statistics, 35(1), 26–53. https://doi.org/10.3102/1076998609345252
- Snijders, T. A. B., & Bosker, R. J. (1999). Multilevel analysis: An introduction to basic and advanced multilevel modeling. SAGE.
- Spybrook, J., Bloom, H., Congdon, R., Hill, C., Martinez, A., Raudenbush, S. W. (2011). Optimal design plus empirical evidence: Documentation for the “Optimal Design” software. https://sites.google.com/site/optimaldesignsoftware/home
- Stapleton, L. M., Pituch, K. A., & Dion, E. (2015). Standardized effect size measures for mediation analysis in cluster-randomized trials. The Journal of Experimental Education, 83(4), 547–582. https://doi.org/10.1080/00220973.2014.919569
- Teerenstra, S., Eldridge, S., Graff, M., de Hoop, E., & Borm, G. F. (2012). A simple sample size formula for analysis of covariance in cluster randomized trials. Statistics in Medicine, 31(20), 2169–2178. https://doi.org/10.1002/sim.5352
- Teerenstra, S., Lu, B., Preisser, J. S., Van Achterberg, T., & Borm, G. F. (2010). Sample size considerations for GEE analyses of three-level cluster randomized trials. Biometrics, 66(4), 1230–1237. https://doi.org/10.1111/j.1541-0420.2009.01374.x
- Teerenstra, S., Moerbeek, M., Van Achterberg, T., Pelzer, B. J., & Borm, G. F. (2008). Sample size calculations for 3-level cluster randomized trials. Clinical Trials (London, England), 5(5), 486–495. https://doi.org/10.1177/1740774508096476
- Torgerson, D. J. (2001). Contamination in trials: Is cluster randomisation the answer? BMJ (Clinical Research ed.), 322(7282), 355–357. https://doi.org/10.1136/bmj.322.7282.355
- Valente, J. Y., Cogo-Moreira, H., Swardfager, W., & Sanchez, Z. M. (2018). A latent transition analysis of a cluster randomized controlled trial for drug use prevention. Journal of Consulting and Clinical Psychology, 86(8), 657–665. https://doi.org/10.1037/ccp0000329
- Van Breukelen, G. J. P. (2006). ANCOVA versus change from baseline: More power in randomized studies, more bias in nonrandomized studies. Journal of Clinical Epidemiology, 59(9), 920–925. https://doi.org/10.1016/j.jclinepi.2006.02.007
- Van Breukelen, G. J. P. (2013). ANCOVA versus change from baseline in nonrandomized studies: The difference. Multivariate Behavioral Research, 48(6), 895–922. https://doi.org/10.1080/00273171.2013.831743
- Van Breukelen, G. J. P., & Candel, M. J. J. M. (2012a). Calculating sample sizes for cluster randomized trials: We can keep it simple and efficient!. Journal of Clinical Epidemiology, 65(11), 1212–1218. https://doi.org/10.1016/j.jclinepi.2012.06.002
- Van Breukelen, G. J. P., & Candel, M. J. J. M. (2012b). Efficiency loss due to varying cluster size in cluster randomized trials is smaller than literature suggests. Statistics in Medicine, 31(4), 397–400. https://doi.org/10.1002/sim.4449
- Van Breukelen, G. J. P., Candel, M. J. J. M., & Berger, M. P. F. (2007). Relative efficiency of unequal versus equal cluster sizes in cluster randomized and multicentre trials. Statistics in Medicine, 26(13), 2589–2603. https://doi.org/10.1002/sim.2740
- Verbeke, G., & Molenberghs, G. (2000). Linear mixed models for longitudinal data. Springer.
- Winkens, B., Van Breukelen, G. J. P., Schouten, H. J. A., & Berger, M. P. F. (2007). Randomized clinical trials with a pre- and a post-treatment measurement: Repeated measures versus ANCOVA models. Contemporary Clinical Trials, 28(6), 713–719. https://doi.org/10.1016/j.cct.2007.04.002
References specific to this appendix
- Cochran, W. G. (1968). Errors of measurement in statistics. Technometrics, 10(4), 637–666. https://doi.org/10.2307/1267450
- Fox, J. (1997). Applied regression analysis, linear models, and related methods. SAGE.
- Fuller, W. A. (1995). Estimation in the presence of measurement error. International Statistical Review / Revue Internationale de Statistique, 63(2), 121–147. https://doi.org/10.2307/1403606
- Fuller, W. A., & Hidiroglou, M. A. (1978). Regression estimation after correcting for attenuation. Journal of the American Statistical Association, 73(361), 99–104. https://doi.org/10.1080/01621459.1978.10480011
- Lachin, J. M. (1981). Introduction to sample size calculation and power analysis for clinical trials. Controlled Clinical Trials, 2(2), 93–113. https://doi.org/10.1016/0197-2456(81)90001-5
- Raaijmakers, J. G. W Pieters,., & J., P. M. (1987). Measurement error and ANCOVA: Functional and structural relationship approaches. Psychometrika, 52(4), 521–538. https://doi.org/10.1007/BF02294817
References occurring in the main text and in this appendix
- Grilli, L., & Rampichini, C. (2011). The role of sample cluster means in multilevel models: A view on endogeneity and measurement error issues. Methodology, 7(4), 121–133. https://doi.org/10.1027/1614-2241/a000030
- Porter, A. C., & Raudenbush, S. W. (1987). Analysis of covariance: Its model and use in psychological research. Journal of Counseling Psychology, 34(4), 383–392. https://doi.org/10.1037/0022-0167.34.4.383
- Rausch, J. R., Maxwell, S. E., & Kelley, K. (2003). Analytic methods for questions pertaining to a randomized pretest, posttest, follow-up design. Journal of Clinical Child and Adolescent Psychology, 32(3), 467–486. https://doi.org/10.1207/S15374424JCCP3203_15
- Senn, S. J. (1989). Covariate imbalance and random allocation in clinical trials. Statistics in Medicine, 8(4), 467–475. https://doi.org/10.1002/sim.4780080410
- Shin, Y., & Raudenbush, S. W. (2010). A latent cluster-mean approach to the contextual effects model with missing data. Journal of Educational and Behavioral Statistics, 35(1), 26–53. https://doi.org/10.3102/1076998609345252
- Snijders, T. A. B., & Bosker, R. J. (1999). Multilevel analysis: An introduction to basic and advanced multilevel modeling. SAGE.
- Winkens, B., Van Breukelen, G. J. P., Schouten, H. J. A., & Berger, M. P. F. (2007). Randomized clinical trials with a pre- and a post-treatment measurement: Repeated measures versus ANCOVA models. Contemporary Clinical Trials, 28(6), 713–719. https://doi.org/10.1016/j.cct.2007.04.002
Appendix:
Cluster randomized trials with a pretest and posttest
Section three-, two-, and one-level ANCOVA: theory and methods
Application of EquationEquation (9)(9)
(9) to data leads to underestimation of
due to imperfect reliability of the pretest cluster mean
as estimator of the true pretest cluster mean
. Specifically, for large number of clusters k the estimator of
when applying EquationEquation (9)
(9)
(9) to data, does not converge to
. Instead, for large k we have that (Grilli & Rampichini, Citation2011, p. 124; Shin & Raudenbush, Citation2010, p. 29; Snijders & Bosker, Citation1999, p. 30):
(A.1)
(A.1) where
is the ICC at pretest, defined by EquationEquation (3)
(3)
(3) , n is the sample size per cluster, and
is the reliability of the pretest cluster mean as estimator of
. Only if both k and n are large does
approach
Three things are noteworthy about EquationEquation (A.1)(A.1)
(A.1) . First,
obeys the Spearman-Brown formula for the reliability of a sum (or mean) score of n items as a function of the number of items and the reliability
of a single item. Here, the items are persons and the true score is the true pretest cluster mean. Second, if
EquationEquation (A.1)
(A.1)
(A.1) for
reduces to the equation for the attenuation of a regression weight by measurement error in the covariate (Cochran, Citation1968; Fuller, Citation1995; Fuller & Hidiroglou, Citation1978; Raaijmakers & Pieters, Citation1987). Of course, the case
is unrealistic if we measure the same individuals in the same clusters at both time points, pretest and posttest, see EquationEquation (7b)
(7b)
(7b) . Third and last, from (A.1) it follows that the regression weight of
in EquationEquation (9)
(9)
(9) is a weighted sum of the between- and the within-cluster regression weights, and equal to the between-cluster weight
only if
(large sample size per cluster) so that
or if
(no contextual effect).
Despite the bias in the estimation of due to imperfect reliability of
no bias results in the treatment effect estimation, because the predictors in EquationEquation (9)
(9)
(9) are uncorrelated (unconfounded) as explained in the main text. In fact, due to this uncorrelatedness any value used for
and for
in EquationEquation (9)
(9)
(9) gives the same treatment effect estimate apart from sampling error. The merit of including covariates into the analysis of a CRT are, just as in an RCT, a gain in power and precision for treatment effect testing and estimation by reducing unexplained outcome variance, at least in linear models. Specifically, including
as covariate reduces the unexplained between-cluster variance
to
(or more precisely,
see EquationEquations (7a)
(7a)
(7a) , (8), and Equation(9)
(9)
(9) ). Likewise, including
as covariate reduces the unexplained within-cluster variance
to
(or more precisely,
) . Now,
for the model in EquationEquation (9)
(9)
(9) is an increasing function of both unexplained variances and including both covariates would thus seem to reduce
and thereby increase the power and precision for the treatment effect test and estimation. As explained in the next section, however, omitting the within-cluster covariate does not change the precision of treatment effect estimation.
Section three-, two-, and one-level ANCOVA: illustration by simulation
Applying EquationEquation (9)(9)
(9) with or without the within-cluster covariate
gives the same standard error for the treatment effect. At first glance, this is counterintuitive because the covariate reduces the unexplained outcome variance within clusters and thus also the SE of the treatment effect, which is an increasing function of the within-cluster variance as well as of the between-cluster variance. The explanation for this can be seen in : Including the within-cluster covariate into the model, while reducing the estimated within-cluster variance
with an amount
(see EquationEquations (7)–(9)), also increases the estimated between-cluster variance
with an amount
(cf. Snijders & Bosker, Citation1999, p 100). This is important because the sampling variance (i.e. squared standard error) of the treatment effect is proportional to
(see EquationEquation (11)
(11)
(11) and the section on sample size calculation), and it will thus not change if the two variance components change as indicated above.
The surprising increase of as a result of adding the within-subject covariate can be understood in terms of ANOVA variance component estimation. In a oneway between-subject ANOVA with a random instead of a fixed group factor (here: the clusters),
and
where n is the sample size per cluster,
is the variance of the random cluster effect, and
is the residual (within-cluster) variance. So,
and
. If adding a within-subject covariate reduces the
and thus also
by an amount
this also increases
by an amount
because the
is unaffected by the within-subject covariate. This increase becomes ignorably small if n is large.
Section sample size (power) calculation
Analogously to Equationequation (11)(11)
(11) for the sampling variance of the treatment effect when using CHANGE of cluster means, the following equation applies when using ANCOVA:
(A.2)
(A.2) where k is the total number of clusters in the CRT, and all variances concern cluster means and are given in EquationEquations (4)–(6). Further,
is the correlation between pretest and posttest cluster means within the same treatment condition, and
in EquationEquation (13)
(13)
(13) is the squared correlation between the 0/1 treatment indicator and the pretest cluster mean (Fox, Citation1997). The factor
is known as Variance Inflation Factor (VIF) as it indicates how much the sampling variance of the treatment effect estimator is increased by correlation with the covariate compared to the case of no such correlation (Fox, Citation1997). Here,
is the correlation in the CRT at hand, as
is conditional on the design matrix (joint distribution of treatment and covariate) of that CRT. As k increases,
goes to zero and the VIF goes to one due to the cluster randomized treatment assignment. EquationEquation (A.2)
(A.2)
(A.2) then reduces to 4/k times the residual variance of the posttest cluster means, which would be
if the covariate’s regression weight were known. EquationEquation (A.2)
(A.2)
(A.2) is the same as for a classical RCT (as in Porter & Raudenbush, Citation1987; Rausch, Maxwell & Kelley, Citation2003; Senn, Citation1989; Winkens et al., Citation2007), but now applied to cluster means. In the design stage of a CRT,
is of course not known yet and may be replaced for the purpose of sample size calculation with an educated guess, for instance, that it will not exceed 4/(k-3), where k is the total number of clusters. This upper bound is based on the fact that the Fisher transformed correlation is approximately normally distributed with standard error
(Lachin, Citation1981) and the fact that, for correlations from −0.50 to +0.50, the Fisher transformed and untransformed correlation are almost equal. Alternatively, the expectation of the VIF might be used for sample size planning, but its derivation is complicated by the fact that it is a nonlinear function of
Using EquationEquation (4)(4)
(4) , the correlation
in EquationEquation (A.2)
(A.2)
(A.2) can be rewritten as:
(A.3)
(A.3) where
is the reliability of the pretest cluster mean as defined in EquationEquation (A.1)
(A.1)
(A.1) , and
is likewise the reliability of the posttest cluster mean. The proof of (A.3) is given at the end of this section. If
the correlation between pre- and posttest cluster means is a weighted mean of the between-cluster pre-post correlation
and the within-cluster pre-post correlation
analogous to EquationEquation (A.1)
(A.1)
(A.1) . Further, from EquationEquation (A.2)
(A.2)
(A.2) with
due to randomization it follows that if
then the ratio of the sampling variances in EquationEquations (11)
(11)
(11) and (A.2) is
implying that ANCOVA is more efficient than CHANGE unless
In fact, ANCOVA is always more efficient than CHANGE if
and
This can be verified by subtracting the expression for
in EquationEquation (A.2)
(A.2)
(A.2) from that in EquationEquation (11)
(11)
(11) , then dividing by
and rewriting into
where
. However, if the posttest variance is larger than the pretest variance, the regression weight
for the pretest cluster mean as predictor of the posttest cluster mean in ANCOVA can approach one, making ANCOVA close to CHANGE. Further, in small samples,
can occur due to sampling error, leading to a loss of efficiency of ANCOVA.
To compute the sample size for ANCOVA on cluster means or its equivalents in , replace in EquationEquation (15)(15)
(15)
with the SD of the posttest residual
of EquationEquation (12)
(12)
(12) , so, adjusted for the treatment and the pretest covariate, and replace the ICC
used in the DE, see EquationEquation (9)
(9)
(9) , with the ICC of the posttest residual.
Proof of EquationEquation (A.3)(A.3)
(A.3) : Using EquationEquation (4)
(4)
(4) gives
Taking EquationEquations (A.1)(A.1)
(A.1) and (3), and using
and
for t = 1,2, then gives EquationEquation (A.3)
(A.3)
(A.3) .