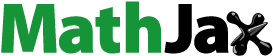
Abstract
Active and semi-active suspensions for passenger cars traditionally enhance comfort through body control, and vehicle handling by reducing the tyre load variations induced by road irregularities. Active suspensions can also be designed to track a desired yaw rate profile through the control of the lateral load transfer distribution between the front and rear axles. This paper considers an integrated system including semi-active and active suspension actuation to control the yaw, roll, pitch and heave dynamics excited by the driving actions. To this purpose, two novel real-time-capable implicit nonlinear model predictive control (NMPC) formulations, excluding and including cost function weight adaptation, are proposed and compared with the passive vehicle, and the controlled vehicle with two combinations of skyhook and active roll control, the first based on a pseudoinverse decoupling transformation for obtaining the damping force contributions, and the second using an inverse formulation. The algorithms are assessed through an experimentally validated simulation model, along manoeuvres corresponding to sub-limit and limit handling operation, to analyse the trade-off between body motion reduction and cornering response enhancement. The results show that the adaptable NMPC configuration provides the best performance in all scenarios, also for significant variations of the main vehicle and tyre parameters.
1. Introduction
Semi-active and active suspensions are frequently adopted in modern cars to improve comfort and handling. Such systems could become more widely spread in the next generations of automated vehicles, to enable the users to comfortably carry out other activities during vehicle operation [Citation1].
Controllable suspensions are based on actuators between the sprung and unsprung masses, and thus influence the motion of the sprung mass and the vertical tyre force distribution among the vehicle corners. Differently from active suspensions, semi-active actuators cannot introduce energy into the system, i.e. they are implemented in the form of shock absorbers with controllable damping characteristics, and therefore are effective only during transients. Semi-active implementations are more frequent than active suspensions because they represent a balanced compromise in terms of cost, component weight, power consumption, and performance [Citation2,Citation3]. However, active suspensions offer significantly enhanced functionality, including the capability of: a) shaping the level of vehicle understeer in steady-state conditions, and b) increasing yaw and sideslip damping during transients [Citation4].
This paper proposes and compares controllers for a next-generation hydraulic suspension system set-up by Tenneco Automotive. The hardware architecture enables independent damping force control on each vehicle corner, and active anti-roll moment control at the axle level.
The research contributions are:
A novel real-time-capable nonlinear model predictive control (NMPC) implementation for the case study integrated semi-active and active suspension system. The algorithm uses a five-degree-of-freedom internal model considering tyre nonlinearities, jacking forces, and anti-features of the suspension geometry, which enable meaningful model-based control of the pitch and heave dynamics induced by the driving actions. The NMPC benefits from the inclusion of a cost function weight adaptation mechanism to vary the control priorities based on the driving conditions. The NMPC architecture is modular, i.e. it can be interfaced with external control functions, in this specific case a skyhook controller targeting the compensation of the effect of road irregularities.
A performance comparison of the proposed NMPCs, excluding and including weight adaptation, with benchmarking active roll moment and skyhook damping controllers, referred to as pseudo-inverse and inverse, depending on the involved decoupling transformation used to calculate the reference damping forces in the skyhook equations (as detailed in Section 4.5). The assessment is carried out through simulations with an experimentally validated vehicle model.
The remainder is organised as follows: Section 2 summarises the literature, and identifies the knowledge gap addressed by this study; Section 3 presents the simulation framework and control architecture; Section 4 describes the novel NMPC and benchmarking implementations; Section 5 discusses the simulated manoeuvres and considered performance indicators; Section 6 analyses the simulation results; finally, Section 7 summarises the main conclusions.
2. Literature review
Controllable suspensions are widely discussed in the literature, mostly to: i. mitigate the motion of the sprung mass caused by road irregularities; ii. reduce the vertical tyre load oscillations on irregular surfaces, and thus improve road holding; and iii. compensate the heave, pitch and roll motions of the sprung mass, excited by the driving actions, i.e. by traction/braking and cornering.
In many implementations targeting i.-iii., e.g. [Citation5–11], a high-level algorithm calculates the total reference heave force at the centre of gravity, as well as the reference anti-pitch and anti-roll moments for the sprung mass. These are then converted into individual forces for the four actuators at the vehicle corners, through the so-called decoupling transformation, often based on a pseudo-inverse control allocation matrix. To achieve iii., the integrated chassis controller (ICC) in [Citation12] uses a low-level control allocation algorithm that accounts for the anti-properties of the suspension system. Body motion control can also be implemented through algorithms directly generating the actuator control inputs, see the roll controllers in [Citation13–15], the linear quadratic regulator (LQR) for heave, pitch and roll control in [Citation16], and the LQRs and robust roll controllers in [Citation17].
In [Citation4] and [Citation18–33], the controlled suspensions enhance the vehicle yaw rate response (objective iv. of controllable suspension systems) through variable front-to-total anti-roll moment distribution. In fact, for a given lateral acceleration, increased front anti-roll moment and decreased rear anti-roll moment increase the magnitude of the front slip angle and reduce the rear one, thus making the vehicle more understeering. On the one hand, because of the nonlinearity of the phenomenon, many front-to-total anti-roll moment distribution controllers are not model-based, see [Citation18–26]. On the other hand, most of the available model-based anti-roll moment distribution designs use simplified vehicle models including lateral and yaw dynamics, where the lateral tyre force is linearly varying with the slip angle, and the cornering stiffness is parabolically dependent on the vertical tyre load, e.g. see [Citation27–32]. Reference [Citation33] combines the parabolic cornering stiffness variation with vertical load and a simplified version of the Pacejka magic formula. In [Citation4] and [Citation34] Ricco et al. highlight the significant limitations of the model in [Citation27], propose a linearised model for front-to-total anti-roll moment distribution design in the frequency domain, and highlight the resulting performance benefits in limit handling conditions.
A recent trend is the implementation of model predictive controllers (MPCs) for suspension control, whose real-time operation is made possible by the increased computational capability of automotive microcontrollers, and the effectiveness of real-time optimisation solvers [Citation35]. For example, the MPC in [Citation36] addresses the heave, pitch and roll dynamics of the sprung mass induced by unknown road disturbances, while in [Citation37] Shao et al. evaluate a distributed MPC for heave and pitch control on irregular roads. The road profile is considered a known input in the road preview controllers in [Citation38–44], which target i., and, depending on the application, the improvement of other aspects, e.g. ii., while considering actuation effort and constraints. However, these MPCs neglect the vehicle cornering dynamics, and do not include tangential tyre force formulations in their prediction models. In [Citation45] Zhu et al. propose a cascade control structure, with an upper level MPC that uses the simplified tyre model from [Citation27] to reduce the roll motion and improve the yaw rate response. In [Citation46] Adireddy et al. present an MPC-based ICC with an 8-degree-of-freedom prediction model, which neglects the heave and pitch dynamics, similarly to the real-time NMPC in [Citation47]. Reference [Citation48] shows the potential of linear time-varying MPC for ICC in the linear cornering response region, with maximum lateral accelerations of ∼4 m/s2, in which controlled suspensions have very limited impact on vehicle dynamics. Similarly, the NMPC-based ICC in [Citation49] is tested at low lateral accelerations, and the active suspension system enhances only ride comfort.
In conclusion, a real-time-capable MPC architecture that integrates the compensation of the sprung mass motions caused by the driving actions, ride comfort enhancement, and yaw rate control for operation at the limit of handling is still missing. Moreover, the available prediction model formulations for front-to-total anti-roll moment distribution and vehicle dynamics control neglect the anti-lift, -squat and -dive properties of the suspension system, as well as the jacking force effects. The identified gaps will be addressed in the remainder.
3. Case study vehicle and simulation framework
3.1. Reference vehicle and suspension actuation system
The case study vehicle is an Audi E-Tron prototype (see its main parameters in Table ) equipped with: i) two on-board centralised electric motors, one per axle, each of them connected to the wheels through a single-speed transmission, mechanical differential, half-shafts, and constant velocity joints; and ii) the novel integrated CVSA2-Kinetic suspension system by Tenneco [Citation50], including four hydraulically interconnected dampers, i.e. one per corner, according to the CVSA2 (continuously variable semi-active suspension solution) damping set-up, in which the hydraulic dampers are equipped with two external electro-hydraulic valves that independently control the damping characteristics for the rebound and compression motions, thus providing a semi-active contribution. The system is upgraded with a Kinetic roll control system, consisting of hydraulic lines between the dampers, replacing the mechanical anti-roll bars, with a pressure control unit including a pump and valves that are used to add an active contribution, to achieve the desired front and rear anti-roll moments. As a consequence, the force of each damper is given by the superposition of the independent damping from CVSA2 and the active contributions from the pump of the Kinetic system. The suspension controller therefore generates six inputs, i.e. four control currents for semi-active control, and two reference anti-roll moments for active roll control. The active anti-roll moments are expressed through active force contributions, which are constrained by the hydraulic arrangement to be equal and opposite for the hydraulic dampers on the same axle. The reference active forces are sent to the low-level controller of the actuation system, developed by Tenneco, which generates the expected actuation for the involved valves and pump, and whose detailed operation is beyond the scope of this study.
Table 1 Main vehicle parameters.
3.2. Simulation framework
The simulation framework, see Figure , consists of the following functional blocks:
A virtual driver model, which generates the accelerator pedal position,
, brake pedal position,
, and steering wheel angle,
. For tracking the reference speed profiles, proportional integral (PI) controllers are used to output the desired pedal positions, while the
profiles are directly generated with fixed look-up tables of
as a function of time, without a feedback contribution.
A drivability layer, which outputs the individual electric motor and braking torque levels,
and
, where the subscript
indicates the front or rear axles, and the subscript
indicates the left or right sides.
A reference generator, which defines the reference yaw, roll, pitch and heave rates (respectively
, see [Citation4] for its definition,
,
, and
), as well the reference anti-roll moment, anti-pitch moment, and anti-heave force (
,
, and
), starting from the driver steering input,
, and the measured/estimated signals, such as vehicle speed,
, and the variables in the state vector,
.
A vehicle stability controller (VSC) [Citation51,Citation52], based on the combination of heuristic rules and proportional integral derivative (PID) controllers. Emergency conditions are identified through the magnitude of the yaw rate error, i.e. the VSC is activated based on thresholds that are different depending on the understeering or oversteering condition of the vehicle, computed with respect to (w.r.t.)
. The VSC algorithm specifies the: a) variation of the total longitudinal tyre force, e.g. the powertrain torque is brought down to zero independently from the driver input on the accelerator pedal, and the brake force demand can be increased or reduced w.r.t. the driver request; and/or b) generation of a direct yaw moment, through PID controllers with different tunings depending on the understeering or oversteering condition of the vehicle, according to the current industrial practice in VSC design. The PID outputs are saturated – including appropriate anti-windup – based on the estimated friction level on the individual corners, see [Citation53]. a) and b) are achieved through the actuation of the friction brakes, where b) is generated mainly on the inner rear or outer front corners, up to their respective saturation levels. Once tyre force saturation is reached, also the other tyre located on the same vehicle side is actuated. The VSC outputs are the modified motor and braking torque levels,
and
, the latter to be actuated by a brake-by-wire system.
The proposed NMPC (see Sections 4.1–4.4), which outputs the reference value of the variation
of the front-to-total anti-roll moment distribution ratio for the active part of the suspension system w.r.t. its nominal value,
, as well as the current levels,
, for the valves of the controllable dampers. The
values are computed from the reference currents,
, generated by the benchmarking inverse skyhook algorithm, targeting ride comfort enhancement. Further damping control functions, external to the NMPC, can be integrated in the architecture, by summing the respective reference currents before they are sent to the NMPC. The
levels deviate from the externally generated values, to consider the NMPC objectives in terms of cornering and body motion control, while embedding actuation constraints.
The front-to-total anti-roll moment distribution ratio function, which calculates the total active and semi-active anti-roll moment distribution factors,
and
, for the benchmarking inverse controller.
The benchmarking pseudo-inverse and inverse damping force controllers (see Section 4.5), which generate the reference damping forces,
, starting from
,
,
, and
.
The actuation algorithm for the benchmarking skyhook damping controllers, developed by the involved suspension component supplier, which calculates
starting from
and the measured actuator speeds.
The active suspension force distributor, which calculates the total active anti-roll moment starting from the lateral acceleration
, and, by combining it with the
value from the NMPC or the benchmarking inverse controller, generates the reference active suspension forces,
.
The high-fidelity vehicle simulation model for control system assessment, implemented via the software package VSM by AVL [Citation54], including consideration of the actuation dynamics of the semi-active and active suspension components, which are approximated through first order models with 20 and 35 ms time constants. The model was experimentally validated through vehicle tests executed in the framework of the European project EVC1000 [Citation55]. Figure reports examples of comparisons of experimental measurements with the model outputs in steady-state and transient cornering conditions (see Figure for the definition of the main variables). The good match makes the model a valid tool for control performance assessment.
Figure 1 Simplified schematic of the simulation environment. The blocks with white background refer to the proposed suspension controllers; the blocks with grey background refer to the vehicle simulation model, and the powertrain and friction brake controllers.

Figure 2 Vehicle model validation results. Top: skidpad test results; bottom: transient steering manoeuvre at approximately constant torque demand, from an initial vehicle speed of ∼100 km/h. ‘Experimental’: vehicle measurements; ‘Simulation’: results from the model for control system assessment; ‘Internal’: results from the NMPC prediction model (see Section 4) running in open loop along the same test.

The block diagram in Figure also includes the switches for activating/de-activating the NMPC, where in the latter case only the benchmarking algorithms are operational.
4. Controller formulations
4.1. NMPC internal model
Model concept
Figure shows the top, rear and side views of the vehicle prediction model concept, also referred to as internal model, with indication of the main variables and sign conventions. The model formulation specifically targets suspension control applications, and considers the lateral and yaw vehicle dynamics, the heave, roll and pitch dynamics of the sprung mass, as well as the anti-features and jacking force effects of the front and rear suspensions. Since the involved industrial company is interested in suspension control only (typical suspension controllers operate independently from VSC systems), and expressed the requirement of limiting the computational load associated with the implicit NMPC, the longitudinal vehicle dynamics and rotational wheel dynamics have been neglected in the prediction model, although they are present in the high fidelity simulation model for control assessment. These dynamics could be included in the internal model of a model predictive controller for vehicle stability control based on the actuation of the friction brakes, e.g. see [Citation56], which is not the topic of this manuscript. Similarly, as the NMPC addresses the body motions induced by the driving actions and yaw rate tracking, and works together with a skyhook algorithm (see Figure ) that compensates the effect of road irregularities, the vertical motions of the unsprung masses have not been included in the internal model. The potential benefit of the specified additional degrees of freedom will be assessed in future research, focused on integrated direct yaw moment and active/semi-active suspension control.
Figure 3 Top, rear and left side views of the vehicle, with indication of the main variables and parameters used in the internal model formulation.

In the side view, the suspension is described through an equivalent swinging arm, connected to the sprung mass by a cylindrical joint, whose position, defined by the vertical and longitudinal distances and
(see details in [Citation57]), can be directly obtained from the geometry of the specific suspension arrangement, or the relevant anti-dive/-lift/-squat percentages. For the computation of the equivalent vertical forces transmitted by the suspension links to the chassis because of the friction braking torques, on the wheel side the joint of the equivalent suspension arm is considered to be located at the centre of the tyre contact patch, as in Figure . On the contrary, for the computation of the vertical suspension link force related to the powertrain torque contribution, the arm is considered connected to the wheel centre, to account for the effect of the reaction torque of the on-board powertrains, which is applied to the sprung mass. Hence, the longitudinal load transfer during traction and braking is split between a component through the equivalent suspension link/s, neglected in the available MPC implementations targeting cornering response enhancement, and a component through the suspension springs and actuators. This feature enables realistic prediction of the pitch and heave dynamics induced by the longitudinal tyre forces.
Similarly, the model accounts for the jacking forces, i.e. the vertical force components induced by the lateral tyre forces through the suspension links in case of non-zero roll centre height, see [Citation58]. The resulting force, caused by the lateral tyre force difference among the two wheels of the same axle, is applied to the roll centre of each suspension. The model neglects the jacking force effects associated with roll axis migration, which will be the object of future analyses.
Model formulation
The prediction model dynamics are described by the following force and moment balance equations, under the assumption of small steering, sideslip and roll angles:
Lateral force balance at the vehicle level
(1)
(1)
Yaw moment balance at the vehicle level
(2)
(2)
Roll moment balance of the sprung mass
(3)
(3)
Pitch moment balance of the sprung mass
(4)
(4)
Heave force balance of the sprung mass
(5)
(5)
The terms are given by:
(7)
(7) where
is the force of the
passive spring;
is the virtual damping force of the
suspension actuator;
is the virtual active force provided by the actuators on the
axle, considered to be equal and opposite on the two vehicle sides, to match the characteristics of the considered electro-hydraulic hardware, and to generate the expected anti-roll moment; and
and
are the installation ratios – approximated by constant values – of the suspension springs and actuators, where, based on (7), the latter generate a total force given by
. The passive spring force is modelled as:
(8)
(8) where
is the linear stiffness of the
passive spring, and
is the respective displacement, defined as:
(9)
(9) Within the prediction model, a continuous formulation, derived through curve fitting, approximates
as a function of the relative actuator speed,
, calculated with the time derivative of an expression equivalent to (9), and the current level of the relevant damping valve,
:
(10)
(10) (10) provides a good approximation of the experimental behaviour of the available damping actuation hardware, see Figure .
Figure 4 Static front and rear damping force characteristics of the case study actuators, as functions of actuator speed, for three current levels (minimum, medium and maximum). Comparison between experimental measurement points, indicated by the dots, on an actuator test rig, and the NMPC formulation in (10).

The total active force contribution is designed to ensure a desired level of roll angle compensation. The total roll moment associated with a lateral acceleration is approximately given by
. The active suspension force contribution targets a level of roll moment compensation defined by the gain
, while distributing the active anti-roll moments between the front and rear axles according to the front-to-total distribution ratio
, which is used to control the cornering response. Hence,
and
are expressed as:
(11)
(11) where
is the lateral acceleration measured by the inertial measurement unit (IMU) installed on the vehicle; and
is the nominal front-to-total active anti-roll moment ratio.
was set to 0.64, a value for which the cornering response is a desirable trade-off between steady-state and transient manoeuvring requirements, and is aligned to that of the passive version of the same vehicle with mechanical anti-roll bars.
Under reasonable simplifications, the resulting vertical forces applied by the equivalent rigid links to the vehicle body, , are given by a moment balance about the centre of the tyre contact patch:
(12)
(12) where
is obtained from the electric motor and friction brake torques,
and
, and the inertial force of the unsprung mass;
is the longitudinal acceleration measured by the IMU;
is the unsprung mass of a corner of the
axle; and
is the transmission gear ratio. In (12) the term
, where
is the longitudinal traction force caused by the powertrain, represents the effect of the reaction torque of the on-board powertrain on the sprung mass, while the moment
is neglected in the implementation. Similarly, the suspension jacking forces are approximated as:
(13)
(13) where
is the externally estimated lateral tyre force, considered constant along the prediction horizon, for decreasing the computational load.
The lateral tyre forces, , which vary along the prediction horizon in (1) and (2), are computed through the version 5.2 of the magic formula as a function of the axle slip angle
, vertical tyre load
, tyre slip ratio
, camber angle
(its static value was used in the simulations), and tyre-road friction factor
, where the subscript ‘est’ indicates estimated variables that are considered to remain constant along the prediction horizon, for computational efficiency:
(14)
(14) As the tyre model considers the effect of the interaction between the longitudinal and lateral slips [Citation59], the formulation is suitable for modelling the vehicle response at and beyond the limit of handling.
is expressed through the following linearised formulations:
(15)
(15) where
is the average front steering angle. With the maximum slip angle magnitude seen in the broad range of test scenarios considered in Section 4 being only ∼10 deg, the approximation in (15) holds, which alleviates the computational load. The vertical tyre load,
, is calculated as the sum of the static load
, the spring and actuator force
, the vertical force components through the rigid links,
and
, and the load transfers,
, associated with the unsprung mass:
(16)
(16) where the static loads are:
(17)
(17) and the
terms are given by:
(18)
(18) where
is the centre of gravity height of the respective unsprung mass.
The internal NMPC model is expressed through the following nonlinear continuous time formulation, based on the re-arrangement of (1)–(18):
(19)
(19) where the state vector,
, is:
(20)
(20) and the control input vector,
, which includes the control actions, is:
(21)
(21) The good match between the internal model running in open-loop and the experimental results in Figure (Section 3.2) confirms the validity of the adopted assumptions.
In the following NMPC implementation, the current values of the states in (20) are used as initial conditions for the prediction. In particular, in a real vehicle application, ,
, and
are directly measured by the IMU;
,
,
and
are inferred from the fusion of the displacement and speed measurements of the suspension actuators at the individual corners, according to the production algorithms already implemented by the involved industrial company; and
is estimated through sensor fusion, e.g. see the broad set of options in [Citation60–65].
4.2. Optimal control problem formulation
NMPC uses a model of the plant to predict and optimise the future behaviour of the system. The control action is obtained by solving, at each sampling time, a finite horizon optimal control problem, using the current value of the states. The optimisation yields an optimal control sequence, whose first element is applied to the plant, according to the receding horizon approach [Citation66].
The proposed NMPC minimises the cost function , subject to constraints, according to:
(22)
(22) where
is the number of steps of the prediction horizon
, in this implementation equal to the control horizon
, i.e.
, with
being the discretization time;
is the discretization step;
and
are the lower and upper limits for
;
and
are the lower and upper limits for
;
is the discretised version of the model in (19);
is the terminal cost; and
is the stage cost for each time step, defined as a least-squares function:
(23)
(23) where
,
,
,
,
, and
are constant non-dimensionalisation factors, corresponding to the expected maximum value of the respective variable in the most critical conditions; and
,
,
,
,
,
, and
are the cost function weights, respectively prioritising yaw rate error minimisation, roll rate reduction, pitch rate reduction, heave rate reduction, and control effort penalisation in terms of deviation of the damping valve currents and anti-roll moment distribution from their nominal values. The reference heave, pitch and roll rates for the sprung mass are set to zero. The reference currents,
, are calculated through a pre-existing centralised skyhook formulation, see (29) in Section 4.5, which accounts for the front-to-total ratio,
, computed from the damping force distribution output by the NMPC at the previous time step. More specifically, the skyhook forces from (29) are sent to inverse look-up tables corresponding to Figure , which generate
. The skyhook algorithm: a) enables the compensation of the effect of road irregularities, which is not a priority of the proposed NMPC formulation, and is already effectively carried out by the controller available at Tenneco Automotive. The NMPC-induced deviations from the reference skyhook currents address the body motions induced by the longitudinal and lateral accelerations, and the yaw rate and sideslip angle response; b) highlights the capability of the architecture to incorporate contributions from independent control functions, according to the current industrial practice in automotive suspension control; and c) steers the numerical optimisation of the implicit NMPC towards reasonable values, from which the controller can deviate.
The following constraints have been implemented in (22), to consider the actuation hardware capabilities:
Limitation on the individual current levels, i.e.
, with
0.32 A and
1.6 A.
Limitation on the variation of the front-to-total anti-roll moment distribution ratio w.r.t. the nominal value, i.e.
, with
0.2 and
0.8.
Limitation on the active force contributions, i.e.
, with
7 kN and
7 kN.
4.3. Weight adaptation
Through (23), the NMPC incorporates different objectives in the same cost function, e.g. body control and cornering response enhancement. To maximise effectiveness, the controller can adapt its priorities to the driving scenarios by modifying its weights, e.g. to focus on body control during normal driving, and on yaw rate tracking in limit handling. Following extensive testing, the lateral acceleration magnitude, , was selected as the weight scheduling variable, which is continuously modified. In fact, the effect of the anti-roll moment distribution on the cornering response is directly related to the lateral load transfers, which are proportional to
.
A simulation-based brute force algorithm was used to heuristically calibrate the NMPC weights for high tyre-road friction conditions, while considering a set of manoeuvres, each of them designed to target only low, medium or high . A calibration cost function,
, was minimised for each manoeuvre (see their list in the following Section 5), while producing a vehicle response that is free of any unwanted oscillations.
, with
1,..,3, is the weighted linear combination of the root mean square (‘
’ in the subscripts) values of the yaw rate error, and roll, pitch and heave rates:
(24)
(24) where:
(25)
(25) with
and
being the initial and final times of the relevant part of the manoeuvre; and
,
,
and
being normalisation factors, defined as the values of the indicators for the passive vehicle, obtained along test scenario 5 in Section 5. In (24) the weights define the relative significance of the body control
indicators and handling indicator
. Based on the experience of the involved industrial partner, three sets of weights, defined by
, were used, depending on the nature of the considered test scenario, to: i) prioritise body control in non-critical vehicle dynamics conditions, which corresponds to the cost function
; ii) achieve a balance between body control and vehicle dynamics in manoeuvres with significant lateral accelerations, although well within the cornering limit, which corresponds to
; and iii) prioritise yaw rate tracking in limit handling operation, evaluated through
.
Therefore, the three sets of weights were chosen through the aforementioned calibration method; more specifically, referring to the test scenarios defined in Section 5: i) test scenario 3 was used for selecting the weights below a lateral acceleration magnitude of 3.5 m/s2, where such value approximately corresponds to the maximum in this manoeuvre, for which the objective was to minimise the cost function
; ii) test scenario 4 was used for optimising the weights in the 3.5–6 m/s2 lateral acceleration range, typical of this test, with the objective to minimise
; and iii) test scenario 5 was used for the weights above 6 m/s2, as
exceeds 10 m/s2 in this manoeuvre, with the objective of minimising
In the online implementation, the weights are organised into maps, and vary linearly between the three sets of calibrated data to simplify the scheme and minimise the computational requirements, see Figure (
,
and
are not reported as they are constant). Whilst the increase in
with
is self-explanatory due to the prioritisation of yaw rate tracking during significant cornering, it was also found that for a given
value, an increase in
with
tends to further reduce
, because of the impact of the pitch rate on the longitudinal load transfer dynamics. The resulting maps were tested with excellent results across the test scenarios in Section 5. Future developments will evaluate more complex weight scheduling schemes.
4.4. Real-time implementation of the NMPC algorithm
The proposed NMPC was implemented in implicit form via the ACADO toolkit [Citation35], which offers a powerful interface for NMPC development, with the following settings: Gauss Newton Hessian approximation, multiple shooting discretisation, fourth order implicit Runge Kutta integrator, and qpOASES solver. The qpOASES solver, see its details in [Citation67], is responsible for solving the optimal control problem of the NMPC as described in Section 4.2. It efficiently finds the optimal values of the control actions, as per (21), at each time step, to minimise the cost function in (22), while meeting the constraints outlined at the end of Section 4.2.
The controller sampling time, , and number of optimisation steps,
, were set to 11 ms and 2, corresponding to a 22 ms prediction horizon. The number of iterations of the optimiser was set to 1, to ensure computational efficiency. Diminishing performance improvements were found with increasing
, and given that the execution time increases with increasing
, a low value of
was used to ensure the real-time implementability of the controller, see Section 6 for the sensitivity analysis on
. The discretisation time of the internal model is 1 ms, which ensures its numerical stability without significantly affecting the computational time.
The indicated parametrisations enable the controller to run in real-time with good margin on the dSPACE MicroAutoBox II system (900 MHz, 16 Mb flash memory) in Figure . For example, during a sinusoidal steering manoeuvre at the limit of the handling, a maximum execution time of 6.8 ms was achieved.
4.5. Skyhook controllers
The benchmarking skyhook controllers use pseudoinverse and inverse formulations for computing the damping forces at the individual corners. The equivalent reference heave damping force at the centre of gravity of the sprung mass and the reference pitch and roll damping moments, respectively ,
and
, are computed as functions of the estimated or measured
,
, and
, through nonlinear look-up tables, see Figure , according to a skyhook approach based on the ride comfort and body motion control criteria deriving from the experience of Tenneco Automotive in production suspension controllers.
Figure 7 Nonlinear look-up tables for the computation of the reference heave force, and pitch and roll moments.

,
and
can be expressed as functions of the damping forces at the corners:
(26)
(26)
Hence, in the pseudoinverse case, is computed through a right pseudoinverse transformation of (26) as:
(27)
(27) with appropriate damping force saturations, functions of the individual actuator speeds, being imposed on the outputs of (27). In the pseudoinverse controller, which corresponds to the conventional suspension control implementation commercially proposed by the involved industrial partner, the active forces are obtained through (11) by imposing
, i.e. the active anti-roll moment contribution is only used for roll angle compensation.
In the second benchmarking controller, i.e. the so-called inverse controller, an additional condition, on top of those in (26), is imposed, to account for the desirable front-to-total damping moment distribution :
(28)
(28) where
is used to enhance vehicle dynamics in transient cornering conditions. If the inverse controller is implemented in isolation,
is obtained as the sum of a nominal value and a contribution proportional to the yaw rate error. If the inverse controller operates in conjunction with the NMPC,
is equal to the resulting value from the NMPC at the previous time step. By adding (28) to (26), the system of equations includes four conditions to be achieved through four actuators, and therefore the damper forces are calculated through an inverse formulation:
(29)
(29) The inverse formulation of the skyhook damping force contribution is coupled with an active contribution using an anti-roll moment distribution calculated through a PI controller of the yaw rate error, see the state-of-the-art design methodology in [Citation4] and [Citation34]. To prevent undesired oscillations during normal driving, the yaw rate based variations of
and
are progressively activated when
exceeds 0.4 g, and are fully activated for
0.6 g [Citation4].
5. Considered configurations, manoeuvres and performance indicators
The simulation analysis in Section 6, using the model for control system assessment in Section 3, compares the following vehicle and controller configurations:
Passive: the case study vehicle without controllable dampers and active roll control, but including conventional passive anti-roll bars.
Pseudoinverse: the controlled vehicle, i.e. without anti-roll bars, with the damper forces computed through (27), and using a constant active anti-roll moment distribution ratio, equal to
.
Inverse: the controlled vehicle with the damper forces computed through (29), and active anti-roll moment distribution ratio from a PI controller.
NMPC1: the NMPC formulation in (1)-(23), with constant weights in the cost function in (22)-(23).
NMPC2: the proposed NMPC including the heuristic weight adaptation in Section 4.3.
The following transient manoeuvres are simulated:
Test scenario 1: a straight line manoeuvre at the constant speed
50 km/h, on an experimentally available ride comfort road profile used by the involved company. This scenario is introduced to show that the additional functions of the NMPC architecture – which focuses on vehicle dynamics – do not compromise ride comfort w.r.t. the benchmarking skyhook controllers.
Test scenario 2: a straight line hard braking test with an average deceleration of 9.3 m/s2, on the verge of the anti-lock braking system (ABS) intervention, from a vehicle speed of 110 km/h, in high tyre-road friction conditions, i.e. with a friction factor
1. The manoeuvre allows the assessment of the reduction – brought by the controlled suspension configurations – of the pitch and heave rates during the initial brake application and final brake release. As the test does not involve the ABS intervention, and the vehicle speed profile is an external input to be tracked by the driver model, the longitudinal vehicle dynamics are the same for all configurations, and the slip ratio behaviour can be excluded from the comparison.
Test scenario 3: a sinusoidal steering test with a maximum steering wheel angle amplitude,
, of 30 deg, starting from a vehicle speed of 80 km/h, in high tyre-road friction conditions. The torque demand is constant and equal to the value required to keep the vehicle at the initial speed in straight line conditions, i.e.
decreases during the test. This also applies to test scenarios 4, 5, and 6. The focus is the assessment of the reduction of the roll, pitch and heave rates through the semi-active system, and the roll angle peaks through the active anti-roll moment.
Test scenario 4: a sinusoidal steering test with
70 deg, starting from
80 km/h, for
1. The target is to achieve a good trade-off between handling and body control.
Test scenario 5: the same as scenario 3, apart from
150 deg, and the fact that the simulations are run for both
1 and
0.75, on a flat road profile, and on the same road profile as in test scenario 1. The main objective is vehicle stabilisation through yaw rate tracking.
Test scenario 6: a sine-with-dwell test with
150 deg, starting from a vehicle speed of 82 km/h in high friction conditions, with the same objective as scenario 5.
, which accounts for the vehicle cornering performance.
, i.e. the maximum rear axle sideslip angle magnitude, which is a cornering stability indicator.
, given by:
(30)
(30) which assesses the magnitude of the total lateral load transfer.
,
,
, as well the maximum roll angle magnitude,
, assessing the body control quality.
, which assesses the overall performance in cornering conditions. In particular, test scenario 3 is evaluated through
; scenario 4 through
; and scenarios 5 and 6 through
.
and
, which assess ride comfort on irregular roads:
(31)
(31)
6. Simulation results
Table in the Appendix reports the performance indicator values for test scenarios 3–6 (scenarios 1 and 2 are trivial given the absence of cornering). For all manoeuvres, is the lowest for NMPC2, which by itself provides a clear indication on the best configuration. A more detailed analysis is included in the following subsections.
Test scenario 1: straight ride comfort road at constant speed. In this test, the lateral acceleration is negligible, and therefore the performance is only determined by the semi-active contributions. Figure (a) shows a sample of the time history of , and highlights the benefits of the pseudoinverse, inverse and NMPC2 configurations w.r.t. the passive vehicle, particularly at the peak at ∼1 s, when the heave acceleration is reduced from almost 6 to 2.5 m/s2. Figure (b) and (c) report
and
, and their percentage variations,
and
, w.r.t. the passive configuration (the symbol ‘
’ will be used with the same meaning in the remainder). Overall, NMPC2 brings a similar percentage reduction to the benchmarking controllers, and outperforms all other cases in terms of
, with a > 25% reduction w.r.t. the passive configuration.
Figure 8 Summary plots for test scenario 1. (a) time histories of the heave acceleration ; (b) values of
and
; and (c) values of
and
, i.e. the percentage variations of the performance indicators w.r.t. the passive case.

Test scenario 2: hard braking test. Given that also in this scenario only the damping force contributions are influential, the benefits are visible only during the transients occurring after 0.5 and 3.5 s, corresponding to the initial brake input application and final brake pressure release, see the profiles of ,
, and
in Figure (a). While the benchmarking controllers only marginally reduce the peak values of
and
, the NMPCs bring a significant decrease.
Figure 9 Summary plots for test scenario 2. (a) time histories of the vehicle speed , pitch rate
, and heave rate
; (b) values of
and
; and (c) values of
and
, i.e. the percentage variations of the performance indicators w.r.t. the passive case.

Similar trends are visible in the histograms in Figure (b)-(c), reporting , which is the most relevant indicator in this scenario, and
, together with their percentage variations,
and
, amounting to ∼19% for
for the pseudoinverse and inverse cases, and exceeding 28% for the NMPCs.
Test scenarios 3 and 4: sinusoidal steering tests with low and medium steering amplitudes. Test scenario 3 implies maximum values of of ∼3 m/s2, for which only body control – and especially roll control – is relevant. The active roll control contribution, which has the same total anti-roll moment control law in all configurations, nearly halves the roll angle peaks in Figure (a), according to the compensation level defined by
. Moreover, all controllers bring
reductions in excess of 45% (Figure (b)), and show substantially equivalent performance, see
, with a very marginal penalty for NMPC1, since the benchmarking skyhook controllers have their damping terms specifically optimised for body control, similarly to the adaptive cost function weights of NMPC2, while NMPC1 uses a trade-off calibration to meet the requirements for the whole operating range.
Figure 10 Summary plots for test scenario 3 ((a) time histories of roll angle , roll rate
, pitch angle
, and pitch rate
; and (b) values of
,
,
, and
); and for test scenario 4 ((c) values of
,
,
, and
).

In test scenario 4, the car experiences a maximum value of of ∼6 m/s2, which is beyond the range of typical driving conditions, but still well within the cornering limit. Therefore, the results, see Table and Figure (c), are similar to those in scenario 3. Interestingly, the improved roll dynamics and consequent marginally reduced peaks of lateral load transfer bring an enhancement of the cornering response of all controlled configurations w.r.t. the passive vehicle. This is associated with a reduction of
, symptomatic of vehicle stability improvement, which is slightly more moderate (∼20%) for the pseudoinverse configuration, and the highest for the NMPCs. Like in all scenarios, NMPC2 provides the highest reduction (∼43%) of the relevant cost function (
), and in the specific manoeuvre is followed by the inverse controller.
Test scenarios 5 and 6: sinusoidal steering and sine-with-dwell tests at the limit of handling. Figure reports the profiles of the main variables during test scenario 5, which is critical in terms of vehicle stability, since the passive configuration has ,
, and
values respectively exceeding 15 deg/s, 10 deg, and 6 deg. The pseudoinverse controller guarantees good body control performance, with a 3.6 deg peak value of roll angle, and ∼47%
reduction; however, the yaw rate errors and maximum rear sideslip angles, respectively ∼12 deg/s and ∼9 deg, are still well beyond acceptable thresholds. The configurations with controllable front-to-total anti-roll moment distribution bring a significant improvement of the cornering response, namely reduced
and
in comparison with the pseudoinverse and passive cases, and enable safe behaviour. In particular, these extreme conditions highlight the superior performance of the proposed NMPCs, which, for example, results in a substantial 13% decrease of
and
, and a 3% reduction of
for NMPC2 w.r.t. the state-of-the-art inverse controller. For completeness, Figure shows the NMPC2 weight profiles along the manoeuvre, which prioritise yaw rate tracking at high lateral accelerations, according to the adaption mechanism of Section 4.3, tailoring performance to the driving scenario. The trends remain the same when performing the manoeuvre on the irregular road profile, see Table 2.
Figure 11 Summary plots for test scenario 5 (VSC de-activated): (a) time histories of lateral acceleration , yaw rate
, rear axle sideslip angle
, roll angle
, roll rate
, pitch rate
, corner damping forces
, and active axle forces
; (b) values of
,
, and
; and (c) values of
,
,
, and
.

As a robustness check, the test scenario 5 simulations were repeated by imposing 0.75 in the model for control system assessment, but without modifying any controller parameters, i.e. by leaving the tyre-road friction factor set to 1 in the internal models of the NMPCs, and for computing the reference yaw rate. Despite this, the NMPC performance remains safe, and clearly exceeds the one of the passive configuration as well as those of the benchmarking skyhook controllers, e.g. see the reduction of the peaks of yaw rate and rear sideslip angle in Figure (a), and the performance indicators in Figure (b)-(c). When also the VSC system is active, see Figure (d)–(f), the direct yaw moment actuation brings a major reduction of the magnitude of the oscillations and overshoots, especially in terms of sideslip angle, which enhances vehicle stability. Nevertheless, the ranking of the configurations w.r.t. the suspension controllers remains the same as for the cases without VSC, i.e. NMPC2 brings the lowest
value.
Figure 13 Summary plots for test scenario 5 with 0.75, and incorrect tyre-road friction information sent to the NMPCs: in (a), (b) and (c) only the suspension controllers are active, while in (d), (e) and (f) both the suspension controllers and the VSC are active.

Interestingly, based on Table 2, in high tyre-road friction conditions (see also the experimental results in [Citation4]), the controlled suspension system on its own is more effective that the VSC in isolation in controlling the yaw rate and sideslip response. This is caused by: i) the high nonlinearity of the specific tyres at high lateral load transfers; and ii) the continuous operation of the proposed active suspension system, whereas the VSC intervenes only in case of significant yaw rate errors, and – in the specific tuning of this paper – not very intrusively, since the brake interventions are clearly perceived by the driver and passengers. In case of reduced , because of the decreased lateral load transfer, the suspension on its own is not as effective as in high tyre-road friction conditions, nevertheless it supports the VSC.
For a fair assessment of the results, it must be considered that the active suspension effect on the cornering response is very dependent on the nonlinearity level of the lateral tyre force with respect to the vertical tyre load, i.e. the benefit is caused by the fact that tyre performance decreases more on the inner corner than it increases on the outer corner of the same axle, because of the lateral load transfers. Therefore, the design and tuning of the active suspension contribution to enhance the cornering response is very subtle (see [Citation4]) and reliant of the properties of the individual tyre makes, which can change during the vehicle life span, e.g. when the tyres are replaced with different ones. On the contrary, the reference direct yaw moment output by a VSC can be predictably generated, rather independently from the installed tyres, up to the tyre saturation level.
Given the intrinsic reliance of the NMPC implementations on their internal model, controller robustness was also evaluated by varying, within the model for control system assessment: i) the tyre cornering stiffness (by up to ±20%), achieved through the calibration of the respective magic formula scaling factor; and ii) the vehicle mass and yaw mass moment of inertia, which were increased by up to 20%. In the meantime, all controller parameters – including those subject to variations in the sensitivity analysis – were kept unchanged. The results are in Figure (a). For both i) and ii) the cornering dynamics variation is more significant for the pseudoinverse and inverse configurations than for the NMPCs, which provide consistently better results than the benchmarking implementations. Moreover, for NMPC2, Figure (b) reports the sensitivity of
and
to the number of prediction steps
. A moderate increase of
brings a marginal performance improvement, at the price of increased computational effort, which compromises the real-time implementability on the available control hardware, see Section 4.4.
Figure 14 Sensitivity analysis of the performance, expressed by and
along test scenario 5, of: (a) the proposed controllers to vehicle parameter variations; and (b) NMPC2 to the number of prediction steps N.

The trends are confirmed by test scenario 6, where the ,
and
reductions w.r.t. the passive vehicle range from 20% for the pseudoinverse configuration to >60% for NMPC2.
7. Conclusions
This study presented two novel real-time-capable NMPC implementations for combined semi-active and active suspension control, tailored to a new hydraulic actuation system developed by Tenneco Automotive. The algorithms target the compensation of the body motions induced by the driving actions, and cornering response enhancement. They are based on nonlinear 5-degree-of-freedom vehicle models, including consideration of tyre slip behaviour as well as suspension anti-properties and jacking force effects. The NMPCs can be integrated with other algorithms, based on different control technologies, targeting complementary aspects, e.g. ride comfort enhancement on irregular roads. The novel controllers were compared with: a) a benchmarking skyhook formulation using a pseudoinverse transformation, combined with an active roll control contribution with fixed front-to-rear moment distribution, corresponding to the current level of implementation on production vehicles; and b) a second skyhook controller, i.e. the so-called inverse formulation, coupled with a front-to-total anti-roll moment distribution algorithm for yaw rate control, the latter designed through a recently published state-of-the-art linear model-based methodology.
The simulation analysis, using an experimentally validated model of a case study electric vehicle, brings the following conclusions:
The NMPCs enhance the level of body control during acceleration and braking transients, covered by test scenario 2, with >28% reduction of the root mean square value of the pitch rate (
) w.r.t. the passive configuration, against the ∼19% decrease brought by the benchmarking controllers.
In transient cornering at low-to-medium lateral acceleration levels, corresponding to test scenarios 3 and 4, the NMPCs and skyhook controllers tend to provide rather similar body control performance, with a marginal benefit for the NMPC formulation with weight adaptation (NMPC2), and a reduction (e.g. ≥ 20% in scenario 4) of the peak values of the rear axle sideslip angle (
).
During limit handling operation, see test scenarios 5 and 6, all controllers with front-to-total anti-roll moment distribution capability bring a major enhancement in the cornering response and active safety, e.g. with >65% and >50% reductions of the root mean square value of the yaw rate error,
, and
. Importantly, the NMPCs enable better performance than the inverse skyhook controller both in terms of vehicle dynamics and body control, e.g. see the 13% decrease of
and
, and the 14% and 8% decrements of the peak values of roll angle magnitude (
) and root mean square roll rate (
) for NMPC2 and NMPC1 in test scenario 5. The trends are confirmed also in case of VSC activation.
Despite embedding a detailed vehicle model in their online algorithms, both NMPCs provide higher robustness than the benchmarking controllers w.r.t. significant parameter variations, namely of vehicle mass and yaw mass moment of inertia, tyre cornering stiffness, as well as estimated tyre-road friction factor.
The weight adaptation mechanism of NMPC2 allows this set-up to be the best among the considered suspension configurations in all scenarios, see the values of the cost functions
, without the compromises, typical of the calibration of the other algorithms, related to the different operating conditions and respective control objectives. Also, on irregular road profiles, see test scenario 1, NMPC2 provides comparable ride comfort results to those of the benchmarking skyhook controllers.
Disclosure statement
No potential conflict of interest was reported by the author(s).
References
- Ueno K, Fujibayashi T, Sasaki M, et al. Vehicle control techniques for safety, environmental performance, and ride comfort. Hitachi Rev. 2018;67(1):64–71.
- Saveresi SM, Poussot-Vassal C, Spelta C, et al. Semi-active suspension control for vehicles. Amsterdam: Elsevier; 2010.
- Tseng HE, Hrovat D. State of the art survey: active and semi-active suspension control. Veh: Syst Dyn. 2015;53(7):1034–1062. doi:10.1080/00423114.2015.1037313.
- Ricco M, Zanchetta M, Rizzo GC, et al. On the design of yaw rate control via variable front-to-total anti-roll moment distribution. IEEE Trans Veh Technol. 2020;69(2):1388–1403. doi:10.1109/TVT.2019.2955902.
- Yao J, Taheri S, Tian S, et al. A novel semi-active suspension design based on decoupling skyhook control. J Vibroeng. 2014;16(3):1318–1325.
- Kadir ZA, Hudha K, Jamaluddin H, et al. Active roll control suspension system for improving dynamics performance of passenger vehicle. Proc Int Conf Model Identif Control. 2011: 492–497.
- Fang Z, Shu W, Du D, et al. Semi-active suspension of a full-vehicle model based on double-loop control. Procedia Eng. 2011;16:428–437. doi:10.1016/j.proeng.2011.08.1107.
- Samin PM, Jamaluddin HJ, Rahman RA, et al. Semi-active suspension for ride improvement using stability augmentation system control algorithm. J Mek. 2008;26:86–95.
- Ikenaga S, Lewis FL, Campos J, et al. Active suspension control of ground vehicle based on a full-vehicle model. Am Control Conf. 2000;6:4019–4024.
- Chokor A, Talj R, Doumiati M, et al. A global chassis control system involving active suspensions, direct Yaw control and active front steering. IFAC-PapersOnLine. 2019;52(5):444–451. doi:10.1016/j.ifacol.2019.09.071.
- Na J, Huang Y, Pei Q, et al. Active suspension control of full-car systems without function approximation. IEEE Trans Mechatron. 2020;25(2):779–791. doi:10.1109/TMECH.2019.2962602.
- Lindvai-Soos D, Horn M. New level of vehicle comfort and vehicle stability via utilisation of the suspensions anti-dive and anti-squat geometry. Veh Syst Dyn. 2018;56(7):1002–1027. doi:10.1080/00423114.2017.1378818.
- Fleps-Dezasse M, Büntze T, Svaricek F, et al. LPV feedforward control of semi-active suspensions for improved roll stability. Contr Eng Pract. 2018;78:1–11. doi:10.1016/j.conengprac.2018.06.007.
- Soltani A, Bagheri A, Azadi S. Integrated vehicle dynamics control using semi-active suspension and active braking systems. Proc Inst Mech Eng Part K: J Multi-Body Dyn. 2017;232(3):314–329.doi:10.1177/146441931773318.
- Dahmani H, Pagès O, El Hajjaji A. Observer-based state feedback control for vehicle chassis stability in critical situations. IEEE Trans Contr Syst Tech. 2016;24(2):636–643.doi:10.1109/TCST.2015.2438191.
- Zhao J, Wong PK, Ma X, et al. Chassis integrated control for active suspension, active front steering and direct yaw moment systems using hierarchical strategy. Veh Syst Dyn. 2017;55(1):72–103. doi:10.1080/00423114.2016.1245424.
- Chokor A, Talj R, Doumiati M, et al. Effect of roll motion control on vehicle lateral stability and avoidance. Am Control Conf. 2020: 4868–4875.
- Cooper N, Crolla D, Levesley M. Integration of active suspension and active driveline to ensure stability while improving vehicle dynamics. SAE Tech. Paper 2005-01-0414. 2005.
- Sorniotti A, D’Alfio N. Vehicle dynamics simulation to develop an active roll control system. SAE Tech Paper 2007-01-0828. 2007.
- Her H, Suh J, Yi K. Integrated control of the differential braking, the suspension damping force and the active roll moment for improvement in the agility and the stability. Proc IMechE Part D: J Autom Eng. 2015;229(9):1145–1157. doi:10.1177/0954407014550502
- Her H, Koh Y, Joa E, et al. An integrated control of differential braking, front/rear traction, and active roll moment for limit handling performance. IEEE Trans Veh Tech. 2016;65(6):1071–1078.
- Termous H, Shraim H, Talj R, et al. Coordinated control strategies for active steering, differential braking and active suspension for vehicle stability, handling and safety improvement. Veh Syst Dyn. 2019;57(10):1494–1529. doi:10.1080/00423114.2018.1521001.
- Yan M, Pi D, Li Y, et al. The design of anti-roll moment distribution for dual-channel active stabilizer bar system. Chin Control Decis Conf. 2018: 6301–6308.
- Xu Y, Ahmadian M, Sun R. Improving vehicle lateral stability based on variable stiffness and damping suspension system via MR damper. IEEE Trans Veh Tech. 2014;63(3):1071–1078. doi:10.1109/TVT.2013.2282824.
- Bodie MO, Hac A. Closed loop yaw control of vehicles using magneto-rheological dampers. SAE Tech Paper 2000-01-0107. 2000.
- Lu SB, Li YN, Choi SB, et al. Integrated control on MR vehicle suspension system associated with braking and steering control. Veh Syst Dyn. 2011;49(1-2):361–380. doi:10.1080/00423110903401889.
- Williams DE, Haddad WM. Nonlinear control of roll moment distribution to influence vehicle yaw characteristics. IEEE Trans Control Syst Technol. 1995;3(1):110–116. doi:10.1109/87.370716.
- Williams DE, Haddad WM. Active suspension control to improve vehicle ride and handling. Veh Syst Dyn. 1997;28(1):1–24. doi:10.1080/00423119708969346.
- Yao J, Lv G, Qv M, et al. Lateral stability control based on the roll moment distribution using a semiactive suspension. Proc IMechE Part D: J Automob Eng. 2017;231(12):1627–1639. doi:10.1177/0954407016681386.
- Cho W, Suh J, You SH. Integrated motion control using a semi-active damper system to improve Yaw-roll-pitch motion of a vehicle. IEEE Access. 2021;9:52464–52473. doi:10.1109/ACCESS.2021.3070366.
- Wang J, Wilson DA, Xu W, et al. Integrated vehicle ride and steady-state handling control via active suspensions. Int J Veh Des. 2006;42(3-4):306–327. doi:10.1504/IJVD.2006.010435.
- Wang J, Wilson DA, Xu W, et al. Active suspension control to improve vehicle ride and steady-state handling. 44th IEEE Conference on Decision and Control, and European Control Conference. 2005.
- Lakehal-Ayat M, Diop S, Fenaux E. An improved active suspension yaw rate control. Am Control Conf. 2002.
- Ricco M, Percolla A, Rizzo GC, et al. On the model-based design of front-to-total anti-roll moment distribution controllers for yaw rate tracking. Veh Syst Dyn. 2020;60(2):569–596. doi:10.1080/00423114.2020.1825753.
- Houska B, Ferrau HJ, Diehl M. An auto-generated real-time iteration algorithm for nonlinear MPC in the microsecond range. Automatica (Oxf). 2011;47(10):2279–2285. doi:10.1016/j.automatica.2011.08.020.
- Nguyen M, Canale M, Sename O, et al. A model predictive control approach for semi-active suspension control problem of a full car. IEEE 55th Conference on Decision and Control. 2016.
- Shao S, Zhou H, Liu H. Distributed model predictive control and implementation for vehicle active suspensions. IFAC-PapersOnline. 2018;51:961–966. doi:10.1016/j.ifacol.2018.10.056.
- Ma X, Wong PK, Zhao J, et al. Design and testing of a nonlinear model predictive controller for ride height control of automotive semi-active air suspension systems. IEEE Access. 2018;6:63777–63793. doi:10.1109/ACCESS.2018.2876496.
- Gohrle C, Wagner A, Schindler A, et al. Active suspension controller using MPC based on a full-car model with preview information. Am Control Conf. 2012.
- Gohrle C, Schindler A, Wagner A, et al. Design and vehicle implementation of preview active suspension controllers. IEEE Trans Contr Sys Tech. 2014;22(3):1135–1142. doi:10.1109/TCST.2013.2272342.
- Mai VN, Yoon DS, Choi SB, et al. Explicit model predictive control of semi-active suspension systems with magneto-rheological dampers subject to input constraints. J Intell Material Syst Struct. 2020;31:1157–1170. doi:10.1177/1045389X20914404
- Theunissen J, Sorniotti A, Gruber P, et al. Regionless explicit model predictive control of active suspension systems with preview. IEEE Trans Ind Electr. 2020;67(6). doi:10.1109/TIE.2019.2926056.
- Song S, Wang J. Incremental model predictive control of active suspensions with estimated road preview information from a lead vehicle. J Dyn Syst Meas Contr. 2020;12:142–150. doi:10.1115/1.4047962.
- Jurisch M. Vertical trajectory planning: an optimal control approach for active suspension systems in autonomous vehicles. Veh Syst Dyn. 2022;60(11):3788–3809. doi:10.1080/00423114.2021.1979238.
- Zhu Q, Ayalew B. Predictive roll handling and ride control of vehicles via active suspensions. Am Control Conf. 2014: 2102–2107.
- Adireddy G, Shim T. MPC based integrated chassis control to enhance vehicle handling considering roll stability. ASME Dyn Syst Control Conf. 2011;2:877–884.
- Dalboni M, Tavernini D, Montanaro U, et al. Nonlinear model predictive control for integrated energy-efficient torque-vectoring and anti-roll moment distribution. IEEE/ASME Trans Mechatron. 2021;26(3):1212–1224. doi:10.1109/TMECH.2021.3073476.
- Chang S, Lee B, Park Y, et al. Integrated chassis control for improving on-center handling behavior. SAE Int J Passeng Cars – Mech Syst. 2014;7(3):1002–1008. doi:10.4271/2014-01-0139.
- Wang Q, Zhao Y, Lin F, et al. Integrated control for distributed in-wheel motor drive electric vehicle based on state estimations and nonlinear MPC. Proc IMechE Part D: J Autom Eng. 2022;236(5):893–906. doi:10.1177/09544070211030444.
- Monroe Intelligent Suspension Driv Tenneco Automotive BVBA. https://www.monroeintelligentsuspension.com/electronic/pages/products.php, last accessed on 7 November 2022.
- Van Zanten AT. Bosch ESP systems: 5 years of experience. SAE Trans: J Pass Cars. 2000;109(7):428–436. doi:10.4271/2000-01-1633.
- Shino M, Nagai M. Yaw-moment control of electric vehicle for improving handling and stability. JSAE Rev. 2001;22(4):473–480. doi:10.1016/S0389-4304(01)00130-8.
- Koibuchi K. Behavior control system of vehicle distinctive of oversteered and understeered conditions. Patent US5702165A. 1997.
- https://www.avl.com/documents/10138/2095827/AVL+VSM+4%E2%84%A2+-+Solution+Brochure, last accessed on 18 Aug. 2023.
- Armengaud E, et al. EVC1000–integrated corner solution for innovative electric vehicles. 8th Transp Res Arena (TRA). 2020.
- Metzler M, Tavernini D, Gruber P, et al. On prediction model fidelity in explicit nonlinear model predictive vehicle stability control. IEEE Trans Contr Sys Technol. 2021;29(5):1964–1980. doi:10.1109/TCST.2020.3012683.
- Dixon JC. Suspension geometry and computation. Chichester: Wiley; 2009.
- Gerrard M. Roll centres and jacking forces in independent suspensions – A first principles explanation and a designer’s toolkit. SAE Tech Paper 1999-01-0046. 1999.
- Pacejka H. Tyre and vehicle dynamics. 3rd ed. Amsterdam: Elsevier; 2012.
- Antonov S, Fehn A, Kugi A. Unscented Kalman filter for vehicle state estimation. Veh: Syst Dyn. 2011;49(9):1497–1520. doi:10.1080/00423114.2010.527994.
- Mazzilli V, Ivone D, De Pinto S, et al. On the benefit of smart tyre technology on vehicle state estimation. Veh Syst Dyn. 2022;60(11):3694–3719. doi:10.1080/00423114.2021.1976414.
- Chen BC, Hsieh FC. Sideslip angle estimation using extended Kalman filter. Veh Syst Dyn. 2008;46:353–364. doi:10.1080/00423110801958550.
- Chindamo D, Lenzo B, Gadola M. On the vehicle sideslip angle estimation: a literature review of methods, models, and innovations. App Sc. 2018;8(3):1–20. doi:10.3390/app8030355.
- Melzi S, Sabbioni E. On the vehicle sideslip angle estimation through neural networks: numerical and experimental results. Mech Syst Sign Proc. 2011;25(6):2005–2019. doi:10.1016/j.ymssp.2010.10.015.
- Zhang B, Du H, Lam J, et al. A novel observer design for simultaneous estimation of vehicle steering angle and sideslip angle. IEEE Trans Ind Elec. 2016;63(7):4357–4366. doi:10.1109/TIE.2016.2544244.
- Grüne L, Pannek J. Nonlinear model predictive control. London, Dordrecht Heidelberg, New York: Springer; 2011.
- Ferreau HJ. qpOASES User’s Manual, April 2017, https://www.coin-or.org/qpOASES/doc/3.2/manual.pdf, last accessed on 06 June 2023.
Appendix
Table 2. Performance indicator values for test scenarios 3–6 (different indicators are used for test scenarios 1 and 2). The bold fonts highlight the controller configuration with the lowest cost function value in the respective scenario.