ABSTRACT
Public transport (PT) usage was severely impacted during the COVID-19 pandemic, resulting in up to a 90% reduction in many cities in 2020. Numerous studies have been conducted since then to determine the relationship between individual-level factors (such as gender, attitudes, etc.) and the decrease in PT usage during the pandemic. Despite the evidence provided, findings are dispersed, and for several factors contradictory, making it challenging to reach any generalised conclusion. Furthermore, a comprehensive comparison of the effect sizes among travellers’ factors affecting PT use during this period is yet to be compiled. This paper aims to address these gaps by systematically reviewing the existing evidence and synthesising the effect sizes of travellers’ factors through a meta-analysis. We first identified 36 studies that statistically assessed the contribution of 15 individual-level factors on PT usage during the COVID-19 pandemic. By merging the empirical evidence of those studies, the direction of the association between those factors and PT usage was analysed. Then, after selecting comparable studies, meta-analyses were conducted for each factor to estimate the corresponding pooled effect sizes. The meta-analysis established that car availability, teleworking opportunities and high educational level contributed the most to reducing PT use during the pandemic. These factors increased the odds of reducing PT usage compared with the pre-pandemic by about three times. Factors such as COVID-19 risk perception, gender, high income and health had a moderate effect on the decision to stop using PT. PT habits, travel distance and physical accessibility also influenced PT use during the pandemic. Geographical location and the pandemic period explained part of the heterogeneity found. The findings provided in this study can help policy-makers understand the impacts of travellers’ factors on the decision to reduce PT usage during future pandemics/epidemics and guide public policies accordingly.
Introduction
Public transport (PT) usage was severely affected during the COVID-19 pandemic. Its lowest levels were reached during the outbreak that occurred in the first half of 2020. In this period, PT demand dropped by up to 80%−90% in cities where stay-at-home orders were implemented (Gramsch et al., Citation2022; Teixeira & Lopes, Citation2020). A characterisation of these changes has been provided for cities of different countries, including the US (Liu et al., Citation2020; Wang & Noland, Citation2021; Xiao et al., Citation2022), the UK (Vickerman, Citation2021), Spain (Fernández Pozo et al., Citation2022), Germany (Eisenmann et al., Citation2021), India (Padmakumar & Patil, Citation2022), Chile (Lizana et al., Citation2023), and China (Jiang & Cai, Citation2022), to name some.Footnote1 Even in cities without mandatory restrictions, PT demand experienced drops as high as 60% (Jenelius & Cebecauer, Citation2020; Mützel & Scheiner, Citation2022).
Eventually, PT demand started a slow recovery process after governments gradually removed the most restrictive policies from mid-2020. exemplifies this process, presenting a four-year monthly variation (2019 to 2022) of the PT demand in 11 PT systems worldwide. Regarding this recovery process, most evidence indicates that during 2020 and 2021, PT demand remained substantially below pre-pandemic levels. For example, it was reported by Qi et al. (Citation2023) that as late as January 2021, PT demand still exhibited reductions of between 50% and 80% in the 20 cities they analysed in the US. Gramsch et al. (Citation2022) reported a drop as high as 60% at the end of September 2020 in Chile, a similar relative change to the one reported for Madrid (Fernández Pozo et al., Citation2022). Furthermore, PT demand continued to be lower than pre-pandemic levels even in contexts where successful initial counter-measures against the virus were implemented. Sweden (Jenelius & Cebecauer, Citation2020), Australia (Beck et al., Citation2021) and Taiwan (Mützel & Scheiner, Citation2022) are some of these cases. Given this generalised impact on PT usage and the consequential long-lasting effects, there has been a growing interest in understanding the factors that influenced travellers’ decisions to reduce their use of PT.
Figure 1. Relative change in monthly PT demand for several PT systems. Monthly average PT demand between January and September 2019 was used as a reference (Sources are presented in Table A.2).
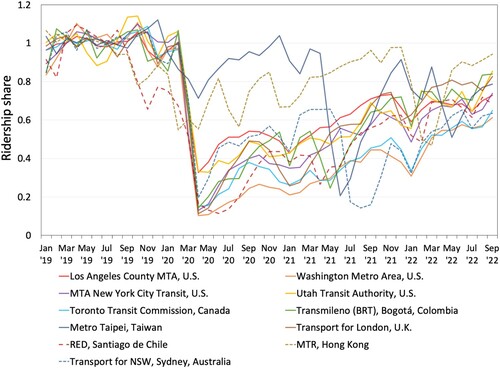
Individual-level factors, which refer to characteristics or attributes that are specific to each person, have been widely studied to determine their influence on PT usage during the pandemic (Bansal et al., Citation2022; Beck et al., Citation2021; He et al., Citation2022). This literature emerged to characterise these relationships, focusing on describing the effects of individual-level factors such as demographics (El Zein et al., Citation2022), socioeconomic status (Jiao & Azimian, Citation2021), and psychological factors (Downey et al., Citation2022; Kim et al., Citation2021). Despite the abundant evidence, comparisons of the nature of these relationships have remained limited, and the existing findings are inconclusive and scattered across many sources. Diverse contexts, different definitions of the travel outcomes, dissimilar specifications of the individual-level factors, and variable units and modelling frameworks may be some of the characteristics that have restricted their comparability. Inconsistent findings between the relationships of individual-level factors and PT usage have also been reported, making it even more challenging to establish definitive conclusions. For example, some studies have reported contradictory associations between gender, age and educational level with PT usage during the pandemic (Almlöf et al., Citation2021; Jiao & Azimian, Citation2021; Palm et al., Citation2021). As a result, clear conclusions based on consistent and reliable data analysis are yet to be provided.
Despite the relevance to generating clear conclusions about the effect of individual-level factors on PT usage, most of the existing literature only provides analysis based on their effect direction (positive, negative or non-statistically significant), paying less attention to the comparison of the effect sizes or magnitude of those effects. As recently has been criticised by Parady and Axhausen (Citation2023), literature in transport frequently focuses its analyses and conclusions on whether the effect of a specific factor is statistically significant rather than assessing the effect size of that relationship. Based on this, there is also a necessity for synthesising the effect sizes of the relationships between individual-level factors and PT usage in the context of the COVID-19 pandemic. This paper aims to address these gaps by (1) systematically reviewing studies that quantitatively assessed the influence of individual-level factors on PT usage during the COVID-19 pandemic, (2) synthesising the effect sizes for each factor through a meta-analysis, (3) providing a comparison of the pooled effect sizes between factors, and (4) analysing the role of moderator variables in the pooled effect sizes.
The rest of this paper is structured as follows. First, the description of the methodology used for the systematic review and meta-analysis is provided in Section 2. Section 3 discusses the relationship between individual-level factors and the modelling perspectives that characterised individuals’ PT usage during the COVID-19 pandemic. Section 4 presents the main findings of the estimation and comparison of the pooled effect sizes. Finally, a discussion is given in Section 5.
Methodology
Literature review
The contribution of individual-level factors to using PT during the pandemic was systematically reviewed and summarised, focussing on quantitative evidence. The review was conducted following the methodological procedure described by Van Wee and Banister (Citation2016). First, search terms that include the following strings (“COVID-19” OR “SARS-CoV-2”) AND (“public transport*” OR “public transit”) AND (“travel behavio*” OR “mobility pattern*” OR “travel pattern*” OR “demand” OR “usage”) were sought in Scopus and WOS. The selection criteria included:
Research papers published from January 2020 to December 2022, which had been peer-reviewed and written in English.
Research papers focusing on empirical evidence of the changes in PT demand levels at a disaggregated level during the COVID-19 pandemic.
Research papers oriented to quantifying statistically the effect of individual-level factors on PT usage during the COVID-19 pandemic.
The search generated a total of 448 studies after the removal of duplicates. Manuscripts were selected in two stages: screening and full reading. In the screening process, titles, abstracts and keywords were analysed, resulting in 197 papers. The excluded studies were found either to be unrelated to travellers’ PT usage or COVID-19. In the next stage, we excluded those studies where the application of qualitative methods meant that they did not statistically quantify the effect of any individual-level factor (such as age, gender or attitudes). Forward snowballing was also implemented, incorporating four studies by this means. Therefore, after the full-text assessment based on the inclusion criteria, 36 articles were finally selected for analysis. The literature review results are presented in Section 3, which synthesises the different perspectives adopted to study travellers’ PT usage during the COVID-19 pandemic (See ) and the effect direction (positive or negative) of individual-level factors on them ().
Table 1. Summary of the studies that assessed the impacts of individual-level factors on PT usage during the COVID-19 pandemic.
Table 2. Summary of the effect directions of individual-level factors on the reduction of PT usage during the COVID-19 pandemic.
Meta-analysis
A meta-analysis is a statistical procedure that combines and summarises the results of multiple studies (Borenstein et al., Citation2009). In the transport domain, this technique has been applied mainly in transport economics (Button, Citation2019). However, examples can also be found in works that studied the relationships between transport and employment (Bastiaanssen et al., Citation2020), the built environment (Laura et al., Citation2021) and cognitive mechanisms (Hoffmann et al., Citation2017). The typical output of a meta-analysis includes a single pooled effect size and a confidence interval. As homogeneity in terms of the nature of the effect sizes is a pre-requisite for conducting any meta-analysis (Hoffmann et al., Citation2017), choosing studies based on the same modelling approach has been shown as a feasible way to deal with this issue (Bastiaanssen et al., Citation2020). As a result, this consideration led to the selection in our study of the effect sizes reported for studies using logistic regression models (LRMs), which included a total of 16 studies. The two main advantages of this model approach were the similar specification of the individual-level factors across studies, which increased the number of comparable effect sizes available, and its straightforward interpretation of its effect sizes (in terms of odds ratios).
After identifying the studies that had used LRMs, effect sizes (coefficients) of the individual-level factors and a measure of their statistical significance (standard errors, p-values or t-statistics) were compiled. Random-effect models (REMs) were then fit to estimate the pooled effect size for each individual-level factor. For this, we followed the “gold standard” in meta-analysis, calculating the weights for each effect size as the inverse of its squared standard error, assigning the greatest importance to the most precise associations (Littell et al., Citation2008). It may be noted that REMs are employed in meta-analysis when there is both within-study and between-study heterogeneity in the effect sizes (Bastiaanssen et al., Citation2020). REMs produce a lower statistical significance and a wider confidence interval (CI) than a fixed-effect model. Consequently, they are widely accepted in meta-analysis studies as a more conservative modelling approach that leads to more robust pooled/combined effect sizes (Borenstein et al., Citation2009). We also took into account the clustered structure of some associations to avoid bias in the weight of each effect size, as more than one association/effect size (k) can be provided per study (N) for the same factor. Additionally, we included both significant and non-significant effects in the meta-analysis, as it is known that dropping the latter may increase the risk of bias in the pooled effect size (Button, Citation2019). We also tested the influence of the geographical region and the pandemic period in the pooled effect sizes between studies.
Systematic literature review results
Overview
The systematic review identified 36 studies where the impacts of individual-level factors on travellers’ PT usage during the COVID-19 pandemic were statistically tested (see for a comprehensive summary). Most existing evidence came from data collected during different periods in 2020 and only a few from later periods (with only six in the first half of 2021). The studies were mainly conducted in Asia (16), Europe (10) and North America (6), with a large number concentrated in the US, China, India and the Netherlands. With a few exceptions (Almlöf et al., Citation2021; Marra et al., Citation2022), almost all selected papers relied on online surveys distributed digitally, such as social platforms like Facebook and Instagram, emails to PT-oriented groups, and links shared by respondents. The obvious limitations of this approach, as recognised by these studies, concern the overrepresentation of respondents with internet access and the underrepresentation of PT passengers without internet. Traditional questionnaires included sections asking for demographic and socioeconomic characteristics, travel behaviour at the time of the survey and during the pre-pandemic, and respondents’ perceptions of COVID-19 risk and associated mitigation strategies. Studies based on both revealed and stated preferences (SP) may be found among the selected literature. The studies that employed revealed preferences aimed to quantify the intensity of travellers’ PT usage. When pre-pandemic PT usage was needed, retrospective information was asked of participants, which clearly relied on the accuracy of participants’ memories. As many as nine studies adopted an SP approach. This approach allowed them to generate a dataset of choices based on different levels of the attributes of the considered modes and to explore travellers’ mode choice decisions in hypothetical scenarios. The hypothetical scenarios included different COVID-19 emergency contexts (in terms of number of new cases, death toll and vaccination scenarios) (Bansal et al., Citation2022) and the different virus mitigation measures (e.g. disinfection in PT buses, social distancing) (Bansal et al., Citation2022).
PT usage characterisation in individual-level studies
The selected studies characterised the PT usage of travellers during the COVID-19 pandemic using different definitions of the dependent variable. Based on the nature of the dependent variable, four main categories of studies were identified: (i) studies that analysed the decision to choose PT among the presence of alternative modes (16 studies), (ii) studies that focused on measuring if travellers reduced the number of PT trips compared with the pre-pandemic (13 studies), (iii) studies that measured the intensity of PT use by considering the number of trips made by travellers (4 studies), and (iv) studies that investigated the intention/willingness to use PT (3 studies).
In category (i), mode choice was the main outcome observed (Chen et al., Citation2022; Das et al., Citation2021). In this category, discrete choice models (DCMs) and logistic regressions (LRMs) were commonly employed. Category (ii) includes outcomes such as whether individuals reduced their trips made by PT during the pandemic (4 studies), whether they stopped at all (4 studies) or whether they shifted from PT to an alternative mode (5 studies). The modelling approach widely adopted in this category was logistic regression. In category (iii), studies employed modelling approaches for continuous outcomes such as OLS, binomial and zero-inflated Poisson. Category (iv) shows studies where ordered outcomes were considered in structural equations modelling under different behavioural theories (Zhang et al., Citation2021). Specific details of the specification of the PT outcome variable and modelling approach are provided in . As the selected studies considered different directions to define the PT outcome variables (e.g. to choose PT vs not choose PT), it was necessary to adopt one direction and transform (“flip”) the effects found for the definition that employed the opposite one. Therefore, the adopted consensus was to reflect the effects of individual-level factors on a pro-reduction view of PT usage during the pandemic. Consequently, the PT usage outcomes for categories (i) and (ii), for instance, characterise the decision of not choosing PT and reducing PT trips/shifting from PT to an alternative mode. The same consensus is kept for the rest of this work to obtain a more straightforward interpretation of the effect of individual-level factors.
Effect direction of individual-level factors on PT usage
This section summarises the relationships reported in the selected 36 studies between individual-level factors and the reduction of PT usage in the context of the COVID-19 pandemic. A total of 15 different individual-level factors were identified: demographics (gender, age, race and ethnicity, education, children and household size), socioeconomic status (income, car availability, teleworking possibilities, and full-time employment), perceived importance to the COVID-19 risk and mitigation strategies, healthcare needs (disability and poor health) and transport-oriented attributes (pre-COVID frequent PT user, travel distance, and PT physical accessibility). The analysis of the selected papers also identified different approaches used to consider the effect of individual-level factors on PT usage: (a) direct effects, (b) interaction effects, and (c) explaining class membership, being the first one (a) the most frequent. A list of these factors and their associated relationships with PT usage reduction may be found in .
For the direct effects approach (a), highlights the fact that demographic factors presented heterogeneous effect directions across studies, with a mix of positive, negative and non-significant effects for the same factor. For instance, in the case of gender, 37% of the associations indicated that male travellers were more likely to reduce PT use compared to females (Abdullah et al., Citation2020, Citation2021; Jiao & Azimian, Citation2021), whereas 16% found the opposite effect (Beck et al., Citation2021; Palm et al., Citation2021), and 47% found no significant relationship (Aaditya & Rahul, Citation2023). Similarly, only 39% of the studies that reported results for age showed that older adults were more likely to reduce PT usage (Mashrur et al., Citation2022). In the case of race and ethnicity, evidence is also contradictory, with some associations reporting a positive association (Jiao & Azimian, Citation2021) and others a negative one (Soria et al., Citation2023). shows that educational level was positively related to reducing PT use (El Zein et al., Citation2022), and that household size presents negative effects (the higher the household size, the lower the reduction in PT use) (Jiao & Azimian, Citation2021). The evidence presented for the presence of children at home agrees that this factor did not cause any significant effect on travellers’ PT use.
Regarding socioeconomic status, the influence of income level, car availability, teleworking, and full-time employment show more agreement across studies. For income level, 14 studies (78%) found a positive association between this factor and the reduction in PT usage (Parker et al., Citation2021; Schaefer et al., Citation2021). This result suggests that individuals of higher income levels were more likely to reduce PT use during the pandemic than travellers of lower levels. For car availability, ten associations (71% of the reported effects) presented statistically significant positive relationships (Mazanec et al., Citation2023; Palm et al., Citation2021), while for teleworking, 76% of the associations also found significant positive effects on the reduction of PT use (Mashrur et al., Citation2022; Schaefer et al., Citation2021). Regarding full-time employment, some studies reported that such travellers tended to use more PT more often (negative effect) than students, freelancers or the unemployed (Aaditya & Rahul, Citation2023; Zhao & Gao, Citation2022). However, other works found the opposite relationship (Das et al., Citation2021).
In order to analyse the influence of COVID-19 risk perception, studies included travellers’ perception of the severity of the virus and the importance they gave to the implementation of mitigation strategies, such as hygiene/cleanliness, social distancing and mandatory use-of-face masks (Aaditya & Rahul, Citation2023; Abdullah et al., Citation2020; Zhao & Gao, Citation2022). The studies tested these variables directly on the PT outcome (specifying dummies) (El Zein et al., Citation2022; Palm et al., Citation2021; Soria et al., Citation2023) or indirectly following a latent variable approach based on five-point Likert scale indicators (Abdullah et al., Citation2020; Rankavat et al., Citation2023). As expected, the perception of the severity of the virus and the importance given to mitigation strategies showed positive correlation when they were used together to construct a latent variable and showed a positive effect on the reduction of PT use when specified separately (Abdullah et al., Citation2020). A total of 17 positive associations (77%) showed that the higher the importance given to the COVID-19 risk and mitigation strategies among travellers, the higher the reduction in travellers’ PT use (Basnak et al., Citation2022; Vallejo-Borda et al., Citation2022). However, more complex psychological mechanisms through which travellers adjusted their behaviour by the changes in their attitudes and perceptions to use PT were also recognised by Kim et al. (Citation2021), Zhang et al. (Citation2021) and Vallejo-Borda et al. (Citation2022).
Unexpected effect directions were found for the factors that account for individuals’ healthcare needs. Travellers with a disability and those with poor health showed a positive association with the use of PT during the pandemic, compared to individuals without these conditions (He et al., Citation2022; Jiao & Azimian, Citation2021; Palm et al., Citation2021). Potential explanations may be found in this group's low availability of alternative modes. Significant effects were also found for transport-related factors such as travel distance (for commuting trips), PT physical accessibility and whether a traveller was a pre-COVID frequent PT user. The evidence showed that travelling frequently by PT during the pre-pandemic negatively impacted people’s decision to reduce PT (Palm et al., Citation2021; Soria et al., Citation2023), providing evidence that pre-pandemic mobility habits also played a key role in the decision of whether to use PT during the pandemic. Regarding travel distance, two studies reported negative effects (Abdullah et al., Citation2020; Liu et al., Citation2022), meaning that longer travel distances showed a negative association with the reduction in PT use. In the case of PT physical accessibility, its effect showed that during the pandemic, the longer the access time to get a PT service, the higher the reduction in PT use (Das et al., Citation2021; Tan & Ma, Citation2021).
More complex influences of individual-level factors were also studied by considering their role modifying the effects of other variables (category (b), 3 studies) or to account for taste variation among travellers using a latent class approach (category (c), 3 studies). In the former, travellers’ characteristics such as gender, age, income, educational level and ethnicity were found to modify the effects of PT crowding (CRO), the number of COVID-19 cases (C19), the implementation of mandatory face masks (MSK) and the share of the population vaccinated (MSV) on the choice of PT mode (Bansal et al., Citation2022; Basnak et al., Citation2022). For instance, it was found that female and elderly travellers showed a higher sensitivity to crowding in PT modes. The mandatory use of face masks in PT modes had a smaller influence on male travellers than on females and a relatively greater positive effect on elderly passengers. Schaefer et al. (Citation2021) also stated that people who worked from home feared catching the virus more when using PT, highlighting the complex relationship between attitudes and travel behaviour. In the latter category, the role of individual-level factors was to help define groups of travellers that share a more homogenous perception of disutility in terms of level of service attributes (e.g. travel time, travel cost, crowding) (Chen et al., Citation2022; Shelat et al., Citation2022). In this case, the relationship observed was the association of an individual to a cluster rather than a direct or interaction effect. For instance, it was identified in these works that the cluster associated with female travellers, the elderly and high-income perceived a higher adverse impact of the time travelled in PT and a higher positive effect of preventive measures such as social distancing in PT modes.
In conclusion, individual-level factors played various roles regarding how they were included in a given study. The most frequent approach among them was their specification as direct explanatory variables of the changes in travellers’ PT use during the pandemic. It was found from the evidence gathered in this approach that for many factors (e.g. gender, age), the findings revealed contradictory results, making it difficult to establish clear conclusions. Moreover, as the analysis in this section relied only on the effect direction of each association, the relevance in term of the effect size of those effects still need to be provided.
Meta-analysis results
Preparation
To ensure the comparability of the effect sizes of individual-level factors on PT usage, studies that employed logistic regression models (LRMs) were chosen. This choice was made based on the analysis of the 36 selected studies, which showed that the selection of LRM-based studies provided the potential for the most robust synthesis by offering the highest number of comparable individual studies compared with other modelling approaches. LRMs were used as a modelling approach in both of the two main categories of PT usage studies, namely those to (i) choose/not choose PT and (ii) reduce PT usage. LRMs have the advantage of involving the specification of individual-level factors as direct explanatory variables of PT usage (rather than as interaction effects). Another characteristic of LRMs that facilitated the cross-study comparison is the fact that most of their factors were specified as dummies, which removes potential difficulties associated with the measurement units. For instance, income was usually specified as a discrete number of categories with similar qualitative thresholds across studies (low/mid/high-income).
An additional advantage of LRMs is that there is a straightforward interpretation of their coefficients. The exponential value of a coefficient indicates the corresponding variables’ contribution in terms of its odds ratio. In general, an odds ratio is defined as the ratio of the probability of the occurrence of an event relative to the probability of the event not occurring. As LRMs were applied in category outcomes (i) and (ii), the interpretation based on the odds ratios is defined as the probability of reducing PT trips/choosing an alternative mode (not PT) relative to the event this not occurring. In the case of category (i) studies, the alternative modes were car or ride-hailing. An odds ratio higher than one indicates that the factor analysed increases the probability of the event (reducing PT usage/not choosing PT in our case), while a value lower than one indicates the opposite. If, for example, the odds ratio associated with a certain dummy variable were 2.0, that would mean that an individual with that characteristic has two times the probability of experiencing the event “reducing PT usage” compared with an individual without that characteristic. Similarly, if an odds ratio is lower than one, let us say 0.5, an individual with that attribute is half as likely to experience the event “reduction of PT usage” compared to those without that characteristic. In cases where the PT usage outcome needed to be transformed to fit the adopted consensus (e.g. from increase to reduction in PT use), the respective odds ratios were re-estimated by taking their inverse.
Pooled effect sizes
A total of 16 comparable studies that examine the effect of individual-level factors using LRMs were finally included in the meta-analysis. We performed meta-analyses separately for the 15 factors shown in . A standardisation was required to ensure that all the factors specified as dummy variables shared the same reference categories. The category references adopted for the demographic factors in the meta-analysis were: gender (1:male; 0:female), age (1:>65 years old; 0:first age category level, which frequently ranged from 18 to 35 years old depending on the study), race and ethnicity (1:Black/Hispanic/Indigenous; 0:White), high-educational level (1:University/college degree, 0:no degree), children (1:presence of children at home; 0:otherwise) and household size (1:two or more; 0:otherwise). Similarly, for socioeconomic factors, we defined references for income (1:high-income level; 0:low-income level), car availability (1:at least one car owned or available; 0:no car available/owned) and employment (1:full-time employment: 0:student/not employed). Additionally, healthcare needs (1:condition of disability or poor health; 0:otherwise), travel frequent PT user (1:pre-COVID frequent PT user; 0:otherwise) and travel distance (1:travel distance longer than 5 km, 0:travel distance shorter than 5 km). In the case of teleworking, several frequency categories were included (1:1–2 days per week, 3–4 days per week, etc.), considering as a reference category the possibility of no teleworking. Similarly, the studies specified physical accessibility to PT using several dummy categories (1:10-20 min, > 20 min, etc.), adopting as reference category in this work walking times lower than 6.5 min. Finally, the COVID-19 risk perception factor was measured by specifying dummies and five-point Likert scale variables. In this case, the interpretation of the odds ratio is then associated with how likely a traveller was to reduce their PT use during the pandemic by increasing one unit of COVID-19 risk perception. Table A.3 in the Appendix offers a detailed description of the associations employed for this factor.
For some dummy associations, the reference category was transformed (“flipped”) to agree with the criteria mentioned previously, i.e. to relate to reductions in PT use. In the case of two-category dummy variables, the procedure to obtain the transformed odds ratio is straightforward in LRMs and only involves taking the inverse of the exponential LRM coefficients, as the magnitude of the standard errors is the same. In the case of multiple categories, the procedure to estimate the transformed effect size is similar, but the standard errors need to be re-estimated using, for example, Fieller’s method. Applying the previously mentioned criteria allowed the generation of comparable effect sizes.
illustrates the synthesised effect sizes (odds ratios) and confidence intervals (CIs) for the associations of individual-level factors with the reduction of PT usage across comparable studies based on the previously mentioned random effect models. Ten pooled effect sizes were found to be statistically significant, four not significant (older adults, children, household size, and full-time employment), and one close to being significant (PT physical accessibility). also shows that repeatedly, the CIs are comparable among the associations within each individual-level factor (i.e. within-study variability is reasonably constant across studies). However, as the mean of the pooled effect sizes, represented by the blue diamond, is not contained in all CIs for a specific factor, a substantial heterogeneity between studies can be seen. In fact, we found that the statistic I2 indicated the presence of a relevant heterogeneity among the effect sizes of the studies analysed. As most of the I2 values of the factors meta-analysed ranged from 60% to 90%, it was possible to infer that the observed differences in effect sizes in each individual-level factor were due to real differences in the underlying effect rather than just random variation. This outcome ratified the choice of random effect models to estimate the pooled effect sizes to handle both within- and between-study heterogeneity.
Figure 2. Effect sizes (odds ratios) of individual-level factors on PT usage reduction during the COVID-19 pandemic. Blue diamonds represent pooled effects. Confidence intervals at 95%. The vertical line (value 1) indicates no effect.
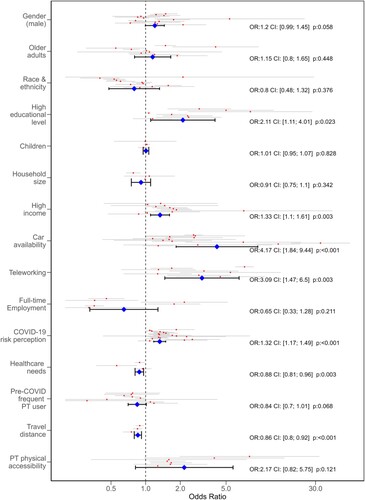
Among the factors meta-analysed, car availability, teleworking and high educational level were the factors with the largest pooled effect sizes. In the case of car availability, all the studies consistently reported odds ratios higher than 1, indicating a positive association (+) between car availability and the reduction of PT usage during the pandemic. The overall random effect for this factor was equal to an OR+ of 4.17 (CI: 1.84; 9.44, p = <0.001). This result suggests that individuals who owned or had at least one car available during the pandemic were almost four times more likely to reduce their PT usage compared with the pre-pandemic than those without this possibility. Similarly, individuals with the possibility of teleworking were three times more likely to reduce their PT usage compared to those who did not (OR+ = 3.08, CI: 1.46; 6.50, p = 0.003). The next individual-level factor in terms of magnitude was high educational level. Based on the pooled effect of this factor (OR+ = 2.11, CI: 1.11; 4.01, p = 0.023), we found that travellers with a university or college degree had odds of reducing PT usage that were about two times the one of those individuals without one.
A second group of individual-level factors, including gender (male), income, COVID-19 risk perception and healthcare needs, showed a more modest pooled effect size. Those who identified themselves as male had 20% more chance to reduce PT usage than those who identified themselves as female (OR+ = 1.20, CI: 0.99; 1.45, p = 0.058). Many authors explain this by the fact that females had fewer transport mode options than males, alluding to social and cultural aspects (Das et al., Citation2021). High income presented similar strength (OR+ = 1.33, CI:1.10; 1.61, p = 0.003); individuals with high-income levels were 33% more likely to decrease PT use than those from the lowest income level. The combined effect for the risk perception of COVID-19 showed an OR+ = 1.32 (CI: 1.18; 1.49, p = <0.001). This implies that those who experienced a higher degree of concern associated with the severity of the virus were 31% more likely to reduce PT usage. Regarding the healthcare needs factor associated with disability conditions and poor health, the meta-analysis estimated a pooled OR- = 0.88 (CI: 0.81; 0.96, p = 0.034). This indicates a negative association between this factor and the reduction of PT use. In particular, the odds of a person with this condition travelling less by PT were 12% lower than individuals without it (i.e. suggesting a positive effect with using PT).
For the transport-related factors, the pooled effect of travel distance showed that travellers with longer travel distances than 5 km were 14% less likely to reduce PT use than those with shorter distances (OR- = 0.86, CI: 0.80; 0.92, p = <0.001). A negative combined association was found for the factor pre-COVID frequent PT user (OR- = 0.84, CI: 0.70; 1.01, p = 0.068). This means frequent pre-pandemic PT users were 16% less likely to travel less by PT during the pandemic. The pooled effect for PT physical accessibility indicated a positive relation with PT usage reduction (OR+ = 2.16, CI: 0.82; 5.75, p = 0.121). Consequently, walking more than 6.5 min to access a PT stop increased the probability of reducing PT use almost two times compared to those travellers with shorter access time.
The remaining individual-level factors were those whose pooled effect sizes were not statistically significant. This occurred because their CIs crossed the threshold of 1, which is the boundary between an odds ratio defining a positive or negative association between an explanatory variable and the dependent variable. This means that for those factors, the heterogeneity among the associations reported in terms of direction and size did not allow us to establish with statistical certainty whether they were associated positively or negatively with a reduction in PT usage. This situation was observed for employment, whose OR-ns = 0.65 (CI: 0.35; 1.19, p = 0.211) indicated that travellers with full-time jobs were 35% less likely to reduce PT usage than other groups. Another factor with a similar outcome was older adults (OR+ns = 1.15, CI: 0.80; 1.65, p = 0.447). For this factor, even though the pooled effect estimated a positive effect on the reduction of PT usage, this was not statistically significant. For race and ethnicity, the combined effect showed an OR-ns of 0.80 (CI: 0.48; 1.32, p = 0.376), meaning that someone who belongs to one of these categories was 20% less likely to reduce PT use compared with white individuals. The pooled effect size of the presence of children at home (OR+ns = 1.01, CI: 0.95; 1.06, p = 0.828), and household size (OR+ns = 0.91, CI: 0.75, p = 0.342) were also not significant, as their pooled effects were located very close to 1, indicating no effect on PT usage reduction.
Subgroup and influence analyses
To account for different research contexts and the category of the dependent variable specification, we applied a subgroup analysis, which allows us to determine whether the inclusion of moderator variables can explain that some associations produce lower or higher odds ratios than others (Laura et al., Citation2021). Two hypotheses that were defined a priori were tested: there are statistically significant differences in the pooled effect sizes depending on (H1) the geographical region of the study and (H2) the period of the pandemic when the surveys were delivered. In order to investigate H1 and H2, two dummy moderators were statistically tested. For the definition of the region, effect sizes were grouped into geographical areas, namely the Asia region and the North America/Europe (NA/EUR) region. For the pandemic period, two groups were defined, considering that most of the studies were conducted between March 2020 and April 2021. We decided to define one group as those studies conducted by surveys delivered between March and August 2020 (representing the outbreak and the first recovery process) and the second group with data from September 2020 to April 2021 (associated with later waves and lower mobility restrictions). Additionally to the two hypotheses presented, we also analysed differences among the pooled effect sizes depending on the category of the PT outcome employed in the studies (PTC vs PTR) and the metric types used in specific factors. Related to the latter, we tested for the factor COVID-19 risk perception, whether its different specifications (dummy vs Likert) generated dissimilar pooled effect sizes. In addition, an influence analysis was performed to assess the effect of excluding associations whose values differed substantially from the overall effect. This analysis allows us to observe the sensitivity in the pooled effect sizes to certain studies, enabling further analysis of the robustness of the results. The identification of outliers was conducted iteratively for each factor until all CIs of each association overlapped with the confidence interval of the pooled effect. Nonetheless, as excluding outliers may lead to biased results in meta-analyses, it is recommended to use its outcomes cautiously (Borenstein et al., Citation2009). presents the results for the subgroup and influence analyses.
Table 3. Subgroup and influence analyses for the effect sizes of individual-level factors on PT reduction during the COVID-19 pandemic.
The results shown in indicate that (H1) the geographical region successfully accounted for variation in the observed effect sizes, while (H2) the pandemic period was found to be significant only for one factor. Statistically significant differences were found between the pooled effect sizes of gender (p = <0.001), older adults (p = 0.102), educational level (p = 0.004) and full-time employment (p = 0.033) of the Asian and NA/EUR regions. In particular, it was found that factors such as gender and age have almost no impact in explaining the reduction in PT use in studies conducted in the NA/EUR region (OR-ns = 0.94, p = 0.473 and OR-ns = 0.91, p = 0.708). In contrast, for the Asia region, the same factors increased by almost 50% the chance that a traveller reduced their PT use (OR+ = 1.51, p = <0.001 and OR+ = 1.68, p = 0.077). The difference observed was even higher when the educational level was analysed (OR+ = 4.22, p = <0.001 for the Asian region and OR+ = 1.51, p = 0.014 for the NA/EUR region). Cultural and social differences between the regions analysed may explain these dissimilarities. Regarding the pandemic periods, we found differences statistically significant only for the effect sizes of the factor pre-COVID frequent PT user. In particular, the result showed that during the first period of the pandemic being a pre-COVID frequent PT user was irrelevant to explain the decision of travelling by PT (OR-ns = 0.94, p = 0.373). However, this changed during the second period, where those who were regular users of PT showed 41% more chances to travel by PT, than those who were not (OR- = 0.71, p = <0.001).
Regarding the role of the type of the modelling PT outcome in the effect sizes, the results showed significant differences only for gender and car availability. It was observed for gender that this factor was only relevant among PTC studies (OR+ = 1.50 vs. OR+ns = 1.01). In the case of car availability, despite its effect was significant for both approaches (PTC and PTR studies), it was significantly higher for the former (OR+ = 8.32 vs. OR+ = 2.05). These results suggest that gender and car availability had more relevance in models where the dependent variable was specified as the decision of choosing/not choosing PT during the COVID-19 pandemic. We also tested for potential differences in the effect sizes of the factor COVID-19 risk perception, as for this factor the effect sizes of dummy and ordinal variables were retrieved. However, the subgroup analysis showed no significant dissimilarity (p = 0.704).
In the influence analysis, the exclusion of outliers generated three main outcomes: some factors increased their consistency becoming statistically significant, some reduced substantially their effect size, and others did not show a relevant difference. Among the factors that became significant were race and ethnicity, and PT physical accessibility. The analysis showed that for PT physical accessibility (which original OR and p-value were 2.16 and 0.121, respectively), the exclusion of its most extreme effect size (OR = 8.0) caused a more consistent pooled effect (OR+ = 1.55, CI: 1.03; 2.33, p = 0.038). Similarly, for race and ethnicity, the exclusion of the effect size provided by Jiao and Azimian (Citation2021) (OR = 1.2) resulted in this factor becoming statistically significant (OR- = 0.65, CI: 0.44; 0.94, p = 0.021). The most substantial change in terms of effect size was observed for car availability and teleworking, which decreased the magnitude of their effect from 4.2 to 1.8, and 3.1 to 2.3, respectively.
Discussion
To our knowledge, the review reported here is the first study to provide a comprehensive review of the effects of individual-level factors on public transport (PT) usage during the COVID-19 pandemic. We conducted such a comparison by systematically reviewing the existing evidence and performing a meta-analysis of the effect sizes of the individual-level factors across comparable studies. The systematic review identified 36 relevant studies executed between 2020 and 2021, of which 16 generated data that could be analysed through a meta-analysis. By choosing comparable studies and factor specifications for the meta-analysis, we were able to compare the factors’ pooled effect sizes. Our study complements early works presented in the light of the pandemic (De Vos, Citation2020; Gkiotsalitis & Cats, Citation2020; Tirachini & Cats, Citation2020), by being the first to quantitatively summarise the impacts of individual-level factors on people’s PT usage and to offer a comprehensive comparison between them.
The systematic review found that individual-level factors exhibited heterogeneous levels of consistency in terms of the effect direction reported across studies. Regarding this, two main groups of individual-level factors were observed. Factors such as car availability, teleworking, high-level income, high educational level and COVID-19 risk perception showed consistent positive associations with the reduction of PT use compared with the pre-pandemic across studies. On the other hand, ambiguous effect directions were found for factors such as gender (male), age (older adults), race and ethnicity, and employment. For these inconclusive effects, the mean of the pooled effect size and their confidence interval helped to determine their effect directions statistically. We obtained, then, that males and older adults were factors positively related to the reduction of PT usage, while employment (full-time) showed a negative association. However, of them, only the pooled effect of gender was statistically significant. We also found that contextual factors such as the region where studies were conducted and the pandemic period helped to understand factors’ effect differences. In particular, when controlling for region (North America/Europe vs. the Asia region), we found that the effects of gender and age were only statistically significant for studies conducted in the latter group. Cultural and social differences may explain these differences. The findings of this study can help us understand specific population groups’ restrictions and needs during pandemics/epidemics. The results highlight the relevance of inequality associated with the use of PT during the COVID-19 pandemic by some of the more vulnerable population segments: women, older adults, people with healthcare needs, those without the possibility of teleworking and those who travel longer distances. From a social point of view, public transport authorities should consider the needs of these population segments when deciding to adjust service levels in the event of a pandemic (DeWeese et al., Citation2020).
Notable differences in the effect size of each factor in reducing travellers’ probability of using PT during the pandemic were also found. Unexpectedly, the meta-analysis revealed that people’s car availability was the factor with the highest negative effect on the use of PT during the pandemic. Its pooled effect revealed that individuals with at least one car available were four times more likely to reduce their PT use during the pandemic than those without that possibility. Similarly, the possibility given to some individuals to work from home increased their odds of reducing PT trips by about three times compared to those without teleworking availability. A more modest effect on PT usage was observed for the other individual-level factors. Factors such as gender, high income level, and COVID-19 risk perception only increased the odds of travelling less by PT by no more than 30%. The meta-analysis also revealed that with the available evidence, it was not possible to generate reliable pooled effects for factors such as age and employment because of the high inconsistency of their effects. The substantial difference observed in this study among the impact of travellers’ associated factors on PT usage highlights the need in the transport domain for not only discussing effect directions, but also comparing effect sizes (Parady & Axhausen, Citation2023).
How much of the effects observed in this review associated with the reduction in PT usage are still present today seems a relevant interrogation to addressing policies to encourage PT use. How many of those who shifted from PT to private modes have returned and how these processes can be supported remains to be seen. Habits and attitudes developed by travellers during the pandemic on alternative modes to PT may also play a key role next (Hoffmann et al., Citation2017). Additionally, the impact of new trends and technologies related to electro-mobility, such as EVs, e-bikes and e-scooters (Reck et al., Citation2022), and autonomous vehicles on travellers’ mode choice decisions has only started to be assessed (Yuen et al., Citation2022). In this scenario, where the possibility of teleworking and online shopping have also been established, it seems unlikely there will be a complete recovery of individual PT usage for everyone. Therefore, growing subsidies to PT systems may be required to keep fare prizes at bay, avoiding increasing existing inequalities on already vulnerable population segments, which PT usage has demonstrated to be less adaptable, even in the most severe circumstances like the COVID-19 pandemic.
Supplemental Material
Download MS Word (56.1 KB)Disclosure statement
No potential conflict of interest was reported by the author(s).
Additional information
Funding
Notes
1 A summary of studies where the impacts of PT demand on aggregated PT demand was characterised is provided in the supplementary material.
References
- Aaditya, B., & Rahul, T. M. (2023). Long-term impacts of COVID-19 pandemic on travel behaviour. Travel Behaviour and Society, 30, 262–270. https://doi.org/10.1016/j.tbs.2022.10.005
- Abdullah, M., Ali, N., Javid, M. A., Dias, C., & Campisi, T. (2021). Public transport versus solo travel mode choices during the COVID-19 pandemic: Self-reported evidence from a developing country. Transportation Engineering, 5, 100078. https://doi.org/10.1016/j.treng.2021.100078
- Abdullah, M., Dias, C., Muley, D., & Shahin, M. (2020). Exploring the impacts of COVID-19 on travel behavior and mode preferences. Transportation Research Interdisciplinary Perspectives, 8, 100255. https://doi.org/10.1016/j.trip.2020.100255
- Almlöf, E., Rubensson, I., Cebecauer, M., & Jenelius, E. (2021). Who continued travelling by public transport during COVID-19? Socioeconomic factors explaining travel behaviour in Stockholm 2020 based on smart card data. European Transport Research Review, 13(1). https://doi.org/10.1186/s12544-021-00488-0
- Bansal, P., Kessels, R., Krueger, R., & Graham, D. J. (2022). Preferences for using the London Underground during the COVID-19 pandemic. Transportation Research Part A: Policy and Practice, 160, 45–60. https://doi.org/10.1016/j.tra.2022.03.033
- Basnak, P., Giesen, R., & Munoz, J. C. (2022). Estimation of crowding factors for public transport during the COVID-19 pandemic in Santiago, Chile. Transportation Research Part A: Policy and Practice, 159, 140–156. https://doi.org/10.1016/j.tra.2022.03.011
- Bastiaanssen, J., Johnson, D., & Lucas, K. (2020). Does transport help people to gain employment? A systematic review and meta-analysis of the empirical evidence. Transport Reviews, 40(5), 607–628. https://doi.org/10.1080/01441647.2020.1747569
- Beck, M. J., Hensher, D. A., & Nelson, J. D. (2021). Public transport trends in Australia during the COVID-19 pandemic: An investigation of the influence of bio-security concerns on trip behaviour. Journal of Transport Geography, 96, 103167. https://doi.org/10.1016/j.jtrangeo.2021.103167
- Borenstein, M., Hedges, L. V., Higgins, J. P. T., & Rothstein, H. R. (2009). Introduction to meta-analysis. Wiley.
- Button, K. (2019). The value and challenges of using meta-analysis in transportation economics. Transport Reviews, 39(3), 293–308. https://doi.org/10.1080/01441647.2018.1464078
- Chen, C., Feng, T., Gu, X., & Yao, B. (2022). Investigating the effectiveness of COVID-19 pandemic countermeasures on the use of public transport: A case study of The Netherlands. Transport Policy, 117, 98–107. https://doi.org/10.1016/j.tranpol.2022.01.005
- Cho, S.-H., & Park, H.-C. (2021). Exploring the behaviour change of crowding impedance on public transit due to COVID-19 pandemic: Before and after comparison. Transportation Letters, 13(5-6), 367–374. https://doi.org/10.1080/19427867.2021.1897937
- Das, S., Boruah, A., Banerjee, A., Raoniar, R., Nama, S., & Maurya, A. K. (2021). Impact of COVID-19: A radical modal shift from public to private transport mode. Transport Policy, 109, 1–11. https://doi.org/10.1016/j.tranpol.2021.05.005
- Delclos-Alio, X., Gutierrez, A., Miravet, D., Tomas-Porres, J., Vich, G., & Clave, S. A. (2022). Impact of the COVID-19 pandemic on tourist public transportation use and on its determinants: Evidence from a Catalan coastal destination. Tourism Management Perspectives, 44, 101003. https://doi.org/10.1016/j.tmp.2022.101003
- De Vos, J. (2020). The effect of COVID-19 and subsequent social distancing on travel behavior. Transportation Research Interdisciplinary Perspectives, 5, 100121. https://doi.org/10.1016/j.trip.2020.100121
- DeWeese, J., Hawa, L., Demyk, H., Davey, Z., Belikow, A., & El-geneidy, A. (2020). A tale of 40 cities: A preliminary analysis of equity impacts of COVID-19 service adjustments across North America. Findings. https://doi.org/10.32866/001c.13395
- Downey, L., Fonzone, A., Fountas, G., & Semple, T. (2022). The impact of COVID-19 on future public transport use in Scotland. Transportation Research Part A: Policy and Practice, 163, 338–352. https://doi.org/10.1016/j.tra.2022.06.005
- Eisenmann, C., Nobis, C., Kolarova, V., Lenz, B., & Winkler, C. (2021). Transport mode use during the COVID-19 lockdown period in Germany: The car became more important, public transport lost ground. Transport Policy, 103, 60–67. https://doi.org/10.1016/j.tranpol.2021.01.012
- Elias, W., & Zatmeh-Kanj, S. (2021). Extent to which COVID-19 will affect future use of the train in Israel. Transport Policy, 110, 215–224. https://doi.org/10.1016/j.tranpol.2021.06.008
- El Zein, A., Beziat, A., Pochet, P., Klein, O., & Vincent, S. (2022). What drives the changes in public transport use in the context of the COVID-19 pandemic? Highlights from Lyon metropolitan area. Regional Science Policy & Practice, 14(S1), 122–141. https://doi.org/10.1111/rsp3.12519
- Fernández Pozo, F., Wilby, R., Vinagre Díaz, M. R., J, J., & Rodríguez González, A. B. (2022). Data-driven analysis of the impact of COVID-19 on Madrid's public transport during each phase of the pandemic. Cities, 127, 103723. https://doi.org/10.1016/j.cities.2022.103723
- Gkiotsalitis, K., & Cats, O. (2020). Public transport planning adaption under the COVID-19 pandemic crisis: literature review of research needs and directions. Transport Reviews, 41(3), 374–392. https://doi.org/10.1080/01441647.2020.1857886
- Gramsch, B., Guevara, C. A., Munizaga, M., Schwartz, D., & Tirachini, A. (2022). The effect of dynamic lockdowns on public transport demand in times of COVID-19: Evidence from smartcard data. Transport Policy, 126, 136–150. https://doi.org/10.1016/j.tranpol.2022.06.012
- He, Q., Rowangould, D., Karner, A., Palm, M., & LaRue, S. (2022). Covid-19 pandemic impacts on essential transit riders: Findings from a U.S. Survey. Transportation Research Part D: Transport and Environment, 105, 103217. https://doi.org/10.1016/j.trd.2022.103217
- Hoffmann, C., Abraham, C., White, M. P., Ball, S., & Skippon, S. M. (2017). What cognitive mechanisms predict travel mode choice? A systematic review with meta-analysis. Transport Reviews, 37(5), 631–652. https://doi.org/10.1080/01441647.2017.1285819
- Hsieh, H. S., & Hsia, H. C. (2022). Can continued anti-epidemic measures help post-COVID-19 public transport recovery? Evidence from Taiwan. Journal of Transport & Health, 26, 101392. https://doi.org/10.1016/j.jth.2022.101392
- Ito, H., & Kawazoe, N. (2023). Examining transportation mode changes during COVID-19 in Toyama, Japan. Regional Studies, Regional Science, 10(1), 253–272. https://doi.org/10.1080/21681376.2023.2180425
- Jenelius, E., & Cebecauer, M. (2020). Impacts of COVID-19 on public transport ridership in Sweden: Analysis of ticket validations, sales and passenger counts. Transportation Research Interdisciplinary Perspectives, 8, 100242. https://doi.org/10.1016/j.trip.2020.100242
- Jiang, S., & Cai, C. (2022). Unraveling the dynamic impacts of COVID-19 on metro ridership: An empirical analysis of Beijing and Shanghai, China. Transport Policy, 127(C), 158–170. doi:10.1016/j.tranpol.2022.09.002
- Jiao, J., & Azimian, A. (2021). Exploring the factors affecting travel behaviors during the second phase of the COVID-19 pandemic in the United States. Transportation Letters, 13(5-6), 331–343. https://doi.org/10.1080/19427867.2021.1904736
- Khadem Sameni, M., Barzegar Tilenoie, A., & & Dini, N. (2021). Will modal shift occur from subway to other modes of transportation in the post-corona world in developing countries? Transport Policy, 111, 82–89. https://doi.org/10.1016/j.tranpol.2021.07.014
- Kim, M.-H., Lee, J., & Gim, T.-H. T. (2021). How did travel mode choices change according to Coronavirus Disease 2019? Lessons from Seoul, South Korea. International Journal of Urban Sciences, 25(3), 437–454. https://doi.org/10.1080/12265934.2021.1951823
- Laura, A., Graham, C., Alexa, D., Md, K., & David, T. (2021). Exploring built environment impacts on transit use – an updated meta-analysis. Transport Reviews, 41(1), 73–96. doi:10.1080/01441647.2020.1806941
- Littell, J. H., Corcoran, J., & Pillai, V. (2008). Systematic reviews and meta-analyis. Cambridge University Press.
- Liu, J., Cao, Q., & Pei, M. (2022). Impact of COVID-19 on adolescent travel behavior. Journal of Transport & Health, 24, 101326. https://doi.org/10.1016/j.jth.2021.101326
- Liu, L., Miller, H. J., & Scheff, J. (2020). The impacts of COVID-19 pandemic on public transit demand in the United States. PLoS One, 15(11), e0242476. https://doi.org/10.1371/journal.pone.0242476
- Lizana, M., Choudhury, C., & Watling, D. (2023). Using smart card data to model public transport user profiles in light of the COVID-19 pandemic. Travel Behaviour and Society, 33, 100620. https://doi.org/10.1016/j.tbs.2023.100620
- Marra, A. D., Sun, L., & Corman, F. (2022). The impact of COVID-19 pandemic on public transport usage and route choice: Evidences from a long-term tracking study in urban area. Transport Policy, 116, 258–268. https://doi.org/10.1016/j.tranpol.2021.12.009
- Mashrur, S. M., Wang, K., & Habib, K. N. (2022). Will COVID-19 be the end for the public transit? Investigating the impacts of public health crisis on transit mode choice. Transportation Research Part A: Policy and Practice, 164, 352–378. https://doi.org/10.1016/j.tra.2022.08.020
- Mazanec, J., Harantova, V., Stefancova, V., & Bruhova Foltynova, H. (2023). Estimating Mode of Transport in Daily Mobility during the COVID-19 Pandemic Using a Multinomial Logistic Regression Model. International Journal of Environmental Research and Public Health, 20(5), 4600. https://doi.org/10.3390/ijerph20054600
- Mützel, C. M., & Scheiner, J. (2022). Investigating spatio-temporal mobility patterns and changes in metro usage under the impact of COVID-19 using Taipei Metro smart card data. Public Transport, 14(2), 343–366. https://doi.org/10.1007/s12469-021-00280-2
- Padmakumar, A., & Patil, G. R. (2022). COVID-19 effects on urban driving, walking, and transit usage trends: Evidence from Indian metropolitan cities. Cities, 126, 103697. https://doi.org/10.1016/j.cities.2022.103697
- Palm, M., Allen, J., Liu, B., Zhang, Y., Widener, M., & Farber, S. (2021). Riders who avoided public transit during COVID-19. Journal of the American Planning Association, 87(4), 455–469. https://doi.org/10.1080/01944363.2021.1886974
- Parady, G., & Axhausen, K. W. (2023). Size matters: the use and misuse of statistical significance in discrete choice models in the transportation academic literature. Transportation. https://doi.org/10.1007/s11116-023-10423-y
- Parker, M. E. G., Li, M., Bouzaghrane, M. A., Obeid, H., Hayes, D., Frick, K. T., Rodriguez, D. A., Sengupta, R., Walker, J., & Chatman, D. G. (2021). Public transit use in the United States in the era of COVID-19: Transit riders’ travel behavior in the COVID-19 impact and recovery period. Transport Policy, 111, 53–62. https://doi.org/10.1016/j.tranpol.2021.07.005
- Qi, Y., Liu, J., Tao, T., & Zhao, Q. (2023). Impacts of COVID-19 on public transit ridership. International Journal of Transportation Science and Technology, 12(1), 34–45. https://doi.org/10.1016/j.ijtst.2021.11.003
- Rankavat, S., Gurram, A. R., Pawar, D. S., & Kushwaha, V. (2023). Study of COVID-19 impact on users’ perception for transport modes choice in India. IATSS Research, 47(1), 73–83. https://doi.org/10.1016/j.iatssr.2023.01.005
- Reck, D. J., Martin, H., & Axhausen, K. W. (2022). Mode choice, substitution patterns and environmental impacts of shared and personal micro-mobility. Transportation Research Part D: Transport and Environment, 102, 103134. https://doi.org/10.1016/j.trd.2021.103134
- Ross, G. M. (2021). Public transport and public health: Regulatory focus and the impact of COVID-19 on the choice of public transport mode. Journal of Transport & Health, 22, 101238. https://doi.org/10.1016/j.jth.2021.101238
- Schaefer, K. J., Tuitjer, L., & Levin-Keitel, M. (2021). Transport disrupted - Substituting public transport by bike or car under COVID 19. Transportation Research Part A: Policy and Practice, 153, 202–217. https://doi.org/10.1016/j.tra.2021.09.002
- Shelat, S., Cats, O., & van Cranenburgh, S. (2022). Traveller behaviour in public transport in the early stages of the COVID-19 pandemic in the Netherlands. Transportation Research Part A: Policy and Practice, 159, 357–371. https://doi.org/10.1016/j.tra.2022.03.027
- Soria, J., Edward, D., & Stathopoulos, A. (2023). Requiem for transit ridership? An examination of who abandoned, who will return, and who will ride more with mobility as a service. Transport Policy, 134, 139–154. https://doi.org/10.1016/j.tranpol.2023.02.016
- Tan, L., & Ma, C. (2021). Choice behavior of commuters’ rail transit mode during the COVID-19 pandemic based on logistic model. Journal of Traffic and Transportation Engineering (English Edition), 8(2), 186–195. https://doi.org/10.1016/j.jtte.2020.07.002
- Teixeira, J. F., & Lopes, M. (2020). The link between bike sharing and subway use during the COVID-19 pandemic: The case-study of New York's Citi Bike. Transportation Research Interdisciplinary Perspectives, 6, 100166. https://doi.org/10.1016/j.trip.2020.100166
- Tirachini, A., & Cats, O. (2020). COVID-19 and Public Transportation: Current Assessment, Prospects, and Research Needs. Journal of Public Transportation, 22, 1–21. https://doi.org/10.5038/2375-0901.22.1.1
- Vallejo-Borda, J. A., Giesen, R., Basnak, P., Reyes, J. P., Lira, B. M., Beck, M. J., Hensher, D. A., & Ortuzar, J. D. (2022). Characterising public transport shifting to active and private modes in South American capitals during the COVID-19 pandemic. Transportation Research Part A: Policy and Practice, 164, 186–205. https://doi.org/10.1016/j.tra.2022.08.010
- Van Wee, B., & Banister, D. (2016). How to write a literature review paper? Transport Reviews, 36(2), 278–288. https://doi.org/10.1080/01441647.2015.1065456
- Vickerman, R. (2021). Will Covid-19 put the public back in public transport? A UK perspective Transport Policy, 103, 95–102. https://doi.org/10.1016/j.tranpol.2021.01.005
- Wang, H., & Noland, R. B. (2021). Bikeshare and subway ridership changes during the COVID-19 pandemic in New York City. Transport Policy, 106, 262–270. https://doi.org/10.1016/j.tranpol.2021.04.004
- Xiao, W., Wei, Y. D., & Wu, Y. (2022). Neighborhood, built environment and resilience in transportation during the COVID-19 pandemic. Transportation Research Part D: Transport and Environment, 110, 103428. https://doi.org/10.1016/j.trd.2022.103428
- Yuen, K. F., Choo, L. Q., Li, X., Wong, Y. D., Ma, F., & Wang, X. (2022). A theoretical investigation of user acceptance of autonomous public transport. Transportation, 50(2), 545–569. https://doi.org/10.1007/s11116-021-10253-w
- Zafri, N. M., Khan, A., Jamal, S., & Alam, B. M. (2023). Impact of COVID-19 on public transport usage in an anticipated ‘new normal’ situation: The case of a South Asian country based on first wave data. Asian Transport Studies, 9, 100099. https://doi.org/10.1016/j.eastsj.2023.100099
- Zhang, X., Liu, D., Wang, Y., Du, H., & Miwa, T. (2021). Behavioral Intentions of Urban Rail Transit Passengers during the COVID-19 Pandemic in Tianjin, China: A Model Integrating the Theory of Planned Behavior and Customer Satisfaction Theory. Journal of Advanced Transportation, 2021, 1–12. https://doi.org/10.1155/2021/8793101
- Zhao, P., & Gao, Y. (2022). Public transit travel choice in the post COVID-19 pandemic era: An application of the extended Theory of Planned behavior. Travel Behaviour and Society, 28, 181–195. https://doi.org/10.1016/j.tbs.2022.04.002