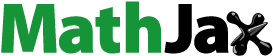
ABSTRACT
For users to adopt information systems, they must develop trust in such systems. Even though trust theories consistently define trust as dynamic, the development of trust over time has received little empirical attention. The present study examined the development of trust in a newly introduced information system and its association with antecedents related to the individual (e.g. disposition to trust), the information system (e.g. reliability), and the context (e.g. support) at different time points. We further assessed users’ reliance, performance, and well-being as outcomes of trust. Employees (N = 313) of a German public university assessed a newly introduced invoice processing system on four occasions (before system launch, after initial use, five months after launch, ten months after launch). Results from latent growth curve modelling show a non-linear increase of trust in the information system over time with changing predictors: Person factors were stronger predictors of trust in early phases, whereas system characteristics were stronger predictors later in the process. Moreover, users’ trust in the information system correlated positively with reliance, performance, and well-being. Our results highlight the central role of trust for the successful adoption of information systems at work, and offer specific suggestions for their building and maintenance.
1. Introduction
Organisations are increasingly introducing information systems to successfully handle the growing complexities of their daily work processes (e.g. Brauner et al. Citation2019; Kappelman et al. Citation2018). Indeed, usage of information systems has been shown to positively affect users’ performance, decision quality, and well-being (Petter, DeLone, and McLean Citation2008; Stone, Good, and Baker-Eveleth Citation2007). Prior studies have also revealed that information system usage is facilitated by users’ trust in the system (Hertel et al. Citation2019; Lippert Citation2007; Turel and Gefen Citation2013). The significance of this insight cannot be overstated, because it can help explain why some adoption initiatives succeed and others fail (Bahmanziari, Pearson, and Crosby Citation2003; Lippert and Davis Citation2006; McKnight Citation2005).
However, important questions on users’ trust in information systems remain unanswered. In particular, it is not sufficiently understood how trust in information systems develops over time. Although theoretical models assume such trust to develop dynamically (Hoff and Bashir Citation2015; Hu et al. Citation2019; Rousseau et al. Citation1998), empirical evidence is yet lacking, in line with a general neglect of longitudinal designs in information systems research (Hoehle, Huff, and Goode Citation2012; Zheng, Pavlou, and Gu Citation2014). Moreover, theoretical models remain rather general on the specifics of such developments. While some models make assumptions on different phases of trust development (Rousseau et al. Citation1998; Söllner and Pavlou Citation2016), little is known about the exact trajectories of trust in information systems in the workplace. Indeed, trust might develop in quite different ways: For example, trust might start low and then steadily increase with time. Alternatively, users might have unrealistically high expectations toward a novel system (e.g. Glikson and Woolley Citation2020), leading to rapidly decreasing trust because the system does not work reliably, for instance. A third possibility is that medium initial trust levels are followed by phases of increasing, stable, and decreasing trust levels. Understanding trust development in work-related information systems over time is thus important for epistemic reasons. Moreover, a more thorough understanding would enable organisations to better prepare for and conduct the introductions of information systems. This includes establishing both, targeted preventions and interventions for trust building at the right time points.
Moreover, it is not yet known whether and how the relevance of different antecedents of trust might develop with time, i.e. whether certain antecedents are more or less important at certain phases of trust development. Despite considerable knowledge on trust antecedents in general (i.e. user attributes, system characteristics, context, and service structures, e.g. DeLone and McLean Citation2003; Thielsch, Meeßen, and Hertel Citation2018), research also needs to address potential dynamics in the relevance of these antecedents across time. Specifically, different antecedents might have varying influences at different stages of trust development (Bijlsma and Koopman Citation2003; Dietz and den Hartog Citation2006; McKnight Citation2005; van der Werff and Buckley Citation2017). Having such knowledge would contribute to the development of hands-on practical advice for organisations to steadily increase users’ trust in information systems.
Taken together, the major goals of our study are to empirically validate and extend initial theoretical assumptions on different phases of trust development in information systems and to consider the interplay between such trust trajectories and the relative importance of trust antecedents and outcomes. The following research questions are addressed in this research: (1) How does trust in an information system develop over time? (2) How is trust in information systems related with different trust antecedents at different time points? (3) How is trust in information systems related with different outcomes across time?
Building on theories of trust in technology and information systems (McKnight et al. Citation2011; Meeßen, Thielsch, and Hertel Citation2020), the present study addresses trust development in a newly introduced information system. Specifically, we examined the trajectory of trust in a new information system over a period of 11 months and four waves of data. In addition, we considered person-related (i.e. disposition to trust), system-related (e.g. reliability), and context-related (e.g. support) trust antecedents and examined their relative relevance at different time points. Finally, we measured different outcomes of trust in information systems, including users’ reliance on the system, performance, and well-being.
Our study has various implications for theory and practice. Building on trust theories that consider trust as a dynamic construct (e.g. Hoff and Bashir Citation2015; Lee and See Citation2004; Rousseau et al. Citation1998; van der Werff et al. Citation2019), our study is one of the first empirical investigations of trust in information systems at work using a truly longitudinal design. In doing so, this study contributes to existing theory by examining trust trajectories more deeply as well as trust relations with its antecedents and outcomes at different stages of system introductions. Further, our study is situated in an actual working context and follows a full-cycle approach (Mortensen and Cialdini Citation2010), constituting the validation of theoretical considerations and experimental laboratory findings (Hertel et al. Citation2019; Meeßen et al. Citation2020) within the field. Finally, our study identifies success factors for adopting an information system in the work context with respect to the system itself as well as organisational context and service structures.
2. Theoretical background
2.1. Information system adoption at work
Information systems support working environments that are characterised by increasing data amounts and complexity of data (e.g. Kappelman et al. Citation2018). By collecting, processing, organising, storing, or distributing information, information systems support data analysis, control, coordination, visualisation, and decision-making in organisations (Rainer and Prince Citation2021). However, despite these capabilities of information systems, many adoption processes fail because users do not use the new systems (in the intended ways) (e.g. Campbell and Grimshaw Citation2016; Kim and Pan Citation2006; Korpelainen and Kira Citation2013). Factors that improve system acceptance and usage have therefore been central in information systems research. For instance, DeLone and McLean (Citation2003) identified three success factors of information system usage within their information system success model: Information quality (i.e. accuracy, timeliness, completeness, relevance, and consistency of information provided by the system), system quality (i.e. usability, availability, reliability, adaptability, and response time of the system), and service quality (i.e. the overall support delivered by the service provider).
More recent research extended the information system success model (DeLone and McLean Citation2003) and additionally included trust as a central success factor for overcoming risks associated with using information systems (i.e. lack of control, potential errors; e.g. Thielsch, Meeßen, and Hertel Citation2018). Trust was introduced to information technology research within earlier theoretical conceptualisations (Lee and See Citation2004; McKnight et al. Citation2011), that differentiated human-technology trust from human-human trust. Showing that humans can develop trust in a technology itself, and not only in its human surrogates, this work paved the way for investigating and emphasising the role that trust plays as users develop usage intentions and behaviours toward a technology. Based on these theoretical foundations, Meeßen, Thielsch, and Hertel (Citation2020) recently developed a model on trust in information systems, that presumes information system adoption and trust development to be dynamic. Specifically, the authors assumed that trust in an information system leads users to develop usage intentions, which ultimately result in the use of the system, thereby enabling users to (re)evaluate their trust in the system in repeated feedback cycles.
2.2. Trust in information systems and its dynamics
We follow McKnight and his colleagues by defining trust in technology as the ‘belief that a specific technology has the attributes necessary to perform as expected in a given situation in which negative consequences are possible’ (McKnight et al. Citation2011, 7). More specifically, trust in information systems describes the willingness of a user to depend on and be vulnerable toward the system in uncertain and risky situations (Gefen, Benbasat, and Pavlou Citation2008). Users are vulnerable toward information systems for at least two reasons. First, users cannot completely control information systems because the systems usually exceed the information processing capacities of human users, and the exact functioning of an information system largely stays unclear to its end-users (Chen, Chiang, and Storey Citation2012; Lee and Gao Citation2005; see also Shin Citation2021 for AI systems). Second, information systems are not always free from error: Incorrect programming, impaired algorithms, or misapplication by other users represent risks of using information systems that might negatively affect work results, performance evaluation, and status at work (Brauner et al. Citation2019).
Empirical studies suggest that trust in information systems can help users to overcome these uncertainties and risks (van der Heijden, Verhagen, and Creemers Citation2003; Wang, Ngamsiriudom, and Hsieh Citation2015). Specifically, if users trust a technology, they have been found to build usage intentions more often, which in turn results in higher usage rates (e.g. Gefen, Karahanna, and Straub Citation2003; Pavlou and Gefen Citation2004; Sharma and Sharma Citation2019). When trust is lacking, however, users are more likely to avoid using an information system and develop counterproductive behaviours, such as additional workarounds (McKnight Citation2005; Thielsch, Meeßen, and Hertel Citation2018). In these cases of low trust, information systems provide low value for their users as well as the organisation (Shin Citation2020, Citation2022). If trust, however, is apparent, it has been shown to function as a precondition of the beneficial effects such systems can provide, such as freeing up users’ cognitive capacities (Hertel et al. Citation2019), increasing performance, and improving well-being (Meeßen et al. Citation2020; Tam, Loureiro, and Oliveira Citation2019).
Even though earlier studies have revealed important insights into the role of trust in information system usage, most used cross-sectional designs focusing on only one (initial) interaction between users and systems (Hoehle, Huff, and Goode Citation2012), neglecting the theoretical conceptualisation of trust as a ‘dynamic concept that is prone to changes based on the behavior of the trusted agent’ (Glikson and Woolley Citation2020, 10; Crisp and Jarvenpaa Citation2013; Hoff and Bashir Citation2015; Lee and See Citation2004; Schoorman, Mayer, and Davis Citation2007). Trust can develop and change when the relationship between the trustor (the party who trusts) and the trustee (the target of trust) matures (Audrey Korsgaard Citation2018), such that the term trust dynamics describes the evolution of trust over time (e.g. Cabiddu et al. Citation2022; Govindan and Mohapatra Citation2012). Prior studies’ focus on users’ initial interaction with a system, however, leaves assumptions on these dynamics largely uninvestigated (see also Lin et al. Citation2014; Shin, Lee, and Hwang Citation2017; Venkatesh et al. Citation2011).
Notably, initial theoretical models exist that describe and predict trust development, mainly for interpersonal or inter-organisational interactions. For instance, Rousseau et al. (Citation1998) assumed three phases of trust emergence: (1) building (trust (re)formation), (2) stability, and (3) dissolution. Söllner and Pavlou (Citation2016) focused on the development of human-technology trust and proposed a trust lifecycle consisting of six phases. Accordingly, trust relationships start with initial trust levels (phase 1), which are either confirmed or disconfirmed based on first interactions (phase 2). Then, trust builds in linear patterns within further interactions (phase 3), and stabilises after some time (phase 4). Negative experiences with the target of trust can lead to trust dissolutions (phase 5), so that trust needs to be repaired (phase 6) to finally result in a stable state again. However, these assumptions have not yet been examined empirically in contexts of occupational work.
Theories on trust development suggest that the repeated usage of a technology influences its users’ trust levels in the system (Hoff and Bashir Citation2015; Lee and See Citation2004; Lippert and Swiercz Citation2005; Söllner and Pavlou Citation2016). Specifically, usage experiences increase users’ knowledge of an information system’s functioning, which is likely to contribute to higher levels of trust by reducing associated uncertainties and perceived risks and by increasing feelings of safety, reliance, and familiarity (Ashraf et al. Citation2020; Meeßen, Thielsch, and Hertel Citation2020). System usage, thus, not only represents a trust outcome but is also a precursor for the assessment of the system’s trustworthiness in subsequent usage, and enables its ongoing (re)evaluation (Hoff and Bashir Citation2015; Mou, Shin, and Cohen Citation2017). Trust, therefore, develops based on a history of interactions between the user and the system over a period of time. Positive user experiences especially increase the evaluation of an information system’s trustworthiness, which in turn positively predicts consecutive trust (Hoff and Bashir Citation2015; Meeßen, Thielsch, and Hertel Citation2020). Thus, trust in information systems is assumed to lead to more trust, therefore being a ‘self-reinforcing phenomenon’ (van der Werff and Buckley Citation2017, 746; see author Audrey Korsgaard Citation2018). We, therefore, assumed that trust develops in upward trajectories and hypothesised:
H1: Trust in an information system increases with time.
2.3. Trust antecedents
Models on trust in technology and information systems (Lippert and Swiercz Citation2005; McKnight et al. Citation2011; Meeßen, Thielsch, and Hertel Citation2020) suggest that a user’s trust depends on the trustor’s disposition (i.e. the user’s disposition to trust) and the system’s trustworthiness (e.g. its reliability and credibility). Indeed, empirical studies generally support positive relationships of users’ trust dispositions and the system’s trustworthiness with trust in technology (Costante, den Hartog, and Petkovic Citation2011; Hoff and Bashir Citation2015; Wang, Ngamsiriudom, and Hsieh Citation2015; Yu, Balaji, and Khong Citation2015). However, we know very little about the dynamics of the relationship between an information system’s trustworthiness and users’ trust in this system and how these relationships develop over time as users become more experienced with the system. Similarly, studies have yet to address how a user’s disposition to trust technology relates to trust in information systems at earlier and later points in time.
Trust disposition toward technology has been defined as a person’s ‘general tendency to be willing to depend on technology’ across situations and technologies (McKnight et al. Citation2011, 7). McKnight et al. (Citation2011) further operationalised trust disposition through two factors, namely (1) users’ faith (the tendency to assume that technologies have favourable attributes), and (2) their trusting stance in general toward technology (the assumption that technology can be relied upon).
We argue that a user’s disposition to trust technology is positively related to the user’s initial trust in information systems. When users use an information system for the first time, they know very little about its features and its functioning. Such early interactions are therefore characterised by users having high uncertainty and ambiguity (Gill et al. Citation2005). If little information on the system (i.e. the trustee) is available, users rely on more general attitudes and dispositions, making users’ disposition to trust technology a central determinant of trust at early time points (Meeßen, Thielsch, and Hertel Citation2020; Schoorman, Mayer, and Davis Citation2007). Users’ disposition to generally trust technology can provide them the ability to make a leap of faith and show trusting behaviours, irrespective of knowledge on the system’s actual trustworthiness (Mayer, Davis, and Schoorman Citation1995; Wang, Ngamsiriudom, and Hsieh Citation2015). We therefore assumed:
H2: Users’ disposition to trust technology is positively related to their initial trust in an information system (i.e. before system launch).
We additionally argue that with time and experience with the information system, trust disposition should become less important for users’ trust in the information system. At the beginning of information system adoption, when users cannot assess whether they can trust a particular information system based on information about the system itself, trust disposition should be a major determinant of trust. Its importance should, however, diminish as users gather more specific information about the system on which to base their trust assessment (Gefen, Benbasat, and Pavlou Citation2008). More general person dispositions, which are unspecific to contexts, situations, and technologies (McKnight et al. Citation2011), should, therefore, become less relevant for trust over time. Instead of relying on their general trust tendencies, users will, over time, gain the information to make reasoned trust decisions based on their experiences. We therefore predicted:
H2a: The strength of the relationship between disposition to trust technology and trust in an information system decreases over time as users use the system.
In addition to users’ general disposition to trust technology, we argue that a system’s perceived trustworthiness is positively associated with users’ trust in the system. Notably, perceived trustworthiness has been explicitly distinguished from trust within extant accounts (Gefen Citation2002; Mayer, Davis, and Schoorman Citation1995). Whereas trust represents an emergent state of willingness to depend on and be vulnerable toward a trustee (i.e. the information system), perceived trustworthiness describes the trustor’s evaluation of the trustees’ characteristics (i.e. system features, service structures) (Mayer, Davis, and Schoorman Citation1995; Little Citation1988). Earlier research has already found technology trustworthiness to consist of different facets. For instance, McKnight et al. (Citation2011) distinguished three factors of technology trustworthiness: functionality (i.e. the extent to which users can fulfil their intended tasks with the help of the technology), reliability (i.e. the extent to which the technology works continually and accurately), and helpfulness (i.e. the extent to which the technology helps the user). Later research by Thielsch, Meeßen, and Hertel (Citation2018) built on the information system success model (DeLone and McLean Citation2003) and found that information quality (i.e. credibility of the information provided), system quality (i.e. reliability of the system), as well as context and service quality (i.e. support structures, participation, and involved persons perceived ability) are predictors of trust.
We argue that higher trustworthiness perceptions of the system are related to higher trust in the system. More specifically, we predicted trust to be positively affected by both, the information system’s properties and organisational context and service structures. In line with Thielsch, Meeßen, and Hertel (Citation2018), we argue that an information system’s properties consist of its reliability, the credibility of provided information, its usability, and its design aesthetics. Generally, users consider these properties when they assess whether they can trust the system (Lee and See Citation2004; McKnight et al. Citation2011; Muir Citation1994). Specifically, the more users perceive that an information system has the necessary properties to perform well (i.e. working without malfunctions, producing believable outputs), and to fulfil their needs when mastering a situation (i.e. being easy to use, having an appealing design), the more they should trust it (Beldad, de Jong, and Steehouder Citation2010; McKnight et al. Citation2011) We predicted:
H3: Properties of an information system (reliability, credibility, usability, design aesthetics) are positively related to trust in the information system after system launch.
Further, we argue that trust in an information system is positively related to organisational context and service structures. These include the availability of support structures that information system users can draw upon when problems occur, a degree of participation when it comes to decisions affecting the system, and the abilities of involved persons that are responsible for the system (Thielsch, Meeßen, and Hertel Citation2018). The role of organisational context and service structures for information system trust has been stressed in prior information systems research (Cheung and Lee Citation2006; Thatcher et al. Citation2011; Venkatesh and Davis Citation2000). Accordingly, trust in an information system is not only influenced by the properties of the system itself but also by broader structures surrounding the system (Lippert and Swiercz Citation2005; Thatcher et al. Citation2011). If users perceive that such structures exist and, therefore, that they are being given enough professional assistance, especially when problems occur, this should positively affect users’ trust in the information system itself. We assumed:
H4: Organizational context and service structures (support, participation, abilities of involved persons) are positively related to trust in the information system after system launch.
Simultaneously, we assumed that the significance of the relationships between the different trustworthiness components and trust changes with time. Within their model on trust in information systems, Meeßen, Thielsch, and Hertel (Citation2020) argued that the effect of trustworthiness perceptions on trust is moderated by usage experiences with the system. Specifically, experiences with the system should moderate the relationship between trustworthiness and trust, so that more experiences strengthen the relationship. The more users use a system, the more they can reliably assess the system’s trustworthiness (Chang et al. Citation2010). Therefore, we proposed that the strength of the relationship between the system’s perceived trustworthiness and trust in the information system increases with time and experience:
H3a: The strength of the relationship between properties of an information system and trust in the system increases over time as users use the system.
H4a: The strength of the relationship between organizational context and service structures and trust in the system increases over time as users use the system.
2.4. Trust outcomes
In work contexts, trust in specific technologies has been shown to be positively related to behavioural intentions to use the technology (Lu et al. Citation2011), user satisfaction (Kassim et al. Citation2012), and customer acceptance (Suh and Han Citation2002). Similarly, trust in information systems is positively correlated with performance, well-being, and post-usage satisfaction (Tam, Loureiro, and Oliveira Citation2019; Thielsch, Meeßen, and Hertel Citation2018). Further, initial research has also demonstrated that trust in an information system increases a system’s positive effects on users’ cognitive resources and well-being. In a recent study (Hertel et al. Citation2019), participants in a simulated business context were able to save cognitive capacities for additional tasks and reported higher well-being when using an information system as compared to a control group. Interestingly, these positive effects only occurred for participants who trusted the system to a certain extent (Hertel et al. Citation2019).
We argue that trust in an information system is positively related to reliance on the information system, with one main indicator being forgetting old work processes. In line with findings from research on directed forgetting (Hertel et al. Citation2019; Meeßen et al. Citation2020), users of information systems should rely on the system’s functions more the more that they trust them. Generally, the introduction of a new information system represents a change process (e.g. Emad Citation2010), during which employees need to adapt their work routines from old processes to new processes. If work processes have been performed by employees themselves over a long period, replacing these procedures via using the system implies a certain degree of reliance on the system (Bresnen, Goussevskaia, and Swan Citation2005; Lindkvist et al. Citation2017). If users trust the system and its ability to perform the work process, they should be more willing to rely on the system and thus forget old work processes. We predicted:
H5: Trust in an information system is positively related to users’ reliance on the system.
We additionally argue that trust in the information system is positively related to users’ performance. The reliance on the information presented by the system that is relevant for task executions can lead to time savings, higher work quality, and task simplifications (Bravo, Santana, and Rodon Citation2015; Etezadi-Amoli and Farhoomand Citation1996; Petter, DeLone, and McLean Citation2008). If users, however, do not trust the system and its information, resulting behaviours (e.g. double-checking information) may in turn negatively affect performance (e.g. by increasing the time of task completion). Conversely, the more users trust the information system, the more they should rely on the information presented, increasing their performance (Lee and See Citation2004). Indeed, trust in an information system has already been shown to be positively related to performance (e.g. Hertel et al. Citation2019; Meeßen et al. Citation2020; Müller et al. Citation2020). We predicted:
H6: Trust in an information system is positively related to users’ performance.
Finally, we predicted trust to be associated with the affective experiences of system users. Specifically, we assumed that trust in an information system is negatively associated with users’ perception of strain while using the system. Completing work tasks can generally be considered a stressor, especially when tasks require the processing of large amounts of information, when time pressure is evident, or when results are associated with one's reputation in the organisation (Cavanaugh et al. Citation2000; de Jonge and Dormann Citation2006; Mazzola and Disselhorst Citation2019). Such stressors can, however, be compensated by the availability of resources (Bakker and Demerouti Citation2007). If task completion is supported by an information system that fits users’ needs for completing tasks and is trusted and relied upon, information systems should act as such a resource. Consequently, users should feel greater relief since the information system supports their task completion (Turetken, Ondracek, and Ijsselsteijn Citation2019). This, in turn, is likely to reduce users’ strain perceptions during task completion. Further, the system usage itself can lead to increased feelings of strain, for instance if the system works very unreliably. Again, high trust in the information system, for instance to work reliably, should reduce strain perceptions during system usage. The positive influence of trust in an information system on the well-being of users by being negatively associated with strain perceptions has already been demonstrated in laboratory settings (Hertel et al. Citation2019; Meeßen et al. Citation2020; Müller et al. Citation2020). We predicted:
H7: Trust in an information system is negatively related to users’ strain during usage.
Our research model is depicted in .
3. Method
3.1. Participants and setting
Participants in this study were N = 313 employees of a large German public university. The university generally is divided into a central administration, including several departments, staff units, as well as decentralised divisions, including the university’s faculties and institutes. The university in question is in the process of digitalising its administrative processes. For instance, the hiring of academic staff and virtual team collaboration are well organised and work with elaborated digital support. Yet, other processes are still in their infancy as far as digitalisation is concerned (e.g. selection process for masters’ students is done manually).
Likewise, invoices have previously been processed analogously. First, they were received centrally and forwarded via internal, physical mail to the responsible persons, who further processed the invoices with the help of an account stamp, released them for payment, and again forwarded them to the responsible department via mail. To digitalise this procedure, an information system capable of invoice processing was introduced to the organisation’s processes on 18 August 2020. With its introduction, the organisation followed the prescription that by November 2020, German administrations had to be able to receive and process invoices electronically (ERechV 2017, 2020). While invoices needed an average of 10.54 days to be fully processed via the previous, analog invoice processing approach (average processing time across all invoices from the last five months of analog invoice processing), its digitalisation reduced the average time of invoice processing by more than eight days to 2.26 days (average processing time across all invoices from the first five months of system usage).
All employees who were required to use the newly introduced electronic invoice system were potentially relevant participants in the study. Participants were allowed to start and withdraw from the study at any time so out of 1176 potential participants, 26.6% chose to participate at any of the four time points. The sample’s average age was 46.18 years (SD = 10.64, Min = 21, Max = 65), and 73% of the participants were female. Participants were employed at the organisation for M = 14.85 years (SD = 10.98, Min = 0, Max = 58), 36% worked in its central administration, and 62% worked in decentralised divisions.
3.2. Research design
The study followed a longitudinal design with four waves of data collection. Following previous longitudinal trust studies (van der Werff and Buckley Citation2017), we collected data over 11 months, with four surveys distributed unevenly over the period. Specifically, we collected data about a month before the information system’s launch (T0; July 2020), shortly after its initial use (T1; August 2020), five months after its launch (T2; January 2021), and ten months after its launch (T3; June 2021). This design allowed us to examine short-, mid-, and long-term attitudes toward the information system and compare them to pre-usage expectations.
The study was designed in close cooperation with the employees responsible for the introduction of the information system, who provided both, a content-related introduction to invoice processing and the identification of potential participants. All those employees of the organisation tasked with processing invoices in their daily work were identified as potential participants. These included employees from the central administration as well as employees from decentralised divisions. The introduction of the information system was communicated within the organisation through multiple channels (emails, employee portal). Further, so-called multipliers were trained for system usage around half a year before its launch. Multipliers were instructed to share their knowledge with their colleagues, but no mandatory training events for all users were conducted. Theoretically, it could have been the case that users of the system got to know the system only after its launch. The study was approved by the faculty’s ethics committeeFootnote1 as well as the university’s personnel board.
3.3. Procedure
The study was conducted online. Employees eligible for the study received an invitation email at each of the four time points with a link to the study. The potential participants were informed about the study’s objectives and longitudinal nature, as well as that participation was voluntary and without financial incentives. At each time point, we sent a reminder email two weeks after the first invitation. Each survey was online for about three weeks and participants could fill it out within working hours. Participation at each time point took approximately 20 to 25 minutes.
To correctly match the data of each person from the different time points, an eight-digit personal code was used, which consisted of various personal details (e.g. number of letters in the mother's first name) and was requested at each of the four surveys. Participants also received detailed information on the study and data processing at the beginning of each survey and were asked to give their informed consent on their participation. At T0, participants were asked to rate their current trust in the upcoming system, their general trust disposition as well as control and demographic variables. Control variables included technology competence, conscientiousness, and need for control. Demographics included sex, age, duration of employment, and department. Finally, participants were again asked to give their consent on their data usage and could give feedback on the study.
At T1 to T3, participants were first asked whether they had already participated at one of the former time points. For those who did not participate already or could not certainly recall whether they did, the study also included a rating of trust disposition, control variables, and demographic variables after the other scales were presented. Those who already participated did not have to answer these scales again. Within each of the time points, we collected users’ perceptions of the system’s reliability, credibility, usability, and design aesthetics as well as of the provided support structures, their perceived participation, and the abilities of involved persons. Measures assessing trust in the system were followed by measures assessing users’ reliance, performance, and strain.Footnote2 Procedures of the study at the different time points are summarised in .
3.4. Measures
Participants rated all items on a 7-point Likert scale (1 = fully disagree to 7 = fully agree) if not stated otherwise. All items were provided in German. For scale reliabilities, we calculated Cronbach’s alpha () for scales with three or more items, and Spearman-Brown correlations (
) for two-item scales. Reliabilities can be found in the next sections and are additionally displayed in the main diagonal of .
We measured trust disposition on a three-item scale adapted from Lankton, McKnight, and Tripp (Citation2015) and McKnight, Choudhury, and Kacmar (Citation2002) (e.g. ‘I usually trust computer technology until it gives me a reason not to trust it’; = .83). We used a three item-scale to measure the information system’s reliability (McKnight et al. Citation2011; e.g. ‘The system is very reliable’;
= .92–.93) and the Web-CLIC’s subscale to measure its credibility (Thielsch and Hirschfeld Citation2019; three items; e.g. ‘The information provided by the system is credible’;
= .95–.97). The two-item UMUX-LITE was used to measure the information system’s usability (Lewis, Utesch, and Maher Citation2013; e.g. ‘The capabilities of the system meet my requirements’;
= .68–.69). A single holistic item was used to measure the information system’s design aesthetic (Thielsch, Meeßen, and Hertel Citation2018; ‘I find the system’s design appealing'). We used two items from a scale developed by Thielsch, Meeßen, and Hertel (Citation2018) to measure support structures (e.g. ‘If problems occur with the system, support is available’;
= .62–.74), a two-item scale to measure participation (Baroudi and Orlikowski Citation1988; e.g. ‘I can make change requests and adjustments that concern the system’;
= .63–.70), as well as a three item-scale to measure the involved persons’ abilities (Hertel, Konradt, and Orlikowski Citation2004; e.g. ‘I trust in the professional competence of the people responsible for the system’;
= .80–.96). Trust in the information system was measured on a three-item scale developed and successfully used in prior studies (Hertel et al. Citation2019; Meeßen et al. Citation2020; Thielsch, Meeßen, and Hertel Citation2018; e.g. ‘I completely trust the system’;
= .92–.95). We measured performance on a four-item scale developed by Etezadi-Amoli and Farhoomand (Citation1996; e.g. ‘Using the system improves the quality of my work’;
= .93–.95). Strain was measured using the stress in general scale developed by Stanton et al. (Citation2001; three items; e.g. ‘I find working with the system exhausting’;
= .93). Reliance was measured through users’ forgetting of a work process. Therefore, we identified a work process that was previously performed by the user but was now taken over by the system and, therefore, could be forgotten. The process was copying and printing invoices, which was required before but not under usage of the system, as invoices are processed and saved electronically. We asked participants to rate the frequency of the process occurrence on a 7-point scale ranging from 1 = never to 7 = several times a day at each time point and calculated the differences between T0 and the other three time points as reliance measures. As control variables, we measured participants’ technology competence (four items adapted from Neyer, Felber, and Gebhardt (Citation2012); e.g. ‘For me, dealing with new computer technology is mostly a challenge’;
= .86), conscientiousness (three items adapted from Körner et al. (Citation2008); e.g. ‘I try to be very conscientious in performing all the tasks assigned to me’;
= .83) and need for control (three items adapted from de Rijk et al. (Citation1998); e.g. ‘I place a high value on being in control of what I do and how I do it’;
= .81). Construct validity of the scales was confirmed by high intercorrelations among the scales’ items, which was complemented by high values in item factor loadings, and scales’ composite reliabilities (CR) and average variances extracted (AVE) (e.g. Hair et al. Citation2014). Discriminant validity was checked for using the Fronell-Larcker Criterion (Fornell and Larcker Citation1981), confirming it for each scale. A full list of scales and items used within our study and the validity assessments can be found in the Appendix.
3.5. Common method bias and multicollinearity
Given our reliance on self-reports and single sources, we applied various measures to control for potential common method biases (MacKenzie and Podsakoff Citation2012). For statistical control, we performed Harman’s single factor test. Results of the unrotated factor solutions for the three measurement points T1 to T3 revealed that a single factor accounted for between 45 to 46% of the variance. Therefore, no general factor accounted for the majority (i.e. > 50%) of variance. Furthermore, we performed confirmatory factor analyses with the assumed factor structure (twelve factors: trust disposition, reliability, credibility, usability, design aesthetics, support, participation, persons involved, trust, reliance, performance, and strain) and compared it to a one-factor model, as well as other plausible alternative models (three-factor model and seven-factor model). Results revealed that the hypothesised twelve-factor model fitted our data acceptably, and significantly better than the alternative one-factor, three-factor, and seven-factor models (see ). Thus, results speak against an overall common method factor and once again support the distinctiveness of our constructs.
Table 1. Confirmatory factor analyses comparing the hypothesised twelve-factor model to alternative models for the first measurement point (T1).
Table 2. Confirmatory factor analyses comparing the hypothesised twelve-factor model to alternative models for the second measurement point (T2).
Table 3. Confirmatory factor analyses comparing the hypothesised twelve-factor model to alternative models for the third measurement point (T3).
Finally, we tested multicollinearity by regressing trust predictors on trust, and calculating tolerance (<.01 indicating multicollinearity) and VIF-values (>10 indicating multicollinearity). Tolerance values ranged from .207 to .950, while VIF values ranged from 1.05 to 4.84. These results suggest that multicollinearity is unlikely to be a major concern in our data (Hair et al. Citation2010).
4. Results
Data were analysed using IBM SPSS Statistics (Version 28) and IBM SPSS Amos Graphics (Version 28). Our data set included missing data (46.61% missing values) since all participants were included that participated in at least one of the four time points. We used full information maximum likelihood (FIML) to handle missing data. The FIML procedure enables the use of all available information by integrating the likelihood function over the variables with missing data, which maximises closeness of fit (Wothke Citation2000). FIML has been shown to produce unbiased estimates of standard errors in the presence of missing data (Enders and Bandalos Citation2001) and has been successfully used in past longitudinal studies with high attrition rates (e.g. Galambos, Barker, and Krahn Citation2006; McClelland, Acock, and Morrison Citation2006). Since FIML requires missingness to be at random (MAR; Enders and Bandalos Citation2001; Wothke Citation2000), we conducted t-tests comparing participants with and without missing data in age, sex, duration of employment, technology competence, trust disposition, conscientiousness, and need for control. None of these were significant. We additionally performed the Little test (Little Citation1988), which tests for missing values occurring purely randomly, therefore allowing for the global assessment of missingness completely at random (MCAR). The Little test supported the assumption of missingness to be completely at random. Our sample, therefore, met the requirements for using the FIML procedure.
Before testing hypotheses, we tested measurement invariance as a precondition for latent growth modelling. Four goodness-of-fit indices were used for interpreting model fit: (1) chi-square, (2) comparative fit index (CFI; Bentler Citation1990), (3) Tucker Lewis index (TLI; Bentler and Bonett Citation1980), and (4) root mean square error of approximation (RMSEA; Steiger Citation1990). Descriptive statistics for all variables including intercorrelations and scale reliabilities can be found in . All scale reliabilities exceeded the recommended threshold of Cronbach’s > .70 (for scales with three or more items; Streiner Citation2003) or rSB > .60 (for scales with two items; Eisinga, te Grotenhuis, and Pelzer Citation2013).
Table 4. Bivariate correlations among the variables, descriptive statistics, and scale reliabilities.
4.1. Measurement invariance
Measurement invariance testing can be used to check for the stability of the conceptual framework participants relied on when responding to survey items on trust. Following the guidelines by Vandenberg and Lance (Citation2000), we computed four models (configural invariance, metric invariance, scalar invariance, and partial scalar invariance) and used the likelihood ratio test (Bollen Citation1989) and changes of .01 or greater in CFI (Cheung and Rensvold Citation2002) to compare the models. shows the results of our measurement invariance tests.
Table 5. Measurement invariance tests.
We first assessed configural invariance to test whether free and fixed loadings of trust patterns were the same across time points. Results showed good model fit, (30, N = 313) = 49.84, p < .05, CFI = .99, TLI = .97, RMSEA = .05, indicating that stable loadings of trust items at the different time points were warranted. In a second step, we assessed metric invariance by constraining factor loadings to be equal at each time point. The comparison of the fit indices between the configural and metric model (
(36, N = 313) = 57.29, p < .05, CFI = .99, TLI = .97, RMSEA = .04) indicated no significant decrease in model fit and, therefore, metric invariance. Finally, by additionally constraining item intercepts to be equal across time points, we assessed scalar invariance. Results revealed a significant chi-square difference and change in CFI (
(42, N = 313) = 76.22, p < .05, CFI = .98, TLI = .96, RMSEA = .05) and, therefore, the existence of some level of non-invariance of item intercepts. To achieve partial scalar invariance, we identified items that showed higher levels of variance over time (Yoon and Millsap Citation2007). We identified two items at T0 (‘I heavily rely on the system’ and ‘I am comfortable with relying on the system’) as having the highest levels of variance and removed constraints on these items’ intercepts. The resulting model’s chi-square decreased slightly on a non-significant level as compared to the metric model,
(40, N = 313) = 59.68, p < .05, CFI = .99, TLI = .97, RMSEA = .04, so that criteria on partial scalar invariance could be met (Putnick and Bornstein Citation2016). It is, therefore, feasible to conclude that trust has sufficiently stable properties over the four time points so that further longitudinal analyses could be conducted.
4.2. Hypotheses testing
We used univariate latent growth modelling to test our hypothesis on the growth of trust over time. To assess the structure of the factor residuals and determine whether change in trust was linear, we compared linear to optimal growth models. Linear change was modelled by fixing the change factor loadings in the model equal to 0, 1, 6, and 11 to reflect the unevenly spaced measurement occasions (Lance, Vandenberg, and Self Citation2000). An increase of 1 represents an interval of 1 month. Optimal change was modelled by fixing the first two change factor loadings to 0 and 1 and leaving the second two free to be estimated. For both models, we additionally compared heteroscedastic models (with freely estimated residual variances) to homoscedastic models (with equal residuals; Willett and Sayer Citation1994). The optimal heteroscedastic Amos model is depicted in .
Results of the four resulting univariate models are displayed in . These indicated that the optimal change function significantly increased the model fit as compared to the linear model ((5, N = 313) = 16.35, p < .01, CFI = .92, TLI = .83, RMSEA = .09). Constraining the residual variances, however, resulted in a poorer model fit for both, linear (
(8, N = 313) = 39.49, p < .001, CFI = .77, TLI = .71, RMSEA = .11) and optimal (
(6, N = 313) = 32.10, p < .001, CFI = .81, TLI = .68, RMSEA = .12) models. We therefore accepted the optimal heteroscedastic model as the most accurate representation of change in trust over time,
(3, N = 313) = 10.14, p < .05, CFI = .95, TLI = .82, RMSEA = .09. The mean latent growth curve of trust over time is depicted in .
Table 6. Goodness-of-fit indices and difference tests for univariate latent growth model comparisons.
Parameter estimates of the accepted model can be found in . Slope factor means were positive and statistically significant (b = .335, SE = .111, p < .01), indicating that participants’ levels of trust in the information system increased with time. Specifically, trust increased by .335 each studied time point, beginning with an average score of 3.852. Results, therefore, provide support for hypothesis 1. Furthermore, results indicated that individuals initially trusted the system to varying extents (statistically significant factor variance estimate; b = 1.01, SE = .277 p < .001). Growth rates of trust however did not significantly vary between users (non-significant slope variance; b = .225, SE = .153, p = .140) and did not significantly correlate with initial trust levels (r = –.035, p = .782), indicating that the extent of initial trust did not affect trust growth.
Table 7. Univariate latent growth model parameter estimates.
To test hypotheses 2 to 7, we followed van der Werff and Buckleys’ (Citation2017) procedure and created four augmented latent growth models, and expanded our established baseline growth model by predictors and outcomes. This allowed us to estimate the structural relationships between trust antecedents and trust as well as trust and outcomes at the four different time points in the growth curve. For this purpose, we coded the zero point for time at the time point for which we wanted to examine the relationships between antecedents, trust, and outcomes in each of the four augmented models (following Biesanz et al. Citation2004). For instance, to assess the relationship between trust antecedents, trust, and trust outcomes at T1, we set the second and third factor loadings to 0 and 5, while allowing the first and last to be freely estimated. We then regressed the eight potential trust antecedents onto the model’s intercept to test which significantly predicted it. Finally, we regressed the intercept on the three outcomes (reliance, performance, and strain) to test whether they were significantly associated at the different time points.Footnote3 A figure of the conditional Amos model to model associations at T2 can be found in . Results of the analyses can be found in .
Table 8: Results from Growth Models Showing Relationships of Trust in Information Systems with Antecedents, and Outcomes at Each Time Point
Hypothesis 2 proposed trust disposition to be significantly related to initial trust. Indeed, results showed that trust disposition was significantly related to initial trust in the information system at T0 ( = .216, SE = .064, p < .01), supporting hypothesis 2. Further, we proposed that the strength of the relationship between trust disposition and trust decreases with time. Indeed, trust disposition and trust were unrelated at all later measurement points (T1:
= .061, SE = .040, p = .208; T2:
= .064, SE = .041, p = .164; T3:
= .023, SE = .043, p = .601). We further tested whether differences between coefficients at T0 and T1, T2 and T3 were significant using the following estimation (C = standardised coefficient of the association between trust disposition and trust at T0; D = standardised coefficient of the association between trust disposition and trust at T1, T2, or T3; SE = standard error):
Results revealed significant differences between the coefficients at T0 ( = .216, SE = .064, p < .01) and T1 (
= .061, SE = .040, p = .208), T2 (
= .064, SE = .041, p = .164) and T3 (
= .023, SE = .043, p = .601). Therefore, our results provide support for hypothesis 2a.
Furthermore, we predicted that the information system’s properties and extant context and service structures are associated with trust in the system after its launch (i.e. at T1 to T3; hypotheses 3 and 4). Results indicated that the different system properties significantly predicted trust at least at one of the three later measurement time points (reliability at T1 ( = .216, SE = .055, p < .05) and T2 (
= .304, SE = .060, p < .001); credibility at T2 (
= .184, SE = .062, p < .05) and T3 (
= .253, SE = , p < .01); usability at T1 (
= .640, SE = .051, p < .001), T2 (
= .384, SE = .060, p < .001), and T3 (
= .388, SE = .062, p < .001)), except for design aesthetics, which were unrelated with trust at each time point. Since different system properties were positively related to trust at each time point after the system’s launch, our findings supported hypothesis 3. Hypothesis 3a further assumed the strengths of the relations between system properties and trust to increase with time. We found that the usability-trust association significantly decreased between T1 (
= .640, SE = .051, p < .001) and T2 (
= .384, SE = .060, p < .001) as well as between T1 and T3 (
= .388, SE = .046, p < .001). For reliability and credibility, we found no significant differences between coefficient. Thus, results do not provide support for hypothesis 3a.
For context and service structures, out of the different components, only the abilities of involved persons significantly predicted trust at T3 ( = .185, SE = .067, p < .01). Results, therefore, only partially provide support for hypothesis 4. Even though the association descriptively increased from T1 (
= .134, SE = .051, p = .096) to T3 (
= .185, SE = .067, p < .01), no significant differences in the strength of associations could be found, therefore providing no support for hypothesis 4a.
In hypotheses 5 to 7, we made assumptions on how trust is associated with outcomes. Specifically, we assumed that trust is positively associated with reliance on the system and performance, while being negatively associated with strain perceptions. We found trust to be significantly and positively related with reliance on the information system at T1 ( = .265, SE = .155, p < .01) and T2 (
= .195, SE = .162, p < .05), however it was unrelated at T3 (
= .083, SE = .199, p = .474). Results therefore only partially provided evidence for hypothesis 5. Our data provided support for hypotheses 6 and 7, since trust was consistently positively related with performance at T1 (
= .808, SE = .117, p < .001), T2 (
= .784, SE = .096, p < .001), and T3 (
= .810, SE = .092, p < .001) as well as negatively related with strain (T1:
= –.777, SE = .128, p < .001; T2:
= –.758, SE = .100, p < .001; T3:
= –.776, SE = .092, p < .001).
5. Discussion
In light of the growing number of information systems introduced to workplaces, the present study empirically investigated the dynamic nature of trust in a newly introduced information system. Thereby, we followed the theoretical assumption of trust being a dynamic construct (Crisp and Jarvenpaa Citation2013; Glikson and Woolley Citation2020; Hoff and Bashir Citation2015; Lee and See Citation2004; Schoorman, Mayer, and Davis Citation2007), which has been largely neglected within empirical research to date (Gefen, Benbasat, and Pavlou Citation2008; Hoehle, Huff, and Goode Citation2012). Thus, to strengthen the knowledge on trust dynamics, we investigated the relevance of different trust antecedents at different stages of system adoption. Finally, we examined the associations between trust in the information system and different trust outcomes. Our findings provide a nuanced understanding of how trust develops, spanning initial to long-term trust, and how trust is related to different antecedents and outcomes at different time points.
We tested our hypotheses by following the adoption process of a novel information system in an organisation across 11 months and four measurement points. Extant research shed much light on the degree of trust at given time points (Hoehle, Huff, and Goode Citation2012). However, understanding change in a variable, requires its repeated measurement across more than two time points (Ployhart and Vandenberg Citation2010; see also van der Werff and Buckley Citation2017). Our study is one of the first to investigate trust development in information systems at work using more than two (i.e. four) waves of data collection.
We hypothesised trust to increase with time. Our findings indeed indicated upward, non-linear trust trajectories, supporting our first hypothesis. More specifically, we found that trust developed in faster rates at the beginning as compared to later stages of system usage. This finding is in line with theoretical approaches on the development of trust in general (Lewicki, Tomlinson, and Gillespie Citation2006) as well as trust in information systems more specifically (Gefen, Benbasat, and Pavlou Citation2008; Söllner and Pavlou Citation2016). Following the differentiation between calculus- and knowledge-based trust (McKnight, Cummings, and Chervany Citation1998, Citation2011), early trust relationships might be perceived as rather calculus-based and derived from cognitive cues (Li, Hess, and Valacich Citation2008). These can be formed more quickly than knowledge-based judgments, which become more relevant as trusting relationships mature. Our findings on initial trust further indicate that trust in information systems does not start at zero. Since our first measurement time point occurred before the system’s launch, participants already trusted the system to some extent, even before having usage experiences. This is in line with Söllner and Pavlou’s (Citation2016) assumption that a phase of initial trust building precedes the first interaction with an information system. Initial trust development was followed by a rather stable phase of trust development between measurements two and three. This, again, corresponds with Rousseau et al.’s (Citation1998) phase of trust stability. Building on findings of interpersonal trust, one might transfer the concepts of the encounter and adjustment phase in human interactions (Chen and Klimoski Citation2003) to human-information system relations. The encounter phase, likewise, reflects a phase where users interact heavily with the system to learn its features and become accustomed to using it. Within the adjustment phase, the user has adjusted to the system’s usage and its integration into the workflow. Interestingly, a phase of faster trust growth followed this phase of stability between measures three and four (between five and ten months of system usage), contingent to the provision of additional training events between these two measurement points. These training events provided further knowledge on the system, which in turn might have increased users’ knowledge-based trust in the system (McKnight, Cummings, and Chervany Citation1998). However, given the lack of a control condition without such additional training in our research design, this interpretation has to be validated in future research.
Besides the development of trust itself, our study also investigated the relevance of different trust antecedents at different time points of system usage. Our findings are summarised in .
Table 9. Relevance of different trust antecedents for trust in the information system at different time points.
Our second hypothesis related to the association of a trustors’ disposition to trust technology, a stable person factor, with trust in the information system at the different measurement times. We found that disposition to trust technology was significantly related to initial trust (i.e. at T0), which supported our hypothesis. Our finding is in line with the assumption that the disposition to generally trust technology is especially relevant in initial stages of a relationship, when actual usage experiences are absent (Li, Hess, and Valacich Citation2008; Mayer, Davis, and Schoorman Citation1995; McKnight, Cummings, and Chervany Citation1998). Since trusting tendencies between individuals differ due to different factors (e.g. age, gender, culture; Hoff and Bashir Citation2015), this might have determined our finding that individuals initially trusted the system to varying extents. We further found that the disposition to trust technology was unrelated to trust at all measurement times after system launch, again supporting our hypothesis. This finding is consistent with Hoff and Bashir's (Citation2015) differentiations between factors influencing trust prior to interacting with a technology (i.e. dispositions) and during the interaction (i.e. system performance).
Further, our research also answers questions on the relevance of different trustworthiness cues on trust at different time-points in system adoption. This longitudinal examination of trust cues has already been subject to research on interpersonal trust (e.g. van der Werff and Buckley Citation2017), but it has remained relatively unstudied for trust relations between humans and information systems. While we assumed that trust in the information system after its launch (i.e. T1 to T3) would be associated with both the system’s properties as well as context and service structures, our findings mainly provide support for the former (hypothesis 3). Across all measurements, at least two of the four different properties of the information system (i.e. reliability, credibility, usability, design aesthetics) were positively related with users’ trust. The relevance of features as identified from qualitative studies (Thielsch, Meeßen, and Hertel Citation2018) and partly confirmed within cross-sectional, experimental settings (i.e. reliability and credibility; Meeßen et al. Citation2020) were, thus, validated in a longitudinal field setting as well. We further found differences in the significance of relationships between the respective system features and trust.
The information system’s usability was related to trust at all three time points during system usage, which underlines its relevance for trust building (e.g. Acemyan and Kortum Citation2012; Lippert and Swiercz Citation2005). Further, the strength of the association between usability and trust decreased across time, indicating usability to be especially relevant for early trust building. In order to explain this finding, it is helpful to consider the definition of usability, which describes it as users’ satisfaction with the experience of interacting with a system (Sasse Citation2005). Such assessments are formed by users within a short amount of time during initial interactions with a system (Lindgaard et al. Citation2011), providing a basis for early trust evaluation. It might also be that with more frequent system usage, users become more familiar with the systems’ pitfalls and discover ways to bypass those, so that usability tends to become less important.
Reliability was also significantly related with trust at earlier time points (T1 and T2) but not in the long-term (T3), while credibility was unrelated with trust at T1, but significantly related at the later time points (T2 and T3). Again, the duration users need to assess these system features can be used as a potential explanation for our findings. System malfunctions in the likes of long loading-times or errors (DeLone and McLean Citation2003; Hoff and Bashir Citation2015; McKnight et al. Citation2011) can be grasped by users within superficial interactions. On the other hand, the assessment of a system’s credibility (i.e. the believability of its output Fogg and Tseng Citation1999; Prat and Madnick Citation2008) might require experiences with the system within different usage scenarios, such that its influence on trust only becomes significant after sufficient interactions. Our finding of credibility being unrelated with trust at T1 might also come from the type of information system we investigated. In our study, participants interacted with an administrative system for invoice processing. Compared to decision support systems or management support systems, for instance, the quality of information provided by the system might be a less relevant influential factor for early trust building (O’Brien and Marakas Citation2010). This might explain findings from other studies that indeed indicated significant associations between a system’s credibility and trust in early usage stages (e.g. Meeßen et al. Citation2020; Shin, Lee, and Hwang Citation2017).
Finally, we found no significant association between the system’s design aesthetics and trust at any of the time points. This is interesting, since its role for system trust has been stressed, especially in the context of Web applications and e-commerce (e.g. Cyr Citation2008; Seckler, Opwis, and Tuch Citation2015). However, design aesthetics were found to be particularly relevant if users had a choice on whether or not to use a system (Beldad, de Jong, and Steehouder Citation2010; Kim et al. Citation2016). In our study, using the information system for invoice processing was mandatory. In such scenarios, a system’s functioning in terms of usability, reliability, and credibility seems to be more relevant than an appealing design aesthetic.
Organisational context and service structures were unrelated to trust at each time point, except for the perceived abilities of involved persons, which were significantly associated with trust ten months after the system’s launch. Even though earlier research found context and service structures to be relevant antecedents of system trust (Thielsch, Meeßen, and Hertel Citation2018), system features have indeed been found to be the strongest predictors of trust. While being significantly related with trust in single regressions, support, participation and abilities of involved persons were unrelated with trust when being regressed on trust simultaneously with reliability and credibility (Thielsch, Meeßen, and Hertel Citation2018). We, therefore, exploratorily calculated our conditional growth models only with support, participation, and abilities of involved persons and, indeed, found that both participation and abilities of involved persons were significantly related with trust at all three time points.
Taken together, our findings underline the relevance of system features for trust building in early to mid-term trusting relations. Simultaneously, they provide hints that after a time of familiarisation with the system and its features, the broader context also becomes a relevant trust cue. Our study, thereby, provides longitudinal evidence that supports theoretical assumptions that as trust changes with time, so do its antecedents (Fulmer and Dirks Citation2018; Hoff and Bashir Citation2015).
Finally, we also assessed the relationship of trust with different outcomes. While our findings confirmed the strong relations of trust in an information system with performance and well-being (Hertel et al. Citation2019; Lee and See Citation2004; Meeßen et al. Citation2020; Thielsch, Meeßen, and Hertel Citation2018), they also provide initial evidence for a positive relation of trust with reliance in the field. Specifically, we found that trust was positively associated with users’ forgetting of irrelevant work processes taken on by the system. This extends initial research that found trust to trigger directed forgetting of information processed by the system (Hertel et al. Citation2019). We, however, did not find the association between trust and reliance at T3. It may be that after ten months of system usage, the forgetting of copying and printing invoices might not come from trust in the system, but from users’ habituation to novel work routines.
5.1. Theoretical implications
Our findings confirm theoretical assumptions that trust is a dynamic construct (e.g. Glikson and Woolley Citation2020; Hoff and Bashir Citation2015; Hu et al. Citation2019), also in the context of human-information system interaction. Moreover, they illustrate a specific trajectory that is somewhat inconsistent with earlier theoretical assumptions. While extant theoretical models on trust development (Rousseau et al. Citation1998; Söllner and Pavlou Citation2016) assumed a rather linear progression from trust building to trust stability, our findings indicate that a plateau of stability can also be followed by another phase of trust building. Theories on trust in information systems might therefore also consider transitions between trust building and trust stability (e.g. trust builds, remains stable for some time, then builds again, etc.). Such a stepwise development might have different implications for the theoretical conceptualisation of trust in information systems, and it might also hint toward potential external influences (e.g. information or training interventions) that can accelerate trust development again after a plateau phase. In our study, the trainings after six months of system usage probably contributed to the further increase of trust in the information system. Other factors might include prior experiences with other information systems or social influences from colleagues or superiors (Homburg, Wieseke, and Kuehnl Citation2010; Shi and Chow Citation2015). Including such external influences as moderators of the relationship between system experience and trust would further sharpen our knowledge on the ideal conditions for introducing an information system. Finally, it would be also interesting to explore whether negative trust developments follow similar stepwise processes (e.g. Lewicki and Brinsfield Citation2017, for interpersonal trust).
Our findings also have theoretical implications on how information system knowledge moderates the associations of different trust predictors with trust. We derived our assumptions on the interplay between time and the relevance of trust predictors using the theoretical model by Meeßen et al. (Citation2020). While we expected that with time and experience the relevance of a system’s trustworthiness for user trust would generally strengthen, our findings indicate dynamics on the level of single trust predictors, calling for more specific theoretical models.
5.2. Practical implications
Several implications for the introduction of information systems at work can be drawn from our research. First, given that we found trust in the information system to be related with several positive work outcomes, our findings underline the relevance of efficient trust management (Chasin, Riehle, and Rosemann Citation2019). Organisations might therefore want to make trust development a key goal for information system introduction processes. Including trust building goals into the general project plan of introducing a system might help to maintain the awareness for trust building throughout the different phases of the process. Moreover, evaluating trust during the introduction process should be helpful to detect signals of negative trust developments. More precisely, multiple evaluations at different time points may not only contribute to an increased sensitivity of the project team but may also elevate trust-building efforts by providing feedback from users, which can be used to adjust the introduction process. This is in line with current project management techniques that promote agile working methods over historic styles of pre-planning the entire introduction procedure. It also aligns with our findings of trust being a dynamic construct, which does not form once and then remains constant, but changes over time. Trust evaluations at different time points can also be used to derive specific interventions in order to constantly increase trust. Importantly, such evaluations are relevant both before and after the system’s launch. Before system launch, organisations might want to use evaluations to uncover user demands that include the system (i.e. important functions) and the introduction process (i.e. trainings, participation). However, such demands are rather hypothetical, since future users can only imagine their usage of the system. Actual work and usage realities might reveal further demands that have not been considered before. Therefore, evaluations after system launch are also recommended. Constant communication about the current project status from the project team toward (future) users might further benefit the overall change compliance. In summary, we call for a trust- and user-oriented software introduction, resulting in adjustments of technical aspects and contextual usage conditions by the project team if necessary. In this regard, our findings emphasise the relevance of system features for users to develop and maintain trust in the system. Usability played a significant role for trust development particularly during initial interactions, and might therefore be ensured by a balance between a system having enough options available to perform tasks while simultaneously having a straightforward structure making it easy to use. Relying on usability experts when developing or choosing a system is one way to ensure good usability. Piloting a system is another opportunity, also to potentially apply modifications. Furthermore, organisations might want to make sure that an information system works reliably. Potential malfunctions and errors can therefore be detected and addressed before launch, for instance, by providing a test phase of system usage. Further, it is vital that the information provided by the system is credible. Immaculate data quality should, therefore, be aspired both before system launch and in the long run, for instance, by regular checkups on data quality.
In line with extant recommendations (e.g. Thielsch, Meeßen, and Hertel Citation2018), our results further support that organisations should ensure that persons who are responsible for or otherwise involved in the introduction of the information system are highly qualified. This can be achieved by hiring competent and conscientious employees. Further though, the remaining employees need to be informed who the responsible persons are, what they are tasked with, how they are trained, and how they work. Thereby, users of the information system may not only build trust in the system itself but also in the persons involved. Finally, our research provides first hints toward the relevance of holding training events on how to use the system, also after system launch. System introductions should, therefore, be accompanied by a sophisticated training concept that regularly refreshes and further increases users’ knowledge of the system.
5.3. Limitation and future research directions
Findings from our study need to be considered in light of some limitations. First, our study was situated within a single organisation, and thus a particular context (Davison and Martinsons Citation2016). Specifically, our study was placed in the central and decentralised divisions of a German university performing administrative tasks. University administrations are considered to have slower transformation rates than private enterprises (Scheer Citation2015) as well as having rather risk- and technology-averse employees (e.g. Buurman et al. Citation2012; Carlsson, Daruvala, and Jaldell Citation2012). Such differences to private enterprises might lead to different findings (i.e. faster growth rates of trust) in less conservative contexts. However, the high levels of both participants’ average technology competence and disposition to trust technology, do not confirm the assumption that our study took place in a conservative, technology-averse context. Nevertheless, in order to generalise our findings, research from other contexts, especially different organisational but also cultural settings, is required.
Second, by accompanying the adoption of an information system for invoice processing, we investigated a specific type of information system. The usage of a system that provides support for business operations as compared to, for instance, a decision support system or a management information system, might be associated with fewer risks. Decision support systems or management information systems not only help users with the execution of their daily tasks, but they also provide decision-relevant information and analyses (O’Brien and Marakas Citation2010). This might increase user resistance and, consequently, influence the speed and change the pattern of trust development. However, by using an invoice processing system, large amounts of money can be disposed of, the release of which should also not be underestimated as a responsible task. Still, research investigating the adoption to other types of information systems is required in order to generalise our findings.
Third, we found another increase of trust between our last two measurement points which might be associated with the provision of additional training events. Since our research design did not include a control condition that did not receive such trainings, a causal relation between the provision of training events and trust could not be confirmed. Future research implementing control group designs would, therefore, be important for further investigating training effects on system adoption.
Fourth, and as previously reported, our data set included missing data. Even though we used methods accounting for the missingness, studies with full data sets are more reliable, since our calculations included estimations. However, participant attrition is a common issue in longitudinal field research (Barry Citation2005), especially when participation is unincentivised. Within our study, we communicated to users why it was relevant to participate at each time point, but we also allowed participants to start the study at any of the four time points. This was mainly for practical reasons, in order to reach as many users of the system as possible, such as those who joined the organisation after one of the previous measurement points; however, this led our data set to include missingness. Within further research, in order to ensure complete data sets, one might only allow participants to participate the study when also having participated in each of the previous measurement points and additionally offer incentives for complete participations.
On a related note, it should be mentioned that our results are based on self-reports. Although objective data descriptively show that processing times of invoices have been clearly reduced by the new system on an organisational level, the validation of trust effects based on objective performance data on the individual level would be desirable. Future studies might thus want to export objective performance measures (i.e. processing times; quantity of processed invoices; indicators of mistakes) from the system on an individual level and investigate their associations with users’ trust in the system.
Our final limitation is related to our study’s duration and distribution of our measurement points. Even though our design allowed us to investigate initial, short-, mid-, and long-term trust, more measurement points would have created an even more detailed picture of trust development. Additionally, a more in-depth investigation of the development of trust between, for instance, one and five months of system usage would further enhance our knowledge on trust dynamics in the short-term to mid-term. Surveys after several years of system usage would further answer questions about the stability of trust, trust antecedents and trust outcomes beyond initial familiarisation.
Acknowledgements
We thank all employees that gave us valuable insight into their work with the new information system. Furthermore, we thank Dr. Miriam Höddinghaus for her support during data collection and preparation and Dr. Celeste Brennecka for her support with proofreading the manuscript.
Disclosure statement
No potential conflict of interest was reported by the author(s).
Data availability statement
Anonymised data are available upon request from the corresponding author.
Additional information
Funding
Notes
1 An approval by the ethics committee of the Faculty of Psychology & Sports Science of the University of Münster has been gathered for the research project ‘Getrost Vergessen’ under approval number 2019-03-GH-FA, which this study has been part of.
2 Questionnaires at T0 to T3 included further scales and questions which are unrelated to our hypotheses and therefore not presented at this point. These were requested by the organization in order to further evaluate the information systems’ introduction process.
3 For T0, we only regressed trust disposition on trust, since system properties, context and service structures and outcomes were not assessed, as the system was not used at that time point.
References
- Acemyan, C. Z., and P. Kortum. 2012. “The Relationship Between Trust and Usability in Systems.” Proceedings of the Human Factors and Ergonomics Society, 1842–1846. doi:10.1177/1071181312561371.
- Ashraf, M., J. Ahmad, W. Sharif, A. A. Raza, M. Salman Shabbir, M. Abbas, and R. Thurasamy. 2020. “The Role of Continuous Trust in Usage of Online Product Recommendations.” Online Information Review 44 (4): 745–766. doi:10.1108/OIR-05-2018-0156.
- Audrey Korsgaard, M. 2018. “Reciprocal Trust. A Self-Reinforcing Dynamic Process.” In The Routledge Companion to Trust. 1st ed., edited by R. H. Searle, A.-M. I. Nienaber, and S. B. Sitkin, 14–18. London: Routledge.
- Audrey Korsgaard, M., J. Kautz, P. Bliese, K. Samson, and P. Kostyszyn. 2018. “Conceptualising Time as a Level of Analysis: New Directions in the Analysis of Trust Dynamics.” Journal of Trust Research 8 (2): 142–165. doi:10.1080/21515581.2018.1516557.
- Bahmanziari, T., M. Pearson, and L. Crosby. 2003. “Is Trust Important in Technology Adoption? A Policy Capturing Approach.” Journal of Computer Information Systems 43 (4): 46–54. doi:10.1080/08874417.2003.11647533.
- Bakker, A. B., and E. Demerouti. 2007. “The job Demands-Resources Model: State of the Art.” Journal of Managerial Psychology 22 (3): 309–328. doi:10.1108/02683940710733115.
- Baroudi, J. J., and W. J. Orlikowski. 1988. “A Short-Form Measure of User Information Satisfaction: A Psychometric Evaluation and Notes on Use.” Journal of Management Information Systems 4 (4): 44–59. doi:10.1080/07421222.1988.11517807.
- Barry, A. E. 2005. “How Attrition Impacts the Internal and External Validity of Longitudinal Research.” Journal of School Health 75 (7): 267–270. doi:10.1111/j.1746-1561.2005.00035.x.
- Beldad, A., M. de Jong, and M. Steehouder. 2010. “How Shall I Trust the Faceless and the Intangible? A Literature Review on the Antecedents of Online Trust.” Computers in Human Behavior 26 (5): 857–869. doi:10.1016/j.chb.2010.03.013.
- Bentler, P. M. 1990. “Comparative fit Indexes in Structural Models.” Psychological Bulletin 107 (2): 238–246. doi:10.1037/0033-2909.107.2.238.
- Bentler, P. M., and D. G. Bonett. 1980. “Significance Tests and Goodness of fit in the Analysis of Covariance Structures.” Psychological Bulletin 88 (3): 588–606. doi:10.1037/0033-2909.88.3.588.
- Biesanz, J. C., N. Deeb-Sossa, A. A. Papadakis, K. A. Bollen, and P. J. Curran. 2004. “The Role of Coding Time in Estimating and Interpreting Growth Curve Models.” Psychological Methods 9 (1): 30–52. doi:10.1037/1082-989X.9.1.30.
- Bijlsma, K., and P. Koopman. 2003. “Introduction: Trust Within Organisations.” Personnel Review 32 (5): 543–555. doi:10.1108/00483480310488324.
- Bollen, K. A. 1989. “A New Incremental fit Index for General Structural Equation Models.” Sociological Methods & Research 17 (3): 303–316. doi:10.1177/0049124189017003004.
- Brauner, P., R. Philipsen, A. Calero Valdez, and M. Ziefle. 2019. “What Happens When Decision Support Systems Fail? The Importance of Usability on Performance in Erroneous Systems.” Behaviour & Information Technology 38 (12): 1225–1242. doi:10.1080/0144929X.2019.1581258.
- Bravo, E. R., M. Santana, and J. Rodon. 2015. “Information Systems and Performance: The Role of Technology, the Task and the Individual.” Behaviour & Information Technology 34 (3): 247–260. doi:10.1080/0144929X.2014.934287.
- Bresnen, M., A. Goussevskaia, and J. Swan. 2005. “Organizational Routines, Situated Learning and Processes of Change in Project-Based Organizations.” Project Management Journal 36 (3): 27–41. doi:10.1177/875697280503600304.
- Buurman, M., J. Delfgaauw, R. Dur, and S. van den Bossche. 2012. “Public Sector Employees: Risk Averse and Altruistic?” Journal of Economic Behavior & Organization 83 (3): 279–291. doi:10.1016/j.jebo.2012.06.003.
- Cabiddu, F., L. Moi, G. Patriotta, and D. G. Allen. 2022. “Why Do Users Trust Algorithms? A Review and Conceptualization of Initial Trust and Trust Over Time.” European Management Journal. doi:10.1016/j.emj.2022.06.001.
- Campbell, R. H., and M. Grimshaw. 2016. “User Resistance to Information System Implementations: A Dual-Mode Processing Perspective.” Information Systems Management 33 (2): 179–195. doi:10.1080/10580530.2016.1155951.
- Carlsson, F., D. Daruvala, and H. Jaldell. 2012. “Do Administrators Have the Same Priorities for Risk Reductions as the General Public?” Journal of Risk and Uncertainty 45 (1): 79–95. doi:10.1007/s11166-012-9147-3.
- Cavanaugh, M. A., W. R. Boswell, M. Roehling, and J. W. Boudreau. 2000. “An Empirical Examination of Self-Reported Work Stress among U.S. Managers.” Journal of Applied Psychology 85 (1): 65–74. doi:10.1037/0021-9010.85.1.65.
- Chang, L. J., B. B. Doll, M. van ‘t Wout, M. J. Frank, and A. G. Sanfey. 2010. “Seeing is Believing: Trustworthiness as a Dynamic Belief.” Cognitive Psychology 61 (2): 87–105. doi:10.1016/j.cogpsych.2010.03.001.
- Chasin, F., D. M. Riehle, and M. Rosemann. 2019. “Trust Management – an Information Systems Perspective.” Proceedings of the 27th European Conference on Information Systems, 1–13, Stockholm, Sweden.
- Chen, H., R. H. L. Chiang, and V. C. Storey. 2012. “Business Intelligence and Analytics: From Big Data to Big Impact.” MIS Quarterly 36 (4): 1165–1188. doi:10.2307/41703503.
- Chen, G., and R. J. Klimoski. 2003. “The Impact of Expectations on Newcomer Performance in Teams as Mediated by Work Characteristics, Social Exchanges, and Empowerment.” Academy of Management Journal 46 (5): 591–607. doi:10.2307/30040651.
- Cheung, C. M. K., and M. K. O. Lee. 2006. “Understanding Consumer Trust in Internet Shopping: A Multidisciplinary Approach.” Journal of the American Society for Information Science and Technology 57 (4): 479–492. doi:10.1002/asi.20312.
- Cheung, G. W., and R. B. Rensvold. 2002. “Evaluating Goodness-of-fit Indexes for Testing Measurement Invariance.” Structural Equation Modeling: A Multidisciplinary Journal 9 (2): 233–255. doi:10.1207/S15328007SEM0902_5.
- Costante, E., J. den Hartog, and M. Petkovic. 2011. “Online Trust Perception: What Really Matters.” In Proceedings of the 1st Workshop on Socio-Technical Aspects in Security and Trust (STAST ‘11), 52–59. doi:10.1109/STAST.2011.6059256.
- Crisp, C. B., and S. L. Jarvenpaa. 2013. “Swift Trust in Global Virtual Teams: Trusting Beliefs and Normative Actions.” Journal of Personnel Psychology 12 (1): 45–56. doi:10.1027/1866-5888/a000075.
- Cyr, D. 2008. “Modeling web Site Design Across Cultures: Relationships to Trust, Satisfaction, and e-Loyalty.” Journal of Management Information Systems 24 (4): 47–72. doi:10.2753/MIS0742-1222240402.
- Davison, R. M., and M. G. Martinsons. 2016. “Context is King! Considering Particularism in Research Design and Reporting.” Journal of Information Technology 31 (3): 241–249. doi:10.1057/jit.2015.19.
- de Jonge, J., and C. Dormann. 2006. “Stressors, Resources, and Strain at Work: A Longitudinal Test of the Triple-Match Principle.” Journal of Applied Psychology 91 (6): 1359–1374. doi:10.1037/0021-9010.91.5.1359.
- DeLone, W. H., and E. R. McLean. 2003. “The DeLone and McLean Model of Information Systems Success: A Ten-Year Update.” Journal of Management Information Systems 19 (4): 9–30. doi:10.1080/07421222.2003.11045748.
- de Rijk, A. E., P. M. le Blanc, W. B. Schaufeli, and J. de Jonge. 1998. “Active Coping and Need for Control as Moderators of the Job Demand-Control Model: Effects on Burnout.” Journal of Occupational and Organizational Psychology 71 (1): 1–18. doi:10.1111/j.2044-8325.1998.tb00658.x.
- Dietz, G., and D. N. den Hartog. 2006. “Measuring Trust Inside Organisations.” Personnel Review 35 (5): 557–588. doi:10.1108/00483480610682299.
- Eisinga, R., M. te Grotenhuis, and B. Pelzer. 2013. “The Reliability of a Two-Item Scale: Pearson, Cronbach, or Spearman-Brown?” International Journal of Public Health 58 (4): 637–642. doi:10.1007/s00038-012-0416-3.
- Emad, G. R. 2010. “Introduction of Technology into Workplace and the Need for Change in Pedagogy.” Procedia Social and Behavioral Sciences 2 (2): 875–879. doi:10.1016/j.sbspro.2010.03.119.
- Enders, C. K., and D. L. Bandalos. 2001. “The Relative Performance of Full Information Maximum Likelihood Estimation for Missing Data in Structural Equation Models.” Structural Equation Modeling 8 (3): 430–457. doi:10.1207/S15328007SEM0803_5.
- Etezadi-Amoli, J., and A. F. Farhoomand. 1996. “A Structural Model of End User Computing Satisfaction and User Performance.” Information & Management 30 (2): 65–73. doi:10.1016/0378-7206(95)00052-6.
- Fogg, B. J., and H. Tseng. 1999. “The Elements of Computer Credibility.” In CHI ‘99: Proceedings of the SIGCHI Conference on Human Factors in Computing Systems, 80–87. doi:10.1145/302979.303001.
- Fornell, C., and D. F. Larcker. 1981. “Evaluating Structural Equation Models with Unobservable Variables and Measurement Error.” Journal of Marketing Research 18 (1): 39. doi:10.2307/3151312.
- Fulmer, C. A., and K. Dirks. 2018. “Multilevel Trust: A Theoretical and Practical Imperative.” Journal of Trust Research 8 (2): 137–141. doi:10.1080/21515581.2018.1531657.
- Galambos, N. L., E. T. Barker, and H. J. Krahn. 2006. “Depression, Self-Esteem, and Anger in Emerging Adulthood: Seven-Year Trajectories.” Developmental Psychology 42 (2): 350–365. doi:10.1037/0012-1649.42.2.350.
- Gefen, D. 2002. “Reflections on the Dimensions of Trust and Trustworthiness among Online Consumers.” ACM SIGMIS Database: The DATABASE for Advances in Information Systems 33 (3): 38–53. doi:10.1145/569905.569910.
- Gefen, D., I. Benbasat, and P. A. Pavlou. 2008. “A Research Agenda for Trust in Online Environments.” Journal of Management Information Systems 24 (4): 275–286. doi:10.2753/MIS0742-1222240411.
- Gefen, D., E. Karahanna, and D. W. Straub. 2003. “Trust and TAM in Online Shopping: An Integrated Model.” MIS Quarterly 27 (1): 51–90. doi:10.2307/30036519.
- Gill, H., K. Boies, J. E. Finegan, and J. McNally. 2005. “Antecedents of Trust: Establishing a Boundary Condition for the Relation Between Propensity to Trust and Intention to Trust.” Journal of Business and Psychology 19 (3): 287–302. doi:10.1007/s10869-004-2229-8.
- Glikson, E., and A. W. Woolley. 2020. “Human Trust in Artificial Intelligence: Review of Empirical Research.” Academy of Management Annals 14 (2): 627–660. doi:10.5465/annals.2018.0057.
- Govindan, K., and P. Mohapatra. 2012. “Trust Computations and Trust Dynamics in Mobile Adhoc Networks: A Survey.” IEEE Communications Surveys & Tutorials 14 (2): 279–298. doi:10.1109/SURV.2011.042711.00083.
- Hair, J. F., W. C. Black, B. J. Babin, and R. E. Anderson. 2010. Multivariate Data Analysis. 7th ed. New York: Pearson Prentice Hall.
- Hair, J. F., G. T. M. Hult, C. M. Ringle, and M. Sarstedt. 2014. A Primer on Partial Least Squares Structural Equation Modelling (PLS-SEM). Thousand Oaks, CA: SAGE Publications.
- Hertel, G., U. Konradt, and B. Orlikowski. 2004. “Managing Distance by Interdependence: Goal Setting, Task Interdependence, and Team-Based Rewards in Virtual Teams.” European Journal of Work and Organizational Psychology 13 (1): 1–28. doi:10.1080/13594320344000228.
- Hertel, G., S. M. Meeßen, D. M. Riehle, M. T. Thielsch, C. Nohe, and J. Becker. 2019. “Directed Forgetting in Organisations: The Positive Effects of Decision Support Systems on Mental Resources and Well-Being.” Ergonomics 62 (5): 597–611. doi:10.1080/00140139.2019.1574361.
- Hoehle, H., S. Huff, and S. Goode. 2012. “The Role of Continuous Trust in Information Systems Continuance.” Journal of Computer Information Systems 52 (4): 1–9. doi:10.1080/08874417.2012.11645571.
- Hoff, K. A., and M. Bashir. 2015. “Trust in Automation: Integrating Empirical Evidence on Factors That Influence Trust.” Human Factors 57 (3): 407–434. doi:10.1177/0018720814547570.
- Homburg, C., J. Wieseke, and C. Kuehnl. 2010. “Social Influence on Salespeople’s Adoption of Sales Technology: A Multilevel Analysis.” Journal of the Academy of Marketing Science 38 (2): 159–168. doi:10.1007/s11747-009-0157-x.
- Hu, W.-L., K. Akash, T. Reid, and N. Jain. 2019. “Computational Modeling of the Dynamics of Human Trust During Human–Machine Interactions.” IEEE Transactions on Human-Machine Systems 49 (6): 485–497. doi:10.1109/THMS.2018.2874188.
- Kappelman, L., V. Johnson, C. Maurer, E. McLean, R. Torres, A. David, and Q. Nguyen. 2018. “The 2017 SIM IT Issues and Trends Study.” MIS Quarterly Executive 17 (1): 53–88.
- Kassim, E. S., S. F. A. K. Jailani, H. Hairuddin, and N. H. Zamzuri. 2012. “Information System Acceptance and User Satisfaction: The Mediating Role of Trust.” Procedia – Social and Behavioral Sciences 57: 412–418. doi:10.1016/j.sbspro.2012.09.1205.
- Kim, N., B. Koo, J. Yoon, and K. Cho. 2016. “Understanding the Formation of User’s First Impression on an Interface Design from a Neurophysiological Perspective-EEG Pilot Study.” In HCIK '16: Proceedings of HCI Korea, 139–145. doi:10.17210/hcik.2016.01.139.
- Kim, H.-W., and S. L. Pan. 2006. “Towards a Process Model of Information Systems Implementation: The Case of Customer Relationship Management (CRM).” ACM SIGMIS Database 37 (1): 59–76. doi:10.1145/1120501.1120506.
- Körner, A., M. Geyer, M. Roth, M. Drapeau, G. Schmutzer, C. Albani, S. Schumann, and E. Brähler. 2008. “Persönlichkeitsdiagnostik mit dem NEO-Fünf-Faktoren-Inventar: Die 30-Item-Kurzversion (NEO-FFI-30).” PPmP: Psychotherapie Psychosomatik Medizinische Psychologie 58: 238–245. doi:10.1055/s-2007-986199.
- Korpelainen, E., and M. Kira. 2013. “Systems Approach for Analysing Problems in IT System Adoption at Work.” Behaviour & Information Technology 32 (3): 247–262. doi:10.1080/0144929X.2011.624638.
- Lance, C. E., R. J. Vandenberg, and R. M. Self. 2000. “Latent Growth Models of Individual Change: The Case of Newcomer Adjustment.” Organizational Behavior and Human Decision Processes 83 (1): 107–140. doi:10.1006/obhd.2000.2904.
- Lankton, N. K., D. H. McKnight, and J. Tripp. 2015. “Technology, Humanness, and Trust: Rethinking Trust in Technology.” Journal of the Association for Information Systems 16 (10): 880–918. doi:10.17705/1jais.00411.
- Lee, J. D., and J. Gao. 2005. “Trust, Information Technology, and Cooperation in Supply Chains.” Supply Chain Forum: International Journal 6 (2): 82–89. doi:10.1080/16258312.2005.11517150.
- Lee, J. D., and K. A. See. 2004. “Trust in Automation: Designing for Appropriate Reliance.” Human Factors 46 (1): 50–80. doi:10.1518/hfes.46.1.50.30392.
- Lewicki, R. J., and C. Brinsfield. 2017. “Trust Repair.” Annual Review of Organizational Psychology and Organizational Behavior 4: 287–313. doi:10.1146/annurev-orgpsych-032516-113147.
- Lewicki, R. J., E. C. Tomlinson, and N. Gillespie. 2006. “Models of Interpersonal Trust Development: Theoretical Approaches, Empirical Evidence, and Future Directions.” Journal of Management 32 (6): 991–1022. doi:10.1177/0149206306294405.
- Lewis, J. R., B. S. Utesch, and D. E. Maher. 2013. “UMUX-LITE: When There’s No Time for the SUS.” In CHI’13: Proceedings of the SIGHI Conference on Human Factors in Computing Systems, 2099–2102. doi:10.1145/2470654.2481287.
- Li, X., T. J. Hess, and J. S. Valacich. 2008. “Why Do We Trust New Technology? A Study of Initial Trust Formation with Organizational Information Systems.” The Journal of Strategic Information Systems 17 (1): 39–71. doi:10.1016/j.jsis.2008.01.001.
- Lin, J., B. Wang, N. Wang, and Y. Lu. 2014. “Understanding the Evolution of Consumer Trust in Mobile Commerce: A Longitudinal Study.” Information Technology and Management 15 (1): 37–49. doi:10.1007/s10799-013-0172-y.
- Lindgaard, G., C. Dudek, D. Sen, L. Sumegi, and P. Noonan. 2011. “An Exploration of Relations Between Visual Appeal, Trustworthiness and Perceived Usability of Homepages.” ACM Transactions on Computer-Human Interaction 18 (1). doi:10.1145/1959022.1959023.
- Lindkvist, L., M. Bengtsson, D. M. Svensson, and L. Wahlstedt. 2017. “Replacing Old Routines: How Ericsson Software Developers and Managers Learned to Become Agile.” Industrial and Corporate Change 26 (4): 571–591. doi:10.1093/icc/dtw038.
- Lippert, S. K. 2007. “Investigating Postadoption Utilization: An Examination into the Role of Interorganizational and Technology Trust.” IEEE Transactions on Engineering Management 54 (3): 468–483. doi:10.1109/TEM.2007.900792.
- Lippert, S. K., and M. Davis. 2006. “A Conceptual Model Integrating Trust into Planned Change Activities to Enhance Technology Adoption Behavior.” Journal of Information Science 32 (5): 434–448. doi:10.1177/0165551506066042.
- Lippert, S. K., and P. M. Swiercz. 2005. “Human Resource Information Systems (HRIS) and Technology Trust.” Journal of Information Science 31 (5): 340–353. doi:10.1177/0165551505055399.
- Little, R. J. A. 1988. “A Test of Missing Completely at Random for Multivariate Data with Missing Values.” Journal of the American Statistical Association 83 (404): 1198–1202. doi:10.1080/01621459.1988.10478722.
- Lu, Y., S. Yang, P. Y. K. Chau, and Y. Cao. 2011. “Dynamics Between the Trust Transfer Process and Intention to use Mobile Payment Services: A Cross-Environment Perspective.” Information & Management 48 (8): 393–403. doi:10.1016/j.im.2011.09.006.
- MacKenzie, S. B., and P. M. Podsakoff. 2012. “Common Method Bias in Marketing: Causes, Mechanisms, and Procedural Remedies.” Journal of Retailing 88 (4): 542–555. doi:10.1016/j.jretai.2012.08.001.
- Mayer, R. C., J. H. Davis, and F. D. Schoorman. 1995. “An Integrative Model of Organizational Trust.” Academy of Management Review 20 (3): 709–734. doi:10.5465/amr.1995.9508080335.
- Mazzola, J. J., and R. Disselhorst. 2019. “Should We be ‘Challenging’ Employees?: A Critical Review and Meta-Analysis of the Challenge-Hindrance Model of Stress.” Journal of Organizational Behavior 40 (8): 949–961. doi:10.1002/job.2412.
- McClelland, M. M., A. C. Acock, and F. J. Morrison. 2006. “The Impact of Kindergarten Learning-Related Skills on Academic Trajectories at the End of Elementary School.” Early Childhood Research Quarterly 21 (4): 471–490. doi:10.1016/j.ecresq.2006.09.003.
- McKnight, D. H. 2005. “Trust in Information Technology.” In The Blackwell Encyclopedia of Management, edited by G. B. Davis, 329–331. Malden, MA: Blackwell.
- McKnight, D. H., M. Carter, J. B. Thatcher, and P. F. Clay. 2011. “Trust in a Specific Technology: An Investigation of Its Components and Measures.” ACM Transactions on Management Information Systems 2 (2): 1–25. doi:10.1145/1985347.1985353.
- McKnight, D. H., V. Choudhury, and C. Kacmar. 2002. “The Impact of Initial Consumer Trust on Intentions to Transact with a Web Site: A Trust Building Model.” The Journal of Strategic Information Systems 11: 297–323. doi:10.1016/S0963-8687(02)00020-3.
- McKnight, D. H., L. L. Cummings, and N. L. Chervany. 1998. “Initial Trust Formation in New Organizational Relationships.” The Academy of Management Review 23 (3): 473–490. doi:10.5465/amr.1998.926622.
- Meeßen, S. M., M. T. Thielsch, and G. Hertel. 2020. “Trust in Management Information Systems (MIS): A Theoretical Model.” Zeitschrift Für Arbeits- Und Organisationspsychologie 64 (1): 6–16. doi:10.1026/0932-4089/a000306.
- Meeßen, S. M., M. T. Thielsch, D. M. Riehle, and G. Hertel. 2020. “Trust is Essential: Positive Effects of Information Systems on Users’ Memory Require Trust in the System.” Ergonomics 63 (7): 909–926. doi:10.1080/00140139.2020.1758797.
- Mortensen, C. R., and R. B. Cialdini. 2010. “Full-Cycle Social Psychology for Theory and Application.” Social and Personality Psychology Compass 4 (1): 53–63. doi:10.1111/j.1751-9004.2009.00239.x.
- Mou, J., D. H. Shin, and J. Cohen. 2017. “Understanding Trust and Perceived Usefulness in the Consumer Acceptance of an E-Service: A Longitudinal Investigation.” Behaviour & Information Technology 36 (2): 125–139. doi:10.1080/0144929X.2016.1203024.
- Muir, B. M. 1994. “Trust in Automation: Part I. Theoretical Issues in the Study of Trust and Human Intervention in Automated Systems.” Ergonomics 37 (11): 1905–1922. doi:10.1080/00140139408964957.
- Müller, L. S., S. M. Meeßen, M. T. Thielsch, C. Nohe, D. M. Riehle, and G. Hertel. 2020. “Do Not Disturb!: Trust in Decision Support Systems Improves Work Outcomes Under Certain Conditions.” In MuC’20: Proceedings of the Conference on Mensch Und Computer, 229–237. doi:10.1145/3404983.3405515.
- Neyer, F. J., J. Felber, and C. Gebhardt. 2012. “Entwicklung und Validierung einer Kurzskala zur Erfassung von Technikbereitschaft.” Diagnostica 58 (2): 87–99. doi:10.1026/0012-1924/a000067.
- O’Brien, J. A., and G. M. Marakas. 2010. Management Information Systems. 10th ed. New York: McGraw-Hill/Irwin.
- Pavlou, P. A., and D. Gefen. 2004. “Building Effective Online Marketplaces with Institution-Based Trust.” Information Systems Research 15 (1): 37–59. doi:10.1287/isre.1040.0015.
- Petter, S., W. DeLone, and E. McLean. 2008. “Measuring Information Systems Success: Models, Dimensions, Measures, and Interrelationships.” European Journal of Information Systems 17 (3): 236–263. doi:10.1057/ejis.2008.15.
- Ployhart, R. E., and R. J. Vandenberg. 2010. “Longitudinal Research: The Theory, Design, and Analysis of Change.” Journal of Management 36 (1): 94–120. doi:10.1177/0149206309352110.
- Prat, N., and S. Madnick. 2008. “Measuring Data Believability: A Provenance Approach.” In Proceedings of the 41st Annual Hawaii International Conference on System Sciences (HICSS 2008), 393. doi:10.1109/HICSS.2008.243.
- Putnick, D. L., and M. H. Bornstein. 2016. “Measurement Invariance Conventions and Reporting: The State of the Art and Future Directions for Psychological Research.” Developmental Review 41: 71–90. doi:10.1016/j.dr.2016.06.004.
- Rainer, R. K., and B. Prince. 2021. Introduction to Information Systems. Hoboken, NJ: John Wiley & Sons.
- Rousseau, D. M., S. B. Sitkin, R. S. Burt, and C. Camerer. 1998. “Not so Different After all: A Cross-Discipline View of Trust.” The Academy of Management Review 23 (3): 393–404. doi:10.5465/AMR.1998.926617.
- Sasse, M. A. 2005. “Usability and Trust in Information Systems.” In Trust and Crime in Information Societies, edited by R. Mansell and B. Collins, 319–348. Cheltenham: Edward Elgar.
- Scheer, A. W. 2015. Whitepaper - Hochschule 4.0. https://www.researchgate.net/publication/281116948_Whitepaper_-_Hochschule_40.
- Schoorman, F. D., R. C. Mayer, and J. H. Davis. 2007. “An Integrative Model of Organizational Trust: Past, Present, and Future.” The Academy of Management Review 32 (2): 344–354. doi:10.5465/amr.2007.24348410.
- Seckler, M., K. Opwis, and A. N. Tuch. 2015. “Linking Objective Design Factors with Subjective Aesthetics: An Experimental Study on How Structure and Color of Websites Affect the Facets of Users’ Visual Aesthetic Perception.” Computers in Human Behavior 49: 375–389. doi:10.1016/j.chb.2015.02.056.
- Sharma, S. K., and M. Sharma. 2019. “Examining the Role of Trust and Quality Dimensions in the Actual Usage of Mobile Banking Services: An Empirical Investigation.” International Journal of Information Management 44: 65–75. doi:10.1016/j.ijinfomgt.2018.09.013.
- Shi, S., and W. S. Chow. 2015. “Trust Development and Transfer in Social Commerce: Prior Experience as Moderator.” Industrial Management & Data Systems 115 (7): 1182–1203. doi:10.1108/IMDS-01-2015-0019.
- Shin, D. 2020. “How Do Users Interact with Algorithm Recommender Systems? The Interaction of Users, Algorithms, and Performance.” Computers in Human Behavior 109: 106344. doi:10.1016/j.chb.2020.106344.
- Shin, D. 2021. “The Effects of Explainability and Causability on Perception, Trust, and Acceptance: Implications for Explainable AI.” International Journal of Human Computer Studies 146. doi:10.1016/j.ijhcs.2020.102551.
- Shin, D. 2022. “Expanding the Role of Trust in the Experience of Algorithmic Journalism: User Sensemaking of Algorithmic Heuristics in Korean Users.” Journalism Practice 16 (6): 1168–1191. doi:10.1080/17512786.2020.1841018.
- Shin, D. H., S. Lee, and Y. Hwang. 2017. “How Do Credibility and Utility Play in the User Experience of Health Informatics Services?” Computers in Human Behavior 67: 292–302. doi:10.1016/j.chb.2016.11.007.
- Söllner, M., and P. A. Pavlou. 2016. “A Longitudinal Perspective on Trust in IT Artefacts.” In 24th European Conference on Information Systems (ECIS). Istanbul, Turkey.
- Stanton, J. M., W. K. Balzer, P. C. Smith, L. F. Parra, and G. Ironson. 2001. “A General Measure of Work Stress: The Stress in General Scale.” Educational and Psychological Measurement 61 (5): 866–888. doi:10.1177/00131640121971455.
- Steiger, J. H. 1990. “Structural Model Evaluation and Modification: An Interval Estimation Approach.” Multivariate Behavioral Research 25 (2): 173–180. doi:10.1207/s15327906mbr2502_4.
- Stone, R. W., D. J. Good, and L. Baker-Eveleth. 2007. “The Impact of Information Technology on Individual and Firm Marketing Performance.” Behaviour and Information Technology 26 (6): 465–482. doi:10.1080/01449290600571610.
- Streiner, D. L. 2003. “Starting at the Beginning: An Introduction to Coefficient Alpha and Internal Consistency.” Journal of Personality Assessment 80 (1): 99–103. doi:10.1207/S15327752JPA8001_18.
- Suh, B., and I. Han. 2002. “Effect of Trust on Customer Acceptance of Internet Banking.” Electronic Commerce Research and Applications 1 (3–4): 247–263. doi:10.1016/S1567-4223(02)00017-0.
- Tam, C., A. Loureiro, and T. Oliveira. 2019. “The Individual Performance Outcome Behind E-Commerce: Integrating Information Systems Success and Overall Trust.” Internet Research 30 (2): 439–462. doi:10.1108/INTR-06-2018-0262.
- Thatcher, J. B., D. H. McKnight, E. W. Baker, R. E. Arsal, and N. H. Roberts. 2011. “The Role of Trust in Postadoption IT Exploration: An Empirical Examination of Knowledge Management Systems.” IEEE Transactions on Engineering Management 58 (1): 56–70. doi:10.1109/TEM.2009.2028320.
- Thielsch, M. T., and G. Hirschfeld. 2019. “Facets of Website Content.” Human-Computer Interaction 34 (4): 279–327. doi:10.1080/07370024.2017.1421954.
- Thielsch, M. T., S. M. Meeßen, and G. Hertel. 2018. “Trust and Distrust in Information Systems at the Workplace.” PeerJ 2018: 9. doi:10.7717/peerj.5483.
- Turel, O., and D. Gefen. 2013. “The Dual Role of Trust in System Use.” Journal of Computer Information Systems 54 (1): 2–10. doi:10.1080/08874417.2013.11645666.
- Turetken, O., J. Ondracek, and W. Ijsselsteijn. 2019. “Influential Characteristics of Enterprise Information System User Interfaces.” Journal of Computer Information Systems 59 (3): 243–255. doi:10.1080/08874417.2017.1339367.
- Vandenberg, R. J., and C. E. Lance. 2000. “A Review and Synthesis of the Measurement Invariance Literature: Suggestions, Practices, and Recommendations for Organizational Research.” Organizational Research Methods 3 (1): 4–70. doi:10.1177/109442810031002.
- van der Heijden, H., T. Verhagen, and M. Creemers. 2003. “Understanding Online Purchase Intentions: Contributions from Technology and Trust Perspectives.” European Journal of Information Systems 12 (1): 41–48. doi:10.1057/palgrave.ejis.3000445.
- van der Werff, L., and F. Buckley. 2017. “Getting to Know You: A Longitudinal Examination of Trust Cues and Trust Development During Socialization.” Journal of Management 43 (3): 742–770. doi:10.1177/0149206314543475.
- Van Der Werff, L.,.A. Legood, F. Buckley, A. Weibel, and D. De Cremer. 2019. “Trust Motivation: The Self-Regulatory Processes Underlying Trust Decisions.” Organizational Psychology Review 9 (2-3): 99–123. doi:10.1177/2041386619873616.
- Venkatesh, V., and F. D. Davis. 2000. “Theoretical Extension of the Technology Acceptance Model: Four Longitudinal Field Studies.” Management Science 46 (2): 186–204. doi:10.1287/mnsc.46.2.186.11926.
- Venkatesh, V., J. Y. L. Thong, F. K. Y. Chan, P. J.-H. Hu, and S. A. Brown. 2011. “Extending the Two-Stage Information Systems Continuance Model: Incorporating UTAUT Predictors and the Role of Context.” Information Systems Journal 21 (6): 527–555. doi:10.1111/j.1365-2575.2011.00373.x.
- Wang, S. W., W. Ngamsiriudom, and C. H. Hsieh. 2015. “Trust Disposition, Trust Antecedents, Trust, and Behavioral Intention.” The Service Industries Journal 35 (10): 555–572. doi:10.1080/02642069.2015.1047827.
- Willett, J. B., and A. G. Sayer. 1994. “Using Covariance Structure Analysis to Detect Correlates and Predictors of Individual Change Over Time.” Psychological Bulletin 116 (2): 363–381. doi:10.1037/0033-2909.116.2.363.
- Wothke, W. 2000. “Longitudinal and Multi-Group Modeling with Missing Data.” In Modeling Longitudinal and Multilevel Data: Practical Issues, Applied Approaches and Specific Examples, edited by T. D. Little, K. U. Schnabel, and J. Baumert, 219–240. Mahwah, NJ: Lawrence Erlbaum Associates, Inc.
- Yoon, M., and R. E. Millsap. 2007. “Detecting Violations of Factorial Invariance Using Data-Based Specification Searches: A Monte Carlo Study.” Structural Equation Modeling: A Multidisciplinary Journal 14 (3): 435–463. doi:10.1080/10705510701301677.
- Yu, P. L., M. S. Balaji, and K. W. Khong. 2015. “Building Trust in Internet Banking: A Trustworthiness Perspective.” Industrial Management & Data Systems 115 (2): 235–252. doi:10.1108/IMDS-09-2014-0262.
- Zheng, Z., P. A. Pavlou, and B. Gu. 2014. “Latent Growth Modeling for Information Systems: Theoretical Extensions and Practical Applications.” Information Systems Research 25 (3): 547–568. doi:10.1287/isre.2014.0528.
Appendix
A1.1. Full item list
Trust Disposition (Lankton, McKnight, and Tripp Citation2015; McKnight, Choudhury, and Kacmar Citation2002; = .83)
I usually trust computer technology until it gives me a reason not to trust it.
I generally give computer technology the benefit of the doubt when I first use it.
My typical approach is to trust new computer technologies until they prove to me that I shouldn’t trust them.
Reliability (McKnight et al. Citation2011; = .92–.93)
The system is very reliable.
The system does not malfunction for me.
The system is extremely dependable.
Credibility (Thielsch & Hirschfeld; = .95–.97)
The information provided by the system is credible.
I can trust the information provided by the system.
The information provided by the system is reliable.
Usability (Lewis, Utesch, and Maher Citation2013; p = .68–.69)
The capabilities of the system meet my requirements.
The system is easy to use.
Design aesthetics (Thielsch, Meeßen, and Hertel Citation2018)
I find the system’s design appealing.
Support (Thielsch, Meeßen, and Hertel Citation2018; p = .62–.74)
If problems with the system occur, support is available.
The support is helpful.
Participation (Baroudi and Orlikowski Citation1988; p = .63–.70)
I am sufficiently provided with information about changes and decisions concerning the system.
I can make change requests and adjustments that concern the system.
Abilities of Involved Persons (Hertel, Konradt, and Orlikowski Citation2004; = .80–.96)
The qualification of persons involved is sufficient.
I trust in the professional competence of the people responsible for the system.
I think I can rely on the skills of persons involved
Trust (Thielsch and Hirschfeld Citation2019; = .92–.95)
I completely trust the system.
I heavily rely on the system.
I feel comfortable relying on the system.
Performance (Etezadi-Amoli and Farhoomand Citation1996; = .93–.95)
Using the system improves the quality of my work.
Using the system makes my job easier.
Using the system saves me time.
Using the system helps me fulfil the needs and requirements of my job.
Strain (Stanton et al. Citation2001; = .93)
I find working with the system demanding.
I find working with the system stressful.
I find working with the system onerous.
Reliance
Please indicate how often you print or copy invoices.
Technology Competence (Neyer, Felber, and Gebhardt Citation2012; = .86)
Dealing with modern technology, I am often afraid of failing.
For me, dealing with new computer technology is mostly a challenge.
I am afraid that I rather destroy technological innovations than that I use them properly.
I find it hard to deal with new technology – mostly I am just not able to do so.
Conscientiousness (Körner et al. Citation2008; = .83)
I try to be very conscientious in performing all the tasks assigned to me.
When I make a commitment, I can certainly be counted on.
I am a hardworking person who always gets the job done.
Need for Control (de Rijk et al. Citation1998; = .81)
I highly value being able to set the pace of my tasks.
I highly value having control over what I do and the way I do it.
I highly value doing my own planning.
Table A1. Intercorrelations between scale items of the first measurement point (T1).
Table A2. Intercorrelations between scale items of the second measurement point (T2).
Table A3. Intercorrelations between scale items of the third measurement point (T3).
Table A4. Construct validity measures of the scales for the first measurement point (T1).
Table A5. Construct validity measures of the scales for the second measurement point (T2).
Table A6. Construct validity measures of the scales for the third measurement point (T3).
Table A7. Discriminant validity of the scales for the first measurement point (T1).
Table A8. Discriminant validity of the scales for the second measurement point (T2).
Table A9. Discriminant validity of the scales for the third measurement point (T3).