ABSTRACT
Technology has the potential to enhance the effectiveness of the teaching and learning process. With the integration of technological resources, educators can create dynamic and interactive learning environments that offer diverse learning methods. With the help of these resources, students may be able to understand any topic deeply. Incorporating humanoid robots provides a valuable approach that combines the benefits of technology with the personal touch of human interaction. The role of speech is important in education; students might face challenges due to accent and auditory problems. The display of the text on the robot's screen can be beneficial for students to understand the speech better. In the present study, our objective is to integrate speech transcription with the humanoid robot Pepper and to explore its performance as an educational assistant in online and offline modes of teaching. The findings of this study suggest that Pepper's speech recognition system is a suitable candidate for both modes of teaching, regardless of the participant's gender. We expect that integrating humanoid robots into education may lead to more adaptive and efficient teaching and learning, resulting in improved learning outcomes and a richer educational experience.
1. Introduction
In past years, society has faced many challenges in education because of the widespread impact of the Covid-19 pandemic. The implementation of lockdown compelled us to shift from traditional offline to online teaching mode. With the help of technological advancements, the transition to online mode was feasible and acceptable (Rajeb et al. Citation2023; Trelease Citation2016). However, the online medium has issues such as limited personal interactions and the need for technology access (Joshi et al. Citation2022). Physical classrooms facilitate social interaction and real-time doubt clarification, whereas geographical distance might challenge some students. Thus, online and offline teaching have their advantages and disadvantages. Determining the superiority of any mode is difficult depending on many factors, including constraints and context.
Integrating advanced technologies such as social robots in physical and online teaching environments can enhance teaching methods and learning experiences. Past studies indicated the positive impact of including social robots in physical classrooms. The robots can engage the students and increase their participation during lectures. Few studies demonstrated the advantage of social robot inclusion for language learning (Alemi, Meghdari, and Ghazisaedy Citation2014; Kennedy et al. Citation2016; Randall Citation2019). Pandey, Subedi, and Mishra (Citation2022) showed that incorporating the humanoid robot NAO was helpful for the advancement of language and reading skills of primary students in physical classrooms. In the other study, NAO robot inclusion in higher education was demonstrated to teach numerical methods (Cruz-Ramírez, García-Martínez, and Olais-Govea Citation2022).
In the present study, social robot Pepper was employed to evaluate its usefulness in online and offline mode of teaching. SoftBank Robotics developed a Pepper robot which can interpret and interact with its surroundings using cameras, microphones and sensors. Pepper is equipped with four microphones on its head, and it can catch sounds from multiple directions. Pepper can establish communication with humans using its speech recognition system. However, the facility of speech-to-text conversion is not integrated into Pepper's functionality, which means it is unable to transcribe the audio into text even though it has a display screen to show the text.
Transcription of speech into text can validate the accuracy of the speech recognition system. Speech-to-text conversion is being utilised in various domains, including the education sector. Rustam et al. (Citation2014) comprehensive review shed light on the role and capabilities of speech-to-text conversion in education. Debnath et al. (Citation2023) demonstrated the integration of speech-to-text transformation with audio-visual methods to support teaching to individuals with disabilities. There are many speech-to-text conversion tools available. Our previous research suggested Whisper as a dependable and effective open-source tool (Pande, Rani A, and Mishra Citation2023) for speech-to-text conversion.
The understandability of a delivered lecture depends on the clarity of speech and, hence, the quality of the audio received. The accuracy of audio might vary depending on the selected mode – online or offline and therefore, it is important to evaluate the accuracy of captured audio. To our belief, there is no such study wherein the quality of the delivered lecture by the same teacher for online and offline mode has been assessed. The involvement of robots during the lecture could enhance the learning experience. The use of robots in education has made substantial progress, but to the best of our knowledge, the utilisation of speech recognition coupled with transcription display on the robot's screen is still unexplored. Most of the existing work (Belpaeme et al. Citation2018; Li Citation2015) using humanoid robots such as Nao and Pepper involves the physical presence of participants (teacher and student) in the educational setting. This implies that social robot’s speech recognition capabilities have not been investigated for online teaching mode. These challenges motivate us to frame the following research questions.
RQ1: Will the integration of Pepper with Whisper be an effective solution for online and offline modes of teaching?
RQ2: Is there any difference between male and female voices on the developed system for online and offline mode?
This paper is structured as follows. The next section describes the related works in this area. Methodology section includes data collection and the techniques employed. Further, data analysis and result section show the analysis of the result followed by discussions. Finally, the paper concludes with directions for future research.
2. Related works
To better understand the insights of speech recognition systems with the integration of social robots, it is important to explore the relevant studies in the field of education, including the role of social robots in various domains including education, mode of education – online and offline, impact of gender voice and importance of speech recognition.
2.1. The role of social robots in various domains
Social robots are popularly known for establishing communications with individuals. There are different domains where social robots are employed for communication purposes. One such field is healthcare, where social robots as a companions are utilised to provide emotional support. Abdollahi et al. (Citation2022) utilised the social robot Ryan to engage and provide companionship to older people with depression and dementia by establishing communication through dialogues with them. Furthermore, they integrated artificial emotional intelligence with the robot to make it empathic and establish more effective dialogue communication. The social robot can be employed to establish interactions for the therapy of autistic children (Cabibihan et al. Citation2013).
The service industry, such as hotel and travel, are utilising social robots (Vishwakarma et al. Citation2024). The social robot can be used in the hotel industry for interacting with customers (Nakanishi et al. Citation2020). Pan et al. (Citation2015) employed the Nao robot in the public space of a hotel for greeting purposes and to provide information about the hotel. Further, they analyzed the impact of robot’s speech on guests. Youssef et al. (Citation2021) suggested the application of a social robot as a receptionist with conversational capabilities. Niemelä et al. (Citation2019) explored the possibility of acceptance of social robot in shopping mall. Further, they suggested that there is a need for improved dialog capabilities in robot for better interaction with customers.
Social robots can be employed for entertainment purposes. Kory-Westlund and Breazeal (Citation2019) findings suggest that robot’s speech impacted children’s engagement and entertainment. Vogt et al. (Citation2019) utilised a social robot’s tablet to play educational games with children where the robot provided instructions and feedback.
2.2. Educational activities facilitated by social robots
Social robots are intelligently designed machines with the ability to interact with humans in a natural and convenient manner in different settings (Breazeal, Dautenhahn, and Kanda Citation2016). Belpaeme et al. (Citation2018) conducted a systematic review exploring the roles of social robots in education by addressing their influence on learning outcomes, the impact of their physical appearance and their roles within educational settings. Li (Citation2015) discussed the importance of physical presence of robot over telepresent robot and virtual agents. Furthermore, studies suggested that the physical presence of robots in educational settings has impact on learning enhancement (Kennedy, Baxter, and Belpaeme Citation2015) and cognitive learning (Leyzberg et al. Citation2012).
Lanzilotti et al. (Citation2021) utilised Pepper robot in primary school to evaluate its impact on students’ engagement and cognition through coding activity. Moreover, they found it enjoyable and engaging. Donnermann, Schaper, and Lugrin (Citation2022) demonstrated the benefits of integrating robot-assisted tutoring in higher education. Furthermore, they observed improvements in knowledge, motivation, and exam performance among students who received tutoring from robots, in contrast to those who had no exposure to a tutoring robot.
The social robots can be helpful in improving teamwork and communication among students through Robot-Supported Collaborative Learning (RSCL) activity. Shimada, Kanda, and Koizumi (Citation2012) utilised social robot in a group of grade 6th students for collaborative learning and found the enhancement in children’s motivation. Buchem (Citation2023) assessed the social learning experience and interaction quality in higher education level with the NAO robot and observed that RSCL game-based activity facilitated by robot was accepted by participants, regardless of their familiarity with the robot. Rosenberg-Kima, Koren, and Gordon (Citation2020) employed both instructor and robot facilitation, it was observed through qualitative measures that human facilitation was better in terms of various aspects, but robot facilitation helped improve time management and efficiency. However, quantitative data suggested no significant differences.
In recent years, social robots have emerged as a promising tool to assist in language learning. Lee and Lee (Citation2022) reviewed the efficacy of Robot-Assisted Language Learning (RALL) and observed that RALL had a positive impact on language learning compared to non-RALL conditions. In a different study, Alemi et al. (Citation2015a) performed an experiment with high school students and compared the RALL group with non-RALL group. Furthermore, they showed that RALL group displayed reduced anxiety and a more positive attitude towards learning English vocabulary, finding the process enjoyable and effective. In another study, Alemi et al. (Citation2015b) demonstrated that social robot NAO can be helpful for autistic children in learning a foreign language English.
Past studies demonstrated the effectiveness of social robot integration in educational settings. Such integration helps increase students’ engagement, cognition, learning and performance. This motivates us to include social robot for present study to provide students a comfortable environment where they can understand the lecture in a better way without any hesitation in asking.
2.3. Online and offline teaching
Previous studies have shown the mixed results of online and offline modes of teaching. Wiyono et al. (Citation2021) analysed the data collected from teachers of elementary school and their finding suggested that offline communication is more efficient than online communication with students. Similarly, Daulatabad et al. (Citation2022) showed that traditional offline classes were more effective than online classes. Zhu, Liu, and Hong (Citation2022) examined the perception of online education among young children and their parents, and their findings illustrated the negative impact of online teaching. Furthermore, parents preferred offline education, but this perceivance varied by location and school type. On the other hand, Yen et al. (Citation2018) findings indicated that undergraduate students performed equally well in three of teaching modalities that are face-to-face, online and blended. Veeraiyan et al. (Citation2022) compared online and offline teaching for undergraduate dental students and found equivalent performance in both the modes. In contrast, Pei and Wu (Citation2019) conducted a systematic review and suggested that online learning is more effective than offline learning in enhancing the knowledge and skills of undergraduate students. Rodríguez-Hidalgo and Sibrian (Citation2022) conducted a qualitative study using interviews to evaluate if social robot Sima can be used as a pedagogical tool in the online classrooms. In another study, Sinai and Rosenberg-Kima (Citation2022) utilised perception ratings of participants about social robot Nao inclusion as a motivator during online learning.
Past research revealed that the selection between online and offline mode is difficult because the impact of any of them varies based on scenarios. However, none of the studies highlighted the shortcomings of delivered lecture audio quality using social robots. In the present study, two experiments were conducted with each participant to understand the suitability of the mode for better capturing the audio by speech recognition system of the Pepper robot.
2.4. Impact of male and female voices
The effectiveness of understanding a lecture can be increased if the lecture is delivered with a clear and expressive voice. The modulation in voice is able to capture the attention of target audience successfully. Guldner et al. (Citation2024) examined how intentional voice alterations affect a listener's impression of a speaker. Koch (Citation2017) investigated the correlation between different teaching roles and various acoustic elements in teachers’ voices, considering a child-sensitive perspective. Yeshoda and Rajasudhakar (Citation2023) findings suggested that female teachers’ voice exhibited more variations than male teachers’ voice as measures related to fundamental frequency (F0) as well as short and long term frequency perturbations were higher for females.
There are reports which suggest that impact of male and female voices on Automatic Speech Recognition (ASR) systems. Adda-Decker and Lamel (Citation2005) illustrated that in the analysis of broadcast news and conversational telephone speech in both English and French, females exhibited higher accuracy compared to males in their voices. Similarly, Sawalha and Abu Shariah (Citation2013) showed that Arabic ASR system perceived the female utterances more precisely than male. In contrast, Tatman (Citation2017) findings suggested lower accuracy in the recognition of female speech in context of English dialect.
The above studies indicate that the selection between male and female voices is difficult for any mode of teaching. There is no conclusion about which voice is best for which medium. Considering this, we selected an equal number of participants from both genders.
2.5. Challenges with speech recognition
Accurate speech recognition is challenging for machines including social robots due to various factors (Mubin, Henderson, and Bartneck Citation2014). Speech variability is caused by several factors, such as accents, dialects, and speech disorders, which can greatly impact speech patterns and introduce variations in pronunciation. Furthermore, age is another contributing factor that can lead to differences in speech production (Bóna Citation2014; Das et al. Citation2013). Research studies found that social robot Nao faced problems in comprehending the voice of children (Kennedy et al. Citation2017; So and Lee Citation2023) and older adults (Keizer et al. Citation2019). Similarly, there are reports that demonstrated the challenges with speech recognition system of Pepper robot in understanding the utterences of children (Ekström and Pareto Citation2022) and elders (Carros et al. Citation2020).
Distance from the speaker device also poses significant issues for perfectly recognising the utterances. Previous studies have shown that speech accuracy is negatively impacted when a speaker moves away from the microphone (Nematollahi and Al-Haddad Citation2016; Rodrigues et al. Citation2019). Zahorik and Kelly (Citation2007) suggested the noticeable affect in vocal intensity with increasing distance. Noise present in surroundings also affects the problems for speech recognition systems (Attawibulkul, Kaewkamnerdpong, and Miyanaga Citation2017; Meyer, Dentel, and Meunier Citation2013). Furthermore, teacher's raised voice in response to noise can lead to voice problems (Nusseck et al. Citation2022). The speech recognition systems of social robots such as Nao and Pepper are also susceptible to distance and noise. Kennedy et al. (Citation2017) illustrated how increased distance from Nao robot affects speech recognition accuracy and noise. Similarly, Pande and Mishra (Citation2023) findings also suggested that perceivance of utterances by Pepper’s speech recognition system is optimal at maximum distance of 1-meter. Komatsubara et al. (Citation2014) also mentioned the problem in speech recognition due to noisy school environment during interaction with social robot Robovie.
2.6. Improvement in speech recognition
The enhancement in efficacy of speech recognition systems is important for better interactions between human and machines. Improvement in speech recognition systems in classroom settings can be achieved using Artificial Intelligence (AI) techniques. Previous studies indicated that AI play an important role in increasing speech recognition accuracy. Research showed that deep learning methods such as CNN (Abdel-Hamid et al. Citation2014), RNN (Graves, Mohamed, and Hinton Citation2013), DNN (Hinton et al. Citation2012) and transfer learning (Lin et al. Citation2021) are helpful in improving speech recognition precision. Rebai et al. (Citation2017) utilised Data Augmentation (DA) and Ensemble Method (EM) methods to improve speech recognition within a framework of DNN architecture. Mehra, Ranga, and Agarwal (Citation2024) proposed a late fusion technique that combines audio and image modalities to enhance speech command recognition. Mubin et al. (Citation2012) suggested that performance of speech recognition can be increased using Robot Interaction Language (ROILA) and their findings indicated that ROILA outperformed English. Pande and Mishra (Citation2023) incorporated speech-to-text conversion tool Whisper with Pepper robot to evaluate the speech recognition system performance through evaluation measures.
In previous studies many methods were provided for noise reduction to enhance the speech recognition accuracy. One of the popular methods used in various studies (Ayat, Manzuri-Shalmani, and Dianat Citation2006; Fu et al. Citation2023) is wavelet threshold. Sun et al. (Citation2022) utilised joint acoustic echo cancellation method along with adaptive filter and DNN model. Liao et al. (Citation2023) presented a method, ASR post-processing for readability (APR), to convert noisy ASR output into human readable text. Healy et al. (Citation2013) developed an algorithm to segregate noise from speech to enhance speech intelligibility for listeners with hearing impairments. Li et al. (Citation2023) demonstrated that speech recognition performance in noisy environment can be improved by incorporating lip reading skills and using the cross-modal fusion effect.
Research indicates that there are shortcomings with the speech recognition system of social robots, however, there are also solutions to improve that. In the present study, both the social robot and speech-to-text convertor Whisper were used to understand the statements spoken by participants accurately.
3. Methodology
The experiments were conducted in the Educational Technology Laboratory of NTNU Gjøvik, Norway. The laptop utilised in this experiment had an 11th Gen Intel(R) Core (TM) i5-1145G7 @ 2.60 GHz 1.50 GHz processor, 16 GB RAM, and was running Windows 10 operating system. The overall pipeline is shown in , which are in sync with the following subsections.
Figure 1. Overview of the working of the proposed system with humanoid robot in offline and online settings.
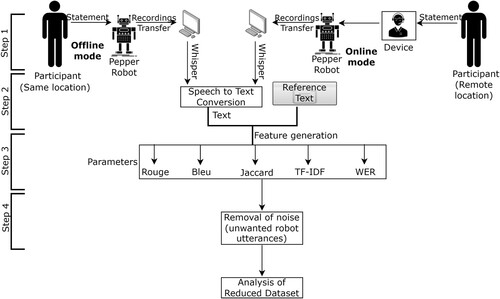
3.1. Step 1 – demographics and experimental procedures
The experiment was conducted in two modes: online and offline. A set of selected statements were provided to participants to speak. These statements were also stored in a local computer as reference text. Four adult volunteer participants were recruited for this experiment, including two males and two females. The participants belong to the age group between 30 and 40, and they all are of Asian descent. Furthermore, their native language is not English. All the participants have experience of teaching at some point in their professional career. As it is evident from past studies that robot has difficulty understanding children and older people’s voice, those age groups were not recruited for experiment. People in the 30–40 age group were recruited because, in our opinion, most of them have some experience in teaching in an academic setting. They spoke the statements, that were received by Pepper. In online mode, the participant was at a remote location and connected through the web. In offline mode, the participant and robot were placed next to each other.
The objective of the experiment is only to evaluate the Pepper’s speech recognition capability. This experiment will serve as a prototype before conducting the experiment in real educational setting.
3.2. Step 2 – recordings of audio-transferring of recordings and speech-to-text conversion
This step involved three operations – recording participants’ audio by the speech recognition system of Pepper, transferring recordings to the local system and converting recordings to text to evaluate the efficacy by comparing it with the reference text.
Pepper captured the participants’ voices in both modes using its service ALAudioRecorder (CitationNaoqi API documentation -ALAudioRecorder, http://doc.aldebaran.com/2-5/naoqi/audio/alaudiorecorder.html, last accessed 2023/05/07). ALAudioRecorder service provides a facility to record audio with the help of a robot's microphone, and the recording is stored in its system.
Transferring these recordings to the local system for further analysis is essential. Paramiko (CitationParamiko documentation, https://www.paramiko.org/, last accessed 2023/05/07) is a Python library that provides a way to transfer the files between Pepper and the local computer. Paramiko allows a secure connection with remote servers through SSH protocols.
Whisper (CitationOpenAI Whisper, https://openai.com/research/whisper, last accessed 2023/05/07) is a useful open-source speech recognition model and has applications in various domains. It is trained using extensive audio recordings. Whisper has many capabilities, such as multilingual speech recognition, translation, and language identification. After transferring audio recordings captured by Pepper to a computer system, these recordings can be employed as input for Whisper. This empowers the generation of corresponding text files from the recorded audio, facilitated by the utilisation of Whisper.
3.3. Step 3 – feature generation: by comparing whisper-produced text with original text
The extraction of meaningful insights from text data is vital to observe the efficiency of speech recognition system. Two text files were saved in the computer: Whisper-generated text file and reference text file. Original statements were kept in reference text. Different metrics were used to evaluate the accuracy of recorded speech. These metrics play an important role in measuring predicted text quality, similarity, and accuracy.
ROUGE Score: ROUGE (Recall-Oriented Understudy for Gisting Evaluation) score (Lin Citation2004) is a metric that is used to assess the text quality of machine-produced text when compared with original texts. The ROUGE score has many types, such as ROUGE-1 (unigram overlap), ROUGE-2 (bigram overlap), and ROUGE-L (longest common subsequence). A higher value of the ROUGE score signifies a higher similarity between produced text and reference text. The precision, recall and F-score can be calculated for each of the ROUGE scores.
BLEU Score: The BLEU (Bilingual Evaluation Understudy) score (Papineni et al. Citation2002) is used for evaluating the quality of machine-produced texts by comparing them with reference texts. The more excellent score indicates a high quality of translations.
TF-IDF Similarity Score: The TF-IDF (Term Frequency-Inverse Document Frequency) (Christian, Agus, and Suhartono Citation2016) score computes the similarity on the basis of frequency terms and their significance across a corpus. A more excellent TF-IDF similarity score implies a better similarity between two texts.
Jaccard Similarity Score: The Jaccard similarity score (Bag, Kumar, and Tiwari Citation2019) determines the similarity between two documents. It computes the number of words present in the intersection divided by the number of words present in the union of these sets. The Jaccard similarity score varies from 0 to 1, where 0 means no similarity and 1 means very similarity.
WER: The Word Error Rate (WER) (Klakow and Peters Citation2002) metric can be applied to estimate the precision of automatic speech recognition systems. The difference between the number of words in reference text and inaccurately identified words in the predicted text can be measured. A smaller WER value suggests good accuracy in transcribing from speech to text.
3.4. Step 4 – removal of the robot-produced unwanted utterances
Pepper has the capability to respond to humans through natural conversations. It could be possible that sometimes, a robot can get some cues from any statement and start its own comments, even if it is not explicitly programmed for that. In such situations, there might be more statements in Whisper-generated text than reference text. It is necessary to remove extra sentences from the text.
There were twenty-four sentences in reference text. Each sentence present in Whisper generated text was compared with each sentence of reference text by retaining maximum values of the scores except WER values. The highest value of scores indicates the much similarity between the texts, while the lowest value of WER values indicates higher accuracy. A threshold of 0.7 was put on the Rouge score so that best-matched sentences could be identified and robot-generated extra comments could be discarded.
4. Results
To answer the research questions, the experiment was conducted in two phases with each of the participants. First, the connection between Pepper and the computer system was established using the Paramiko library. The four audio files were transferred to the computer system for further analysis. These audio files were transcribed into text files using Whisper. Predicted texts were compared with reference texts to evaluate the speech recognition capability of Pepper in online and offline modes. The features were generated corresponding to both text files using different scores. Additional robot responses were cleaned out by applying thresholds to every statement. It was found that the TF-IDF score was relatively high in all the experiments in comparison to other scores, therefore it is not shown from for better visualisation.
illustrates the visualisation of six features for spoken statements by male participants through online mode. The selected values of features are placed in . It can be observed from the figure that the F-score of ROUGE-1 score is usually high for all text records. This implies that Pepper's speech recognition system with Whisper integration is efficient in acquiring individual words and unigrams from the spoken content. However, the variation of the ROUGE-2 score shows that in some instances, Pepper's speech recognition system can capture bi-grams magnificently. At the same time, in other cases, it struggles to capture bi-grams accurately. From the range of the ROUGE-L score, it can be inferred that the performance is good in catching the longest common subsequence based on whisper-generated text and reference text. BLEU scores highlight different scenarios. At times, Pepper's recorded audio perfectly matches the original speech, while there is no overlap between the two texts in some instances. However, in most instances, predicted and reference texts are moderately similar. The variation of Jaccard similarity scores signifies a moderate to high similarity between the words in the predicted and reference text. Varying range of WER values indicate instances of both perfect match and discrepancies between predicted text and original text.
Table 1. Features selected for male participants in the online mode of teaching.
demonstrates the analysis of features generated by male participant's speech in offline mode. The selected values of features are placed in . It can be observed that there are high values for most of the records for F-scores of ROUGE-1, ROUGE-2, and ROUGE-L. This suggests that Pepper's speech recognition system is capable of acquiring both separate words and longer sequences. Further obtained varying values of the BLEU score and Jaccard score confirm that for most of the instances there is a significant similarity between the Whisper-generated texts and reference texts. Moreover, the low WER values suggest an accurate prediction of speech. These values together indicate a strong and accurate speech recognition system that successfully captures speech in offline mode.
Table 2. Features selected for male participants in the offline mode of teaching.
emphasises the visualisation of six performance indicators generated by female participants’ voices in online mode. The selected values of features are placed in . F-scores of ROUGE-1 and ROUGE-L suggest a significant capability of speech recognition systems in accurately identifying words and longer sequences. The F-score of ROUGE-2 implies a moderate to convincing acquiring bi-grams within the generated text by Whisper. BLEU score reveals varying ranges of matching between the predicted and reference texts, with some cases a perfect match while in others, there are fewer similarities. Most of the time, Jaccard's score is high; it shows a significant similarity of words in predicted and reference texts. WER values are mostly low, indicating that the recorded speech by Pepper is strongly like the original.
Table 3. Features selected for female participants in the online mode of teaching.
exhibits the analysis evaluation metrics suggesting the accuracy of the speech recognition system of Pepper. The selected values of features are placed in . The values of F-scores of ROUGE-1 and ROUGE-L indicate the ability to obtain words and long sequences accurately. The F-score of ROUGE-2, which varies from moderate to high, implies that the speech recognition system's ability to identify bi-grams within the text also varies. The varying range of BLEU scores suggests both substantial and less similarity between the Whisper generated and original texts. For most of the instances, the Jaccard score is high, indicating a significant match of words. The inconsistent WER values demonstrate the divergence from the original speech.
Table 4. Features selected for female participants in the offline mode of teaching.
illustrates the average feature values of seven indicators for both genders in online and offline modes. The full table of average feature values is provided as supplementary data due to its size. The average F-score of ROUGE-1 indicates that Whisper-generated text from male and female online speeches are reasonably similar to the reference text. The same is true for the offline speech of a female. However, the F-score of ROUGE-1 corresponding to text of offline male speech signifies a strong matching with reference text. A similar kind of pattern is repeated for average F-scores of ROUGE-2 and ROUGE-L. The values of average BLEU scores suggest that only whisper-generated text for male offline speech matches significantly with the original text. However, male speech in online mode is moderately similar to reference text. The average value of the Jaccard Similarity score indicates that male speech in offline mode has a relatively higher degree of match with an original speech compared to others. At the same time, the male speech in online mode shows slight match with reference speech. However, the score of the online speech of male is relatively worse than the speech of female. The average values of WER suggest that whisper generated text and reference text match to each other quite well for both genders. The average value of TF-IDF similarity suggests that the speech recognition system is efficient in capturing male speech in offline mode. However, the values are acceptable for the offline mode of a female and an online mode of a male as well as a female. Furthermore, overall findings suggest that male voice is more suitable for offline mode than online mode, however for female voice, it is quite similar in all the cases. These results suggest that although there were differences in scores based on modes and gender but these variations are comparatively small.
5. Discussion
Several studies were conducted in the past that focused on the incorporation of technologies in the education system. Mavrikis et al. (Citation2014) demonstrated how speech recognition technology can aid problem-solving and reflective learning. Grassini (Citation2023) explored the potential of artificial intelligence and ChatGPT in the context of education. Some studies highlighted that including robots can affect the learning process (Chen et al. Citation2022; Engwall and Lopes Citation2022; Randall Citation2019). The present study provides essential insights into the humanoid robot Pepper as an educational assistant by assessing the speech recognition system for both online and offline modes. Further, it offers the opportunity to look over the capabilities and limitations of Pepper by integrating it with the speech-to-text conversion tool Whisper through the experiment results.
In relation to RQ1, the result shows that integration of Pepper and Whisper mostly achieves good scores for performance indicators while keeping low values of WER. Research findings show variability in the F-score values of ROUGE across different text records. These F-score values, namely ROUGE-1, ROUGE-2, and ROUGE-L, indicate how accurately the Pepper speech recognition system can capture individual words, bi-grams, and longer subsequences. When the F-score values are high, the speech recognition system performs well and accurately matches predicted and reference text (Topaz et al. Citation2022). This supports the idea that in integration with Whisper, Pepper can efficiently record and convert speech to text on both online and offline mode of teaching. The BLEU score measures the similarity between the predicted and reference text by comparing the overlap of n-grams. A high BLEU (Liu et al. Citation2003) score indicates accurate transcriptions of Pepper's recorded speech. This suggests that Pepper's speech recognition system successfully captures separate words and long sentences. The Jaccard Similarity scores indicate how much the generated text overlaps with the reference text regarding unique words. The consistently high (Pinto et al. Citation2012) scores suggest that Pepper's speech recognition system does an excellent job of picking up essential vocabulary from the spoken content. Even though there may be differences in how things are said, the overall system can extract crucial information from the reference text. The continuous high values of TF-IDF similarity suggest that the speech recognition system is sufficient to capture the audio. The Word Error Rate (WER) values, which show how much the predicted speech differs from the original, are usually less. This indicates the overall system is reasonably accurate at capturing and converting spoken language to text, with only minor differences between predicted and actual speech. Based on the feature values obtained in this study during the evaluation of speech recognition system, make Pepper a suitable candidate for online and offline both modes of teaching in a classroom like settings.
Previous studies also explored the integration of speech recognition systems in classroom settings for better teaching and learning experiences. Matre (Citation2022) conducted a qualitative study for inclusion of speech-to-text (STT) technology in classrooms for helping students to write with the help of their voice. Their findings encompassed teachers’ experiences about this inclusion, revealing that this technology helpful for longer assignments, however the study also addressed some challenges during its implementation such as issues of inaccuracy, inappropriate use, and instances of whispering among students. In another study Matre and Cameron (Citation2024) highlighted that STT is a useful way in enhancing the performance of writing of students with learning difficulties. Sun (Citation2023) demonstrated that the use of ASR enabled experimental group to perform better than control group in learning a second language.
Regarding RQ2, research findings suggest that male voices are better suited for offline modes than for online modes. However, for female voices, there is little difference between the two modes. It is worth noting that although there are differences in scores based on gender and mode, these variations are relatively small. Existing literature is inconclusive in this area. Some studies (Adda-Decker and Lamel Citation2005; Sawalha and Abu Shariah Citation2013) suggested that performance of speech recognition is better for female voices than male voices. However another study by Tatman (Citation2017) reported a contradictory result by showing lower accuracy in the speech recognition of female.
6. Conclusion, limitations and future work
A thorough analysis of Pepper's speech recognition system reveals its impressive ability to capture speech in both online and offline teaching environments accurately. With reference to RQ1, it has been observed that the overall system, including Pepper and Whisper, consistently achieves good scores on various performance indicators such as ROUGE F-score, BLEU, and Jaccard Similarity metrics, and TF-IDF similarity score while maintaining low WER values. In consideration to RQ2, for male and female voice in online and offline mode of teaching, the results of this study demonstrate minor differences in calculated scores. These findings demonstrate the overall system's potential to facilitate educational activities by capturing audio and converting speech-to-text with precision. These findings can contribute to the existing research on humanoid robots in education, highlighting their potential to serve as valuable educational assistants in different educational settings.
The proposed system with Pepper supports in understanding varying accents by transcribing spoken words into text and finally, displaying on Pepper’s display. However, there are a few challenges that may arise while utilising this system. The most prominent issue is related to Pepper's limited vocabulary, which can make it challenging for the system to comprehend complex words. Moreover, background noise can pose an obstacle, as it may inhibit Pepper's ability to perceive spoken utterances accurately. However, there are possibilities to overcome these issues. Challenges regarding noise can be addressed using the approach as described in this study related with unwanted utterances of Pepper. Pepper’s vocabulary can be enhanced, and background noise can be reduced by incorporating artificial intelligence techniques. Furthermore, the experiment conducted during the study was organised in a laboratory environment rather than in a real-world scenario. Therefore, we aim to improve Pepper's voice recognition capability by testing it in different environments along with including participants from diverse age groups in the future work. The inclusion of social robots as educational assistants has the potential to supplement the teaching and learning process.
The developed system with social robot and whisper has several implications. The presence of robot can enhance the students’ engagement and learning. We found that there is no major difference between the audio quality captured by robot, while teaching in offline and online mode. This will provide the teacher a flexibility to deliver lectures remotely as long as a robot as an educational assistant is present. If the teacher prefers to be present in-person, this will open up opportunities for guest lecturers to participate remotely. Furthermore, for teachers there will be no need to repeat their statements, and this will facilitate them to focus on delivering quality lecture. The students can have robot’s support at the time of their choice as they can listen to lecture recordings along with speech transcription.
In the future, additional features, such as the robot identifying when students have questions during a lecture, can be incorporated into the developed system by employing AI techniques. One such feature could be the ability to recognise raised hands, which would help in addressing student concerns. Furthermore, this system could assist in detecting the emotional state of students through facial expression, gesture and speech analysis. In case of any stress, social robot can provide them with emotional support and suggestions. The system could have the potential to provide solutions in response to particular query or suggestions to reduce their cognitive burden, ultimately enhancing the learning experience of students. All these additional functionalities will have the potential to make the proposed system more usable in classroom settings. The effectiveness of such a system could be tested by analyzing the collecting qualitative data such as questionnaires, surveys and interviews collected from both teachers and students.
Supplemental Material
Download MS Excel (19.6 KB)Disclosure statement
No potential conflict of interest was reported by the author(s).
References
- Abdel-Hamid, O., A. R. Mohamed, H. Jiang, L. Deng, G. Penn, and D. Yu. 2014. “Convolutional Neural Networks for Speech Recognition.” IEEE/ACM Transactions on Audio, Speech, and Language Processing 22 (10): 1533–1545. https://doi.org/10.1109/TASLP.2014.2339736.
- Abdollahi, H., M. Mahoor, R. Zandie, J. Sewierski, and S. Qualls. 2022. “Artificial Emotional Intelligence in Socially Assistive Robots for Older Adults: A Pilot Study.” IEEE Transactions on Affective Computing 14 (3): 2020–2032.
- Adda-Decker, M., and L. Lamel. 2005. “Do speech recognizers prefer female speakers?” In Ninth European Conference on Speech Communication and Technology.
- Alemi, M., A. Meghdari, N. M. Basiri, and A. Taheri. 2015b. “The effect of applying humanoid robots as teacher assistants to help Iranian autistic pupils learn English as a foreign language.” In Social Robotics: 7th International Conference, ICSR 2015, Paris, France, October 26-30, 2015, Proceedings 7.
- Alemi, M., A. Meghdari, and M. Ghazisaedy. 2014. “Employing Humanoid Robots for Teaching English Language in Iranian Junior High-Schools.” International Journal of Humanoid Robotics 11 (03): 1450022. https://doi.org/10.1142/S0219843614500224
- Alemi, M., A. Meghdari, and M. Ghazisaedy. 2015a. “The Impact of Social Robotics on L2 Learners’ Anxiety and Attitude in English Vocabulary Acquisition.” International Journal of Social Robotics 7 (4): 523–535. https://doi.org/10.1007/s12369-015-0286-y.
- Attawibulkul, S., B. Kaewkamnerdpong, and Y. Miyanaga. 2017. “Noisy Speech Training in MFCC-based Speech Recognition with Noise Suppression Toward Robot Assisted Autism therapy.” In 2017 10th Biomedical Engineering International Conference (Bmeicon). <Go to ISI>://WOS:000427614400038.
- Ayat, S., M. T. Manzuri-Shalmani, and R. Dianat. 2006. “An Improved Wavelet-Based Speech Enhancement by Using Speech Signal Features.” Computers & Electrical Engineering 32 (6): 411–425. https://doi.org/10.1016/j.compeleceng.2006.05.002.
- Bag, S., S. K. Kumar, and M. K. Tiwari. 2019. “An Efficient Recommendation Generation Using Relevant Jaccard Similarity.” Information Sciences 483: 53–64. https://doi.org/10.1016/j.ins.2019.01.023
- Belpaeme, T., J. Kennedy, A. Ramachandran, B. Scassellati, and F. Tanaka. 2018. “Social Robots for Education: A Review.” Science Robotics 3 (21): eaat5954. https://doi.org/10.1126/scirobotics.aat5954
- Bóna, J. 2014. “Temporal Characteristics of Speech: The Effect of Age and Speech Style.” Journal of the Acoustical Society of America 136 (2): El116–El121. https://doi.org/10.1121/1.4885482.
- Breazeal, C., K. Dautenhahn, and T. Kanda. 2016. “Social Robotics.” In Springer Handbook of Robotics, edited by B. Siciliano, and O. Khatib, 1935–1972. Berlin: Springer International Publishing. https://doi.org/10.1007/978-3-319-32552-1_72.
- Buchem, I. 2023. “Scaling-Up Social Learning in Small Groups with Robot Supported Collaborative Learning (RSCL): Effects of Learners’ Prior Experience in the Case Study of Planning Poker with the Robot NAO.” Applied Sciences 13 (7): 4106. https://doi.org/10.3390/app13074106
- Cabibihan, J.-J., H. Javed, M. Ang, and S. M. Aljunied. 2013. “Why Robots? A Survey on the Roles and Benefits of Social Robots in the Therapy of Children with Autism.” International Journal of Social Robotics 5 (4): 593–618. https://doi.org/10.1007/s12369-013-0202-2
- Carros, F., J. Meurer, D. Löffler, D. Unbehaun, S. Matthies, I. Koch, R. Wieching, D. Randall, M. Hassenzahl, and V. Wulf. 2020. “Exploring Human-Robot Interaction with the Elderly: Results From a Ten-Week Case Study in a Care Home.” In Proceedings of the 2020 CHI Conference on Human Factors in Computing Systems.
- Chen, Y.-L., C.-C. Hsu, C.-Y. Lin, and H.-H. Hsu. 2022. “Robot-Assisted Language Learning: Integrating Artificial Intelligence and Virtual Reality Into English Tour Guide Practice.” Education Sciences 12 (7): 437. https://doi.org/10.3390/educsci12070437
- Christian, H., M. P. Agus, and D. Suhartono. 2016. “Single Document Automatic Text Summarization Using Term Frequency-Inverse Document Frequency (TF-IDF).” ComTech: Computer, Mathematics and Engineering Applications 7 (4): 285–294. https://doi.org/10.21512/comtech.v7i4.3746
- Cruz-Ramírez, S. R., M. García-Martínez, and J. M. Olais-Govea. 2022. “NAO Robots as Context to Teach Numerical Methods.” International Journal on Interactive Design and Manufacturing (IJIDeM) 16 (4): 1337–1356. https://doi.org/10.1007/s12008-022-01065-y
- Das, B., S. Mandal, P. Mitra, and A. Basu. 2013. “Effect of Aging on Speech Features and Phoneme Recognition: A Study on Bengali Voicing Vowels.” International Journal of Speech Technology 16 (1): 19–31. https://doi.org/10.1007/s10772-012-9147-3.
- Daulatabad, V., P. Kamble, N. John, and J. John. 2022. “An Overview and Analogy of Pedagogical Approaches in Online-Offline Teaching Tactics in COVD-19 Pandemic.” Journal of Education and Health Promotion 11 (1): 341. https://doi.org/10.4103/jehp.jehp_11_22.
- Debnath, S., P. Roy, S. Namasudra, and R. G. Crespo. 2023. “Audio-Visual Automatic Speech Recognition Towards Education for Disabilities.” Journal of Autism and Developmental Disorders 53 (9): 3581–3594.
- Donnermann, M., P. Schaper, and B. Lugrin. 2022. “Social Robots in Applied Settings: A Long-Term Study on Adaptive Robotic Tutors in Higher Education.” Frontiers in Robotics and AI 9: 1–12. https://doi.org/10.3389/frobt.2022.831633.
- Ekström, S., and L. Pareto. 2022. “The Dual Role of Humanoid Robots in Education: As Didactic Tools and Social Actors.” Education and Information Technologies 27 (9): 12609–12644. https://doi.org/10.1007/s10639-022-11132-2.
- Engwall, O., and J. Lopes. 2022. “Interaction and Collaboration in Robot-Assisted Language Learning for Adults.” Computer Assisted Language Learning 35 (5-6): 1273–1309. https://doi.org/10.1080/09588221.2020.1799821
- Fu, R., J. Zhang, R. Wang, and T. Xu. 16-18 June 2023. “Improved Wavelet Thresholding Function and Adaptive Thresholding for Noise Reduction.” In 2023 11th International Conference on Intelligent Computing and Wireless Optical Communications (ICWOC).
- Grassini, S. 2023. “Shaping the Future of Education: Exploring the Potential and Consequences of AI and ChatGPT in Educational Settings.” Education Sciences 13 (7): 692. https://doi.org/10.3390/educsci13070692
- Graves, A., A. R. Mohamed, and G. Hinton. 26-31 May 2013. “Speech Recognition with Deep Recurrent Neural Networks.” In 2013 IEEE International Conference on Acoustics, Speech and Signal Processing.
- Guldner, S., N. Lavan, C. Lally, L. Wittmann, F. Nees, H. Flor, and C. McGettigan. 2024. “Human Talkers Change Their Voices to Elicit Specific Trait Percepts.” Psychonomic Bulletin & Review 31: 209–222. https://doi.org/10.3758/s13423-023-02333-y.
- Healy, E. W., S. E. Yoho, Y. Wang, and D. Wang. 2013. “An Algorithm to Improve Speech Recognition in Noise for Hearing-Impaired Listeners.” The Journal of the Acoustical Society of America 134 (4): 3029–3038. https://doi.org/10.1121/1.4820893
- Hinton, G., L. Deng, D. Yu, G. E. Dahl, A. R. Mohamed, N. Jaitly, A. Senior, et al. 2012. “Deep Neural Networks for Acoustic Modeling in Speech Recognition: The Shared Views of Four Research Groups.” IEEE Signal Processing Magazine 29 (6): 82–97. https://doi.org/10.1109/MSP.2012.2205597.
- Joshi, O., B. Chapagain, G. Kharel, N. C. Poudyal, B. D. Murray, and S. R. Mehmood. 2022. “Benefits and Challenges of Online Instruction in Agriculture and Natural Resource Education.” Interactive Learning Environments 30 (8): 1402–1413. https://doi.org/10.1080/10494820.2020.1725896
- Keizer, R. A. C. M. O., L. van Velsen, M. Moncharmont, B. Riche, N. Ammour, S. Del Signore, G. Zia, H. Hermens, and A. N'Dja. 2019. “Using Socially Assistive Robots for Monitoring and Preventing Frailty among Older Adults: A Study on Usability and User Experience Challenges.” Health and Technology 9 (4): 595–605. https://doi.org/10.1007/s12553-019-00320-9.
- Kennedy, J., P. Baxter, and T. Belpaeme. 2015. “The Robot Who Tried Too Hard: Social Behaviour of a Robot Tutor Can Negatively Affect Child Learning.” In Proceedings of the Tenth Annual ACM/IEEE International Conference on Human-Robot Interaction.
- Kennedy, J., P. Baxter, E. Senft, and T. Belpaeme. 2016. “Social Robot Tutoring for Child Second Language Learning.” In 2016 11th ACM/IEEE international conference on human-robot interaction (HRI).
- Kennedy, J., S. Lemaignan, C. Montassier, P. Lavalade, B. Irfan, F. Papadopoulos, E. Senft, and T. Belpaeme. 2017. “Child Speech Recognition in Human-Robot Interaction: Evaluations and Recommendations.” In Proceedings of the 2017 ACM/IEEE International Conference on Human-Robot Interaction (Hri'17), 82–90. https://doi.org/10.1145/2909824.3020229.
- Klakow, D., and J. Peters. 2002. “Testing the Correlation of Word Error Rate and Perplexity.” Speech Communication 38 (1-2): 19–28. https://doi.org/10.1016/S0167-6393(01)00041-3
- Koch, A. B. 2017. “Sounds of Education: Teacher Role and Use of Voice in Interactions with Young Children.” International Journal of Early Childhood 49 (1): 57–72. https://doi.org/10.1007/s13158-017-0184-6
- Komatsubara, T., M. Shiomi, T. Kanda, H. Ishiguro, and N. Hagita. 2014. “Can a Social Robot Help Children's Understanding of Science in Classrooms?” In Proceedings of the second international conference on Human-agent interaction.
- Kory-Westlund, J. M., and C. Breazeal. 2019. “Exploring the Effects of a Social Robot's Speech Entrainment and Backstory on Young Children's Emotion, Rapport, Relationship, and Learning.” Frontiers in Robotics and Ai 6: 54. https://doi.org/10.3389/frobt.2019.00054
- Lanzilotti, R., A. Piccinno, V. Rossano, and T. Roselli. 2021. “Social Robot to Teach Coding in Primary School.” In 2021 International Conference on Advanced Learning Technologies (ICALT).
- Lee, H., and J. H. Lee. 2022. “The Effects of Robot-Assisted Language Learning: A Meta-Analysis.” Educational Research Review 35: 100425. https://doi.org/10.1016/j.edurev.2021.100425.
- Leyzberg, D., S. Spaulding, M. Toneva, and B. Scassellati. 2012. “The Physical Presence of a Robot Tutor Increases Cognitive Learning Gains.” In Proceedings of the annual meeting of the cognitive science society.
- Li, J. 2015. “The Benefit of Being Physically Present: A Survey of Experimental Works Comparing Copresent Robots, Telepresent Robots and Virtual Agents.” International Journal of Human-Computer Studies 77: 23–37. https://doi.org/10.1016/j.ijhcs.2015.01.001.
- Li, D., Y. Gao, C. Zhu, Q. Wang, and R. Wang. 2023. “Improving Speech Recognition Performance in Noisy Environments by Enhancing Lip Reading Accuracy.” Sensors 23 (4): 2053. https://doi.org/10.3390/s23042053
- Liao, J., S. Eskimez, L. Lu, Y. Shi, M. Gong, L. Shou, H. Qu, and M. Zeng. 2023. “Improving Readability for Automatic Speech Recognition Transcription.” ACM Transactions on Asian and Low-Resource Language Information Processing 22 (5): 1–23. https://doi.org/10.1145/3557894
- Lin, C.-Y. 2004. Rouge: A Package for Automatic Evaluation of Summaries. Text summarization branches out.
- Lin, Y., Q. Li, B. Yang, Z. Yan, H. Tan, and Z. Chen. 2021. “Improving Speech Recognition Models with Small Samples for air Traffic Control Systems.” Neurocomputing 445: 287–297. https://doi.org/10.1016/j.neucom.2020.08.092.
- Liu, F.-H., Y. Gao, L. Gu, and M. Picheny. 2003. “Noise Robustness in Speech to Speech Translation.” In Eighth European Conference on Speech Communication and Technology.
- Matre, M. E. 2022. “Speech-to-Text Technology as an Inclusive Approach: Lower Secondary Teachers’ Experiences.” Nordisk Tidsskrift for Pedagogikk og Kritikk 8: 233–247. https://doi.org/10.23865/ntpk.v8.3436.
- Matre, M. E., and D. L. Cameron. 2024. “A Scoping Review on the Use of Speech-to-Text Technology for Adolescents with Learning Difficulties in Secondary Education.” Disability and Rehabilitation: Assistive Technology 19 (3): 1103–1116. https://doi.org/10.1080/17483107.2022.2149865.
- Mavrikis, M., B. Grawemeyer, A. Hansen, and S. Gutierrez-Santos. 2014. “Exploring the Potential of Speech Recognition to Support Problem Solving and Reflection: Wizards Go to School in the Elementary Maths Classroom.” In Open Learning and Teaching in Educational Communities: 9th European Conference on Technology Enhanced Learning, EC-TEL 2014, Graz, Austria, September 16-19, 2014, Proceedings 9.
- Mehra, S., V. Ranga, and R. Agarwal. 2024. “Improving Speech Command Recognition Through Decision-Level Fusion of Deep Filtered Speech Cues.” Signal, Image and Video Processing 18: 1365–1373. https://doi.org/10.1007/s11760-023-02845-z.
- Meyer, J., L. Dentel, and F. Meunier. 2013. “Speech Recognition in Natural Background Noise.” PLoS One 8 (11): 1–14. https://doi.org/10.1371/journal.pone.0079279.
- Mubin, O., C. Bartneck, L. Feijs, H. Hooft van Huysduynen, J. Hu, and J. Muelver. 2012. “Improving Speech Recognition with the Robot Interaction Language.” Disruptive Science and Technology 1 (2): 79–88. https://doi.org/10.1089/dst.2012.0010
- Mubin, O., J. Henderson, and C. Bartneck. 25–29 Aug. 2014. “You Just Do Not Understand Me! Speech Recognition in Human Robot Interaction.” In The 23rd IEEE International Symposium on Robot and Human Interactive Communication.
- Nakanishi, J., I. Kuramoto, J. Baba, K. Ogawa, Y. Yoshikawa, and H. Ishiguro. 2020. “Continuous Hospitality with Social Robots at a Hotel.” SN Applied Sciences 2 (3): 1–13. https://doi.org/10.1007/s42452-020-2192-7
- Naoqi API documentation –ALAudioRecorder. http://doc.aldebaran.com/2-5/naoqi/audio/alaudiorecorder.html, last accessed 2023/05/07.
- Nematollahi, M. A., and S. A. R. Al-Haddad. 2016. “Distant Speaker Recognition: An Overview.” International Journal of Humanoid Robotics 13 (02): 1–45. https://doi.org/10.1142/S0219843615500322.
- Niemelä, M., P. Heikkilä, H. Lammi, and V. Oksman. 2019. “A Social Robot in a Shopping Mall: Studies on Acceptance and Stakeholder Expectations.” In Social Robots: Technological, Societal and Ethical Aspects of Human-Robot Interaction, edited by O. Korn, 119–144. Cham: Springer.
- Nusseck, M., A. Immerz, B. Richter, and L. Traser. 2022. “Vocal Behavior of Teachers Reading with Raised Voice in a Noisy Environment.” International Journal of Environmental Research and Public Health 19 (15): 1–15. https://doi.org/10.3390/ijerph19158929.
- OpenAI Whisper. https://openai.com/research/whisper, last accessed 2023/05/07.
- Pan, Y., H. Okada, T. Uchiyama, and K. Suzuki. 2015. “On the Reaction to Robot’s Speech in a Hotel Public Space.” International Journal of Social Robotics 7 (5): 911–920. https://doi.org/10.1007/s12369-015-0320-0.
- Pande, A., and D. Mishra. 2023. “The Synergy Between a Humanoid Robot and Whisper: Bridging a Gap in Education.” Electronics 12: 19. https://doi.org/10.3390/electronics12193995.
- Pande, A., S. B. Rani A, and D. Mishra. 2023. “A Comparative Analysis of Real Time Open-Source Speech Recognition Tools for Social Robots.” In HCI International Conference.
- Pandey, D., A. Subedi, and D. Mishra. 2022. “Improving Language Skills and Encouraging Reading Habits in Primary Education: A Pilot Study using NAO Robot.” In 2022 IEEE/SICE International Symposium on System Integration (SII).
- Papineni, K., S. Roukos, T. Ward, and W.-J. Zhu. 2002. “Bleu: A Method for Automatic Evaluation of Machine Translation.” In Proceedings of the 40th annual meeting of the Association for Computational Linguistics.
- Paramiko documentation. https://www.paramiko.org/, last accessed 2023/05/07.
- Pei, L., and H. Wu. 2019. “Does Online Learning Work Better Than Offline Learning in Undergraduate Medical Education? A Systematic Review and Meta-Analysis.” Medical Education Online 24 (1): 1666538. https://doi.org/10.1080/10872981.2019.1666538.
- Pinto, D., D. Vilariño, Y. Alemán, H. Gómez, N. Loya, and H. Jiménez-Salazar. 2012. “The Soundex Phonetic Algorithm Revisited for SMS Text Representation.” In Text, Speech and Dialogue: 15th International Conference, TSD 2012, Brno, Czech Republic, September 3–7, 2012. Proceedings 15.
- Rajeb, M., Y. Wang, K. Man, and L. M. Morett. 2023. “Students’ Acceptance of Online Learning in Developing Nations: Scale Development and Validation.” Educational Technology Research and Development 71 (2): 767–792. https://doi.org/10.1007/s11423-022-10165-1
- Randall, N. 2019. “A Survey of Robot-Assisted Language Learning (RALL).” ACM Transactions on Human-Robot Interaction (THRI) 9 (1): 1–36.
- Rebai, I., Y. BenAyed, W. Mahdi, and J.-P. Lorré. 2017. “Improving Speech Recognition Using Data Augmentation and Acoustic Model Fusion.” Procedia Computer Science 112: 316–322. https://doi.org/10.1016/j.procs.2017.08.003.
- Rodríguez-Hidalgo, C., and N. Sibrian. 2022. “Using a Social Robot to Aid Online Learning: Identifying Teachers’ Media and Digital Competencies, Barriers and Opportunities.” In Communication and Applied Technologies: Proceedings of ICOMTA 2022, edited by P. C. López-López, Á. Torres-Toukoumidis, A. De-Santis, Ó. Avilés, and D. Barredo, 117–132. Singapore: Springer.
- Rodrigues, A., R. Santos, J. Abreu, P. Beça, P. Almeida, and S. Fernandes. 2019. “Analyzing the Performance of ASR Systems The Effects of Noise, Distance to the Device, Age and Gender.” In Proceedings of the Xx International Conference on Human-Computer Interaction (Interaccion'2019). https://doi.org/10.1145/3335595.3335635.
- Rosenberg-Kima, R. B., Y. Koren, and G. Gordon. 2020. “Robot-Supported Collaborative Learning (RSCL): Social Robots as Teaching Assistants for Higher Education Small Group Facilitation.” Frontiers in Robotics and Ai 6: 148. https://doi.org/10.3389/frobt.2019.00148
- Rustam, S., H. Wu-Yuin, C. Nian-Shing, and H. Yueh-Min. 2014. “Review of Speech-to-Text Recognition Technology for Enhancing Learning.” Journal of Educational Technology & Society 17 (4): 65–84. http://www.jstor.org/stable/jeductechsoci.17.4.65.
- Sawalha, M., and M. Abu Shariah. 2013. “The Effects of Speakers’ Gender, Age, and Region on Overall Performance of Arabic Automatic Speech Recognition Systems Using the Phonetically Rich and Balanced Modern Standard Arabic Speech Corpus.” In Proceedings of the 2nd Workshop of Arabic Corpus Linguistics WACL-2.
- Shimada, M., T. Kanda, and S. Koizumi. 2012. “How Can a Social Robot Facilitate Children’s Collaboration?” In Social Robotics: 4th International Conference, ICSR 2012, Chengdu, China, October 29-31, 2012. Proceedings 4.
- Sinai, D., and R. B. Rosenberg-Kima. 7-10 March 2022. “Perceptions of Social Robots as Motivating Learning Companions for Online Learning.” 2022 17th ACM/IEEE International Conference on Human-Robot Interaction (HRI).
- So, S., and N. Lee. 2023. “Pedagogical Exploration and Technological Development of a Humanoid Robotic System for Teaching to and Learning in Young Children.” Cogent Education 10 (1): 2179181. https://doi.org/10.1080/2331186X.2023.2179181
- Sun, W. 2023. “The Impact of Automatic Speech Recognition Technology on Second Language Pronunciation and Speaking Skills of EFL Learners: A Mixed Methods Investigation.” Frontiers in Psychology 14: 1–14. https://doi.org/10.3389/fpsyg.2023.1210187.
- Sun, X., C. Cao, Q. Li, L. Wang, and F. Xiang. 23-27 May 2022. “Explore Relative and Context Information with Transformer for Joint Acoustic Echo Cancellation and Speech Enhancement.” In ICASSP 2022 - 2022 IEEE International Conference on Acoustics, Speech and Signal Processing (ICASSP).
- Tatman, R. 2017. “Gender and Dialect Bias in YouTube’s Automatic Captions.” In Proceedings of the First ACL Workshop on Ethics in Natural Language Processing.
- Topaz, M., M. Zolnoori, A. A. Norful, A. Perrier, Z. Kostic, and M. George. 2022. “Speech Recognition Can Help Evaluate Shared Decision Making and Predict Medication Adherence in Primary Care Setting.” PLoS One 17 (8): e0271884. https://doi.org/10.1371/journal.pone.0271884
- Trelease, R. B. 2016. “From Chalkboard, Slides, and Paper to e-Learning: How Computing Technologies Have Transformed Anatomical Sciences Education.” Anatomical Sciences Education 9 (6): 583–602. https://doi.org/10.1002/ase.1620
- Veeraiyan, D. N., S. S. Varghese, A. Rajasekar, M. I. Karobari, L. Thangavelu, A. Marya, P. Messina, and G. A. Scardina. 2022. “Comparison of Interactive Teaching in Online and Offline Platforms among Dental Undergraduates.” International Journal of Environmental Research and Public Health 19 (6): 3170. https://doi.org/10.3390/ijerph19063170
- Vishwakarma, L. P., R. K. Singh, R. Mishra, D. Demirkol, and T. Daim. 2024. “The Adoption of Social Robots in Service Operations: A Comprehensive Review.” Technology in Society 76: 102441. https://doi.org/10.1016/j.techsoc.2023.102441.
- Vogt, P., R. van den Berghe, M. De Haas, L. Hoffman, J. Kanero, E. Mamus, J.-M. Montanier, C. Oranç, O. Oudgenoeg-Paz, and D. H. García. 2019. “Second Language Tutoring Using Social Robots: A Large-Scale Study.” In 2019 14th ACM/IEEE International Conference on Human-Robot Interaction (HRI).
- Wiyono, B. B., A. Wedi, D. E. Kusumaningrum, and S. Ulfa. 27–29 March 2021. “Comparison of the Effectiveness of Using Online and Offline Communication Techniques to Build Human Relations with Students in Learning at Schools.” In 2021 9th International Conference on Information and Education Technology (ICIET).
- Yen, S.-C., Y. Lo, A. Lee, and J. Enriquez. 2018. “Learning Online, Offline, and In-Between: Comparing Student Academic Outcomes and Course Satisfaction in Face-to-Face, Online, and Blended Teaching Modalities.” Education and Information Technologies 23 (5): 2141–2153. https://doi.org/10.1007/s10639-018-9707-5.
- Yeshoda, K., and R. Rajasudhakar. 2023. “Acoustic Characteristics of Voice in Teachers and Nonteachers.” Journal of Voice 1–7. https://doi.org/10.1016/j.jvoice.2023.09.019.
- Youssef, K., S. Said, T. Beyrouthy, and S. Alkork. 2021. “A Social Robot with Conversational Capabilities for Visitor Reception: Design and Framework.” In 2021 4th International Conference on Bio-Engineering for Smart Technologies (BioSMART).
- Zahorik, P., and J. W. Kelly. 2007. “Accurate Vocal Compensation for Sound Intensity Loss with Increasing Distance in Natural Environments.” Journal of the Acoustical Society of America 122 (5): El143–El150. https://doi.org/10.1121/1.2784148.
- Zhu, W., Q. Liu, and X. Hong. 2022. “Implementation and Challenges of Online Education During the COVID-19 Outbreak: A National Survey of Children and Parents in China.” Early Childhood Research Quarterly 61: 209–219. https://doi.org/10.1016/j.ecresq.2022.07.004.