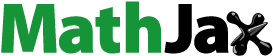
Abstract
The traditional approach decomposing profit inefficiency into the sum of its technical and its allocative components identifies first the frontier projection of each firm based on the exogenous choice of a specific technical measure, e.g., based on slacks, directional, etc. However, in real life situations, firms and organizations are interested in benchmarking themselves against competitors representing the largest feasible profit improvement given market prices. Resorting to the recently defined general direct approach decomposition of profit inefficiency, which decomposes profit loss into the profit technical gap existing between the firm and its frontier projection, and a remaining profit allocative gap, we introduce a decomposition that endogenizes the technical component. This is achieved by securing technical inefficiency reductions that, simultaneously, search to maximize the profit of the projected benchmark. The proposal defines a new measure of technical inefficiency that corresponds to a monetized version of the weighted additive model. We also present a normalized version of the reversed decomposition that is units’ invariant through the definition of a suitable normalization factor.
1. Introduction
Bogetoft and Hougaard (Citation2003) proposed years ago a change of paradigm when measuring economic inefficiency. Rather than focusing solely on reducing technical inefficiency per se, these authors indicate that a firm could be interested in directly improving its economic performance, without focusing primarily on the technical dimension. That is, market conditions and economic performance are brought to the forefront of the efficiency evaluation, while the reduction of technical inefficiency is contingent on achieving a higher economic goal, e.g., profit maximization, and therefore can be seen as the result of dynamic or transitory adjustments towards the final economic goal. In fact, they argue that “technical inefficiency can be part of the (fringe) compensation paid to stake holders…It can create loyal employees and thereby reduce costly turnovers in the labour force”. Bogetoft et al. (Citation2006) added one more argument for adopting what they named “the reverse approach”, suggesting that employees and stakeholders must be convinced to recognize that “doing the right things according to the market conditions is the first goal of an organization”.Footnote1 From a conceptual perspective the reverse approach brings the improvement of the economic performance of the firm to the forefront of efficiency analysis, i.e., solving technical and allocative inefficiencies should be linked to the economic goal of the firm, most notably maximum profit.
The reverse approach is in stark contrast to the traditional approach decomposing profit inefficiency, which focus on technical inefficiency and treats allocative inefficiency as a residual. The latter approach consists of the next two steps: first, a certain technical inefficiency measure is exogenously chosen, as well as its associated Fenchel-Mahler inequality, establishing that, for each firm, its profit inefficiency is greater or equal than its technical inefficiency times a positive normalization factor; and second, the mentioned inequality is transformed dividing by the normalization factor so as to get that the resulting normalized economic inefficiency is greater than or equal to its technical inefficiency, deriving then its allocative inefficiency as a residual. The traditional approach for decomposing profit inefficiency was introduced by Chambers et al.’s (Citation1998), who show the duality between the graph directional distance function (DDF) and the profit function. This duality enables the definition of a Fenchel-Mahler inequality where normalized profit inefficiency is greater than or equal to the directional distance function (Luenberger, Citation1992; Chambers et al.’s, 1996). Despite being the DDF model the most popular choice to decompose economic inefficiency, many authors have adapted this methodology to decompose profit inefficiency based on alternative technical inefficiency measures. For example, Cooper et al. (Citation2011a) show the duality between the weighted additive technical inefficiency measure and the profit function. Depending on the weights this proposal encompasses a number of normalized decompositions of the weighted additive model, including the measure of inefficiency proportions (MIP) introduced by Cooper et al. (Citation1999), the range adjusted measure (RAM) of inefficiency, Cooper et al. (Citation1999), and the bounded adjusted measure (BAM) of inefficiency, Cooper et al. (Citation2011b). In this study, to develop the standard reverse approach, we propose a novel model that considers input and output prices as weights of the additive technical inefficiency measure. Additionally, Aparicio et al. (Citation2016) propose the weighted additive distance function and derive its associated Fenchel-Mahler inequality, while Aparicio et al. (Citation2017) develop the duality that allows decomposing profit inefficiency using the Enhanced Russell Graph Measure introduced by Pastor et al. (Citation1999)—also known as Slack-Based Measure, see Tone (Citation2001). Finally, traditional decompositions of economic inefficiency are possible through the Russell graph measure introduced by Färe and Lovell (Citation1978), who extend the input- and output-oriented counterparts. Halická and Trnovská (Citation2018) present an exact method to calculate the original graph Russell measure through semidefinite programming, and establish its duality with the profit function, which allows its decomposition.
Recently, Pastor et al. (Citation2022) introduced a methodology that provides an alternative to the above traditional approach and does not require to derive duality results and their associated Fenchel-Mahler inequalities. It is based on the natural relationship between each firm, its projection, and the slacks that connect them. This approach is known as the general direct approach because it is valid for any technical inefficiency measure. Once the projection on the frontier associated to a given a measure is identified, the general direct approach decomposes the economic loss associated to profit inefficiency into a profit technical gap, separating observed profit from the profit at the projected benchmark, and a profit allocative gap, which is the profit inefficiency of the projected benchmark with respect to maximum profit. The sum of both profit losses, technical and allocative, yields the difference between maximum profit and observed profit, i.e., the profit inefficiency of the firm under evaluation.
In this paper, inspired by the reverse approach of Bogetoft et al. (Citation2006) and relying on the general direct approach by Pastor et al. (Citation2022), we introduce a new procedure to decompose profit inefficiency that starts defining a new inefficiency measure corresponding to a singular weighted additive model, whose weights are precisely the market prices. This model identifies as projection on the technical frontier the benchmark whose profit is the largest attainable by reducing the input slacks and increasing the output slacks. This procedure is equivalent to find the projection whose allocative inefficiency is the smallest, bringing economic criteria to the forefront of the analysis, i.e., the reverse approach (eventually, if the projected benchmark corresponds to a profit maximizing firm, the remaining allocative inefficiency is zero). The relationship between each firm and its projection exhibiting the largest feasible profit, expressed through an equality, gives rise to a new decomposition based on the general direct approach. That is, it decomposes profit inefficiency into technical and allocative criteria, with the former measuring the profit loss due to technical inefficiency, and the second one is related to the profit inefficiency of the mentioned projection. By dividing the equality by a suitable normalization factor, we end up with the corresponding normalized economic inefficiency decomposition that identifies its second term as its allocative inefficiency, with the novelty that it is directly related to the identified technical projection.
The paper unfolds as follows. In Section 2, we present the traditional approach to decompose profit inefficiency based on the weighted additive technical inefficiency measure. Then, in Section 3 we introduce the new standard reverse profit inefficiency decomposition, based on the equality that relates each firm with its projection through the corresponding slacks. This allows us to derive a new graph technical inefficiency measure and prove its properties. We then present the standard reverse decomposition in monetary terms and, subsequently, considering a suitable normalization factor, introduce its normalized counterpart, which is units’ invariant. Section 4 presents an illustrative numerical example, while Sections 5 and 6 adapt the methodology to the decomposition of cost and revenue inefficiency as particular cases. The last section draws the main conclusions.
2. The traditional approach to decompose profit inefficiency
To ease the understanding of the new methodology we formalize first the background required for the measurement and decomposition of profit inefficiency and present the traditional approach to decompose profit inefficiency based on Fenchel-Mahler inequalities. Data Envelopment Analysis approximates the production technology from observed cross-sectional data, relying on the Activity Analysis approach introduced by Koopmans (Citation1951). Based on the principle of minimum extrapolation, DEA yields the smallest subset of the input-output space as an inner approximation containing all observations and satisfying certain technological assumptions: convexity and free disposability of inputs and outputs. DEA generates convex polyhedral technologies (i.e., intersections of finite numbers of half-spaces), consisting of piecewise linear combinations of a finite sample of firms
thereby allowing for multiple inputs,
and outputs,
In the DEA framework, the production technology can be approximated as follows:
(1)
(1)
Where denotes the input-output vector of each observation j,
Since we are interested in profit inefficiency, we assume that the technology is characterized by variables returns to scale, VRS, ensuring that at least one profit maximizing firm exists and belongs to the strongly (Pareto-Koopmans) efficient production possibility set (Koopmans, Citation1951, p. 60).Footnote2 The strongly efficient production set corresponds to:
(2)
(2)
Let us consider the specific firm j = o, denoted by Let us focus on the traditional approach that seeks a projection on the strongly efficient frontier Equation(2)
(2)
(2) , denoted by
It is assumed that
or, equivalently, that both input-slacks
and output-slacks
are non-negative. Within DEA methods, we can resort to several additive measures to achieve this goal. Here, to consider the reverse approach, we rely on the standard weighted additive technical inefficiency measure (WA) to determine such projection. Following Lovell and Pastor (Citation1995), the corresponding model is (see also Pastor et al., Citation2012):
(3)
(3)
The vectors and
contain, respectively, input and output weights representing the relative importance of unit inputs and unit outputs from a technical perspective.Footnote3 If
is an optimal solution of Equation(3)
(3)
(3) , then the corresponding projection point is defined as
We can determine the efficiency status of the firm under evaluation by looking at the optimal slacks
A firm under evaluation is deemed efficient if, and only if, the optimal slack values are all equal to 0, i.e.,
= 0; otherwise, it is technically inefficient,
> 0.
Regarding the economic dimension, let us assume that a common fixed vector of market prices is known. Consequently, the market value of the inputs of firm
i.e., observed cost, is given by the interior product
while that corresponding to its outputs, i.e., observed revenue, is
We can then define observed profit as revenue less cost, i.e.,
Since each
is expressed in its own quantity units,
and, similarly, each
is expressed in its own quantity units
we conclude that each input price
must be expressed in
and each output price
in
so that the profit generated by firm
is expressed in $. Let us further denote as
the maximum profit associated with sample of firms F,
and, based on it, let us further define the profit inefficiency of firm
as
(4)
(4)
Profit inefficiency can be decomposed considering technical and allocative criteria by making use of the benchmark projection obtained through the traditional approach—model Equation(3)
(3)
(3) . Expressing the firm under evaluation as
and substituting last equality into Equation(4)
(4)
(4) , we obtain the following decomposition:
(5)
(5)
In these expressions we identify a term measuring the firm’s technical profit inefficiency: =
and a second term measuring allocative profit inefficiency:
=
That is,
(6)
(6)
A desirable characteristic of profit inefficiency is that it should satisfy the so-called commensurability property, thereby being units’ invariant. Unfortunately, expression Equation(6)(6)
(6) does not satisfy this property as it depends on the reference monetary units, i.e., $. Units’ invariance can be achieved by dividing profit inefficiency by a suitable normalization factor. Duality theory relates profit inefficiency and technical inefficiency measures through the so-called Fenchel-Mahler inequality in terms of the corresponding normalization factor. Cooper et al. (Citation2011a) proved the following Fenchel-Mahler inequality between normalized (N) profit inefficiency and the value of the Weighted Additive Measure Equation(3)
(3)
(3) .
(7)
(7)
where
is the normalization factor. The normalization of profit inefficiency prompts us to denote it by including
since one can consider that the normalization factor is associated with prices: i.e.,
The decomposition of normalized profit inefficiency is completed by closing the above inequality. In this way, recalling expression Equation(5)
(5)
(5) , normalized allocative inefficiency is defined as
=
/
Last equality shows that normalized allocative inefficiency is interpreted as the profit loss between maximum profit and profit at the efficient projection:
≡
Therefore, normalized profit inefficiency can be decomposed into the weighted additive technical inefficiency measure and normalized allocative inefficiency:
(8)
(8)
It is easy to see that these terms depend on the normalization factor whose economic meaning is dubious, thereby hampering the interpretation of the values of the normalized decomposition.
3. The standard reverse approach to decompose profit inefficiency
Let us now introduce the Standard Reverse method (SR) to decompose profit inefficiency, which does not rely on duality theory and Fenchel-Mahler inequalities. It resorts to the general direct approach, see Pastor et al. (Citation2022), which only needs to know for each firm its efficient projection. In particular, the reverse approach starts identifying the projection of the firm under evaluation,
satisfying
but searching for the maximum profit gains, as shown below. Considering the last equality, together with the profit inefficiency of
and its projection, we now get the next equalities:
(9)
(9)
Under the reverse approach, this expression follows the structure of expression Equation(5)(5)
(5) , by decomposing profit inefficiency in the sum of firms’ technical profit inefficiency:
=
and allocative profit inefficiency:
=
Therefore, we now propose the following equality decomposing profit inefficiency in monetary terms:
(10)
(10)
Contrary to the traditional decomposition in expression Equation(6)(6)
(6) , which is based on technical grounds by relying on the exogenous choice of a technical efficiency measure, e.g., the weighted additive measure Equation(3)
(3)
(3) , the reverse approach Equation(10)
(10)
(10) brings economic performance to the forefront of the efficiency analysis. The economic criterion underlying the reverse approach when searching for the best target on the frontier is to aim at the benchmark with the highest possible profit or, alternatively, that minimizes the firm’s allocative inefficiency with respect to maximum profit
Since the left-hand side term in Equation(10)
(10)
(10) is given for each firm
our goal is to maximize
Hence, we propose to solve the following linear model measuring the technical profit inefficiency term:
(11)
(11)
By using market prices as weights, i.e., and
this is an economically driven weighted additive model Equation(3)
(3)
(3) .Footnote4 Consequently, the objective function is expressed in monetary units. Model Equation(11)
(11)
(11) searches for a target on the production frontier with the maximum feasible profit. In case the optimal solution is
firm
is technically efficient, while if
it is technically inefficient. A relevant feature of the new model is that, as its original weighted additive precursor Equation(3)
(3)
(3) , it is able to classify each firm as strongly efficient by belonging to Equation(2)
(2)
(2) —in case all optimal slacks are equal to 0—or inefficient, otherwise.Footnote5 The virtue of model Equation(11)
(11)
(11) is that it allows us to define in what follows a new technical inefficiency measure, which we now formally call the standard reverse graph technical inefficiency measure. This new measure is instrumental in the normalized decomposition of profit inefficiency. Consequently, we first prove that it is a true technical inefficiency measure satisfying the usual properties.
3.1. The standard reverse technical inefficiency measure
Let us now elaborate the definition of the new technical inefficiency measure associated with the standard reverse approach and show that it satisfies the usual properties (Cooper et al., Citation1999). Linear model Equation(11)(11)
(11) gives us an optimal non-negative objective function value for each firm of our sample, which means that we have exactly J optimal output and input slack values. Assuming that our sample of firms contains at least one inefficient firm, any upper bound for the optimal values of model Equation(11)
(11)
(11) is positive. After solving model Equation(11)
(11)
(11) for all firms, let us consider the upper bound USR of the calculated optimal values:
(12)
(12)
This value corresponds to at least one of the inefficient firms of our sample and, therefore, Now we are ready to define the standard reverse technical inefficiency measure
Definition 1.
For firm of our finite sample, the standard reverse technical inefficiency measure
is defined as:
(13)
(13)
For each firm, there are two remarkable differences between in Equation(10)
(10)
(10) and
as defined in Equation(13)
(13)
(13) . First,
is a pure number, since
is expressed in the same monetary units as
and
and second,
is bounded by 0 and 1, because
is bounded by 0$and
Footnote6 The new introduced technical inefficiency measure
satisfies four basic properties, first introduced by Cooper et al. (Citation1999) for technical efficiency measures, TE = 1−TI. Since in our economic context we are interested in inefficiency measures, we are going to enunciate and proof the corresponding versions for
These properties are indication, strong monotonicity, inefficiency requirement (as called by Sueyoshi & Sekitani, Citation2009) and commensurability. Regarding strong monotonicity, it is relevant to remark that, in the case of the standard reverse approach, this property is satisfied within the sample, i.e., comparing any two firms j = k, l ∈ J, if firm k dominates firm l in the sense of Pareto-Koopmans, then the inefficiency of the latter is always greater than that of the former,
>
as it uses more of at least one input and/or produces less of at least one output.
Proposition 1.
, the SR technical inefficiency measure, satisfies the next four properties.
P1. Indication (of inefficiency): if, and only if,
is a Pareto-efficient firm.
P2. Strong (or strict) monotonicity: is a strongly monotonic inefficiency measure within the sample.
P3. Inefficiency requirement:
P4 Commensurability (or units’ invariance): is invariant to the units of measurement of inputs and outputs.
Proof.
P1. Every weighted additive model Equation(11)(11)
(11) with strictly positive weights satisfies the property of indication (see Cooper et al., Citation1999, and Halická & Trnovská, Citation2021).
P2. Every weighted additive model with strictly positive weights satisfies the property of strong monotonicity (see Cooper et al., Citation1999, and Halická & Trnovská, Citation2021). This implies that the numerator of Equation(13)(13)
(13) satisfies strong monotonicity. Given
i.e., a particular sample of DMUs, we calculate
which is a fixed value for the observations belonging to
Consequently, if
and
belong to
with DMU
dominating DMU
in the sense of Pareto-Koopmans, then
This strict inequality allows deriving that
that is,
>
P3. This property is a direct consequence of Equation(12)(12)
(12) and Equation(13)
(13)
(13) . Moreover, having shown indication and strong monotonicity, the existence of inefficient firms can be assumed, one of which will exhibit a technical inefficiency score of one through the adoption of the upper bound as normalizing factor, while the presence of at least one efficient firm guarantees that the lower bound is also reached. Hence our new technical inefficiency measure classifies the firms in two disjoint subsets: the subset of efficient firms, for which
and the subset of inefficient firms, for which
P4. Since and the units of measurement of numerator and denominator are the same, it is clear that, following Lovell and Pastor (Citation1995),
is units’ invariant. □
Note that the first two properties, indication and strong monotonicity, derive directly from the weighted additive model Equation(11)(11)
(11) , i.e., regardless of its transformation by the normalization factor.
3.2. The normalized standard reverse approach to decompose profit inefficiency
After having defined in the preceding section the technical inefficiency associated with the standard profit reverse approach, and based on equality Equation(10)(10)
(10) , we are ready to deduce the corresponding normalized profit inefficiency decomposition. Since equality Equation(13)
(13)
(13) can be reformulated as
equality Equation(10)
(10)
(10) can be rewritten as follows.
(14)
(14)
Knowing that we can divide each term of Equation(14)
(14)
(14) by this value, obtaining the corresponding normalized profit inefficiency decomposition associated with the reverse approach:Footnote7
(15)
(15)
Note that the upper bound of the technical profit inefficiencies represents a proper normalization factor in the decomposition of normalized profit inefficiency, Last equality also shows that allocative inefficiency, corresponding to the last term, is not obtained as a residual derived from Fenchel-Mahler inequalities, but directly from our reverse approach. We also know its definition in terms of the projection of
and of the obtained normalization factor; i.e.,
(16)
(16)
4. A numerical example
Let us illustrate the new standard reverse profit inefficiency decomposition and compare the obtained results to those of the traditional approach presented in Section 2. We consider eleven firms in the one input–one output space whose input and output values, denoted A thru K, are presented in the first column of . The first row specifies the content of each column. The second row replicates the first one introducing the associated mathematical notation used in previous sections, with the only exception of column 2, where the technical status of each firm—efficient or inefficient according to model Equation(3)(3)
(3) or Equation(11)
(11)
(11) , is reported. The third row identifies each column, C1, C2, …, C9, as well as the relation of each column with the preceding ones. The next eleven rows show the results associated with each firm.
Table 1. The traditional profit inefficiency decomposition based on the weighted additive model (Range Adjusted Measure, RAM). (w,p) = (1, 4).
We start reporting the values of the traditional decomposition choosing the weighted additive model Equation(3)(3)
(3) to measure technical efficiency. Specifically, we rely on the Range-Adjusted Measure (RAM) proposed by Cooper et al. (Citation1999) as it satisfies the set of desirable properties (P1-P4) previously considered to determine the suitableness of the normalized standard reverse technical inefficiency.Footnote8 For this measure, the respective input and output weights are
=
where
with
and
with
Solving model Equation(3)
(3)
(3) with these weights we categorize firms as efficient or inefficient, C2, and determine their input-output targets on the strongly efficient frontier Equation(2)
(2)
(2) —reported in C3. The first three firms are technically efficient as the objective function values zero. The remaining firms are inefficient obtaining a positive value.
Considering now market prices (w,p) = (1,4), we calculate the profit of each firm: finding that B maximizes profit, i.e.,
= 4 × 12 − 1 × 9 = $39. Subsequently, we obtain the profit inefficiency of each firm with respect to maximum profit,
as shown in (C4). The decomposition of profit inefficiency in monetary terms into technical profit inefficiency
and allocative profit inefficiency
according to expression Equation(6)
(6)
(6) is reported in columns C5 and C6. The first term corresponds to the values of the slacks separating each firm from its projection,
Consequently, all profit inefficiency is allocative for the technically efficient firms, while the rest of the firms exhibit both technical and allocative profit inefficiencies. The normalized version of profit inefficiency
obtained through duality—i.e., the Fenchel-Mahler inequality Equation(7)
(7)
(7) —ensures that these measures are units’ invariant. The normalization factor in this example is
=
=
=
= 12. The normalized values of technical and allocative inefficiencies,
and
are presented in columns C8 and C9. While these values do not depend on the units of measurement, their relative values are referred to the normalization factor, whose interpretation lacks economic meaning.
We now report the results of the profit inefficiency decomposition based on the standard reverse approach in . First, the efficiency status of each firm does not change with respect to the traditional approach because this result depends on the value of the slacks, and not on the choice of specific weights. However, we see that using markets prices as weights in order to search for the technical benchmark with the maximum feasible profit, i.e., that minimizing allocative profit inefficiency according to model Equation(8)(8)
(8) , results in different projections. Except for firm D, which is projected to the same reference benchmark using the traditional (RAM) and standard reverse models, all technically inefficient firms identify a different benchmark on the strongly efficient frontier, with the last five being directly projected to the profit maximizing firm B (see column C3).Footnote9 Consequently, while profit inefficiency in monetary terms
is the same in both models, see column C4 in and , its decomposition following expression Equation(10)
(10)
(10) will differ if firms identify alternative benchmarks, see columns C5 and C6. For these firms identifying different benchmarks, allocative profit inefficiency (C6) is now smaller than in the traditional approach:
>
Equivalently, technical profit inefficiency, equal to
is larger:
<
Moreover, allocative profit inefficiency drops to zero for the last five firms because having firm B as reference implies that, once projected to it, they become allocatively efficient. Accordingly, all profit inefficiency is technical.
Table 2. The standard reverse profit inefficiency decomposition. (w,p) = (1, 4).
Finally, we proceed to illustrate the decomposition of the normalized version of profit inefficiency according to the standard reverse approach, expression Equation(15)
(15)
(15) , whose values are presented in the last three columns of (C7, C8 and C9). To achieve this goal we identify first the normalizing factor equivalent to the maximum value of the technical profit inefficiencies, which in this case corresponds to firm K, i.e.,
= $39. This normalization factor is reported at the bottom of C5. Finally, the normalized decomposition Equation(15)
(15)
(15) is presented in the last three columns. These terms are obtained by dividing the preceding three columns by the common normalization factor. Comparing the normalized values of the traditional and standard reverse approaches we see that the values of the traditional approach are unbounded and harder to interpret. In , for firm K, normalized profit inefficiency is 3.25, while its technical and allocative components are 0.833 and 2.417, respectively.Footnote10 Also, these values are driven by the choice of technical inefficiency measure, which is at odds with reality when managers are interested in gaining profit efficiency. In the standard reverse approach, normalizing by the upper bound of the technical profit inefficiencies results in a well-defined valuation of the inefficiencies with respect to the worse technical behavior in economic terms, which provides a suitable interpretation. In this example, normalized profit inefficiency is 1, and since firm K is projected to the profit maximizing benchmark, its technical and allocative components are 1 and 0, respectively.
5. The standard reverse cost inefficiency decompositions
The decomposition of cost inefficiency using the reverse approach, monetary and normalized, follows the same philosophy as the above profit inefficiency decompositions. However, the analysis differs in four basic dimensions. The first one is that the analysis is performed in terms of the input set, rather than the technology. The second one is that only market input prices are required. The third one is that the efficient frontier is defined in terms of the input values. And the last one is that considering cost inefficiency instead of profit inefficiency requires to adapt the rules of action. In this case, following the methodology introduced above for the profit model means that, for each firm, an initial linear program will identify its input-efficient projection, by maximizing the price of its input slacks. Then, based on the calculated optimal input slacks for each firm of our sample, we define a new input-oriented technical inefficiency measure, that is crucial for recovering the associated reverse cost inefficiency decomposition as well as its normalized version. As could be expected, the last-mentioned reverse decompositions have also the same four desirable properties introduced for the profit case.
We start considering a VRS input production possibility set generated by the finite sample of firms Last set is based on the observed output quantities
which is the reference non-zero N-dimensional output vector of the unit under evaluation. A common vector of market input prices
is known, delivering the positive input-cost
of each firm j. The minimum cost of producing
is defined as
The cost inefficiency of firm
is the cost excess between observed cost and minimum cost:
For the same reason argued in the profit case, we assume that there exists at least one inefficient firm. To decompose cost inefficiency we first need to find a projection
of firm
that satisfies
or, equivalently, with non-negative input-slacks,
Last equality gives rise to the next one, simply by obtaining the market value of each of its three elements,
which in turn can be reordered to give
Based on this result, we are going to formulate the standard reverse cost inefficiency decomposition as an equality, relating the cost inefficiency of firms
and its projection
as follows:
(17)
(17)
Equality Equation(17)(17)
(17) decomposes cost inefficiency in the sum of two terms, which we call as technical cost inefficiency
and allocative cost inefficiency
Next, to implement the reverse approach we mirror the profit case, see model Equation(11)
(11)
(11) , and formulate the following input weighted additive model that allows calculating technical cost inefficiency
:
(18)
(18)
This model identifies the projection with the lowest feasible cost inefficiency or, alternatively, with the minimum allocative inefficiency with respect to minimum cost If model Equation(18)
(18)
(18) yields
is an efficient firm of
and, conversely, if
we are facing an input inefficient firm.
To obtain the normalized cost inefficiency decomposition, we express in Equation(17)
(17)
(17) as the product of its input technical inefficiency score, that needs to be defined, times the resulting normalizing factor. The approach we propose is simple, like in the profit case, and consists in identifying first the maximum value across the optimal objective function values associated with all J firms in our sample:
(19)
(19)
Again, the existence of at least one inefficient firm guarantees that is positive, as expressed in Equation(19)
(19)
(19) . Dividing each objective function value by
we obtain a pure number that corresponds to the input-oriented technical inefficiency measure, taking values in the interval [0,1]:
(20)
(20)
As in the profit case, it can be proven that satisfies the four properties that qualifies it as a true technical inefficiency measure. Coming back to equality Equation(17)
(17)
(17) and introducing the just defined inefficiency measure, we get the next standard reverse cost inefficiency decomposition,
(21)
(21)
It is interesting to realize that constitutes a normalizing factor due to its units of measurement, and which that, once again, is common to all firms. Therefore, we get the next reverse normalized cost inefficiency decomposition:
(22)
(22)
6. The standard reverse revenue inefficiency decompositions
The standard reverse revenue inefficiency decomposition is analogous to its cost inefficiency counterpart but considering an output-orientation. Hence, in what follows, we present the main concepts and results related to the monetary and normalized decompositions.
In this case we start considering a VRS production possibility set generated by a finite sample of firms belonging to the output set Last set is based on the observed input quantities,
which is the reference non-zero M-dimensional input vector of the unit under evaluation. A common vector of market output prices,
is known and applies to all firms of
delivering the output-revenue
generated by each firm
The maximum revenue is achieved in at least one firm of our finite sample and is denoted as
Again, as in the two preceding cases, we assume that at least one firm of our sample is inefficient. The revenue inefficiency of firm
is defined as
To decompose revenue inefficiency one needs to find, for each firm
an efficient projection belonging to the frontier of
that satisfies
with
This results in the following reverse revenue inefficiency decomposition defined as an equality, relating the revenue inefficiency of firm
and that of its projection
(23)
(23)
As before, equality Equation(23)(23)
(23) decomposes revenue inefficiency in the sum of technical revenue inefficiency
and allocative revenue inefficiency
Moreover, the projection
should bring the greatest possible revenue gains—or, equivalently, the smallest possible revenue inefficiency with respect to maximum profit
meaning that we seek to maximize the market value of the output-slacks,
The following model calculates
under this criterion:
(24)
(24)
If the optimal solution of Equation(24)(24)
(24) equals
is an efficient firm of
while
signals that it is inefficient. Since we are dealing with a finite sample of firms, and knowing that at least one of them is inefficient, we can calculate the maximum of the objective function value over
and call it
(25)
(25)
Consequently, we propose the following output-oriented technical inefficiency measure, taking values in the interval [0,1]:
(26)
(26)
By reproducing the arguments shown before, it can be proved that is a true technical inefficiency measure over the entire VRS production possibility set, since it satisfies the four conditions enounced before. Using this measure, we decompose revenue inefficiency as follows:
(27)
(27)
which constitutes the reverse revenue inefficiency decomposition for firm
in two components: the technical revenue inefficiency and the allocative revenue inefficiency. Again, let us divide each term of Equation(27)
(27)
(27) by
obtaining the next reverse normalized revenue inefficiency decomposition:
(28)
(28)
7. Conclusions
We develop a new methodology to decompose economic inefficiency that improves the traditional approach, which resorts to duality theory and Fenchel-Mahler inequalities. Our approach is based on the so-called reverse approach suggested by Bogetoft and Hougaard (Citation2003) and Bogetoft et al. (Citation2006), which favours economic gains over technical improvements associated with a specific inefficiency measure. This is the first time that this paradigm is developed for each of the three possible decompositions of economic inefficiency: profit, cost and revenue. Our new approach presents some relevant novelties that deserve to be highlighted. Our first aim is to identify the best possible economic projection for each firm, i.e., the projection that brings the greatest economic gains given market prices, or, equivalently, that results in the smallest possible allocative inefficiency. Interestingly, our approach can be rendered operational through the weighted additive approach introduced by Lovell and Pastor (Citation1995), where the weights are the input and output prices. Based on this model, we can deduce a new graph technical inefficiency measure that captures the essence of the standard reverse approach. Then, inspired by the general direct approach of Pastor et al. (Citation2022), economic inefficiency can be decomposed relying on an equality. The two components of the decomposition correspond to the new graph technical inefficiency measure plus the allocative efficiency of the projected benchmark. The decomposition can be expressed in monetary and normalized terms by defining a specific normalization factor—yielding a normalized technical inefficiency measure that satisfies a customary set of desirable properties. The normalized profit inefficiency decomposition is unit’s invariant, as its traditional counterparts, and easier to interpret numerically.
Disclosure statement
The authors report there are no competing interests to declare.
Additional information
Funding
Notes
1 See Asmild et al. (Citation2013) for an early application to the Canadian banking industry of what Bogetoft and Hougard (2003) called ‘rational inefficiencies’.
2 The restriction that guarantees that T is a VRS technology, is called the convexity constraint, see Banker et al. (Citation1984).
3 If we fix and
we then get the original additive model (Charnes et al., Citation1985). Depending on the weights, we obtain other variants such as the Range-Adjusted Measure (RAM), the Measure of Inefficiency Proportions (MIP), or the Bounded-Adjusted measure (BAM), as discussed in the Introduction. See chapter 6 in Pastor et al. (Citation2022).
4 Model Equation(11)(11)
(11) measuring technical profit inefficiency can be related to the model proposed by Ruiz and Sirvent (Citation2011) searching for the maximum profit that a firm can attain by operating in a technically efficient manner.
5 Directly applying model Equation(11)(11)
(11) to the traditional decomposition of profit inefficiency presented in Equation(7)
(7)
(7) implies that the normalization factor is equal to one. This results in the monetary decomposition of profit inefficiency shown in Equation(10)
(10)
(10) .
6 As shown above, any firm with is an efficient firm.
7 The notation means that although the value of the normalized profit inefficiency corresponds to a pure number, its value is influenced by the numerical values of the market prices. Particularly, if we would consider different market prices
that are not proportional to the initial ones
the resulting normalized profit inefficiency and, consequently, the allocative inefficiency, would yield different values.
8 See Prieto and Zofío (Citation2001) for an early application of the RAM measure to study the effectiveness in the provision of public infrastructure and equipment in Spanish municipalities.
9 We identify with an asterisk the only projection point that does not belong to the sample, (8,11), but conforms the VRS-DEA technology Equation(1)(1)
(1) and, more precisely, the strongly efficient frontier, being a convex combination of firms A and B.
10 The unboundedness and lack of interpretability of the traditional profit inefficiency values is a common drawback of all the decompositions surveyed in the Introduction.
References
- Aparicio, J., Pastor, J. T., & Vidal, F. (2016). The weighted additive distance function. European Journal of Operational Research, 254(1), 338–346. https://doi.org/10.1016/j.ejor.2016.04.006
- Aparicio, J., Ortiz, L., & Pastor, J. T. (2017). Measuring and decomposing profit inefficiency through the Slacks-Based Measure. European Journal of Operational Research, 260(2), 650–654. https://doi.org/10.1016/j.ejor.2016.12.038
- Asmild, M., Bogetoft, P., & Hougaard, J. L. (2013). Rationalising inefficiency: Staff utilisation in branches of a large Canadian bank. Omega, 41(1), 80–87. https://doi.org/10.1016/j.omega.2011.01.011
- Banker, R. D.,Charnes, A., &Cooper, W. W. (1984). Some Models for Estimating Technical and Scale Inefficiencies in Data Envelopment Analysis. Management Science, 30(9), 1078–1092. https://doi.org/10.1287/mnsc.30.9.1078
- Bogetoft, P., & Hougaard, J. L. (2003). Rational inefficiencies. Journal of Productivity Analysis, 20(3), 243–271. https://doi.org/10.1023/A:1027347616038
- Bogetoft, P., Färe, R., & Obel, B. (2006). Allocative efficiency of technically inefficient production units. European Journal of Operational Research, 168(2), 450–462. https://doi.org/10.1016/j.ejor.2004.05.010
- Chambers, R. G., Chung, Y., & Färe, R. (1996). Benefit and distance functions. Journal of Economic Theory, 70(2), 407–419. https://doi.org/10.1006/jeth.1996.0096
- Chambers, R. G., Chung, Y., & Färe, R. (1998). Profit, directional distance functions, and Nerlovian efficiency. Journal of Optimization Theory and Applications, 98(2), 351–364. https://doi.org/10.1023/A:1022637501082
- Charnes, A., Cooper, W. W., Golany, B., Seiford, L., & Stutz, J. (1985). Foundations of data envelopment analysis for Pareto-Koopmans efficient empirical production functions. Journal of Econometrics, 30(1–2), 91–107. https://doi.org/10.1016/0304-4076(85)90133-2
- Cooper, W. W., Park, K. S., & Pastor, J. T. (1999). RAM: A range adjusted measure of inefficiency for use with additive models, and relations to other models and measures in DEA. Journal of Productivity Analysis, 11(1), 5–42. https://doi.org/10.1023/A:1007701304281
- Cooper, W. W., Pastor, J. T., Aparicio, J., & Borras, F. (2011a). Decomposing profit inefficiency in DEA through the weighted additive model. European Journal of Operational Research, 212(2), 411–416. https://doi.org/10.1016/j.ejor.2011.01.054
- Cooper, W. W., Pastor, J. T., Borras, F., Aparicio, J., & Pastor, D. (2011b). BAM: A bounded adjusted measure of efficiency for use with bounded additive models. Journal of Productivity Analysis, 35(2), 85–94. https://doi.org/10.1007/s11123-010-0190-2
- Färe, R., & Lovell, C. A. K. (1978). Measuring the technical efficiency of production. Journal of Economic Theory 19(1), 150–162. https://doi.org/10.1016/0022-0531(78)90060-1
- Halická, M., & Trnovská, M. (2018). The Russell measure model: Computational aspects, duality, and profit efficiency. European Journal of Operational Research, 268(1), 386–397. https://doi.org/10.1016/j.ejor.2018.01.012
- Halická, M., & Trnovská, M. (2021). A unified approach to non-radial graph models in data envelopment analysis: Common features, geometry, and duality. European Journal of Operational Research, 289(2), 611–627. https://doi.org/10.1016/j.ejor.2020.07.019
- Koopmans, T. C. (1951). Analysis of production as an efficient combination of activ- ities. In T. C. Koopmans (Ed.), Activity analysis of production and allocation. John Wiley.
- Lovell, C. A. K., & Pastor, J. T. (1995). Units invariant and translation invariant DEA models. Operations Research Letters, 18(3), 147–151. https://doi.org/10.1016/0167-6377(95)00044-5
- Luenberger, D. G. (1992). New optimality principles for economic efficiency and equilibrium. Journal of Optimization Theory and Applications, 75(2), 221–264. https://doi.org/10.1007/BF00941466
- Pastor, J. T., Aparicio, J., & Zofío, J. L. (2022). Benchmarking Economic Efficiency: Technical and Allocative Fundamentals. International Series in Operations Research and Management Science., Volume 315 Springer Verlag.
- Pastor, J. T., Lovell, C. A., & Aparicio, J. (2012). Families of linear efficiency programs based on Debreu’s loss function. Journal of Productivity Analysis, 38(2), 109–120. https://doi.org/10.1007/s11123-011-0216-4
- Pastor, J. T., Ruiz, J. L., & Sirvent, I. (1999). An enhanced DEA Russell graph efficiency measure. European Journal of Operational Research, 115(3), 596–607. https://doi.org/10.1016/S0377-2217(98)00098-8
- Prieto, A. M., & Zofío, J. L. (2001). Evaluating effectiveness in public provision of infrastructure and equipment: The case of Spanish municipalities. Journal of Productivity Analysis, 15(1), 41–58. https://doi.org/10.1023/A:1026595807015
- Ruiz, J. L., & Sirvent, I. (2011). A DEA approach to derive individual lower and upper bounds for the technical and allocative components of the overall profit efficiency. Journal of the Operational Research Society, 62(11), 1907–1916. https://doi.org/10.1057/jors.2010.140
- Sueyoshi, T., & Sekitani, K. (2009). An occurrence of multiple projections in DEA-based measurement of technical efficiency: Theoretical comparison among DEA models from desirable properties. European Journal of Operational Research, 196(2), 764–794. https://doi.org/10.1016/j.ejor.2008.01.045
- Tone, K. (2001). A slacks-based measure of efficiency in data envelopment analysis. European Journal of Operational Research, 130(3), 498–509. https://doi.org/10.1016/S0377-2217(99)00407-5