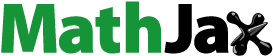
Abstract
Understanding the spatial and temporal distribution of Bovine anaplasmosis is crucial for identifying areas of high prevalence for targeted disease control. This research was aimed at modelling and mapping the B. anaplasmosis potential distribution, and identify hotspots as well as significant variables explaining the occurrence of the disease. The Getis Ord Gi* statistic for Hotspot analysis was used as well as MaxEnt ecological niche modelling. The effects of time, land-use, and agro-ecological regions on B. anaplasmosis occurrence were tested using Analysis of Variance (ANOVA). Results showed that several districts in Zimbabwe are suitable for the occurence of the disease for example Binga, Seke, Buhera, Kwekwe, Gweru, Mhondoro, Chegutu, Sanyati, and in the North: Mbire, Muzarabani, Mt Darwin, Shamva, Bindura, Zvimba and Makonde. Morbidity and mortality hotspots were detected in Gokwe-south, Kwekwe, and Chirumhanzu districts. Binga, Gokwe-south, Gutu, Hurungwe, Mazoe, Nkayi, Shamva, and Kwekwe districts also experienced high disease incidences. Temperature seasonality, precipitation seasonality, mean diurnal range, and isothermality were the most important variables in explaining 93% of B. anaplasmosis distribution. Unlike land-use and agro-ecological regions, time (months) had a significant effect on B. anaplasmosis occurrence with July and September having significantly (p < 0.05) higher cases and deaths than the rest of the months. The results of this study provide insights into the management strategies and control of B. anaplasmosis in Zimbabwe. It is thus concluded that geo-spatial techniques, combined with ecological niche modelling can provide useful insights into disease prevalence and distribution and hence can contribute to effective management and control of B. anaplasmosis in Zimbabwe.
1. Introduction
Tick-borne diseases (TBDs) are common in livestock, especially in poor farming communities characterized by ineffective management systems (Ostfeld and Brunner Citation2015). The most prevalent diseases are anaplasmosis (Gall-sickness), babesiosis (Red-water), ehrlichiosis (formerly cowdriosis: heartwater), and theileriosis (January disease) (De Waal Citation2000). These diseases affect ∼80% of the world’s cattle population and cost between $13.9 and $18 billion per year in acaricides and vaccine purchases and deaths (Demessie and Derso Citation2015). TBDs are a major concern in Zimbabwe where cattle husbandry is one of the most common agricultural practices (Chaminuka et al. Citation2014). According to the country’s Central Veterinary Statistics of 2015, TBDs accounted for 60% of all livestock deaths in Zimbabwe. Cattle are a perennial source of income, providing beef and milk (Guerrini et al. Citation2019). In addition, cattle provide draught power for communal farmers; manure; transport; and are an important component of dowry payment (Chaminuka et al. Citation2014; Sungirai et al. Citation2016). However, TBDs cause high cattle morbidity and mortality, reduced growth rate, reduced milk production, and low fertility in cattle (Rashid et al. Citation2019; Shekede et al. Citation2021). Therefore, their occurrence impacts negatively on national agricultural production as well as hindering access to profitable foreign beef markets (Guerrini et al. Citation2019).
TBDs are mostly found in tropical and subtropical regions around the world (Southern Europe, Australia, South and Central America, Asia, and Africa) where associated tick vectors thrive, with a sporadic distribution in temperate climates (Randolph Citation2008). Bovine anaplasmosis, caused by the rickettsia Anaplasma marginale, has a worldwide distribution (Rodríguez et al. Citation2009). The rickesttsial parasites A. marginale and Anaplasma centrale cause anaplasmosis, a tick-borne non-contagious haemolytic disease that affects both domestic and wild ruminants (Johnson et al. Citation2016). These parasites are transmitted biologically by ticks, mechanically by biting flies, blood-contaminated fomites, and from cow to calf by transplacental transmission (Aubry and Geale Citation2011). Once the host is infected, the parasites cause severe anaemia by infecting red blood cells (Johnson et al. Citation2016). The five tick species that have been reported to transmit A. marginale in southern Africa are Rhipicephalus decoloratus, Rhipicephalus evertsi evertsi, Rhipicephalus microplus, Rhipicephalus simus, and Hyalomma marginatum rufipes (De Waal Citation2000). Compared to other TBDs, anaplasmosis remains one of the most serious causes of high cattle morbidity and mortality in developing countries (Randolph Citation2008). For example, 14 countries in Africa reported anaplasmosis outbreaks to the African Union-Interafrican Bureau for Animal Resources in 2011 (AU-IBAR Citation2011). During the same year, 983 outbreaks were recorded involving 872 deaths with the highest number of outbreaks reported by Zimbabwe (533), followed by Zambia (100) and Kenya (88) (AU-IBAR Citation2011). Anaplasmosis is also on the list of diseases notifiable to the World Organization for Animal Health. Although disease eradicating programs have been successfully achieved in high income and most middle income countries, anaplasmosis remains a major challenge in low income countries (Rashid et al. Citation2019; Shekede et al. Citation2021). In Zimbabwe, the high mortality rates are linked to low dipping frequency, common in communal areas where farmers rely on government support for dipping chemicals (Shekede et al. Citation2021).
Although knowledge of the geographic distribution and suitable habitats of tick species is crucial for the management of TBDs, detailed data on species’ actual and potential distribution as well as anaplasmosis spatial distribution is usually lacking (Tesfamariam et al. Citation2022) as collecting such data is costly and labour-intensive. Furthermore, there is still limited knowledge concerning the disease drivers owing to the global and continental scales at which previous studies were carried out (Buhnerkempe et al. Citation2014; Hammac et al. Citation2014). In contrast, local studies have strongly relied on traditional field-based surveys which are not only costly but also inefficient in describing the precise spatial distribution of the disease (Ostfeld and Brunner Citation2015). For instance, in Zimbabwe, traditional mapping methods as used by Ndebele et al. (Citation2007) and Sungirai et al. (Citation2016) lack spatial accuracy, which results in inaccurate mapping of disease areal distribution. Advances in geospatial technologies and the growing availability of modelling approaches have improved disease mapping and spatial modelling by identifying specific areas of disease occurrence (Yang et al. Citation2005; Phillips et al. Citation2006; Beale et al. Citation2008; Kumar and Stohlgren Citation2009; Johnson et al. Citation2016) thereby offering spatially explicit disease management options.
In Zimbabwe, despite the reported widespread distribution and severe cattle losses incurred to date, effective control of anaplasmosis has not been achieved (Nhokwara et al. Citation2023). Mapping the spatial distribution, including hotspots and coldspots is essential for the effective management of the anaplasmosis. Moreover, an understanding of the environmental conditions suitable for disease prevalence in space and time provides the fundamental basis for managing the disease (Kumar and Stohlgren Citation2009; Kumar et al. Citation2016; Tagwireyi et al. Citation2022). Understanding the spatial distribution of anaplasmosis is important in identifying areas of high disease prevalence which helps in effective disease control thereby reducing economic and social losses attributed to the disease (Herrmann et al. Citation2014; Tagwireyi et al. Citation2022). Knowledge of disease hotspots is important for optimal resource allocation through targeted preventive and control strategies to areas with the greatest need (Shekede et al. Citation2021; Shaweno et al. Citation2021; Ren et al. Citation2022). This is vital in countries with limited resources, such as Zimbabwe, where dipping chemicals are usually insufficient to cater for the whole country (Mashoko et al. Citation2007). Agriculture is the backbone of Zimbabwe’s economy and is the main source of income for most Zimbabwe’s subsistence and commercial farmers. In Zimbabwe, the cattle population is estimated to be between 4 and 5 million (Mashoko et al. Citation2007) with 89% of the herd found in communal areas (Mavedzenge et al. Citation2008). However, the cattle herd is declining as a result of diseases such as foot-and-mouth, theileriosis, anthrax, heartwater, redwater and B. anaplasmosis amongst others (Sungirai et al. Citation2016; Nhokwara et al. Citation2023). Therefore, this study aimed at using geospatial technologies in conjunction with ecological niche modelling to examine the spatial distribution of anaplasmosis in Zimbabwe, its hotspots and frequency of occurrence by using presence-only data and bio-climatic variables.
2. Materials and methods
2.1. Study area
The study was conducted in Zimbabwe, a semi-arid country located in Southern Africa within the tropics at 15°30″ and 22°30″S (latitude) 25°00″ and 33°10″E (longitude) (). Zimbabwe is a landlocked country covering 386,847 km2 of land and 3,910 km2 of water (Kuri et al. Citation2014). Temperature ranges from an average low of 15 °C in winter to around 24 °C in summer. The climate varies with altitude, with the eastern highlands experiencing the lowest mean annual temperature of 18 °C while the northern and southern low lying areas experience the highest temperatures of around 23 °C (Mupangwa et al. Citation2011). The climate is characterized by a cool dry season from May to August, hot and dry season between August and October, and a hot and wet season stretching from November to April (Mupangwa et al. Citation2011). Precipitation averages 750–900 mm in the Highveld and 350 mm in the Lowveld (Kuri et al. Citation2014). Elevation is highest in the eastern parts of the country (>2500 m above sea level) and lowest in the southern and northern parts of the country where it is <300 m above sea level. Main land use types vary from forest plantations, crop farming to animal husbandry. shows that the greater proportion of the cattle population is found in drier regions of the country, i.e. Region IV (450–650 mm) and V (<450 mm). However, the cattle herd is declining as a result of diseases like foot-and-mouth, theileriosis, anthrax, heartwater, redwater, and B. anaplasmosis amongst others (Sungirai et al. Citation2016; Nhokwara et al. Citation2023).
Table 1. Cattle distribution by province and agro-ecological regions.
Movement of tick infested livestock is the main driver of TBD spread from one area to another. Thus, TBDs are managed through rigorous short-interval dipping, disease surveillance, monitoring and reporting as well as strict enforcement of livestock movement control regulations (Peter et al. Citation1998).
2.2. Bovine anaplasmosis data
Spatially referenced confirmed B. anaplasmosis cases (morbidity) and deaths (mortality) data for the period 2010–2011 were obtained from the Department of Veterinary Services (DVS) within the Ministry of Agriculture, Mechanization and Irrigation Development, Zimbabwe. Monthly, all district veterinary offices and animal health centres submit animal disease reports to the provincial veterinary offices where the data is collated. The collated disease information from all the provinces is then submitted to the Information Management Unit (IMU) in the DVS where it is electronically stored. Considering that all provinces are required to submit their monthly disease reports to the IMU, disease data obtained from the IMU in the DVS was considered not to be significantly biased with respect to reporting and highly likely to be representative of the disease situation in the country.
The dataset consisted of 107 B. anaplasmosis occurrence point locations (longitude and latitude) of cases and deaths. The confirmed B. anaplasmosis cases and deaths were recorded from 2010 to 2011 covering most parts of Zimbabwe. The B. anaplasmosis occurrence map shows the presence only location points of the disease covering ∼46% (27/59) of the country’s districts (). The attributes associated with each anaplasmosis case included year and month, cattle owner’s name, province, district, land-use, nearest dip tank and its grid reference, point location (longitude and latitude), sex, and age. Bovine anaplasmosis cases were based on case history, clinical signs, and post-mortem findings by field veterinarians and laboratory confirmed through microscopy. An anaplasmosis case was defined as an animal presenting with signs of anorexia, fever, jaundice (yellow discolouration of mucous membranes) and progressive anaemia (pale mucous membranes), and one or more of the other signs, such as difficulty breathing, rapid pulse, brown urine, loss of coordination, aggression, and decreased milk production. A death case was defined based on post-mortem findings of a markedly anaemic and jaundiced carcass, thin and watery blood, an enlarged spleen, and a distended gall bladder containing thick brown or green bile. Anaplasmosis confirmation was based on a demonstration of the parasites on Giemsa-stained blood smears
The World Organisation for Animal Health (2021) observes that TBD cases captured by the DVS indicate a very high level of under reporting of TBD cases despite the existence of standardized protocols for collection, verification, and publishing of official animal health information. This is attributable to the fact that cases diagnosed by private veterinary practitioners and those attended to by farmers are rarely recorded in official databases (Department of Veterinary Services Citation2022). Furthermore, not all recorded cases have laboratory confirmation due to inadequate sampling equipment and microscopic diagnostic skills, lack of functional microscopes, and sample preparation reagents at district and sub-district offices (Department of Veterinary Services Citation2022).
2.3. Bioclimatic variables
The variables used in modelling B. anaplasmosis suitability included rainfall, elevation, vegetation cover, and temperature (). The bio-climatic variables were selected based on their influence on the survival, reproduction, and questing rates of the tick vectors of anaplasmosis (Hapunik et al. Citation2011). They provide favourable conditions for the life cycle of the disease tick vectors and thus have a preferably high predictive power in modelling the suitable habitats. The different temperature variables account for temperature fluctuations and the sensitivity of the vector to extreme temperatures. Precipitation provides moisture for vegetation growth which is required by the tick vectors for their survival (Herrmann and Gern Citation2010).
Table 2. Variables used in modelling of Bovine anaplasmosis suitability.
2.4. Effects of land-use and agro-ecological region on B. anaplasmosis occurrence
The analysis of variance (ANOVA) implemented through the MASS package in R (Ripley et al. Citation2013) was used to test the effects of time, land-use, and agro-ecological regions on B. anaplasmosis morbidity and mortality. Furthermore, the Tukey’s Honest Significance difference (HSD) test was used to test which means were statistically different after ANOVA F-test showed a significant difference in means between the groups (McHugh Citation2011).
Agro-ecological zones (AEZs) provide a framework for agricultural production and thus determine land use patterns across Zimbabwe. Annual rainfall and agricultural production potential progressively decrease from AEZ 1 to AEZ 5 (). AEZ I receive the highest amounts of rainfall and is the most productive in terms of crop farming while AEZ V receives the least amount of rainfall and is not suitable for rain-fed agriculture. In this regard, extensive cattle production and game ranching are the predominant activities in AEZ V. AEZ 1 occupies 1.5% of the country and predominates the eastern parts of the country which receives the highest amount of rainfall exceeding 1000 mm/year on average. AEZ II is divided into IIa and IIb and occupies 5.7 and 9.3% of central, north eastern, and eastern parts of the country. The main distinguishing feature of the two sub-regions is that AEZ IIb experiences more dry spells than AEZ IIa. AEZ III is located in the central, western, southern, and eastern parts of the country and covers over 16.2% of the country. In terms of area, AEZ IV is the second most extensive region and occupies 29.1% of the country, dominating the central and western parts of the country and borders Regions III and V. The most extensive of the AEZ is AEZ V with AEZ Va and AEZ Vb occupying 29.4 and 8.8%, respectively. AEZ V is the driest in the country and predominates the major river valleys of the southern, western, and northern fringes of the country whilst relative to AEZ Va, AEZ Vb is so dry that it cannot sustain any form of rainfed agriculture and is restricted to the southern-most parts of the country.
Figure 3. Agro-ecological regions as delineated by Manatsa et al. (Citation2020).

According to Moyo (Citation2011), Zimbabwe has multi-form land tenure system comprising freehold, lease, permit, communal, and state land). Communal areas have de facto rights delegated to communities (including chiefs), under the oversight of rural district councils. The Communal Areas cover 42% of Zimbabwe’s land where about 70% of the country’s population resides. On the other hand, large scale and small-scale commercial farmers occupy about 32% of the country’s land under individual land ownership which guarantees exclusive property rights and full control and responsibility over the land and infrastructure. Resettlement Areas cover 10% of the country and were meant to de-congest the communal areas. A further 15% of the country’s land is gazetted as protected forests (2%) and national parks (13%) (Moyo Citation2011).
2.5. Spatial distribution of anaplasmosis
The spatial and temporal distribution of morbidity and mortality was mapped per district. The frequency distribution showing the rate of the disease occurrence over the two year period was classified in ArcMap 10.8. The frequency values were grouped into five distinct classes using the equal interval classification.
In addition, the Gertis Ord GI* statistic (EquationEquation 1(1)
(1) ), calculated using ArcMap 10.8 was used to determine whether B. anaplasmosis morbidity and mortality rates significantly cluster in space across districts of Zimbabwe. A cluster is an aggregation of points in space where the distance between two points is less than the distance between any other point in the cluster and any point not in it (Jacquez Citation2000; Omodior et al. Citation2019). A cluster of high values surrounded by high values is called a hotspot (Jacquez Citation2000; Omodior et al. Citation2019). When features with high values are surrounded by features with high values a hotspot is statistically significant whereas when features with low values are surrounded by features with low values a coldspot is statistically significant (Jacquez Citation2000; ESRI. Citation2014; Omodior et al. Citation2019). When it is applied to a disease, clustering can be defined as an excess of reported cases in time, space, or both space and time (hotspots), or areas with fewer than expected cases (coldspots) (Jacquez Citation1996). The Gertis Ord Gi* is calculated using the following equation (ESRI. Citation2014):
(1)
(1)
Given that:
and
(2)
(2)
Where:
xj is the attribute value for feature j,
wij is the spatial weight between features i and j,
n is the total number of features,
is the mean and s is the variance.
2.6. Bovine anaplasmosis variable selection for modelling
When using prediction models many variables can be used. However, some of the variables can be collinearly related. Variable selection therefore encompasses selecting variables to include in modelling, among the many, by removing those that are redundant (Ratner Citation2010). The selection determines a set of variables that will provide the best fit for the model so that accurate predictions can be made (Ratner Citation2010). Hence, to reduce data duplication and redundancy a cut-off correlation coefficient of 0.90 was used in variable selection (). The final variables selected for modelling anaplasmosis distribution were annual mean temperature (Bio1), mean diurnal range (Bio2), isothermality (Bio3), temperature seasonality (Bio4), precipitation of driest month (Bio 14), precipitation seasonality (Bio15), precipitation of wettest quarter (Bio16), precipitation of warmest quarter (Bio17), precipitation of driest quarter (Bio18), precipitation of coldest quarter (Bio19) and elevation (Elev) ().
Table 3. Bioclimatic variables correlation results for variable selection.
The selected bio-climatic variables showed that areas in the northern, western, and southern parts of Zimbabwe have the highest mean annual temperature whereas eastern and central areas have low annual mean temperature. The highest mean diurnal range is experienced in the western whilst the eastern has the lowest. North-eastern and south-eastern regions have high isothermality as compared to the north-western region which has low. High temperature seasonality is in the western and south-western and relatively low in the north-eastern. Precipitation of the driest month and precipitation of coldest quarter is high in the eastern and south-eastern whilst low in all other parts of the country. A low precipitation seasonality and precipitation of wettest quarter is experienced in the southern and a high in the northern. Precipitation in the warmest quarter is high in the eastern and low in the northern. There is a high elevation in the eastern and central and a low in the northern and southern.
2.7. Bovine anaplasmosis suitability modelling
Ecological niche modelling was carried out using MaxEnt software (Phillips et al. Citation2006). The output was the spatial distribution of B. anaplasmosis in Zimbabwe. MaxEnt uses a machine-learning technique called maximum entropy modelling. Of many species’ distribution algorithms, MaxEnt has proved powerful when modelling rare species with narrow ranges and available scarce presence-only occurrence data (Phillips et al. Citation2006). Several studies have successfully used MaxEnt, for example, Pearson et al. (Citation2007), Wisz et al. (Citation2008), Baldwin (Citation2009), Rebelo and Jones (Citation2010), Sardà-Palomera et al. (Citation2012), Marcer et al. (Citation2013). MaxEnt has also been used for modelling of TBDs (Raghavan et al. Citation2016; Sage et al. Citation2017; Zannou et al. Citation2021). As highlighted by Phillips et al. (Citation2006), the advantages of MaxEnt include: (1) the model requires only presence data, together with environmental information for the whole study area; (2) MaxEnt can utilize both continuous and categorical data, and can incorporate interactions between different variables; (3) efficient deterministic algorithms have been developed that converge to the optimal (maximum entropy) probability distribution; (4) the MaxEnt probability distribution has a concise mathematical definition, and is therefore amenable to analysis, and (5) over-fitting can be avoided by using -regularization.
2.8. MaxEnt model evaluation
The area under the curve (AUC) of a receiver operating characteristic curve (ROC) was used for model performance validation as used by: Sage et al. (Citation2017) and Gomes et al. (Citation2018). A model with AUC values from 0.0 to 0.5 is considered poor, 0.6–0.9 useful, and above 0.9 excellent (Fan et al. Citation2006; Jiménez-Valverde et al. Citation2008; Carter et al. Citation2016).
3. Results
3.1. Effects of land-use and agro-ecological region on B. anaplasmosis occurrence
Results of this study show that between 2010 and 2011 a total of 1781 B. anaplasmosis cases and 446 deaths were recorded across the country. Of the reported cases, 1347 were treated during the period under consideration with more females (52%) being affected by the disease than males (48%). Age wise, adult and sub adult cattle (96%) were more susceptible to the disease than calves. ANOVA results showed that time as indicated by the month of the year significantly influenced B. anaplasmosis occurrence cases (F11 = 4.049, p = 0.000) and deaths (F11 = 2.035, p = 0.034) with September experiencing the highest mean number of cases () and deaths (). The mean number of cases and deaths in September were significantly (p < 0.05) different from all the other months except July and October. In contrast, land-use had no significant influence on mean anaplasmosis cases (F11 = 0.605, p = 0.679) and deaths (F11 = 0.565, p = 0.690). However, communal and resettlement areas recorded relatively non-significant (p > 0.05) higher mean numbers of both parameters compared to the other areas (). Similar findings were noted for cases (F11 = 0.537, p = 0.748) and deaths (F11 = 0.720, p = 0.573) according to agro-ecological regions (). However, agro-ecological region III recorded a relatively non-significant (p > 0.05) higher mean number of cases compared to others, and agro-ecological regions I, II, and IV had relatively non-significant (p > 0.05) higher mean numbers of deaths compared to others.
Figure 5. (a) Variations in Bovine anaplasmosis CASES by month and (b) variations in B. anaplasmosis DEATHS by month.

3.2. Spatial variations in the occurrence ofB. anaplasmosis
The spatial distribution of B. anaplasmosis showed that Kwekwe district had the highest frequency (18–50 cases) while five districts (Binga, Gokwe-south, Gutu, Hurungwe, and Mutare) had a frequency of 11–18 cases of the disease during the study period (). Over 85% of the districts (53/59, 89.8%) had a low frequency (≤11) of anaplasmosis cases with most of them being in the western and north-eastern parts of the country. The death frequency pattern of the disease was similar to that of the cases with Kwekwe recording the highest deaths and most districts (>90%) had ≤6 deaths ().
3.3. Hotspot analysis of B. anaplasmosis habitat suitability
Significant hotspots of anaplasmosis cases and deaths were recorded in only three central districts (Gokwe-south, Kwekwe, and Mutare) of the country. The coldspots (less than expected) for cases were observed in several districts (Binga, Chiredzi, Gutu, Chirumhanzu, Bindura, and Hurungwe). Similar to cases hotspots, deaths hotspots were noted in two districts (Kwekwe and Mutare) while the coldspots were located in Gokwe-north, Kariba, Bindura, Chiredzi, Chipinge, and Hurungwe ().
3.4. MaxENT model performance
The partial receiving operating characteristic (partial ROC) (Gomes et al. Citation2018) was used for validating the results by making use of area under curve (AUC). The AUC criteria considered a model with values from 0.0 to 0.6 as inferior, 0.6–0.9 as useful, and >0.9 as excellent following Allouche et al. (Citation2006). The B. anaplasmosis suitability model had an AUC of >0.71 suggesting that the model is acceptable ().
3.5. Bovine anaplasmosis suitability
Bovine anaplasmosis suitability modelling for Zimbabwe showed that 0.2% of Zimbabwe is highly suitable (>0.75 suitability score) for B. anaplasmosis occurrence and this is located in one north-western district of the country—Binga District (). Approximately a quarter (24.7%) of the country is moderately suitable for B. anaplasmosis and it covers mostly central and north-eastern districts while 34.9% is marginally suitable and is spread over districts surrounding the moderately suitable areas. The lowly suitable, covering two-fifths (40.2%) of the country was found to be located in the southern, north-western, and some eastern and northern districts. A total area of 59.8% of Zimbabwe is suitable for the disease occurrence ().
3.6. Bioclimatic variables of importance
Temperature seasonality (46%), precipitation seasonality (22.8%), mean diurnal range (17.6%), and isothermality (6.3%) were the most important predictor variables explaining more than 92% of the disease occurrence (). Overall, these results showed that temperature variables were more important in explaining the B. anaplasmosis disease suitability.
Table 4. Relative contribution of the environmental variables in explaining the spatial variation in modelled Bovine anaplasmosis suitability in Zimbabwe.
3.7 Relationship between environmental variables and B. anaplasmosis suitability
A non-linear relationship between temperature seasonality and B. anaplasmosis suitability, with the suitability increasing with increasing temperature seasonality observed for Zimbabwe (). Thereafter, increasing temperature seasonality (Bio-4) is negatively related to the disease suitability. The response curve () shows a positive relationship between B. anaplasmosis and precipitation seasonality (Bio-15) especially from 85 to 105 mm. There was a nonlinear relationship between B. anaplasmosis and mean diurnal range (Bio-2) () having a positive relationship from 9.5 to 15.2 °C and a negative relationship past this point. summarises the relationship between environmental variables and B. anaplasmosis suitability.
Response curves as estimated from MAXENT showing log response of B. anaplasmosis suitability to environmental predictors. The probability of presence (logistic output) is shown on the y-axis while the range of the environmental predictor is shown on the x-axis (Gomes et al. Citation2018). To prevent the interaction of variables affect the relationship modelled, the response curves are based on univariate models which show one response at a time. Log Linear models are a type of generalized linear model that are used to model multinomial and ordinal data. They are also known as multinomial logit models, and they are used to model relationships between categorical variables (Gomes et al. Citation2018).
4. Discussion and conclusion
4.1. Discussion
The study was aimed at mapping and modelling the spatio-temporal distribution of B. anaplasmosis in Zimbabwe and examine the main variables influencing its distribution. The observed pattern of occurrence showed that districts mostly in the central and north-eastern parts of the country with pockets in the western and northern parts were characterized by relatively higher morbidity and mortality rates due to the disease. Probably this was an indicator of a high probability of occurrence of B. anaplasmosis tick vectors in these areas. The presence of the tick vectors is a key determinant of the spatial distribution of the disease (Farooqi et al. Citation2018).
Findings from the current study suggest that anaplasmosis cases and deaths tend to cluster in Kwekwe, Gokwe-south, Nkayi, Shamva, and Bindura districts. This indicates that B. anaplasmosis is not randomly distributed across the country. Disease clusters are likely to be associated with favourable environmental conditions that support the vector survival. However, cattle density cannot be ruled out and it is likely to be a contributing factor to the disease clusters since cattle have an association with tick distribution (Bariso and Worku Citation2018). This is because cattle species are one of the ideal hosts of ticks (Ostfeld and Brunner Citation2015). The higher the cattle population, the higher the chances of tick survival and this may result in high disease transmission rates (Ostfeld and Brunner Citation2015).
However, despite ticks being attracted to high cattle abundance areas, they are not prevalent in all areas of high cattle abundance because some areas do not offer preferred climatic conditions for the survival of ticks (Bariso and Worku Citation2018). A combination of cattle density and favourable environmental conditions for the ticks most likely explains the presence of anaplasmosis clusters in certain areas and this requires further investigations. In support of the present findings, Shekede et al. (Citation2021) reported tick hotspots to be predominantly located in the northern, north-eastern and southern parts of the country, which also coincides with the anaplasmosis cases and death hotspots identified in this study. Accordingly, identifying hotspots areas enhances knowledge on the implementation of targeted control of B. anaplasmosis through the construction of dip-tanks and allocation of acaricides in areas of high disease prevalence to reduce mortalities. It also helps in the establishment of veterinary service centres near disease hotspots areas so as to enhance early detection and treatment of affected animals.
Bovine anaplasmosis suitability across Zimbabwe showed some areas to be more suitable for the disease than others. The study identified high suitability to be located mostly in the central and north-eastern with some pockets in the western and northern parts and lowly suitable in the southern, north-western, and extreme east districts. The areas of high suitability are characterized by high temperatures and high rainfall which are favourable to the vector life cycle. However, very low temperatures, extremely high temperatures, and severe dry climatic conditions lead to saturation deficit of ticks which results in decreased tick’s habitat suitability (Herrmann and Gern Citation2010; Tagwireyi et al. Citation2022). This may probably result in very low suitability in other areas.
The study also revealed that environmental suitability is primarily important in understanding B. anaplasmosis. Based on the ecology of B. anaplasmosis, areas with conducive conditions for the survival of its vector explain high disease prevalence (Torina et al. Citation2008). The present results demonstrate the value of collecting geo-referenced livestock health data, such as the B. anaplasmosis occurrence data used in modelling and mapping the suitability of the disease. The results can be successfully used to demarcate B. anaplasmosis risk zones for targeted disease control and preventive interventions.
In this study, temperature seasonality, precipitation seasonality, mean diurnal range, and isothermality were found to be significant variables explaining the spatial suitability of anaplasmosis. Temperature and precipitation are crucial in the tick vector life cycle and create a favourable environment for the growth of ticks that transmit the disease (Torina et al. Citation2008; Tagwireyi et al. Citation2022). The vector ticks prefer high temperatures and high rainfall to thrive. The results are consistent with Johnson et al. (Citation2016) who also found the same factors to be relevant for predicting the suitability of the disease at a global scale. These conditions are more related to the tick’s microhabitat as they have a direct effect on the living conditions of ticks (Khalaf et al. Citation2018; Zannou et al. Citation2021; Tagwireyi et al. Citation2022) and are characteristic of the areas found suitable for the disease.
Time (months) was shown to significantly influence B. anaplasmosis occurrence with July, September, and October experiencing the highest occurrence rates. Ticks have higher summer questing activity thereby increasing the rate of disease transmission during that period although occasional outbreaks can be identified during winter (Bhatnagar et al. Citation2015). Lower winter temperatures and humidity are less favourable for tick growth (Bhatnagar et al. Citation2015). However, Hapunik et al. (Citation2011) stated that high winter cases are likely to occur due to global warming, which increases the average temperature in the winter months.
Bovine anaplasmosis occurrence data for two years were used to provide important insights into the clustering of the disease in Zimbabwe. It integrated space and time (months) in one analytical framework to provide new insights into the epidemiology of B. anaplasmosis (Hanzlicek et al. Citation2016). The technique used in this study, Getis-Ord Gi*statistic determines significant clustering. The current study used both bio-climatic variables and B. anaplasmosis occurrence data to model suitability which differs from former studies that used tick infestation data to predict disease suitability (Estrada-Peña et al. Citation2009). The used bio-climatic variables have global spatial coverage and are easily accessible and obtainable for present or future climatic scenarios (Title and Bemmels Citation2018).
The current research is one of the earliest attempts to map the spread of B. anaplasmosis in Zimbabwe. This makes it easier to make inferences for the whole country compared to other studies (e.g. Buhnerkempe et al. Citation2014; Hammac et al. Citation2014) which had a continental and global scale focus. The study helps to gain knowledge about the spatial distribution of the disease in Zimbabwe, which can be used for the proper allocation of resources, to prioritize areas of greatest need and thus maximize disease control. The use of geospatial analysis is one of the main strengths of this study. Geospatial analysis in combination with bio-climatic modelling has been used productively in several studies (see: Yang et al. Citation2005; Baldwin Citation2009; Rebelo and Jones Citation2010; Sardà-Palomera et al. Citation2012; Marcer et al. Citation2013); using location data in modelling and mapping disease distribution and hotspots.
The findings of this study should be viewed in the light of its limitations. Hence, some potential biases of the study need to be considered. The B. anaplasmosis cases and deaths detailed in this study include only those that were reported and confirmed by veterinarians. Many other anaplasmosis cases and deaths could probably have not been reported by farmers. Hence, the reported cases and deaths in this study could be an underestimate of the problem and the reporting bias may influence the accuracy of the study outcomes. Despite this limitation, the study demonstrated clusters of occurrences of the anaplasmosis.
The modelling did not take into account the cattle population density in different parts of the country. The cattle population in Zimbabwe is estimated to vary between 4 and 5 million (Mashoko et al. Citation2007) and the 2020/21 cattle census reported a population of ∼5.5 million (Anonymous 2021). Based on the recent cattle population statistics, the percentage distribution varied from 10.4% for the north-eastern districts to 18.6% for the south-eastern districts. The central districts had a contribution of 18.4%, northern 17.7%, north-western 12.4%, south-western 11.5%, and eastern 11.2%. Cattle density cannot be ruled out and it is likely to be a contributing factor to the observed disease clusters since there is an association between cattle and tick distribution (Worku et al. 2018). This is because cattle are reported to be one of the ideal hosts of ticks (Ostfeld and Brunner Citation2015). The higher the cattle population, the higher the chances of tick survival and this may result in high disease transmission rates (Ostfeld and Brunner Citation2015). The central districts with a relatively higher cattle population were also characterized by clusters of higher disease morbidity and mortality rates. However, despite, a lower contribution percentage, the north-eastern districts were also characterized by clusters of higher disease morbidity and mortality rates.
In addition, the current analysis is also limited by presence only data availability, which covered a period of two years. This makes it difficult to extrapolate for a longer time periods and to make temporal conclusions on the disease behaviour. Different time frames result in distinct disease patterns and rates due to the shifting of seasons and changes in climate (Hanzlicek et al. Citation2016). As such, to gain more insights into the disease patterns, there is a need for long term data collection.
4.2. Conclusion
This study mapped the spatial distribution, hotspots, determined significant bio-climatic variables and suitable areas for B. anaplasmosis across Zimbabwean districts. Results indicated spatial clusters of the disease in several districts in central and northern Zimbabwe with precipitation and temperature explaining more of the disease distribution in suitable areas. The results of this study provide insights into the management strategies and control of B. anaplasmosis in Zimbabwe. It is thus concluded that geo-spatial techniques, combined with ecological niche modelling can provide useful insights into disease prevalence, occurrence, and distribution.
Disclosure statement
No potential conflict of interest was reported by the author(s).
Data availability statement
Data will be made available on request.
Additional information
Funding
References
- Allouche O, Tsoar A, Kadmon R. 2006. Assessing the accuracy of species distribution models: prevalence, kappa and the true skill statistic (TSS). J Appl Ecol. 43(6):1223–1232. doi: 10.1111/j.1365-2664.2006.01214.x.
- Aubry P, Geale DW. 2011. A review of Bovine anaplasmosis. Transbound Emerg Dis. 58(1):1–30. doi: 10.1111/j.1865-1682.2010.01173.x.
- AU-IBAR. 2011. African Union-Inter African Bureau for Animal Resources (2011). Panafrican Animal Health Yearbook 2011. xiii + 90 pp. http://www.au-ibar.org/index.php?option=com_flexicontent&view=items&cid=71&id=109&Itemid=56&lang=en.
- Baldwin RA. 2009. Use of maximum entropy modeling in wildlife research. Entropy. 11(4):854–866. doi: 10.3390/e11040854.
- Beale L, Abellan JJ, Hodgson S, Jarup L. 2008. Methodologic issues and approaches to spatial epidemiology. Environ Health Perspect. 116(8):1105–1110. doi: 10.1289/ehp.10816.
- Bhatnagar CS, Bhardawaj B, Sharma DK, Meena SK. 2015. Incidence of haemoprotozoan diseases in cattle in Southern Rajasthan, India. Int J Curr Microbiol Appl Sci. 4(3):509–514.
- Buhnerkempe MG, Tildesley MJ, Lindström T, Grear DA, Portacci K, Miller RS, LoMBard JE, Werkman M, Keeling MJ, Wennergren U, et al. 2014. The impact of movements and animal density on continental scale cattle disease outbreaks in the United States. PLOS One. 9(3):e91724. doi: 10.1371/journal.pone.0091724.
- Bariso M, Worku Y. 2018. Cattle ticks and tick borne haemoparasite species identification and associated risk factors in two districts of West Arsi Zone, Ethiopia. J Vet Sci Anim Husb. 6(5):501.
- Carter JV, Pan J, Rai SN, Galandiuk S. 2016. ROC-ing along: evaluation and interpretation of receiver operating characteristic curves. Surgery. 159(6):1638–1645. doi: 10.1016/j.surg.2015.12.029.
- Chaminuka P, Groeneveld RA, van Ierland EC. 2014. Reconciling interests concerning wildlife and livestock near conservation areas: a model for analysing alternative land uses. Ecol Econ. 98:29–38. doi: 10.1016/j.ecolecon.2013.12.006.
- De Waal DT. 2000. Anaplasmosis control and diagnosis in South Africa. Ann N Y Acad Sci. 916(1):474–483. doi: 10.1111/j.1749-6632.2000.tb05327.x.
- Demessie Y, Derso S. 2015. Tick borne hemoparasitic diseases of ruminants: a review. Adv Biol Res. 9(4):210–224. doi: 10.5829/idosi.abr.2015.9.4.9516.
- Department of Veterinary Services. 2022. Zimbabwe integrated tick and tick-borne disease control strategy 2022–2030, Government of Zimbabwe.
- ESRI. 2014. An overview of the Mapping Clusters toolset. [accessed 2021 Feb]. https://pro.arcgis.com/en/pro-app/2.8/tool-reference/spatial-statistics/h-how-hot-spot-analysis-getis-ord-gi-spatial-stati.htm.
- Estrada-Peña A, Naranjo V, Acevedo-Whitehouse K, Mangold AJ, Kocan KM, de la Fuente J. 2009. Phylogeographic analysis reveals association of tick-borne pathogen, Anaplasma marginale, MSP1a sequences with ecological traits affecting tick vector performance. BMC Biol. 7(1):1–13. doi: 10.1186/1741-7007-7-57.
- Fan J, Upadhye S, Worster A. 2006. Understanding receiver operating characteristic (ROC) curves. Can J Emergency Med. 8(1):19–20. doi: 10.1017/S1481803500013336.
- Farooqi SH, Ijaz M, Rashid MI, Nabi H, Islam S, Aqib AI, Hussain K, Khan A, Rizvi SNB, Mahmood S, et al. 2018. Molecular epidemiology of Bovine anaplasmosis in Khyber Pakhtunkhwa, Pakistan. Trop Anim Health Prod. 50(7):1591–1598. doi: 10.1007/s11250-018-1599-2.
- Gomes VHF, IJff SD, Raes N, Amaral IL, Salomão RP, de Souza Coelho L, de Almeida Matos FD, Castilho CV, de Andrade Lima Filho D, López DC, et al. 2018. Species distribution modelling: contrasting presence-only models with plot abundance data. Sci Rep. 8(1):1003. doi: 10.1038/s41598-017-18927-1.
- Guerrini L, Pfukenyi DM, Etter E, Bouyer J, Njagu C, Ndhlovu F, Bourgarel M, de Garine-Wichatitsky M, Foggin C, Grosbois V, et al. 2019. Spatial and seasonal patterns of FMD primary outbreaks in cattle in Zimbabwe between 1931 and 2016. Vet Res. 50(1):1–12. doi: 10.1186/s13567-019-0690-7.
- Hammac GK, Pierlé SA, Cheng X, Scoles GA, Brayton KA. 2014. Global transcriptional analysis reveals surface remodeling of Anaplasma marginale in the tick vector. Parasites Vectors. 7(1):1–11. doi: 10.1186/1756-3305-7-193.
- Hanzlicek GA, Raghavan RK, Ganta RR, Anderson GA. 2016. Bayesian space-time patterns and climatic determinants of Bovine anaplasmosis. PLOS One. 11(3):e0151924. doi: 10.1371/journal.pone.0151924.
- Hapunik J, Víchová B, Karboviak G, Wita I, Bogdaszewski M, Petko B. 2011. Wild and farm breeding cervids infections with Anaplasma phagocytophilum. Ann Agric Environ Med. 18(1):73–77. https://agro.icm.edu.pl/agro/element/bwmeta1.element.agro-5e56ff92-db6f-4edb-be3b-7767dd6059bd/c/fulltext899.pdf.
- Herrmann C, Gern L. 2010. Survival of Ixodes ricinus (Acari: Ixodidae) under challenging conditions of temperature and humidity is influenced by Borrelia burgdorferi sensu lato infection. J Med Entom. 47(6):1196–1204. doi: 10.1603/ME10111.
- Herrmann JA, Dahm NM, Ruiz MO, Brown WM. 2014. Temporal and spatial distribution of tick-borne disease cases among humans and canines in Illinois (2000–2009). Environ Health Insights. 8(s2). doi: 10.4137/EHI.S16017.
- Jacquez GM. 1996. A k nearest neighbour test for space–time interaction. Statist Med. 15(18):1935–1949. doi: 10.1002/(SICI)1097-0258(19960930)15:183C1935::AID-SIM4063E3.0.CO;2-I.
- Jacquez GM. 2000. Spatial analysis in epidemiology: nascent science or a failure of GIS? J Geogr Syst. 2(1):91–97. doi: 10.1007/s101090050035.
- Jiménez-Valverde A, Lobo JM, Hortal J. 2008. Not as good as they seem: the importance of concepts in species distribution modelling. Divers Distrib. 14(6):885–890. doi: 10.1111/j.1472-4642.2008.00496.x.
- Johnson TL, Bjork JKH, Neitzel DF, Dorr FM, Schiffman EK, Eisen RJ. 2016. Habitat suitability model for the distribution of Ixodes scapularis (Acari: Ixodidae) in Minnesota. J Med Entomol. 53(3):598–606. doi: 10.1093/jme/tjw008.
- Khalaf JM, Mohammed IA, Karim AJ. 2018. The epidemiology of tick in transmission of Enterobacteriaceae bacteria in buffaloes in Marshes of the south of Iraq. Vet World. 11(12):1677. doi: 10.14202/vetworld.2018.1677-1681.
- Kumar S, Stohlgren TJ. 2009. Maxent modeling for predicting suitable habitat for threatened and endangered tree Canacomyrica monticola in New Caledonia. J Ecol Nat Environ. 1(4):94–98. http://www.academicjournals.org/JENE.
- Kumar S, Yee WL, Neven LG. 2016. Mapping global potential risk of establishment of Rhagoletis pomonella (Diptera: Tephritidae) using MaxEnt and CLIMEX niche models. J Econ Entomol. 109(5):2043–2053. doi: 10.1093/jee/tow166.
- Kuri F, Murwira A, Murwira KS, Masocha M. 2014. Predicting maize yield in Zimbabwe using dry dekads derived from remotely sensed Vegetation Condition Index. Int J Appl Earth Obs Geoinf. 33:39–46. doi: 10.1016/j.jag.2014.04.021.
- Manatsa D, Mushore TD, Gwitira I, Sakala LC, Ali LH, Chemura A, Masukwedza GI, Mupuro JM, Mugandani R, Wuta M, et al. 2020. Revision of Zimbabwe’s agro-ecological zones. Harare: Zimbabwe National Geospatial Agency (ZINGSA), Government of Zimbabwe.
- Marcer A, Sáez L, Molowny-Horas R, Pons X, Pino J. 2013. Using species distribution modelling to disentangle realised versus potential distributions for rare species conservation. Biol Conserv. 166:221–230. doi: 10.1016/j.biocon.2013.07.001.
- Mashoko E, Muchenje V, Ndlovu T, Mapiye C, Chimonyo M, Musemwa L. 2007. Beef cattle production in a peri-urban area of Zimbabwe. J Sustain Dev Afr. 9:121–132.
- Mavedzenge BZ, Mahenehene J, Murimbarimba F, Scoones I, Wolmer W. 2008. The dynamics of real markets: cattle in southern Zimbabwe following land reform. Dev Change. 39(4):613–639. doi: 10.1111/j.1467-7660.2008.00498.x.
- McHugh ML. 2011. Multiple comparison analysis testing in ANOVA. Biochem Med. 21(3):203–209. https://hrcak.srce.hr/72939.
- Mupangwa W, Walker S, Twomlow S. 2011. Start, end and dry spells of the growing season in semi-arid southern Zimbabwe. J Arid Environ. 75(11):1097–1104. doi: 10.1016/j.jaridenv.2011.05.011.
- Moyo S. 2011. Land concentration and accumulation after redistributive reform in post-settler Zimbabwe. Rev Afr Polit Econ. 38(128):257–276. doi: 10.1080/03056244.2011.582763.
- Ndebele JJ, Muchenje V, Mapiye C, Chimonyo M, Musemwa L, Ndlovu T. 2007. Cattle breeding management practices in the Gwayi smallholder farming area of South-Western Zimbabwe. Livest Res Rural Dev. 19(11). https://www.lrrd.cipav.org.co/lrrd19/12/ndeb19183.htm.
- Nhokwara SM, Kono H, Kubota S, Jubenkanda M. 2023. Communication medium in theileriosis control: the factors that determine disease knowledge among smallholder farmers in Zimbabwe. Trop Anim Health Prod. 55(2):83. doi: 10.1007/s11250-023-03466-x.
- Omodior O, Kianersi S, Luetke M. 2019. Spatial clusters and non-spatial predictors of tick-borne disease diagnosis in Indiana. J Community Health. 44(6):1111–1119. doi: 10.1007/s10900-019-00691-0.
- Ostfeld RS, Brunner JL. 2015. Climate change and Ixodes tick-borne diseases of humans. Phil Trans R Soc B. 370(1665):20140051. doi: 10.1098/rstb.2014.0051.
- Pearson RG, Raxworthy CJ, Nakamura M, Townsend Peterson A. 2007. Predicting species distributions from small numbers of occurrence records: a test case using cryptic geckos in Madagascar. J Biogeogr. 34(1):102–117. doi: 10.1111/j.1365-2699.2006.01594.x.
- Peter TF, Perry BD, O’Callaghan CJ, Medley GF, Shumba W, Madzima W, Burridge MJ, Mahan SM. 1998. The distribution of heartwater in the highveld of Zimbabwe, 1980–1997. Onderstepoort J Vet Res. 65(3):177–187. http://hdl.handle.net/2263/20369.
- Phillips SJ, Anderson RP, Schapire RE. 2006. Maximum entropy modeling of species geographic distributions. Ecol Modell. 190(3–4):231–259. doi: 10.1016/j.ecolmodel.2005.03.026.
- Raghavan RK, Goodin DG, Hanzlicek GA, Zolnerowich G, Dryden MW, Anderson GA, Ganta RR. 2016. Maximum entropy-based ecological niche model and bio-climatic determinants of lone star tick (Amblyomma americanum) niche. Vector Borne Zoonotic Dis. 16(3):205–211. doi: 10.1089/vbz.2015.1837.
- Randolph SE. 2008. The impact of tick ecology on pathogen transmission dynamics. In: Ticks: biology, disease and control. Cambridge: Cambridge University Press; p. 40–72.
- Rashid M, Rashid MI, Akbar H, Ahmad L, Hassan MA, Ashraf K, Saeed K, Gharbi M. 2019. A systematic review on modelling approaches for economic losses studies caused by parasites and their associated diseases in cattle. Parasitology. 146(2):129–141. doi: 10.1017/S0031182018001282.
- Ratner B. 2010. Variable selection methods in regression: ignorable problem, outing notable solution. J Target Meas Anal Mark. 18(1):65–75. doi: 10.1057/jt.2009.26.
- Rebelo H, Jones G. 2010. Ground validation of presence-only modelling with rare species: a case study on barbastelles Barbastella barbastellus (Chiroptera: Vespertilionidae). J Appl Ecol. 47(2):410–420. doi: 10.1111/j.1365-2664.2009.01765.x.
- Ren J, Liu M, Liu Y, Liu J. 2022. Optimal resource allocation with spatiotemporal transmission discovery for effective disease control. Infect Dis Poverty. 11(1):34. doi: 10.1186/s40249-022-00957-1.
- Ripley B, Venables B, Bates DM, Hornik K, Gebhardt A, Firth D, Ripley MB. 2013. Package ‘mass’. Cran R. 538:113–120. http://cran-r.c3sl.ufpr.br/web/packages/MASS/MASS.pdf.
- Rodríguez SD, Ortiz MAG, Ocampo RJy, Murguía CAV. 2009. Molecular epidemiology of Bovine anaplasmosis with a particular focus in Mexico. Infect Genet Evol. 9(6):1092–1101. doi: 10.1016/j.meegid.2009.09.007.
- Sage KM, Johnson TL, Teglas MB, Nieto NC, Schwan TG. 2017. Ecological niche modeling and distribution of Ornithodoros hermsi associated with tick-borne relapsing fever in western North America. PLOS Negl Trop Dis. 11(10):e0006047. doi: 10.1371/journal.pntd.0006047.
- Sardà-Palomera F, Brotons L, Villero D, Sierdsema H, Newson SE, Jiguet F. 2012. Mapping from heterogeneous biodiversity monitoring data sources. Biodivers Conserv. 21(11):2927–2948. doi: 10.1007/s10531-012-0347-6.
- Shaweno D, Trauer JM, Doan TN, Denholm JT, McBryde ES. 2021. Geospatial clustering and modelling provide policy guidance to distribute funding for active TB case finding in Ethiopia. Epidemics. 36:100470. doi: 10.1016/j.epidem.2021.100470.
- Shekede MD, Chikerema SM, Spargo M, Gwitira I, Kusangaya S, Mazhindu AN, Ndhlovu DN. 2021. Spatial clustering of fourteen tick species across districts of Zimbabwe. BMC Vet Res. 17(1):1–9. doi: 10.1186/s12917-021-02792-2.
- Sungirai M, Moyo DZ, De Clercq P, Madder M. 2016. Communal farmers’ perceptions of tick-borne diseases affecting cattle and investigation of tick control methods practiced in Zimbabwe. Ticks Tick Borne Dis. 7(1):1–9. doi: 10.1016/j.ttbdis.2015.07.015.
- Tagwireyi P, Ndebele M, Chikurunhe W. 2022. Climate change diminishes the potential habitat of the bont tick (Amblyomma hebraeum): evidence from Mashonaland Central Province, Zimbabwe. Parasites Vectors. 15(1):237. doi: 10.1186/s13071-022-05346-z.
- Tavirimirwa B, Mwembe R, Ngulube B, Banana NYD, Nyamushamba GB, Ncube S, Nkomboni D. 2013. Communal cattle production in Zimbabwe: a review. Livest Res Rural Dev. 25(12).
- Tesfamariam BG, Gessesse B, Melgani F. 2022. MaxEnt-based modeling of suitable habitat for rehabilitation of Podocarpus forest at landscape-scale. Environ Syst Res. 11(1):1–12. doi: 10.1186/s40068-022-00248-6.
- Title PO, Bemmels JB. 2018. ENVIREM: an expanded set of bioclimatic and topographic variables increases flexibility and improves performance of ecological niche modeling. Ecography. 41(2):291–307. doi: 10.1111/ecog.02880.
- Torina A, Alongi A, Naranjo V, Estrada-Peña A, Vicente J, Scimeca S, Marino AMF, Salina F, Caracappa S, de la Fuente J. 2008. Prevalence and genotypes of Anaplasma species and habitat suitability for ticks in a Mediterranean ecosystem. Appl Environ Microbiol. 74(24):7578–7584. doi: 10.1128/AEM.01625-08.
- Wisz MS, Hijmans RJ, Li J, PeteRSon AT, Graham CH, Guisan A. 2008. Effects of sample size on the performance of species distribution models. Divers Distrib. 14(5):763–773. doi: 10.1111/j.1472-4642.2008.00482.x.
- Yang GJ, Vounatsou P, Zhou XN, Tanner M, Utzinger J. 2005. A Bayesian-based approach for spatio-temporal modeling of county level prevalence of Schistosoma japonicum infection in Jiangsu province, China. Int J Parasitol. 35(2):155–162. doi: 10.1016/j.ijpara.2004.11.002.
- Zannou OM, Ouedraogo AS, Biguezoton AS, Abatih E, Coral-Almeida M, Farougou S, Yao KP, Lempereur L, Saegerman C. 2021. Models for studying the distribution of ticks and tick-borne diseases in animals: a systematic review and a meta-analysis with a focus on Africa. Pathogens. 10(7):893. doi: 10.3390/pathogens10070893.