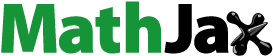
ABSTRACT
Forest damage caused by heavy wet snow accumulation in the canopy is the second most important abiotic forest disturbance agent in Nordic conifer stands after wind. The extent and frequency of snow damage in the future climate in the Nordic region is a major uncertainty. Few mechanistic models of snow damage risk to trees exist that could support forest management scenario analysis and decision making. We propose a snow damage risk model consisting of a numerical weather prediction-based snow accumulation model for forest canopies and a mechanistic critical snow load model. Snow damage probability predictions were validated on snow breakage data from the winters of 2016 and 2018 covering 3.5 million individual trees in south-eastern Norway derived from pre- and post-damage aerial laser scanning campaigns. The proposed model demonstrated satisfactory damage and no-damage class separation with an AUC of 0.72 and 0.77 in Norway spruce and Scots pine, respectively, and an F1 score of 0.7 in conifers taller than 10 m that suffered moderate stem breakage. The model achieved a classification accuracy that is comparable to that of statistical models but is simpler and requires fewer inputs.
Introduction
Heavy wet snow as a forest disturbance agent
Forest damage in the form of both stem breakage and uprooting caused by heavy wet snow accumulation in the tree crowns has occurred several times in Norway in recent years. For instance, in early 2018 along the south-eastern coast, then in 2020–2021 both in inland areas and in the coastal hills in the south-east, and again as recently as 2022–2023. The winter of 2020–2021 brought the greatest amount of snow damage over the past decade, with a conservative estimate of economic loss at EUR 3 million (NRK Vestfold og Telemark Citation2021). To add some context, the few existing estimates for the entire European Union range from 1 to 6 million m3 (3% of the total damage) annually over the past 100 years (Nykänen et al. Citation1997; Schelhaas et al. Citation2003); in Finland, annual insurance payouts due to snow damage averaged EUR 0.5 million over the past three decades, which constituted 7% of the total insurance compensations to forest owners (Lehtonen et al. Citation2016). In Finland and Norway alike, snow has become in the past decade the second most important abiotic forest disturbance agent after wind in terms of insurance compensation. At the same time, a decreasing trend was found by Díaz-Yáñez et al. (Citation2016) in the frequency of snow damage events in Norway during the 1995–2014 period. In addition, any forest damage is capable of having indirect cascading effects triggering other natural disturbances, such as making the weakened trees susceptible to bark beetle attacks or increasing fuel loads, even in forests not typically prone to severe wildfires (Schroeder and Eidmann Citation1993; Klopcic et al. Citation2009; Machado Nunes Romeiro et al. Citation2022). From a short-term perspective, the direct effects of snow damage on the economic performance of individual forest estates and the whole forestry industry alike involve reduced timber quality and value, increased harvesting costs, and timber volume loss. The long-term effects of snow damage manifest themselves in reduced forest growth and lower harvest levels.
Reducing snow damage may require adaptations of the prevalent silvicultural practices. However, the existing body of knowledge on the occurrence in space and time of heavy snowfall, the physics of snow accumulation in tree crowns, and the mechanics of tree resistance to breakage appears to be limited. Furthermore, little scientific literature on adaptations to silvicultural practices exists in Norway and, unlike bark beetle attacks, in respect of which a preparedness plan and silvicultural recommendations were developed by the Norwegian Agriculture Agency (Landbruksdirektoratet Citation2021), nothing similar exists for snow damage. The prevalent practical advice aimed at reducing snow damage is heavy pre-commercial thinning, which is assumed to result in a greater single-tree stability through larger root systems and larger tree stem diameters, as well as more symmetric crowns (Solberg et al. Citation2022; Skogbrand Forsikringsselskap Gjensidig Citation2023). Adopting this practice, however, would mean reduced volumes and quality of timber (Mäkinen and Isomäki Citation2004; Mäkinen and Hein Citation2006; Moore et al. Citation2009; Šilinskas et al. Citation2020). An important direction of research is thus finding silvicultural practices balancing snow damage avoidance and revenue. This requires quantitative biological and economical assessment of any proposed silvicultural practices in terms of snow damage risk.
A risk does exist that the extent and frequency of wet snow damage in Nordic boreal forests might increase in the future as a consequence of the more frequent heavy precipitation events during milder and more humid winters (IPCC Citation2014). However, a clear-cut conclusion is hard to arrive at due to contradictory evidence and multiple parallel trends that might be at work. While forest disturbance due to snow and ice may be expected to become less frequent and severe globally under a future warmer and either drier or wetter climate, in Europe the prevailing expectations are of more snow damage under a warmer and wetter climate and of less damage under a warmer, but drier climate (Seidl et al. Citation2017). Subcontinent-scale studies of forest disturbance in a changing climate offer a more nuanced picture: e.g. while Patacca et al. (Citation2023) expect global warming to make snow damage less frequent in Europe, they conclude that the uncertainty associated with the seasonality and severity of extreme wet snow precipitation events is likely to increase. This is in line with Masson-Delmotte et al. (Citation2021), arguing that major interregional and interannual variability is to be expected in precipitation, and the fine-scale heterogeneous pattern of wet snowfall makes the overall direction of change in snow loads difficult to predict (Räisänen Citation2008, Citation2016; Strasser Citation2008; Lehtonen et al. Citation2016; Hawcroft et al. Citation2018; Ohba and Sugimoto Citation2020). Both O’Gorman (Citation2014) and Räisänen (Citation2016) point out that the future trend in northern Europe towards higher mean annual snowfall in colder areas and lower snowfall in warmer areas is more clear and more systematic than the trend in the intensity of extreme daily snowfall, which may remain virtually unchanged over a broad range of climatological monthly air temperatures. Quante et al. (Citation2021) emphasize that extreme snowfall might become less frequent, but hardly less intense in the future in Western Europe. Räisänen (Citation2016) concludes that a greater share of annual snowfall may in the future occur in periods with close-to-zero air temperatures, leading to higher snow accumulation in the forest canopy.
In their study of future snow loads in the built environment in the Nordic countries, Larsson Ivanov et al. (Citation2022) found that the inland and higher-elevation areas on the east side of the Scandinavian mountains may towards the mid-twenty-first century experience an increase in extreme snow loads in all future climate scenarios (the increase is stronger in low-emission scenarios), while the coastal areas may experience a significant decrease. We note that their predicted spatial pattern of higher extreme snowfall is in strong agreement with the forest snow damage observations made in southern Norway over the past decade (Søgaard et al. Citation2017).
Snow accumulation in tree crowns is controlled by not only the amount of snowfall, air temperature, and relative humidity, but also wind speed. High risk of snow accretion is associated with air temperatures between −3°C and +1°C and wind speeds below 6 m s−1; wind speed is negatively correlated with any type of snow load accumulation due to the fact that higher wind contributes to snow removal from tree crowns (Lehtonen et al. Citation2014). The effect of climate change on future windiness in northern Europe remains uncertain: Gregow et al. (Citation2020) found little consensus in the literature apart from the expectation that autumn wind speed may slightly increase and that westerly winds may become more common due to the eastward extension of cyclone tracks. This prognosis is shared by a number of other recent studies (Groenemeijer et al. Citation2016; Kjellström et al. Citation2018; Ruosteenoja et al. Citation2019). Feser et al. (Citation2015) note that while the number of storms over northwestern Europe may remain unchanged, their intensities may, similarly to those of snowfall, increase. Future snow accumulation in forest canopies will, thus, be to a greater extent controlled by a combination of mean and extreme snowfall levels and surface air temperature, rather than windiness.
Among the Nordic countries, the future trends in snowfall and wind appear to be studied most extensively for Finland and, to a lesser degree, Sweden. Regarding Finland, the general outlook is that forest canopy snow loads are expected to increase in the east and north of Finland and decrease in the south and west, both along the coast and in the interior (Venäläinen et al. Citation2020). Lehtonen et al. (Citation2016) offer additional details by breaking down the total crown snow load into components (rime, dry snow, wet snow, frozen snow, see Lehtonen et al. (Citation2014)): in the upcoming two decades, only small changes are predicted with a 10–30% increase in rime, wet snow, and frozen snow loads in northern Finland and no noticeable change elsewhere.
In Sweden, Larsson Ivanov et al. (Citation2022) found similarly large regional differences in the generic case of future snow loads on the built environment; south Sweden and the east coast will experience a decrease in snow loads, while an increase is expected in the north and on the lee (eastern) side of the Scandinavian mountains.
The existing literature indicates that no generic prognosis can be made regarding the future severity, spatial distribution, and frequency of heavy snow precipitation on a subcontinental (Europe), or even regional (the Nordics) scale. The outlook for forest snow damage has necessarily to be nuanced depending on local climate, topography, and forest composition.
Modeling approaches to snow breakage in conifers
Although several bioeconomic scenario analysis models exist in Norway, these lack the capability to predict the snow damage risk. Output from a snow damage risk model should include both probability of damage and its effect (e.g. share of trees damaged) based on the forest structure and/or single-tree variables used in the scenario model, taking into account climatic variables. A recent review of forest disturbance models in Machado Nunes Romeiro et al. (Citation2022) found very few examples of models that could be used in this way. One of the few models developed specifically for Norwegian conditions (Díaz-Yáñez et al. Citation2019) is based on forest structure variables as well as topographic and climatic variables as input, but predicts the combined risk of wind and snow damage.
There are relatively few attempts to model snow damage risk to trees and branches using a mechanistic modeling approach rather than a statistical one. Some examples include Petty and Worrell (Citation1981), Cannell and Morgan (Citation1989), Peltola et al. (Citation1999), and Nock et al. (Citation2021). Most models of snow damage tend to be statistical models based on observed snow damage (e.g. Valinger and Fridman (Citation1997, Citation1999), Jalkanen and Mattila (Citation2000), Lehtonen et al. (Citation2014), Díaz-Yáñez et al. (Citation2019), and Suvanto et al. (Citation2021)). Such statistical models are only of use if a large amount of snow damage data is available, along with the key input variables in the models in order to make predictions in other locations or into the future (see Suvanto et al. (Citation2021), Nykänen et al. (Citation1997), and Lehtonen et al. (Citation2014)). They do not allow the investigation of damage to individual trees based on tree characteristics and the level of snowfall from a snow damage event as would be possible with a mechanistic model. Considering existing mechanistic models, the Cannell and Morgan (Citation1989) and the Nock et al. (Citation2021) models only deal with branch breakage, while the Peltola et al. (Citation1999) and the Petty and Worrell (Citation1981) models, although they do deal with snow damage to the whole tree, assume either a displaced crown due to the stem shape or an initial deflection due to the wind. No previous paper, to our knowledge, deals with a mechanistic understanding of snow damage to tree stems without including the effect of wind or initial crown displacement. In this paper, we present a simple mechanistic model that does not require any initial stem displacement and predicts only the snow load required to damage the stems of individual trees. It is very similar in philosophy and physics to the model by Petty and Worrell (Citation1981), but it works on the principle that when the snow load reaches a certain level, the system becomes unstable and even the slightest displacement from any cause (e.g. wind or uneven snow distribution in the crown) results in the collapse of the tree. This means that it is possible to calculate the critical snow load for stem damage with no requirement to include a wind speed or to create an asymmetric distribution of snow within the crown.
In this paper, we assess the ability of this idealized mechanistic model (Section 2.3) based on functions in the ForestGALES model (Hale et al. Citation2015) to predict stem breakage in conifers at a single-tree level when exposed to heavy wet snow loads. ForestGALES is a hybrid mechanistic model designed to predict the critical wind speed (CWS) leading to tree stem breakage or uprooting at the single-tree or stand level and includes the option to add a snow load in the tree crown.
In November 2016 and January 2018, parts of south-eastern Norway experienced two heavy wet snowfall events. One of the affected areas was the Fritzøe Skoger forest estate located in both the hemiboreal and boreal zones, where high levels of snow accumulation occurred in the forest canopy in the altitude interval of 100–300 m above sea level (m.a.s.l.), resulting in extensive stem breakage, both higher in the tree crown and closer to the ground. Snow damage was mostly concentrated in Norway spruce (Picea abies), with Scots pine (Pinus sylvestris) representing only approximately one quarter of the damaged trees. No evidence exists, however, indicating that the heavy snowfall events also involved elevated wind speeds, making it impossible to directly apply ForestGALES to explicitly estimate the critical snow load (CSL), rather than CWS. In addition to the CSL, a probability assessment of snow breakage would require a reference (actual or estimated) snow load value that the given trees were exposed to, or alternatively a distribution of values characterizing the local “snow climate.” However, the snow precipitation and snow cover data available for the area of interest (Norwegian Meteorological Institute, Norwegian Water Resources and Energy Directorate, and Norwegian Public Roads Administration Citation2023) was found to be too coarse with a grid size of 1 km and did not adequately capture the observed effect of terrain elevation on snow accumulation. Furthermore, the data was presented as snow load on the ground surface, rather than accumulation of snow in a three-dimensional forest canopy.
We present in this paper (1) a high-resolution (grid size 500 m) numerical weather prediction-based snow accumulation model providing the value of snow accumulation per unit area of forest canopy (Section 2.2), and (2) a mechanistic model to estimate CSL based on a set of tree attributes typically available from a single-tree aerial laser scanning (ALS) or aerial photogrammetric forest inventory (Section 2.3). Thereafter, we compare the predicted snow breakage probabilities to damage observations inferred from the bitemporal forest inventory data collected in 2014 and 2020 and assess the discrimination ability and predictive power of the proposed combination of the snow accumulation and CSL models.
Materials and methods
Study area and single-tree forest inventory data
The Fritzøe Skoger forest is a 73,000-ha private forest estate, where over 60% of the area is productive forest, of which more than 50% is dominated by Norway spruce, according to the forest management plan (). For several decades, the primary regeneration method was planting of pure spruce stands, while in recent years this has transformed into establishing a greater share of mixed stands of Norway spruce and Scots pine. In recent decades, regular thinning has been minimized and stand density has mainly been controlled by pre-commercial thinning. The estate lies in a hilly terrain with elevations ranging from sea level up to 600 m.a.s.l. Most of the forest area has soils originating from shallow and discontinuous till sediments. At elevations lower than 100 m.a.s.l., the dominant conifer species is Norway spruce (>85%); the proportion of Scots pine increases to close to 25% at mid-elevations and becomes dominant (>50%) starting at 600 m.a.s.l.
In 2014 (prior to the snow damage events) and 2020 (after the snow damage events), single-tree forest inventories were conducted by Foran Sverige AB, a forestry mapping and consulting services provider, covering the entire Fritzøe Skoger forest estate, based on two ALS and aerial photography (three natural color and one near infrared bands) campaigns with a nominal point density of 8 and 10 points m−2, respectively. The ALS point cloud had a horizontal and vertical accuracy of 0.21 and 0.28 m, respectively, based on measurements of 7 control profiles; the orthophoto mosaic resulting from the aerial photography campaign had a resolution of 0.25 m and a horizontal accuracy of 0.07 m, based on measurements of 7 ground control points (both accuracies reported for the 2020 aerial surveys). The two datasets contained such single-tree variables as treetop coordinates in ETRS89 UTM 32N, ALS tree height , tree crown radius
derived by tree crown segmentation, both in m. Treetops were identified by finding local maxima in the ALS point cloud that were at least 2 m higher than their surroundings, and their locations relative to neighboring treetops were used to segment the forest canopy into tree crown polygons and estimate crown radii. Diameter at breast height
in cm estimated by control plot-calibrated allometric models as a function of tree species,
, and
. Tree species classification from the pre-damage 2014 inventory was used, based on a combination of spectral, such as NDVI (Tucker Citation1979), and crown geometry properties from a total of 4928 field-identified trees (3314 Norway spruces, 637 Scots pines, 977 deciduous trees) across 152 control plots.
Specifically, the following tree diameter functions were applied.
For Scots pine:
(1)
(1) For Norway spruce:
(2)
(2) where
is diameter at breast height with bark in cm.
The tree diameter functions above were calibrated using a total of 379 control plots established randomly in 45 calibration stands. ALS-based estimates of diameter at breast height were validated against field measurements and aggregated on a calibration stand level
.
Diameter at breast height was subsequently estimated with correction for double bark thickness as follows:
(3)
(3) where
is double bark thickness in cm, estimated by the equations below.
For Scots pine (Brantseg Citation1969):
(4)
(4) For Norway spruce (Vestjordet Citation1967):
(5)
(5) To identify trees that suffered stem breakage as a result of the heavy snowfall in 2016 and 2018, we matched identical trees in the 2014 and 2020 datasets by finding the Euclidean distance between pairs of treetop coordinates, selecting nearest neighbors, and excluding those separated by more than 0.25 m. Heavy accumulation of snow in the tree crowns was the primary cause of stem breakage in the study area in the past decade with no reports on windstorms, forest fires, or other abiotic disturbances causing reduction in treetop heights between 2014 and 2020. The matched dataset was dominated by Norway spruce (63% of the tree count), followed by birch (Betula spp.; 20%), and Scots pine (16%), as classified in the pre-damage 2014 inventory. We filtered out birch and applied a tree height constraint by excluding trees shorter than 10 m and taller than 30 m as measured in 2014. The reason for excluding younger and thus shorter trees was their presumed better ability to withstand high snow loads due to a lower modulus of elasticity and increased flexibility, while trees taller than 30 m were excluded to remove ALS returns potentially misclassified as treetops as, according to the Norwegian forest resource map SR16 (NIBIO Citation2022), the 99th percentile of dominant tree height in the study area was 29.3 m for Norway spruce and 27.3 m for Scots pine.
A given tree was assumed to have suffered snow breakage if its height as measured in 2014 and 2020 recorded a decrease of ≥0, 1, 2, or 3 m (). The four thresholds were applied to the single-tree dataset stratified by tree height in 2014 (
) into three subsets
≥ 10, 15, and 20 m for Norway spruce and Scots pine separately to obtain 24 strata. To balance the individual strata, random samples equal in size to the number of damaged trees were taken from the individual no-damage subsets over 100 iterations. presents the summary statistics of the single-tree stratum defined by
≥ 10 m as estimated in the 2014 inventory and shows characteristic examples of snow breakage of various severity in Norway spruce and Scots pine.
Figure 2. Examples of snow breakage of various degrees of severity in (a) Norway spruce and (b) Scots pine. (Photos by Peter Zubkov.)

Table 1. Summary statistics of the 2014 single-tree inventory stratum defined by ≥ 10 m (
) by tree species.
High-resolution snow accumulation model
Due to the hilly terrain and the lack of representative weather stations in the near vicinity of the study area, the meteorological dataset used in this study was obtained through numerical weather prediction (NWP). The data was prepared with the Weather Research and Forecasting (WRF) model version WRF-ARW 4.1.2 (Skamarock et al. Citation2021). It is a state-of-the-art mesoscale atmospheric model, used both for operational forecasting and for simulation of historic weather (i.e. hindcasting). Short-term hindcast simulations were produced by simulating the weather conditions during the entire months of November 2016 and January 2018 for a domain sized 43.5 by 67.5 km containing the Fritzøe Skoger forest estate at a 500 m horizontal grid spacing and at 51 vertical model levels throughout the atmosphere. All simulations were initiated and forced on the lateral boundaries by the global re-analysis ECMWF–ERA5 dataset (Hersbach Citation2016). In addition to producing standard weather variables, such as wind speed, wind direction, temperature, humidity and precipitation amount, the NWP model outputs detailed information about the precipitation properties, such as mass concentration and liquid water fraction (LWF) of snow and precipitation particles. The level of detail depends on the parameterization scheme of cloud and precipitation processes. In this study, we used the Thompson-Eidhammer aerosol-aware microphysics scheme (Thompson and Eidhammer Citation2014), with improvements to the melting layer parameterization (Iversen et al. Citation2021). Further information regarding the model setup is available in Nygaard et al. (Citation2022).
Wet snow accumulation was calculated as a combination of horizontal and vertical precipitation fluxes for an idealized tree assuming a conic crown with a projected area of 1 m2 in the horizontal and vertical plane (Lehtonen et al. Citation2014). Hourly time series of precipitation, wind, temperature, and humidity were extracted from the WRF hindcast, and the accumulated wet snow was calculated for each time step. The time-dependent snow accumulation follows that of Gregow et al. (Citation2008), however our implementation of the accretion model defines wet snow as precipitation with a LWF in the range of 0–0.5. This is based on physical experiments on wet snow accumulation (Sakakibara et al. Citation2007; Hefny et al. Citation2009) which show that the adhesion of snow to various surfaces is restricted to a certain range of LWF values.
The snow loss component of the model follows Gregow et al. (Citation2008) and involves melting and wind erosion. The melting rate increases with temperature in the range of 0 to +2.3 °C, with all snow melting away above the upper threshold. A cubic function was applied to account for snow loss due to wind erosion.
Mechanistic critical snow load modeling and snow damage probability estimation
To calculate the critical snow load leading to tree stem breakage, we used a simplification of the deflection loading factor (DLF) function in the ForestGALES model that calculates the additional moment created by a tree crown displaced by the accumulated snow. The form of the DLF function is as follows (Locatelli et al. Citation2022):
(6)
(6) where
is the crown depth in m,
is the weight of the snow accumulation in the tree crown in kg,
is the crown weight in kg,
is tree height in m,
is the stem weight of the tree in kg,
is the gravitational acceleration constant (9.81 m s−2),
is the bending moment exerted by the wind on the tree in Nm, and
is a function describing the displacement of the tree at different points
along the tree stem (Gardiner Citation1989; Quine et al. Citation2021). DLF does not depend on the wind speed because it is a multiplier applied to the moment due to the wind and is only a function of the characteristics of the tree (stem shape, stem density, stem stiffness, crown size, crown density). A value of DLF = 1 would mean that there is no contribution of the crown and stem to the overturning moment. The average value of DLF with no snow loading is 1.136 (Hale et al. Citation2015) so that the crown and stem displacement by the wind contributes an additional 13.6% to the overturning moment.
and
are estimated by the equations below:
(7)
(7)
(8)
(8) where
is the crown density in kg m−3,
is the tree crown radius in m,
and
are the regression coefficients.
The function in Equation (6) is given by the following equation, which assumes the stem shape follows a power to the 0.6 function with distance from the treetop (Quine et al. Citation2021):
(9)
(9) where
is the location along the tree stem measured from the treetop in m,
is the force of the wind,
is the modulus of elasticity for the given tree species,
is the ratio of the distance from the treetop to the point where the wind force
is applied to the tree height
, and
is the area moment of inertia at the base of the tree, approximated by:
(10)
(10) where
is the tree diameter at breast height in m.
The contribution from the stem weight in Equation (6) is marginal and can be ignored. The bending moment due to the wind can be approximated by
and we assume that the wind acts at the center of the tree crown, thus:
(11)
(11)
(12)
(12) Equation (9) can thus be simplified as follows:
(13)
(13) Equation (6) can accordingly be rewritten as:
As the denominator in Equation (14) approaches zero, the DLF approaches infinity and the tree collapses under the combined weight of its crown and accumulated snow without any additional wind loading. The CSL in kg can thus be derived as:
(15)
(15) Model parameter values used in Equations (7) to (15) are given in .
Table 2. Model parameter values used to calculate critical snow load (CSL) from Equations (7, 8 and 15) (from Locatelli et al. (Citation2022)).
Probability of tree stem breakage when exposed to a snow load above the CSL is assumed to be a logistic function of the form:
(16)
(16) where
is the difference between the modeled snow load in kg on the given tree (Section 2.2) and the CSL given by Equation (15). Logistic functions are regularly used in assessing the probability of an event varying between 0 and 1 in a smooth sigmoid shape (e.g. Hosmer et al. (Citation2013), Hale et al. (Citation2015)).
For spatial analysis and visualization of the snow accumulation models and snow breakage predictions, we used ArcGIS Pro version 3.1.
Model discrimination ability and accuracy assessment
To assess the discrimination ability of the CSL model, we calculated the value of the area under the receiver operating characteristic (ROC) curve (AUC) for each of the strata. To make an accuracy assessment of the binary snow breakage predictions, we chose to optimize the cutoff value of such as to maximize the sum of sensitivity
and specificity
in the resulting two-by-two confusion matrix, rather than apply a fixed classification threshold of
. For statistical analysis, we used R version 4.2.2 (R Core Team Citation2022), including the cutpointr package (Thiele and Hirschfeld Citation2021) for threshold optimization and accuracy assessment, and the ggplot2 package (Wickham Citation2009) for visualization. The accuracy measures calculated are
,
, and F1 score and are given as follows:
(17)
(17)
(18)
(18)
(19)
(19) where
,
,
, and
are, respectively, the true positives, true negatives, false positives, and false negatives in the corresponding binary confusion matrix.
The reported accuracy measures and were averaged over 100 resampling iterations on a balanced dataset using a bagging procedure.
Results and discussion
Snow accumulation model
The modeled snow loads as shown in tend to capture well the spatial distribution with higher values in the same elevation intervals as the observed forest snow damage. At higher elevations, the model mainly predicts precipitation as dry snow while in low-lying areas the precipitation is mainly modeled as sleet or rain due to a high LWF. We were prevented from quantifying the accuracy of the snow accumulation model by the absence of weather stations in the study area representing the elevation intervals of interest; the only alternative was comparing estimated snow load values with the spatial distribution of tree height decrease between 2014 and 2020 interpreted as snow breakage.
Figure 3. (a) Maximum snow accumulation maps of the study area in November 2016 and (b) January 2018 (numbers relate to terrain elevation contour intervals), (c) maximum mean snow accumulation (shading indicates +/− 2σ) by elevation in the respective time periods, and (d) snow-damaged trees in 2018 (for ) by elevation interval and tree species.
We found two patterns in the snow accumulation in different elevation intervals in November 2016 and January 2018 ((a–c)). In November 2016, the highest accumulation was modeled in the 100–200 m.a.s.l. interval (mean snow accumulation 86 kg m−2 with close to half these values in the adjacent 100 m elevation intervals above and below this range). In January 2018, the highest snow accumulation was modeled at 117 kg m−2 in the same 100–200 m.a.s.l. interval. However, the 2016 maximum was exceeded in a wider belt between 0 and 300 m.a.s.l. with no difference in snow accumulation at higher elevations ((c)). Extensive snow breakage in both Norway spruce and Scots pine was found in the 100–300 m.a.s.l. interval, with less damage to Scots pine at elevations below 100 m.a.s.l. where this species is relatively less common and a gradual increase in the proportion of damaged Scots pine starting at 400 m.a.s.l. ((d)). The distribution of the observed snow damage by elevation intervals reflects the distribution of the two tree species, with Norway spruce being the dominant species at lower elevations ().
The spatial distribution of snow damage demonstrated species-dependent statistical significance (), with clustering and dispersion patterns in the damage class, as indicated by the Getis-Ord Gi* statistic (Getis and Ord Citation1992): snow breakage was strongly clustered in the 100–200 m.a.s.l. interval in Norway spruce and in the 0–300 m.a.s.l. interval in Scots pine. A dispersion pattern was found above 400 m.a.s.l., mostly in snow-damaged Scots pine. The no-damage class showed clustering above 300 m.a.s.l. and dispersion below 200 m.a.s.l. in Norway spruce, with a similar, though less pronounced, pattern in Scots pine ((a and b)).
Figure 4. Snow accumulation (maximum of November 2016 and January 2018) and Getis-Ord Gi*-based spatial clustering of damage and no-damage observations in (a) Norway spruce and (b) Scots pine. Clustering and dispersion by snow accumulation by tree species in the (c) damage and (d) no-damage class (≥1 m).

Both clustering and dispersion correlated with snow accumulation: in the damage class, significant clustering () was found at median snow accumulation values higher than 115 kg m−2 in both Norway spruce and Scots pine. Conversely, in the no-damage class clustering was found in Norway spruce at median snow accumulations below 40 kg m−2, while dispersion was observed at values above 100 kg m−2 ((c and d)).
It should be kept in mind that the snow accumulation model (Section 2.2) was built for a highly simplified conifer crown, taken outside of its spatial and social context, including such factors as forest edge and gap effects, sheltering by neighbors, and snow load sharing with neighboring trees. Furthermore, as noted in Lehtonen et al. (Citation2014), the snow load is greatly affected by individual tree properties and adaptations, such as crown shape. For example, spruce trees at higher elevations tend to develop narrow, candle-shaped crowns that may accumulate higher snow loads than their lowland counterparts due to snow packing and the lower center of gravity preventing shedding of the snow load (Büsgen et al. Citation1929). This means that our model might underestimate snow accumulation at elevations above 400 m.a.s.l.
To the best of our knowledge, this study is the first ever attempt at predicting single-tree snow breakage using high-resolution NWP methods. In Suvanto et al. (Citation2021), the same objective was dealt with by directly applying the snow accumulation model of Lehtonen et al. (Citation2014) to the spatially more coarse ERA5 global reanalysis data and an “average” tree representing an entire forest stand, while in Lehtonen et al. (Citation2014) no predictions of the risk of tree breakage were made based on the NWP models (such as the meso-scale HIRLAM and the global IFS by ECMWF).
Critical snow loads and snow damage predictions
The CSL model (Section 2.3) combined with the snow accumulation model (Section 2.2) demonstrated satisfactory accuracy of snow damage predictions, with the highest classification accuracy achieved in the moderately restrictive tree height and height decrease
strata. Four combinations of the criteria giving the highest and most balanced (in terms of
vs.
) classification performance with their accuracy metrics for Norway spruce and Scots pine are presented in . Applying a height decrease
of at least 2 m to the subset of trees higher than 10 m in 2014 gave the best discrimination ability in terms of AUC (see (b) for the ROC curves). The benefits of applying a more restrictive
were most expressed in terms of sensitivity and negligible in terms of specificity, irrespective of tree species (). Applying the model to the strata defined by a higher
led to a major drop in
across the examined strata with a slight to moderate increase in
and negligible effect on AUC and F1 score. We conclude that the proposed model performed best on those conifers that have passed the juvenile development stage (higher than 10 m) at the time of exposure to a heavy snow load and suffered moderately severe stem breakage (height decrease greater than 2 m by the end of the period) as a consequence. In the following, we present and discuss our findings regarding this stratum, unless otherwise indicated.
Figure 5. (a) Density plots of damage probability () for the no-damage and damage classes by tree species for the entire dataset, showing the optimal
values for the best-performing stratum (
≥ 10 m,
≥ 2 m). (b) ROC curves, incl.
, and (c) sensitivity vs. specificity plot for the same stratum for Norway spruce (NS) and Scots pine (SP). Note the different scaling of the vertical axis, reflecting the class imbalance.
Table 3. Mean classification accuracy metrics (after 100 resampling iterations) of the snow breakage model for the four best performing combinations of minimum tree height in 2014 h2014 and tree height decrease Δh between 2014 and 2020, indicating the stratum size of the damage class and the value of
maximizing the F1 score (NS, Norway spruce; SP, Scots pine). Best-performing combination highlighted with bold.
It should be borne in mind that the value of is only a proxy for the actual loss of tree height as a result of snow breakage and is not corrected for height growth between 2014 and the time of breakage (late 2016 or early 2018). Depending on the site index and assuming a time interval of three vegetation seasons between 2014 and early 2018, the actual point of stem breakage corresponding to a
of 1 m can reasonably be expected to be located 2–3 m below the treetop (Kvaalen et al. Citation2015; Solberg et al. Citation2019). Light snow damage confined to <2 m below the treetop is thus outside the scope of this study and may be represented by a close to zero or positive
.
Two additional sources of uncertainty associated with the single-tree inventory data used are (1) accuracy of treetop identification and tree radius estimation and (2) reliability of the subsequent diameter at breast height estimates. Errors at the treetop identification stage may translate into (a) incorrectly matched treetops between 2014 and 2020, resulting in an imperfect reference dataset, and (b) incorrect diameter at breast height estimates, because crown size is part of the diameter functions (Equation (1 and 2)) and this could lead to incorrect CSL values.
We found that in the best-performing stratum, the damage class had a higher median value of compared to the no-damage class – 0.65 vs. 0.5 in Norway spruce and 0.4 vs. 0.25 in Scots pine ((c)). Across the two classes, Norway spruce had lower CSL values compared to Scots pine; this can traced back primarily to the difference in stem taper between the two species (), with stem taper values in Norway spruce approaching the zone of risk of snow breakage, i.e. 75 and higher (Petty and Worrell Citation1981). There was, however, only a slight difference in the CSL between the damage and no-damage classes in Norway spruce with the direction of the difference inconsistent with what was expected. Scots pine had, in contrast, a larger difference in the CSL of 2000kg vs. 1500 kg in the damage and no-damage classes, respectively, and a correct direction of the relationship ((b)). For the snow accumulation values, we found a major difference (1200 kg vs. 500 kg in the damage and no-damage classes, respectively) in Norway spruce and a smaller difference in Scots pine ((a)). The probability values (
) were also higher in the elevation interval 0–300 m.a.s.l., which is the one with the highest snow accumulation levels, especially in Norway spruce (). The
values correlated stronger with tree snow loads rather than the CSL (), suggesting that the occurrence of snow breakage was predominantly controlled by snow accumulation in both tree species. This is unsurprising considering the finding in Päätalo et al. (Citation1999) that snow accumulation above 60 kg m−2 makes conifers susceptible to stem damage and uprooting and the modeled snow accumulation greater than 60 kg m−2 across the elevation intervals of interest as shown in (c). A snow load of 60 kg m−2 can be conceptually interpreted as 60 cm of fresh snowfall, however Nykänen et al. (Citation1997) offer a word of caution indicating the oversimplified nature of this conversion ignoring LWF in the snow and side wind; besides, such conversion would imply a strong assumption of zero pre-existing snow load in the forest canopy.
Figure 6. Boxplots of (a) snow accumulation in the tree crowns, (b) CSL, and (c) for the no-damage and damage classes by tree species (
≥ 10 m,
≥ 2 m) (NS, Norway spruce; SP, Scots pine).

Figure 7. Boxplot of by elevation, class, and tree species (
≥ 10 m,
≥ 2 m) (NS, Norway spruce; SP, Scots pine).

Table 4. Correlation matrix of the damage probability () versus tree crown snow accumulation (SL) and CSL by tree species (NS, Norway spruce; SP, Scots pine) (
≥ 10 m).
We note that the detected snow breakage cannot be reliably attributed to a specific snow accumulation event, i.e. either November 2016 or January 2018, because of the unavailability of remotely sensed data of sufficiently high spatial resolution collected between 2014 and 2020. The observed snow damage pattern can arguably be interpreted a combined effect of the two events, i.e. that the trees were first weakened by sustained bending in 2016 (Petty and Worrell Citation1981) and suffered extensive stem breakage when exposed to even greater loads in 2018 (Nykänen et al. Citation1997).
Despite its simplicity, the CSL model (Section 2.3) performed reasonably well and demonstrated a satisfactory discrimination ability with a clear, though imperfect separation of classes ((a)). Similarly to the snow accumulation model in Section 2.2, we assumed a highly idealized conical tree crown shape without regard to the effect of tree species and elevation-dependent adaptations, such as candle-shaped vs. broad crowns in Norway spruce at higher vs. lower elevations, respectively (Lehtonen et al. Citation2014). We further assumed that the snow load acts symmetrically at the tree crown’s estimated center point; this may not hold in the situations of asymmetric snow accumulation due to wind or the shielding effect of the neighboring trees leading to an upward shift along the tree stem of the point where the snow load acts. The latter would cause stem breakage close to the treetop, a situation our model is not expressly designed to handle.
Model performance in the context of other existing snow damage models
In Suvanto et al. (Citation2021), the same winter of 2017–2018 marked by heavy snowfall was used to validate their model’s snow damage risk predictions for Finland, and similarly to us, they concluded that crown snow load was the main driver of snow breakage during that winter. Our snow damage model achieved a classification performance that is comparable to that reported in Valinger and Fridman (Citation1999) and Suvanto et al. (Citation2019), both following a statistical approach, but calculated for individual trees, rather than stand or plot level values and using a simpler, physics-based approach. A clear advantage of our proposed model is that it requires as inputs only the most basic ALS or photogrammetric single-tree characteristics and reference snow load values (modeled or historical), making it suitable for applications in forest management planning and scenario analysis.
Similarly to Peltola et al. (Citation1999), we saw that snow accumulations above 60 kg m−2 made both Norway spruce and Scots pine susceptible to stem breakage, however without any additional wind loading – since wind contributes to shedding of the crown snow accumulation (Nykänen et al. Citation1997; Lehtonen et al. Citation2014) – and irrespective of the tree’s location relative to the forest edge.
The performance of our mechanistic model is in line with the earlier finding reported in e.g. Nykänen et al. (Citation1997) that the main biotic factor controlling the CSL in conifers is stem diameter, followed by tree height, MOE, and crown length (see Equation 15), with the former two represented by taper, earlier identified as the single most important factor of tree stability under a snow load (Petty and Worrell Citation1981).
Further work
Obvious directions for further research into the risk of snow damage in Nordic conifer stands include:
Improvements to snow accumulation modeling, including making the model aware of the spatial and social status of trees (e.g. forest edge effects, sheltering by and snow load sharing between neighboring trees, and local topography) and making snow accumulation dependent on the tree species and crown shape.
Validating the snow accumulation model based on actual observations of snowfall composition and snow accretion to forest canopies.
Improvements to the CSL model, such as implementing more advanced stem taper functions, differentiating between tree species and their adaptations in terms of crown shapes and sizes, and importantly, adding functionality to predict the CSL at different heights along the tree stem (see Nykänen et al. (Citation1997)).
Conclusion
In this paper, we present (1) a high-resolution NWP-based snow accumulation model for forest canopies and (2) a mechanistic CSL model for predicting the risk of snow damage in common Nordic conifer tree species and we validate the predictions using snow damage observations collected from single-tree ALS surveys. Despite the simplified and idealized formulation of the CSL model, we found the combined snow damage model to be both intuitive and capable of discriminating between damage and no-damage classes in the event of moderately severe stem breakage and of correctly representing the relative contribution of CSL and snow accumulation to the probability of snow damage. Our accuracy assessment indicates that the proposed model is well suited for applications in scenario analysis and snow damage risk assessment.
Acknowledgements
The authors are grateful to Christer Sandum and Fritzøe Skoger AS for making available the ALS and forest inventory data and to Eva Solbjørg Flo Heggem for pre-processing the forest inventory data.
Disclosure statement
No potential conflict of interest was reported by the author(s).
Data availability statement
The data that support the findings of this study are available from the corresponding author, PZ, upon reasonable request. Restrictions may apply to the availability of the source data, which were used for this study and are available from the corresponding author with the permission of Fritzøe Skoger AS.
Additional information
Funding
References
- Brantseg A. 1969. Furu sønnafjells. Kubering av stående skog. Funksjoner og tabeller, Meddelelser fra Det norske skogforsøksvesen 26 (94–95). Vollebekk (Norway): Det norske skogforsøksvesen.
- Büsgen M, Münch E, Thomson T. 1929. Structure and life of forest trees. 3rd ed. London (UK): Chapman & Hall.
- Cannell MGR, Morgan J. 1989. Branch breakage under snow and ice loads. Tree Physiol. 5(3):307–317. doi:10.1093/treephys/5.3.307.
- Díaz-Yáñez O, Mola-Yudego B, Eriksen R, González-Olabarria JR. 2016. Assessment of the main natural disturbances on Norwegian forest based on 20 years of national inventory. PLoS One. 11(8):e0161361. doi:10.1371/journal.pone.0161361.
- Díaz-Yáñez O, Mola-Yudego B, González-Olabarria JR. 2019. Modelling damage occurrence by snow and wind in forest ecosystems. Ecol Modell. 408:108741. doi: 10.1016/j.ecolmodel.2019.108741.
- Feser F, Barcikowska M, Krueger O, Schenk F, Weisse R, Xia L. 2015. Storminess over the North Atlantic and Northwestern Europe—a review. Q J R Metereol Soc. 141(687):350–382. doi: 10.1002/qj.2364.
- Gardiner B. 1989. Mechanical characteristics of Sitka spruce. Forestry Commission Occasional Paper 24.
- Getis, A, Ord JK. 1992. The analysis of spatial association by use of distance statistics. Geogr Anal. 24(3):189–206. doi:10.1111/j.1538-4632.1992.tb00261.x.
- Gregow H, Puranen U, Venäläinen A, Peltola H, Kellomäki S, Schultz D. 2008. Temporal and spatial occurrence of strong winds and large snow load amounts in Finland during 1961–2000. Silva Fenn. 42(4). doi:10.14214/sf.231.
- Gregow H, Rantanen M, Laurila TK, Mäkelä A. 2020. Review on winds, extratropical cyclones and their impacts in Northern Europe and Finland, Reports 2020:3. Helsinki (Finland): Finnish Meteorological Institute.
- Groenemeijer P, Vajda A, Lehtonen I, Kämäräinen M, Venäläinen A, Gregow H, Púčik T. 2016. Present and future probability of meteorological and hydrological hazards in Europe, Technical Report. Report number: 608166-D 2.5. Delft: ESSL.
- Hale SE, Gardiner B, Peace A, Nicoll B, Taylor P, Pizzirani S. 2015. Comparison and validation of three versions of a forest wind risk model. Environ Model Softw. 68:27–41. doi: 10.1016/j.envsoft.2015.01.016.
- Hawcroft M, Walsh E, Hodges K, Zappa G. 2018. Significantly increased extreme precipitation expected in Europe and North America from extratropical cyclones. Environ Res Lett. 13(12):124006. doi:10.1088/1748-9326/aaed59.
- Hefny R, Kollar LE, Farzaneh M, Payrard C. 2009. Adhesion of wet snow to different cable surfaces. Paper presented at the 13th International Workshop on Atmospheric Icing of Structures, Andermatt; Sep 8–11, 2009; Switzerland.
- Hersbach H. 2016. The ERA5 atmospheric reanalysis. Paper presented at the American Geophysical Union Fall Meeting 2016; Dec 2016; San Francisco.
- Hosmer DW,J, Lemeshow S, Sturdivant RX. 2013. Applied logistic regression. 3rd ed, Wiley Series in Probability and Statistics. Hoboken (NJ): John Wiley & Sons.
- IPCC. 2014. Climate change 2014: synthesis report. Contribution of working groups I, II and III to the fifth assessment report of the intergovernmental panel on climate change. Core Writing Team, Pachauri RK, Meyer LA, editor. Geneva, Switzerland: IPCC.
- Iversen EC, Thompson G, Nygaard BE. 2021. Improvements to melting snow behavior in a bulk microphysics scheme. Atmos Res. 253:105471. doi:10.1016/j.atmosres.2021.105471.
- Jalkanen A, Mattila U. 2000. Logistic regression models for wind and snow damage in Northern Finland based on the National Forest Inventory data. For Ecol Manag. 135(1):315–330. doi:10.1016/S0378-1127(00)00289-9.
- Kjellström E, Nikulin G, Strandberg G, Christensen OB, Jacob D, Keuler K, Lenderink G, van Meijgaard E, Schär C, Somot S, et al. 2018. European climate change at global mean temperature increases of 1.5 and 2 °C above pre-industrial conditions as simulated by the EURO-CORDEX regional climate models. Earth Syst Dyn. 9(2):459–478. doi:10.5194/esd-9-459-2018.
- Klopcic M, Poljanec A, Gartner A, Boncina A. 2009. Factors related to natural disturbances in mountain Norway spruce (Picea abies) forests in the Julian Alps. Écoscience. 16(1):48–57. doi:10.2980/16-1-3181.
- Kvaalen H, Solberg S, May J. 2015. Aldersuavhengig bonitering med laserskanning av enkelttrær. Nibio report 1/67. Ås (Norway): NIBIO.
- Landbruksdirektoratet. 2021. Faglig beredskapsplan for stor granbarkbille. [accessed 2023 May 11]. https://www.landbruksdirektoratet.no/nb/skogbruk/barkbilleberedskap/faglig-beredskapsplan-for-stor-granbarkbille.
- Larsson Ivanov O, Bärring L, Wilcke RAI. 2022. Climate change impact on snow loads in Northern Europe. Struct Saf. 97:102231. doi:10.1016/j.strusafe.2022.102231.
- Lehtonen I, Hoppula P, Pirinen P, Gregow H. 2014. Modelling crown snow loads in Finland: a comparison of two methods. Silva Fenn. 48(3). doi:10.14214/sf.1120.
- Lehtonen I, Kämäräinen M, Gregow H, Venäläinen A, Peltola H. 2016. Heavy snow loads in Finnish forests respond regionally asymmetrically to projected climate change. Nat Hazard Earth Sys. 16(10):2259–2271. doi:10.5194/nhess-16-2259-2016.
- Locatelli T, Hale S, Nicoll B, Gardiner B. 2022. The ForestGALES wind risk model and the fgr R package. Edinburgh (UK): UK Forestry Commision.
- Machado Nunes Romeiro J, Eid T, Antón-Fernández C, Kangas A, Trømborg E. 2022. Natural disturbances risks in European boreal and temperate forests and their links to climate change – a review of modelling approaches. For Ecol Manag. 509:120071. doi:10.1016/j.foreco.2022.120071.
- Mäkinen H, Hein S. 2006. Effect of wide spacing on increment and branch properties of young Norway spruce. Eur J For Res. 125(3):239–248. doi:10.1007/s10342-006-0115-9.
- Mäkinen H, Isomäki A. 2004. Thinning intensity and growth of Norway spruce stands in Finland. For Int J Forest Res. 77(4):349–364. doi:10.1093/forestry/77.4.349.
- Masson-Delmotte VP, Zhai P, Pirani SL, Connors C, Péan S, Berger N, Caud Y, Chen L, Goldfarb MI, Scheel Monteiro PM. 2021. IPCC, 2021: summary for policymakers. In: Climate change 2021: the physical science basis. Contribution of Working Group I to the Sixth Assessment Report of the Intergovernmental Panel on Climate Change.
- Moore J, Achim A, Lyon A, Mochan S, Gardiner B. 2009. Effects of early re-spacing on the physical and mechanical properties of Sitka spruce structural timber. For Ecol Manag. 258(7):1174–1180. doi:10.1016/j.foreco.2009.06.009.
- NIBIO. 2022. SR16 - the Norwegian forest resource map. [accessed 2022 October 10]. https://kilden.nibio.no.
- Nock CA, Jones KF, Greene DF. 2021. Chapter 6. The effect of icing events on the death and regeneration of North American trees. In: Johnson EA, Miyanishi K, editor. Plant disturbance ecology. 2nd ed. San Diego: Academic Press; p. 213–252.
- Norwegian Meteorological Institute, Norwegian Water Resources and Energy Directorate, and Norwegian Public Roads Administration. 2023. Varsom SeNorge. [accessed 2023 April 15]. https://www.senorge.no.
- NRK Vestfold og Telemark. 2021. Rekordmange utbetalinger etter snøskader på skog. [accessed 2023 May 11]. https://www.nrk.no/vestfoldogtelemark/utbetaler-over-20_25-millioner-kroner-for-snobrekk-av-skog-1.15533034.
- Nygaard BE, Ingvaldsen K, Welgaard Ø. 2022. Development of ice load maps for structural design. Paper presented at the IWAIS XIX; June 2022; Montreal, Canada.
- Nykänen M-L, Broadgate M, Kellomäki S, Peltola H, Quine C. 1997. Factors affecting snow damage of trees with particular reference to European conditions. Silva Fenn. 31(2). doi:10.14214/sf.a8519.
- O’Gorman PA. 2014. Contrasting responses of mean and extreme snowfall to climate change. Nature. 512(7515):416–418. doi:10.1038/nature13625.
- Ohba M, Sugimoto S. 2020. Impacts of climate change on heavy wet snowfall in Japan. Clim Dyn. 54(5–6):3151–3164. doi:10.1007/s00382-020-05163-z.
- Päätalo M-L, Peltola H, Kellomäki S. 1999. Modelling the risk of snow damage to forests under short-term snow loading. For Ecol Manag. 116(1):51–70. doi:10.1016/S0378-1127(98)00446-0.
- Patacca M, Lindner M, Lucas-Borja ME, Cordonnier T, Fidej G, Gardiner B, Hauf Y, Jasinevičius G, Labonne S, Linkevičius E, et al. 2023. Significant increase in natural disturbance impacts on European forests since 1950. Glb Chg Bio. 29(5):1359–1376. doi:10.1111/gcb.16531.
- Peltola H, Kellomäki S, Väisänen H, Ikonen V-P. 1999. A mechanistic model for assessing the risk of wind and snow damage to single trees and stands of Scots pine, Norway spruce, and birch. Can J For Res. 29(6):647–661. doi:10.1139/x99-029.
- Petty JA, Worrell R. 1981. Stability of coniferous tree stems in relation to damage by snow. For Int J Forest Res. 54(2):115–128. doi:10.1093/forestry/54.2.115.
- Quante L, Willner SN, Middelanis R, Levermann A. 2021. Regions of intensification of extreme snowfall under future warming. Sci Rep. 11(1). doi:10.1038/s41598-021-95979-4.
- Quine CP, Gardiner B, Moore J. 2021. Chapter 4. Wind disturbance in forests: the process of wind created gaps, tree overturning, and stem breakage. In: Johnson EA, Miyanishi K, editor. Plant disturbance ecology. 2nd ed. San Diego: Academic Press; p. 117–184.
- Räisänen J. 2008. Warmer climate: less or more snow? Clim Dyn. 30(2–3):307–319. doi:10.1007/s00382-007-0289-y.
- Räisänen J. 2016. Twenty-first century changes in snowfall climate in Northern Europe in ENSEMBLES regional climate models. Clim Dyn. 46(1–2):339–353. doi:10.1007/s00382-015-2587-0.
- R Core Team. 2022. R: a language and environment for statistical computing. Version 4.2.2. R Foundation for Statistical Computing. https://www.R-project.org.
- Ruosteenoja K, Vihma T, Venäläinen A. 2019. Projected changes in European and North Atlantic Seasonal Wind Climate Derived from CMIP5 simulations. J Clim. 32(19):6467–6490. doi:10.1175/JCLI-D-19-0023.1.
- Sakakibara D, Nakamura Y, Kawashima K, Miura S. 2007. Experimental result for snow accretion characteristics of communications cable. Paper presented at the 56th International Wire and Cable Symposium (IWCS); Nov 11–14; Lake Buena Vista, Florida, US.
- Schelhaas M-J, Nabuurs G-J, Schuck A. 2003. Natural disturbances in the European forests in the 19th and 20th centuries. Glb Chg Bio. 9(11):1620–1633. doi:10.1046/j.1365-2486.2003.00684.x.
- Schroeder LM, Eidmann HH. 1993. Attacks of bark- and wood-boring coleoptera on snow-broken conifers over a two-year period. Scand J Forest Res. 8(1–4):257–265. doi:10.1080/02827589309382775.
- Seidl R, Thom D, Kautz M, Martin-Benito D, Peltoniemi M, Vacchiano G, Wild J, Ascoli D, Petr M, Honkaniemi J, et al. 2017. Forest disturbances under climate change. Nat Clim Change. 7(6):395–402. doi:10.1038/nclimate3303.
- Šilinskas B, Varnagirytė-Kabašinskienė I, Aleinikovas M, Beniušienė L, Aleinikovienė J, Škėma M. 2020. Scots pine and Norway spruce wood properties at sites with different stand densities. Forests. 11(5):587. doi:10.3390/f11050587.
- Skamarock WC, Klemp JB, Dudhia J, Gill DO, Liu Z, Berner J, Wang W, et al. 2021. A description of the advanced research wrf model version 4.3. In.
- Skogbrand Forsikringsselskap Gjensidig. 2023. Snøskader. [accessed 2023 May 5]. https://www.skogbrand.no/snoskader.
- Søgaard G, Astrup R, Allen M, Andreassen K, Bergseng E, Fløistad IS, Granhus A, et al. 2017. Skogbehandling for verdiproduksjon i et klima i endring. NIBIO Report 3/99. Ås: NIBIO.
- Solberg S, Granhus A, Zubkov P, Merlin M, Løge K. 2022. Hvordan forebygge vind- og snøskader på skog? Norsk Skogbruk (1).
- Solberg S, Kvaalen H, Puliti S. 2019. Age-independent site index mapping with repeated single-tree airborne laser scanning. Scand J Forest Res. 34(8):763–770. doi:10.1080/02827581.2019.1616814.
- Strasser U. 2008. Snow loads in a changing climate: new risks? Nat Hazard Earth Sys. 8(1):1–8. doi:10.5194/nhess-8-1-2008.
- Suvanto S, Lehtonen A, Nevalainen S, Lehtonen I, Viiri H, Strandström M, Peltoniemi M. 2021. Mapping the probability of forest snow disturbances in Finland. PLoS One. 16(7):e0254876. doi:10.1371/journal.pone.0254876.
- Suvanto S, Peltoniemi M, Tuominen S, Strandström M, Lehtonen A. 2019. High-resolution mapping of forest vulnerability to wind for disturbance-aware forestry. For Ecol Manag. 453:117619. doi:10.1016/j.foreco.2019.117619.
- Thiele C, Hirschfeld G. 2021. cutpointr: improved estimation and validation of optimal cutpoints in R. J Stat Softw. 98(11):1–27. doi:10.18637/jss.v098.i11.
- Thompson G, Eidhammer T. 2014. A study of aerosol impacts on clouds and precipitation development in a large winter cyclone. J Atmos Sci. 71(10):3636–3658. doi:10.1175/JAS-D-13-0305.1.
- Tucker CJ. 1979. Red and photographic infrared linear combinations for monitoring vegetation. Remote Sens Environ. 8(2):127–150. doi:10.1016/0034-4257(79)90013-0.
- Valinger E, Fridman J. 1997. Modelling probability of snow and wind damage in Scots pine stands using tree characteristics. For Ecol Manag. 97(3):215–222. doi:10.1016/S0378-1127(97)00062-5.
- Valinger E, Fridman J. 1999. Models to assess the risk of snow and wind damage in pine, spruce, and Birch forests in Sweden. Environ Manag. 24(2):209–217. doi:10.1007/s002679900227.
- Venäläinen A, Lehtonen I, Laapas M, Ruosteenoja K, Tikkanen O, Viiri H, Ikonen V, Peltola H. 2020. Climate change induces multiple risks to boreal forests and forestry in Finland: a literature review. Glb Chg Bio. 26(8):4178–4196. doi:10.1111/gcb.15183.
- Vestjordet E. 1967. Funksjoner og tabeller for kubering av stående gran, Meddelelser fra Det norske skogforsøksvesen 22. Vollebekk (Norway): Det norske skogforsøksvesen.
- Wickham H. 2009. Ggplot2: Elegant Graphics for Data Analysis. New York (US): Springer-Verlag New York.