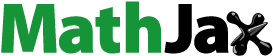
ABSTRACT
Public health spending (PHS) has been extensively investigated concerning population health outcomes (PHO), but its effectiveness has been less analysed. To this end, this study examines the association between domestic general government health expenditure (DGGHE) and PHO in terms of disability-adjusted life year (DALY) while testing the role of health system efficiency (HSE) in its effects. The under-five mortality(U5MR) is also used in the analysis to assess the effectiveness of services. The study uses publicly available data from 2008 to 2018 across forty-three countries in SSA and applies the GMM estimator based on linear and nonlinear moment conditions. The findings indicate that an increase in DGGHE was significantly associated with a decrease in DALY and U5MR, with the lowest decrease in countries with the lowest HSE scores. Therefore, the study urges governments in SSA countries to consider mechanisms that enhance health system efficiency while planning interventions based on increased spending on health.
1. Introduction
Developing countries are confronted with contradictory evidence regarding what matters for improving the health of their citizens. Many nations widely employ public health spending (PHS) to improve population health outcomes (PHO). They also use the same interventions to decrease catastrophic health spending and accelerate progress toward universal health coverage (Yardim et al., Citation2010; Rad et al., Citation2013).
Furthermore, numerous empirical studies suggest that investing in healthcare to improve the population's health may be less effective when factors such as country governance and health system efficiency are not conducive. For instance, empirical evidence supporting the notion that effective governance in low and middle-income countries contributes to greater efficacy in health expenditure is presented by Farag et al. (Citation2013). Likewise, the study conducted by Langnel & Buracom (Citation2020) demonstrates that substantial insights into the effectiveness of health spending can be gleaned from the governance levels observed in SSA (Langnel & Buracom, Citation2020). Kim and Wang (Citation2019) conducted research that emphasises the substantial influence of governance quality on the relationship between PHS and health outcomes. The authors specifically mention how improved governance enhances the positive impact of PHS on health outcomes. Arhin et al. (Citation2023) conclude, in a more comprehensive evaluation of health systems in Sub-Saharan Africa, that underscoring best practices within the health system can increase universal health coverage by a minimum of 19% using the existing health resources in the region (Arhin et al., Citation2023). In the health sector, the country's level of health system efficiency matters for interventions, such as increased PHS (Nketiah-Amponsah, Citation2019) to increase population health. Hence, in addition to increasing PHS, governments in sub-Saharan Africa (SSA) must boost the efficiency of their health systems to manage rising healthcare costs in light of the growing health needs of the population in this region. In the era of sustainable development goals (SDGs), where momentum for change is based on evidence (Allen et al., Citation2018), the availability of clearer evidence on the role of the HSE on the effectiveness of PHS is particularly important.
In the SSA region, PHS remains one of the main drivers for achieving the SDG3 targets (Le Blanc, Citation2015). SSA countries may need to resort to PHS because they no longer attract significant external health aid at a time when they record a high share of out-of-pocket health expenditure that restrains access to healthcare. A focus on PHS, particularly domestic general government health expenditure (DGGHE), appears necessary because DGGHE remains the only significant source for funding health care on a sustainable basis. After all, it is an internal government source of funding. DGGHE ensures optimal delivery and consumption of health services and financially protects the population by reducing catastrophic health expenditure and the cost burden of diseases (Rad et al., Citation2013).
Health funding needed to develop health is limited in the SSA region (Tandon & Cashin, Citation2010). In 2017, the region recorded the lowest per capita health expenditure of 84 U.S. dollars, compared to the global average of 1,065 US dollars. The average government spending on health as a share of general government spending is around 7.2%, despite the 2011 Abuja commitment suggesting allocating 15% of the state budget to health (WHO, Citation2011). While the SSA region has experienced poor PHO for many years and lags in achieving the UHC and the health-related sustainable development goals (WHO, Citation2020), studies found that the key issue is that the low health funds available in the region do not achieve the expected health outcomes (Dieleman et al., Citation2018; Micah et al., Citation2019). depicts the association between DGGHE and DALY (Disability-Adjusted Life Years) in SSA countries in 2018.
Figure 1. Association between DGGHE and the DALY in SSA countries, 2018.
Note. The figure depicts the association between DGGHE and the DALY in 2018 in SSA countries. World development indicators and Global Burden of Disease databases. Copyrights by World Bank and Institute for Health Metrics Evaluation.
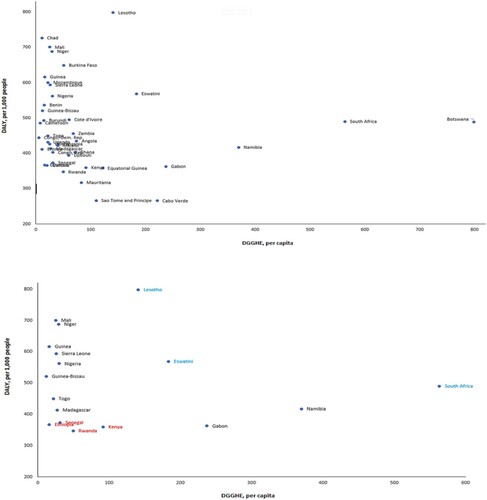
shows that a few countries, namely Ethiopia, Senegal, and Rwanda exhibit a DGGHE significantly lower than the average of $94 per capita. Despite this situation, these countries achieve a DALY level below the average of 471 DALYs per 1,000 people. It is worth noting that although the majority of countries do not attain a lower level of DALY, there are several countries, including South Africa, Eswatini, and Lesotho, that exhibit a much higher DGGHE than the norm, resulting in the much above-average DALY levels. The above circumstances raise concerns regarding the utilisation of PHS in the context of SSA, requiring an investigation to ascertain the factors that affect the effectiveness of PHS. Therefore, beyond the impact analysis of PHS on PHO, an in-depth investigation of the potential factors that affect the effectiveness of PHS in improving PHO appears important.
Since the 1990s, interest in assessing health expenditures at the macro level has increased. The relationship between health spending and population health has been one of the topics of interest most assessed (Crémieux et al., Citation1999; Berger & Messer, Citation2002; Kringos et al., Citation2013; Onofrei et al., Citation2021). In contrast to developed regions, only a few studies have attempted to evaluate the relationship between PHS and PHO in developing regions, especially SSA (Filmer & Pritchett, Citation1999; Lawanson, Citation2012; Nyamuranga & Shin, Citation2019). The exact relationship between PHS and PHO is ambiguous in SSA, as empirical evidence generally appears inconclusive. Some studies conducted in SSA have proven the effectiveness of PHS in improving PHO (Gupta et al., Citation2002; Rajkumar & Swaroop, Citation2008; Novignon et al., Citation2012; Novignon & Lawanson, Citation2017), but others have not (Filmer & Pritchett, Citation1999; Kamiya, Citation2010; Popoola, Citation2018; Akinlo & Sulola, Citation2019). Most of these studies have only investigated the ultimate impact of the PHS on PHO as measured by mortality-based indicators, such as under-five mortality and life expectancy. Doing so may result in distorted findings.
Performing the impact analysis reflects the focus of the studies on the ultimate effects of spending on the outcomes. Whilst giving some insights on the role of spending on health outcomes, these studies have ignored the possibility that the effects of spending, as a predictor variable, may be affected by some factors in such a way as to change the direction or the magnitude of the relationship between the outcomes and the predictors. Some findings in past literature corroborate this statement. For example, investigating the effect of government health spending on PHO measured by infant and under-5 mortality, the study by Farag et al. (Citation2013) found differing findings in the presence and absence of government effectiveness, as they found that improvements in government effectiveness enhance the effect of government health spending (Farag et al., Citation2013). Another study by Kim and Wang (Citation2019) investigated the moderating effect of quality of government on the relationship between the quantity of governance measured by PHS and the PHO measured by the mortality-based indicators. They also found differing results in the presence and absence of the quality of governance. They found that the quality of government plays a role in moderating the relationships between the quantity of government and the PHO (Kim & Wang, Citation2019). Findings from the above studies show that when exploring the link between PHS and PHO, it is important to assess factors affecting the nature of this relationship since this could provide information on why PHS is ineffective in improving PHO.
Furthermore, estimating the effects of PHS on mortality-based PHO indicators may be misleading, as it only counts a portion of the benefits of health spending. For example, an investigation by disease area revealed that more than half of health spending in the United Kingdom was spent on diseases for which no mortality data was documented (Claxton et al., Citation2015). This type of health spending is likely expected to affect health, not through mortality reduction only but also through increased health-related quality of life. Using DALY as a measure of PHO is appropriate as it accounts for both the mortality and morbidity disease burden that can be addressed by health spending.
Moreover, by using DALYs as health outcomes, it is possible to estimate the influence of health spending on a health measure that encompasses survival gains and health-related quality of life (Ochalek et al., Citation2020). Investigating the link between PHS-PHO, focusing only on the ultimate effects on PHO and using mortality-based indicators of PHO, may yield information that makes it challenging to infer the policy imperatives needed to improve PHO. Another issue with prior research is that most studies ignored the dynamic aspect of the relationship between PHS and PHS, the endogeneity, and the unobserved individual effects. This situation may also result in biased model estimates (Baltagi, Citation2021).
This study explores the link between DGGHE and DALY while tackling the abovementioned issues. To the best of our knowledge, this is the first study conducted in SSA to examine the impact of DGGHE on DALY while testing HSE as a moderating factor. Given this, the study addresses two questions: (1) does DGGHE significantly impact DALY? and (2) Does HSE reduce or boost the effect of DGGHE on DALY? This study investigates whether the PHS-PHO hypothesis holds in the SSA region and whether HSE moderates the nexus of PHS-PHO. In other words, the study examines whether and to what extent DGGHE affects DALY and whether HSE affects this relationship in the SSA countries. The study hypothesises that an increase in DGGHE decreases DALY and that the impact of DGGHE on DALY increases when the level of HSE is high. The GMM estimator Ahn and Schmidt (Citation1995) proposed based on linear and nonlinear moment conditions was applied to panel data from 2008 to 2018 from 43 SSA countries sourced from publicly available databases to achieve the study objective.
The potential contributions of this study in answering the questions above are diverse. Instead of focusing on the effects of PHS on PHO, this study examines the factors that influence the strength or amplitude of the link between PHS and PHO. Second, whereas prior studies typically used health system efficiency (HSE) scores to investigate the determinants of a country's health system efficiency, this study employs HSE scores as moderators of the association between PHS and PHO. The study suggests that the health system, as a continuum of multiple factors, may influence this relationship when all these factors are utilised efficiently since these factors affect either PHO or PHS. The World Health Organisation defines the health care system as ‘all actions whose primary purpose is to promote, restore, or maintain health’ (WHO, Citation2000).
Consequently, the health system can either increase or decrease PHO (Nolte & McKee, Citation2004), as it is a framework in which PHOs are produced due to multiple inputs, including health spending. Thirdly, this study measured PHO by DALY, a composite summary measure of population health. This indicator provides a more comprehensive population health state than mortality-based indicators such as U5M, newborn mortality, maternal mortality, and life expectancy indicators, which are commonly used. Although mortality-based indicators are helpful(and indeed, the U5M is used in this study to assess variation in results), they do not give all the information required to assess the health of a population or compare the effectiveness of interventions designed to protect and promote health (Gold et al., Citation2002). For instance, they do not account for the effects of being ill for potentially many years before death or recovery. Last, a GMM estimator is used in this study to deal with the endogenous link between PHS and PHO.
The remainder of this article is organised as follows: Section 2 includes the study data and methodology. All results are provided in Section 3. A discussion of the results and research prospects is conducted in Section 4. Finally, Section 5 provides the conclusion and a policy recommendation.
2. Data and methodology
2.1. Data
2.1.1. Dataset description
The paper examines a panel of forty-three sub-Saharan African countries from 2008 to 2018 subject to data availability. Data were derived from the Global Health Data Exchange (GHDex) and World Development Indicators (WDI) databases (IHME, Citation2022; World Bank, Citation2022). The Data on Disability-Adjusted Life Years (DALY) were extracted from the GHDex database, and the remaining data, except for health system efficiency, were extracted from the WDI database. Health system efficiency was measured by the technical efficiency scores (TES) generated by applying the Stochastic Frontier Approach (SFA). Because DALY is a negative health outcome and the health systems are not set to produce negative outputs, the study used healthy life expectancy (HALE), a summary measure of population health (SMPH), as an output in estimating efficiency score. HALE was regressed on current health expenditures, gross domestic product, and urban population rates. All the variables were in logarithmic form. TES, measures of HSE, were extracted from the regression residuals. The study applied the estimator for the endogenous panel stochastic production frontier model suggested by Karakaplan (Citation2022), considering the output-oriented efficiency measurement. and present the SFM estimates from the Karakaplan estimator and the summary of efficiency scores for the study period, respectively.
Table 1. Karakaplan estimator estimates for the SF model.
Table 2. Summary of efficiency scores for 2008–2018.
describes the variables included in the main analysis, where DALY was used as the dependent variable instead of the usual mortality-related indicators of PHO.
Table 3. Description of variables.
2.1.2. Dependent variable
The dependent variable in this study is the DALY of countries. As in the study by Dhimal et al., this variable is used as a proxy for PHO (Dhimal et al., Citation2021). It is a well-accepted proxy for PHO because it accounts for mortality and morbidity.
2.1.3. Independent variables
This study employed two main independent variables, DGGHE and HSE. DGGHE per capita is an important indicator of how governments spend on health to allow an individual to access health care (Micah et al., Citation2019; Jordi et al., Citation2020). An increase in DGGHE is expected to reduce DALY. Following the study by Zhou, health system efficiency scores were included as an independent variable because they are well known to be associated with improved health outcomes (Zhou, Citation2018). An increase in HSE is expected to lower DALY.
2.1.4. Control variables
The study's basic approach was to ensure control for factors such as malaria, major sicknesses peculiar to African nations, education, government prioritisation, and origin of healthcare funds, which are known to influence the population's health in SSA. Based on the literature, these factors were measured respectively by the following selected variables: Incidence of malaria (Kioko et al., Citation2013), female school enrolment (Mirowsky & Ross, Citation2017), DGGHE share of government expenditures, indicating the government's priority to spend on health out of its own domestic public resources (Ithibu & Amendah, Citation2019) and DGGE share of GDP, indicating the fiscal space for health. However, not all of it is under State control. (Ssozi & Amlani, Citation2015; Ithibu & Amendah, Citation2019). However, the study used the variables CO2 emissions, life expectancy at birth, Out-of-pocket expenditure per capita and GDP as instruments for the regression.
2.2. Study framework
The framework developed by Baron and Kenny was adapted and used to conduct the moderation analysis (Baron & Kenny, Citation1986). presents the study framework, which includes three causal paths that feed into the outcome variable: Path a: the impact of DGGHE (first main predictor), Path b: the impact of HSE scores (second main predictor), and Path c: the impact of the interaction term (DGGHE and HSE scores) that measures the moderating effect of HSE. The fourth causal path representing the impacts of controlled variables is not presented for simplicity. The moderation hypothesis is supported if the interaction term is significant.
Figure 2. Graphical representation of the moderation hypothesis.
Note. The arrows pointing to the DALY represent the path coefficients that measure the impacts of DGGHE, health system efficiency, and the interaction term on the DALY. The interaction term variable is generated as the product of DGGHE and health system efficiency scores. The moderation hypothesis (H2) would be supported if the interaction term were significant. For simplicity, the graph does not present the control variable impacts. Adapted from ‘The moderator–mediator variable distinction in social psychological research: Conceptual, strategic, and statistical considerations,’ by Baron and Kenny, 1986, Journal of personality and social psychology, 51(6), p. 1173. Copyright 1986 by the American Psychological Association, Inc.

2.3. Empirical model
The empirical model utilised in this study was derived from a proposed model by Aiken and West (Citation1991). EquationEquation 1(2)
(2) represents the single regression equation originally developed by Aiken and West.
1)where denotes the coefficient that measures the extent of the association between the independent variable, X, and the dependent variable, Y, while the moderator variable, Z, equals 0. In a similar vein, the coefficient
is used to quantify the association between the moderator variable Z and the outcome variable Y when X equals 0.
denotes the intercept in the equation, whereas
is the model residual. The regression coefficient
is an estimation of the moderating effect of the interaction term. If the coefficient
exhibits statistical significance, indicating a significant moderating effect on the dataset's relationship between X and Y.
EquationEquation 1(2)
(2) was adapted to include the study variables and the country
and period
related subscripts. The resulting study empirical model is presented in Equation 2, which follows.
(2)
(2) Where DALY is the outcome variable, DGGHE and HSE are the two main independent variables, MALA is the incidence of malaria, FEMED is female school enrolment, DHHE_GGE is the DGGHE share of government expenditures, and DGGHE_GDP is the DGGE share of GDP.
is the intercept, and
to
are the coefficients for DGGHE, HSE, interaction term, MALA, FEMED, DGGHE_GGE, and DGGHE_GDP, respectively.
the error term represents unobserved factors or random shocks that affect DALY but are not included in the model.
2.4. Estimation technique
The GMM estimator proposed by Ahn and Schmidt in their seminal 1995 publication titled ‘Efficient Estimation of Models with Conditional Moment Restrictions Containing Unknown Functions’ was employed in this study. This estimator is a component of the System-GMM framework. This estimator enables handling common causes of endogeneity, including simultaneity, measurement error, omitted variable bias, endogenous regressors, dynamic panel data issues, heteroscedasticity, and autocorrelation. This study seeks to address endogeneity resulting from measurement error and endogenous regressors. The data quality issue in SSA is uncertain since there is the potential for measurement errors in recording health outcomes and health spending. The healthcare infrastructure and data collection processes confront several challenges that result in data being missing, inaccurately reported, or inconsistently measured across different countries (Makuta & Ohare, Citation2015). Endogeneity related to endogenous regressors includes reverse causality, where the relationship between PHO and PHS is often bidirectional (Aissaoui et al., Citation2022).
Additionally, endogeneity can arise through technological advancements, as developments in medical technology can impact both PHO and PHS (Hillestad, Citation2005; Khan & AlAjmi, Citation2013). Lastly, income can also influence endogeneity, as wealthier countries often exhibit better PHO and may allocate more public resources towards health (Freeman et al., Citation2020). Because it involves both differenced and level moment conditions, this estimator addresses the issues of endogeneity and serial correlation (Baltagi, Citation2021).
Moreover, the efficiency of this method is reached when the number of cross-sections exceeds the number of periods, noted N > T (Arellano & Bond, Citation1991). The study applied the GMM for estimating linear dynamic panel data models based on linear and nonlinear moment conditions, as Ahn and Schmidt (Citation1995) suggested. The nonlinear moment conditions improve efficiency when added to the difference-GMM moment conditions (Ahn & Schmidt, Citation1995). While these moment conditions are redundant when combined with the system-GMM moment conditions (Blundell & Bond, Citation1998), they remain valid even when the system-GMM level model moment conditions are not. Additionally, whereas GMM estimators with only linear moment conditions have a closed-form solution, this is not the case when nonlinear moment conditions are present. To assess the consistency of their GMM estimator, Ahn and Schmidt (Citation1995) rely on two specification tests: the Arellano–Bond and Sargan-Hansen tests (Arellano & Bond, Citation1991). The Arellano–Bond test for serial correlation [AR(2)] is adapted to test whether there is a second-order serial correlation in the first-differenced residuals. The null hypothesis is that the residuals are serially uncorrelated. If the null hypothesis cannot be rejected, it provides evidence that there is no second-order serial correlation and the GMM estimator is consistent. The Sargan-Hansen test is then applied to test the null hypothesis of the overidentifying restrictions (Hansen, Citation1982; Sargan, Citation1988). Failure to reject this null hypothesis means the instruments are valid and the model specification is accurate. To assess the moderating effect on the DALY-DGGHE relationship, this study used the interaction of DGGHE and HSE scores and applied the moderation approach suggested by Aiken & West (Citation1991), which has been used recently by (Kubo et al., Citation2021; Pham et al., Citation2021). Three steps summarise this approach: (1) estimating the models with and without the interaction term and verifying the significance and goodness of the model with the interaction compared with the model without the interaction term, and (2) conducting the moderation assessment if the model with interaction is significant, The moderation model has to include mean-centered variables (Dalal & Zickar, Citation2012; Dawson, Citation2014), and (3) plotting the interaction points and interpreting the interaction effect. Detailed descriptions of moderator effects and a framework for their estimation and interpretation are presented in Aiken & West (Citation1991). All the analyses were performed with version 16 of the Stata software (StataCorp, Citation2019), where the XTDPDGMM package (Karakaplan & Kutlu, Citation2017; Karakaplan, Citation2018) was used to estimate the study model.
3. Study results
3.1. Descriptive analysis
The descriptive statistics on the main variables included in the econometric models are presented in . Due to time and space constraints, three main variables are addressed.
Table 4. Descriptive statistics.
The mean of DALY in the study sample over the study period is 559 DALYs per 10,000 people, with a standard deviation (S.D.) of 162 DALYs per 10,000 people, respectively. DGGHE per capita had a mean of $113.5 (PPP, constant 2017 international). Health system efficiency scores present a mean value of 0.68. The high S.D. on DGGHE shows wide variations in this variable among countries involved in the study. For example, countries like the Democratic Republic of Congo and Guinea recorded the lowest values of about $2.44 and $4.75, respectively. In contrast, countries like Botswana and Seychelles recorded the highest values of $800.81 and $1,040.55, respectively.
reveals correlations between some major study variables. As expected, DGGHE per capita is negatively correlated with DALY. However, HSE scores are not significantly correlated with DALY. DALY was used as the dependent variable instead of the usual mortality-related indicators of PHO. Other variables were selected according to the literature and the availability of the data.
Table 5. Correlation between main model variables.
3.2. Model estimates
Following the application of the moderation approach suggested by Aiken and West (Citation1991):
Step 1: The restricted (Model 1) and unrestricted (Model 2, including interaction term) models were estimated, excluding control variables. Findings are presented in . The regression between the two models provides a basis for testing Hypothesis 1 of the study. In both models, the coefficient on DGGHE is negative and statistically significant at a 5% level, suggesting that DGGHE is negatively related to DALY. HSE scores are not significant in either model. Compared to Model 1, the magnitude of the coefficient on DGGHE is greater in Model 2 (b = −0.504; p = 0.023) compared to Model 1 (b = −0.167; p = 0.045). The interaction term (−0.881) included in Model 2 is statistically significant at a 10% level. These findings suggest that Model 2 is better than Model 1, as including the interaction term improves the model.
Table 6. Ahn and Schmidt estimator results.
Table 7. Estimates of model and moment selection criteria.
Step 2: The unrestricted model (Model 2) estimated above appears better than the restricted model (Model 1). The unrestricted model provides two main pieces of information. First, it corroborates the negative relationship (found in Model 1) between DALY and DGGHE as it indicates that a percentage change in DGGHE is associated with a 0.504 per cent decrease in DALY in the short run at the 5% significance level, on average ceteris paribus. Hence, DGGHE and DALY exhibit an inelastic relationship. Second, the unrestricted model suggests a potential moderating effect that should exist in the relationship between DALY and DGGHE. The estimates of the moderation model (Main Model) with mean-centred predictors, including some control variables (Kromrey & Foster-Johnson, Citation1998; Iacobucci et al., Citation2016), are presented in the second column in .
To ascertain the validity of the analysis of DALY at the population level concerning health spending, this study employed U5MR to estimate the model presented in column three of . The model mentioned above, referred to as the Model with U5MR, included the U5MR as the outcome variable while maintaining the same independent variables as the Main Model. Focusing on the variables of interest, specifically the DGGHE, HSE, and interaction variable, it is seen that the model produces results that align with the main model but with somewhat varying magnitudes.
Step 3: The study computed () the marginal effect of DGGHE at differing levels of HSE scores. At HSE-scores of 0, the coefficient on DGGHE equals to −0.230 (p = 0.089); at HSE-scores 1 standard deviation lower than the mean, the coefficient on DGGHE equals to −0.052 (p = 0.760); and at HSE-scores 1 standard deviation higher than the mean, the coefficient on DGGHE equals to −0.408 (p = 0.007). indicates that at all levels of HSE scores lower than one standard deviation from the mean, the coefficient or marginal effect of DGGHE is statistically insignificant. In comparison, it is significant at all levels of HSE scores higher than one standard deviation from the mean.
Table 8. Ahn and Schmidt estimator results for the moderation model (Dep. Var. DALY, log).
Table 9. Estimates of model and moment selection criteria.
Table 10. DGGHE marginal effects.
Table 11. Moderator marginal effects.
This situation is depicted in Figure A1, which presents different curves of marginal effects of DALY from DGGHE resulting from the differing levels of HSE scores (minimum, - 1SD, 0, + 1SD, + 2SD, maximum), which correspond to different values of the DGGHE coefficients (). Only the curves of marginal effects of DALY at the points where the coefficients of DGGHE (resulting from the levels of HSE) are statistically significant, indicating that DALY is decreasing due to increases in DGGHE. It can be observed that the slope of the DALY marginal effects goes from positive at the minimum value of HSE scores (although insignificant) to slightly negative at the mean value before becoming significantly steeper, suggesting a greater impact of DGGHE in reducing DALY. This indicates that the moderator strengthens as the relationship between DALY and DGGHE strengthens with the increase in HSE scores. The strengthening nature of the moderation is supported by the fact that all the coefficients for DGGHE, HSE scores, and the moderating variable presented in are negative (Gardner et al., Citation2017).
The study performed a sensitivity analysis to assess the robustness of the findings. The following adjustments were made to the main model (Moderation Model). First, the model instruments were reduced from 35 to 33 to estimate the reduced instrument model, as Roodman (Citation2009) suggested. This study opted to slightly reduce the number of model instruments to ensure that the remaining instruments were pertinent, valid, and suitable for the model identification. This adjustment caused a slight decrease in the DGGHE coefficient from −0.348 to −0.347, which remains statistically significant at a 10% level, and a slight increase in the interaction term from −0.754 to −0.764, which also remains statistically significant at a 5% level. Then, the constant term was dropped from the main model to estimate the no-constant model. The motivation behind this investigation was to realise how sensitive the study results are to the exclusion of the constant term. In econometrics, it is typical to include or exclude a constant term to assess the potential impact on the estimated coefficients and the model's overall fit (Stock & Watson, Citation2019). This model did not alter the parameters of interest from the main model. Findings from these above adjustments to the main model suggest the study results are robust. (Roodman, Citation2009)
4. Discussions
PHS is one of the essential tools for enhancing PHS and achieving health-related SDGs worldwide, especially in SSA, where the precise relationship between PHS and PHO is unclear and requires further investigation. This study aimed to examine the relationship between PHS and PHO, as measured respectively by DGGHE and DALY, emphasising the factors that influence this relationship. This study shows that DGGHE positively reduces DALY in SSA countries and that the HSE moderates this effect.
First, this study's empirical analysis demonstrates that, on average, the relationship between DALY and DGGHE is statistically significant, with a negative impact across the 43 SSA countries for the study period. The analysis indicates an inelastic DGGHE elasticity of −0.348, supporting the study Hypothesis 1, which suggests an increase in DGGHE is related to a decrease in DALY. This was expected as most health facilities in SSA are publicly owned and funded. In this regard, DGGHE, as public health spending, is more likely to impact a greater proportion of the population than private health spending because it is funded by tax dollars and is intended to provide healthcare services to all members of society, regardless of their ability to pay.
These findings are consistent with previous research in the field conducted in SSA, which has also shown similar results and found PHS elasticities ranging from −0.110 to −0.380. (Gupta et al., Citation2002; Farag et al., Citation2013; Novignon & Lawanson, Citation2017). This suggests that the phenomenon observed in this study is not an isolated occurrence but rather a common trend. In contrast, Nicholas et al. (Citation2016) analysis, which used mortality-related indicators, shows that their findings present a different perspective on the impact of PHS on population health. Their study suggests that PHS has no significant effect on maternal mortality in SSA (Nicholas et al., Citation2016),
Second, the results from the current study also reveal that DGGHE and HSE scores interact to predict DALY. The moderating term coefficient of −0.754 (SD = 0.371), which is statistically significant at the 5 per cent level, is consistent with the study Hypothesis 2 that HSE scores affect the relationship between DGGHE and DALY. The study results suggest that the moderator (HSE scores) strengthens as the impact of DGGHE on DALY becomes stronger with the increase in HSE scores. Although the increase in DGGHE reduces DALY for all countries under study, countries on the verge of beginning the implementation of efficiency in the health system will not record more reductions in DALY as a result of increases in DGGHE compared to those countries with an increased level of HSE, i.e. countries with low HSE scores experience a wastage of health resources mobilised through increased DGGHE. These findings align with previous research investigating the impact of HSE on PHO and PHS. Previous studies have consistently shown that countries with higher health system efficiency tend to have better population health outcomes and lower public health spending (Sajadi et al., Citation2020; Arhin et al., Citation2023). These findings further support the notion that improving health system efficiency can improve population health and lead to a more cost-effective use of public health resources. However, it is important to note that no prior study has explored the moderating role of HSE on the link between PHS and PHO, as does this study.
There is considerable value in determining whether interactions have meaningful effects for certain ranges of data points in a sample, individuals or countries (Murphy & Russell, Citation2017). Identifying the range of the moderator for which the predictor-outcome slope is significantly different from zero may allow us to measure the efforts to improve the moderator to make the predictor attain the expected outcome. Several techniques developed (computational tools and visualisations) have proven useful, even to understand better the meaning of interaction effects (Murphy & Aguinis, Citation2022). For example, suggests that DGGHE is relevant to DALY only for those countries with a standard deviation or more below the mean in HSE scores. This is useful as it can show efforts (in terms of increasing HSE scores) to develop to get to the level where DGGHE should effectively reduce DALY. In addition, the study pointed out other factors, such as malaria incidence and DGGHE share of GDP, suggesting that they cannot be overlooked as contributing factors to the reduction of DALY (Bloom & Canning, Citation2008; Girum et al., Citation2019). It must be noted that while the findings of the current study provide evidence in support of increasing DGGHE and improving efficiency in the health system, this may only be a necessary but not sufficient condition as achieving a decline in DALY may depend on other factors not included in this study because of the lack of data at the country-level. The study is limited because some interesting control variables for SSA, such as the density of healthcare professionals, water and sanitation, health behaviours, and bed population ration, did not have enough time series observations, which would have improved a panel data study like this one. The proxy used for health outcomes may not be exhaustive as it captures only negative and not positive outcomes, such as healthy life expectancy, another summary measure of population health. Although these limitations may serve as the basis for future research, they do not invalidate the current study's findings.
5. Conclusion
This study aims to examine the impact of DGGHE on DALY and assess the moderating effect of health system efficiency in the SSA countries. The study results indicate that DGGHE significantly reduces DALY across all the models specified in the study. The study results also indicate a synergistic effect of DGGHE and HSE in reducing DALY, i.e. improved HSE scores reinforce the effect of DGGHE. The HSE thus plays a moderating role, influencing the effectiveness of DGGHE in reducing DALY since the impact of DGGHE is greater on DALY in countries with high HSE scores and lower in countries with low HSE scores. These results explain the non-existent, insignificant or extremely small impact of public health spending recorded in some countries, namely that the inefficiency of public health spending may be the result of incredibly low HSE scores since the production of PHO takes place in the countries’ health systems. This study uncovers unambiguous evidence of the significant beneficial effects of macro-level PHS on PHO when HSE scores are higher. However, several additional factors should be considered when examining the efficacy of DGGHE. The literature indicates that health care (through which PHS impacts PHO) accounts for only about 28% of the PHO improvement (Park et al., Citation2015).
The findings of this study have significant policy implications, particularly for policy and decision-makers in the Sub-Saharan Africa (SSA) region. They must prioritise improving health system efficiency as an integral component of their public health policies. To address specific inefficiencies within health systems, the study recommends targeted strategies which may involve promoting initiatives that enhance the organisation and delivery of services. The study further advocates for governments to implement comprehensive reforms, drawing upon evidence-based practices and considering each country's situation. It is anticipated that this strategy would result in more effective resource allocation and sustainable enhancements in health outcomes, therefore ensuring that public health spending is aligned with equitable health improvements in various contexts within the SSA region.
Authors contribution
WNSK conceptualised the manuscript, conducted data analysis and produced the first draft.
JM conceptualised the manuscript, restructured and reviewed the various versions of the manuscript.
Ethics approval
The Ethics Committee of the University of KwaZulu-Natal approved this study.
Data availability
The data that support the findings of this study are available upon request from the corresponding author Josue Mbonigaba, [email protected] +27768706031.
Disclosure statement
No potential conflict of interest was reported by the author(s).
Correction Statement
This article has been corrected with minor changes. These changes do not impact the academic content of the article.
References
- Ahn, SC & Schmidt, P, 1995. Efficient estimation of models for dynamic panel data. Journal of Econometrics 68(1), 5–27.
- Aiken, LS & West, SG, 1991. Multiple regression: Testing and interpreting interactions. Sage Publications, Inc.
- Aissaoui, N, Hamaizia, L, Brika, SKM & Laamari, A, 2022. The effectiveness of health care finance in promoting health: Does the condition of health get better by spending more? Pan African Medical Journal 42.
- Akinlo, AE & Sulola, AO, 2019. Health care expenditure and infant mortality in sub-Saharan Africa. Journal of Policy Modeling 41(1), 168–78.
- Allen, C, Metternicht, G & Wiedmann, T, 2018. Initial progress in implementing the Sustainable Development Goals (SDGs): A review of evidence from countries. Sustainability Science 13(5), 1453–67.
- Andrews, DW & Lu, B, 1999. Consistent model and moment selection criteria for GMM estimation with application to dynamic panel data models.
- Arellano, M & Bond, S, 1991. Some tests of specification for panel data: Monte Carlo evidence and an application to employment equations. The Review of Economic Studies 58(2), 277–97.
- Arhin, K, Oteng-Abayie, EF & Novignon, J, 2023. Assessing the efficiency of health systems in achieving the universal health coverage goal: Evidence from Sub-Saharan Africa. Health Economics Review 13(1), 1–16.
- Baltagi, B, 2021. Econometric analysis of panel data. 6th edition. Springer, Switzerland.
- Baron, RM & Kenny, DA, 1986. The moderator–mediator variable distinction in social psychological research: Conceptual, strategic, and statistical considerations. Journal of Personality and Social Psychology 51(6), 1173.
- Berger, MC & Messer, J, 2002. Public financing of health expenditures, insurance, and health outcomes. Applied Economics 34(17), 2105–13.
- Bloom, DE & Canning, D, 2008. Population health and economic growth. Health and Growth 53, http://hdl.handle.net/10986/28036.
- Blundell, R & Bond, S, 1998. Initial conditions and moment restrictions in dynamic panel data models. Journal of Econometrics 87(1), 115–43.
- Claxton, K, Martin, S, Soares, M, Rice, N, Spackman, E, Hinde, S, Devlin, N, Smith, PC & Sculpher, M, 2015. Methods for the estimation of the national institute for health and care excellence cost-effectiveness threshold. Health Technology Assessment 19, 1.
- Crémieux, PY, Ouellette, P & Pilon, C, 1999. Health care spending as determinants of health outcomes. Health Economics 8(7), 627–39.
- Dalal, DK & Zickar, MJ, 2012. Some common myths about centering predictor variables in moderated multiple regression and polynomial regression. Organizational Research Methods 15(3), 339–62.
- Dawson, JF, 2014. Moderation in management research: What, why, when, and how. Journal of Business and Psychology 29(1), 1–19.
- Dhimal, M, Chirico, F, Bista, B, Sharma, S, Chalise, B, Dhimal, ML, Ilesanmi, OS, Trucillo, P & Sofia, D, 2021. Impact of air pollution on global burden of disease in 2019. Processes 9(10), 1719.
- Dieleman, JL, Haakenstad, A, Micah, A, Moses, M, Abbafati, C, Acharya, P, Adhikari, TB, Adou, AK, Kiadaliri, AA & Alam, K, 2018. Spending on health and HIV/AIDS: Domestic health spending and development assistance in 188 countries, 1995–2015. The Lancet 391(10132), 1799–829.
- Farag, M, Nandakumar, A, Wallack, S, Hodgkin, D, Gaumer, G & Erbil, C, 2013. Health expenditures, health outcomes and the role of good governance. International Journal of Health Care Finance and Economics 13(1), 33–52.
- Filmer, D & Pritchett, L, 1999. The impact of public spending on health: Does money matter? Social Science & Medicine 49(10), 1309–23.
- Freeman, T, Gesesew, HA, Bambra, C, Giugliani, ERJ, Popay, J, Sanders, D, Macinko, J, Musolino, C & Baum, F, 2020. Why do some countries do better or worse in life expectancy relative to income? An analysis of Brazil, Ethiopia, and the United States of America. International Journal for Equity in Health 19(1), 1–19.
- Gardner, RG, Harris, TB, Li, N, Kirkman, BL & Mathieu, JE, 2017. Understanding “It depends” in organizational research. Organizational Research Methods 20(4), 610–38.
- Girum, T, Shumbej, T & Shewangizaw, M, 2019. Burden of malaria in Ethiopia, 2000-2016: Findings from the Global Health Estimates 2016. Tropical Diseases, Travel Medicine and Vaccines 5(1), 1–7.
- Gold, MR, Stevenson, D & Fryback, DG, 2002. HALYS and QALYS and DALYS, Oh My: Similarities and differences in summary measures of population Health. Annual Review of Public Health 23(1), 115–34.
- Gupta, S, Verhoeven, M & Tiongson, ER, 2002. The effectiveness of government spending on education and health care in developing and transition economies. European Journal of Political Economy 18(4), 717–37.
- Hansen, LP, 1982. Large sample properties of generalized method of moments estimators. Econometrica, 1029–54.
- Hillestad, R, 2005. Can electronic medical record systems transform health care? potential health benefits, savings, And costs. Health Affairs 24(5), 1103–17.
- Iacobucci, D, Schneider, MJ, Popovich, DL & Bakamitsos, GA, 2016. Mean centering helps alleviate “micro” but not “macro” multicollinearity. Behavior Research Methods 48(4), 1308–17.
- IHME, Institute For health metrics and evaluation, 2022. Global Burden of Disease Study 2022 Data Input Sources Tool | GHDx. Institute for Health Metrics Evaluation. http://ghdx.healthdata.org/gbd-2019/data-input-sources.
- Ithibu, A & Amendah, D, 2019. Domestic financing for HIV, T.B. and malaria in Global Fund High Impact Asia countries. https://aidspan.org/download/domestic-financing-for-hiv-tb-and-malaria-in-global-fund-high-impact-asia-countries-2/#https://aidspan.org/download/domestic-financing-for-hiv-tb-and-malaria-in-global-fund-high-impact-asia-countries-2/#.
- Jordi, E, Pley, C, Jowett, M, Abou Jaoude, GJ & Haghparast-Bidgoli, H, 2020. Assessing the efficiency of countries in making progress towards universal health coverage: A data envelopment analysis of 172 countries. BMJ Global Health 5(10), e002992.
- Kamiya, Y, 2010. Determinants of health in developing countries: Cross-country evidence. No 10E009, OSIPP Discussion Paper, Osaka School of International Public Policy, Osaka University. https://www.osipp.osaka-u.ac.jp/archives/DP/2010/DP2010E009.pdfhttps://www.osipp.osaka-u.ac.jp/archives/DP/2010/DP2010E009.pdf.
- Karakaplan, M, 2018. xtsfkk: Stata Module for Endogenous Panel Stochastic Frontier Models. Retrieved 53 from Boston College, Department of Economics, Statistical Software Components (SSC) S458445.
- Karakaplan, Mustafa U, 2022. Panel stochastic frontier models with Endogeneity. The Stata Journal 22(3), 643–663. https://doi.org/10.1177/1536867X221124539.
- Karakaplan, MU & Kutlu, L, 2017. Endogeneity in panel stochastic frontier models: An application to the Japanese cotton spinning industry. Applied Economics 49(59), 5935–9.
- Khan, S & AlAjmi, MF, 2013. Impact of medical technology on expansion in healthcare expenses. International Journal of Advanced Computer Science and Applications 4(4), https://thesai.orghttps://thesai.org.
- Kim, S & Wang, J, 2019. Does quality of government matter in public health?: Comparing the role of quality and quantity of government at the National Level. Sustainability 11(11), 3229.
- Kioko, UM, Mwabu, G & Kimuyu, P, 2013. The economic impact of malaria on wage earnings in Kenya: A household level investigation. International Journal of Educational Research 1(4), http://hdl.handle.net/11295/39535http://hdl.handle.net/11295/39535.
- Kringos, DS, Boerma, W, van der Zee, J & Groenewegen, P, 2013. Europe's strong primary care systems are linked to better population health but also to higher health spending. Health Affairs 32(4), 686–94.
- Kromrey, JD & Foster-Johnson, L, 1998. Mean centering in moderated multiple regression: Much ado about nothing. Educational and Psychological Measurement 58(1), 42–67.
- Kubo, T, Sugawara, D & Masuyama, A, 2021. The effect of ego-resiliency and COVID-19-related stress on mental health among the Japanese population. Personality and Individual Differences 175, 110702.
- Langnel, Z & Buracom, P, 2020. Governance, health expenditure and infant mortality in sub-Saharan Africa. African Development Review 32(4), 673–85.
- Lawanson, A, 2012. Public sector health care financing and health outcomes in sub-Saharan African countries. Economics of health sector governance and financing in Nigeria, 121–48.
- Le Blanc, D, 2015. Towards integration at last? The sustainable development goals as a network of targets. Sustainable Development 23(3), 176–87.
- Makuta, Innocent & Hare, Bernadette O’, 2015. Quality of governance, public spending on health and health status in Sub-Saharan Africa: a panel data regression analysis. BMC Public Health 15(932), 1–12.
- Micah, AE, Chen, CS, Zlavog, BS, Hashimi, G, Chapin, A & Dieleman, JL, 2019. Trends and drivers of government health spending in sub-Saharan Africa, 1995–2015. BMJ Global Health 4(1), e001159.
- Mirowsky, J & Ross, CE, 2017. Education, social status, and health. Routledge. ISBN 1351328085.
- Murphy, KR & Aguinis, H, 2022. Reporting interaction effects: Visualization, effect size, and interpretation. Journal of Management 48.
- Murphy, KR & Russell, CJ, 2017. Mend it or end it: Redirecting the search for interactions in the organizational sciences. Organizational Research Methods 20(4), 549–573. https://doi.org/10.1177/1094428115625322
- Nicholas, A, Edward, N-A & Bernardin, S, 2016. The effect of health expenditure on selected maternal and child health outcomes in Sub-Saharan Africa. International Journal of Social Economics.
- Nketiah-Amponsah, E, 2019. The impact of health expenditures on health outcomes in Sub-saharan Africa. Journal of Developing Societies 35(1), 134–52.
- Nolte, E & McKee, M, 2004. Does health care save lives? Avoidable mortality revisited. The Nuffield Trust, London, England. https://researchonline.lshtm.ac.uk/id/eprint/15535 ISBN 1902089944.
- Novignon, J & Lawanson, AO, 2017. Health expenditure and child health outcomes in Sub-Saharan Africa. African Review of Economics and Finance 9(1), 96–121. https://www.ajol.info/index.php/aref/article/view/162145/151659https://www.ajol.info/index.php/aref/article/view/162145/151659.
- Novignon, J, Olakojo, SA & Nonvignon, J, 2012. The effects of public and private health care expenditure on health status in sub-Saharan Africa: New evidence from panel data analysis. Health Economics Review 2(1), 22.
- Nyamuranga, C & Shin, J, 2019. Public health expenditure and child mortality in Southern Africa. International Journal of Social Economics 46(9), 1137–54.
- Ochalek, J, Wang, H, Gu, Y, Lomas, J, Cutler, H & Jin, C, 2020. Informing a cost-effectiveness threshold for health technology assessment in China: A marginal productivity approach. Pharmacoeconomics 38(12), 1319–31.
- Onofrei, M, Vatamanu, A-F, Vintilă, G & Cigu, E, 2021. Government health expenditure and public health outcomes: A comparative study among EU developing countries. International Journal of Environmental Research and Public Health 18(20), 10725.
- Park, H, Roubal, AM, Jovaag, A, Gennuso, KP & Catlin, BB, 2015. Relative contributions of a set of health factors to selected health outcomes. American Journal of Preventive Medicine 49(6), 961–9.
- Pham, HST, Le, T & Nguyen, LQT, 2021. Monetary policy and bank liquidity creation: Does bank size matter? International Economic Journal 35(2), 205–22.
- Popoola, TO, 2018. Health outcomes in Sub-Saharan Africa Countries: An analysis of key determinants. Modern Health Science 1(1), 8.
- Rad, EH, Vahedi, S, Teimourizad, A, Esmaeilzadeh, F, Hadian, M & Pour, AT, 2013. Comparison of the effects of public and private health expenditures on the health status: A panel data analysis in eastern Mediterranean countries. International Journal of Health Policy and Management 1(2), 163.
- Rajkumar, AS & Swaroop, V, 2008. Public spending and outcomes: Does governance matter? Journal of Development Economics 86(1), 96–111.
- Roodman, D, 2009. A note on the theme of Too many instruments*. Oxford Bulletin of Economics and Statistics 71(1), 135–58.
- Sajadi, HS, Goodarzi, Z, Takian, A, Mohamadi, E, Olyaeemanesh, A, Hosseinzadeh Lotfi, F, Sharafi, H, Noori Hekmat, S, Jowett, M & Majdzadeh, R, 2020. Assessing the efficiency of Iran health system in making progress towards universal health coverage: A comparative panel data analysis. Cost Effectiveness and Resource Allocation 18, 1–11.
- Sargan, JD, 1988. Testing for misspecification after estimating using instrumental variables. Contributions to Econometrics: John Denis Sargan 1, 213–35.
- Ssozi, J & Amlani, S, 2015. The effectiveness of health expenditure on the proximate and ultimate goals of healthcare in Sub-Saharan Africa. World Development 76, 165–79.
- StataCorp, L, 2019. Stata statistical software. Release 16.
- Stock, JH & Watson, MW, 2019. Introduction to econometrics (3rd updated edition) (4th ed.). Pearson. ISBN 978-9352863501.
- Tandon, A & Cashin, C, 2010. Assessing public expenditure on health from a fiscal space perspective [Working Paper]. Washington, D.C.: World Bank Group. ISBN License: Cc BY 3.0 IGO.
- WHO, 2000. The world health report 2000: Health systems: Improving performance. World Health Organization. ISBN 924156198X.
- WHO, 2011. The Abuja declaration: Ten years on. World Health Organization, Geneva.
- WHO, 2020. World health statistics overview 2020: Monitoring health for the SDGs, sustainable development goals. World Health Organization, Geneva.
- World Bank, 2022. World development indicators World Bank Group. Retrieved 05 june 2021 from https://databank.worldbank.org/source/world-development-indicators.
- Yardim, MS, Cilingiroglu, N & Yardim, N, 2010. Catastrophic health expenditure and impoverishment in Turkey. Health Policy 94(1), 26–33.
- Zhou, Y, 2018. Analysis on the effect of medical system efficiency on residents’ health: Based on panel quantile regression model. Chinese Health Economics 12, 66–9. https://caod.oriprobe.com.
Appendix
Figure A1. Moderator marginal effects with 95% CIs. Note. HSE denotes health system efficiency. From the top left to the bottom right, the marginal effects of DGGHE on the DALY are presented when the HSE varies from minimum SD, −1SD, 0SD, + 1SD, + 2SD, to maximum SD, respectively, with 95% confidence intervals. Computed using STATA version 17 software. *p < 0.10; ***p < 0.01.
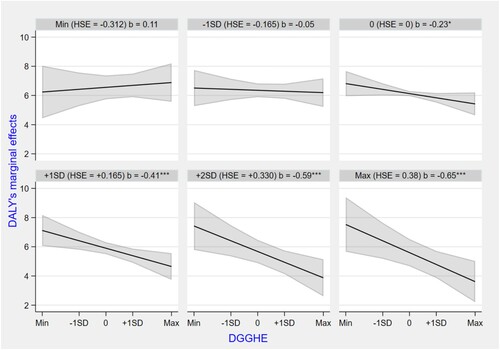
List of countries