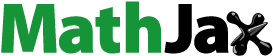
ABSTRACT
There is an evident research gap in the literature on the geography of innovation: very little is known about the innovation activities of rural enterprises in times of crisis. The topic is addressed here by analysing space–time differences in the receipt of research, development and innovation (RDI) funding by Finnish firms before and during the COVID-19 pandemic as a major economic shock. Using firm- and municipal-level panel regression analyses, the main goal was to identify key differences and temporal changes in RDI funding patterns across different region types. The empirical results show that firms located in urban regions have been generally more successful in applying for competitive public RDI funding than firms located in rural regions, but that rural firms have caught up to their urban counterparts during the COVID-19 pandemic. This narrowing of the rural–urban RDI funding gap (1) shows that easy and flexible access to RDI funding during the COVID-19 pandemic appears to be especially attractive for small rural enterprises, (2) points to the strategic flexibility of small rural enterprises in times of crisis, and (3) indicates that the innovation capacity of these firms/regions is more resilient to economic shocks than typically thought.
1. Introduction
As stated by Phillipson et al. (Citation2020), the outbreak of the COVID-19 pandemic and the subsequent government-led measures for its containment have had widespread effects on the functioning of regional economies across Europe and globally. The crisis is expected to have a devastating impact especially on firms located in rural regions, and finding solutions to support firm survival and recovery should accordingly rank high on the policy agenda (Ebersberger and Kuckertz Citation2021). One way to strengthen the rural enterprise economy is through public innovation support. Rural regions have been shown to host a relatively high share of innovating firms due to high rates of public funding of innovation activity (Isaksen and Onsager Citation2010). However, little is yet known about how successful rural enterprises have been compared to urban ones in tapping into public innovation support as a means to adjust their innovation activities after economic shocks. Here, the issue is addressed by investigating the economic policy experiences in Finland during the COVID-19 pandemic, which implied a massive increase in the volume of public research, development and innovation (RDI) funding to firms in times of crisis (Mitze and Makkonen Citation2021). For example, the Finnish government organization for innovation funding and trade, travel, and investment promotion, Business Finland, allocated an additional 994 million euros in 2020 for supporting Finnish firms with innovative new ideas through its funding programme for business development in disruptive circumstances (Business Finland Citation2021). Overall RDI funding volumes issued through Business Finland have tripled in 2020 compared to the pre-COVID period (i.e. 1,740 million euros in 2020 compared to 570 million euros in 2019).
Innovation is commonly considered as fundamental for the long-term economic growth and development path of any region. However, innovation at the firm level might not be sufficient to generate rural development on a regional scale (Shearmur Citation2016; Marques and Morgan Citation2021). As such, the exact mechanisms linking RDI activities to regional economic outcomes are far from being linear. In general, however, there is mounting evidence that RDI fosters productivity, survival and resilience (McCann and Ortega-Argilés Citation2016). Therefore, at the firm level investing in RDI is commonly considered as the best strategy for firm survival in rural regions even in regular times (Sánchez Citation1992; Shearmur Citation2016). According to the literature on the geography of innovation, urban regions seem to be the optimal places for innovation activities to agglomerate (Florida, Adler, and Mellander Citation2017) due to the co-existence of potential collaboration partners, availability of educated workforce, etc. However, there are also studies indicating that the urban–rural divide in RDI might not be as strong as commonly suggested. Moreover, rural regions host many firms engaged in RDI (Shearmur Citation2012, Citation2017).
Innovators located in rural regions commonly rely on technological and scientific information (and, thus, also RDI), which becomes obsolete at a slower pace than market-based information. These innovators thus require a lower frequency of (face-to-face) interactions with collaborators than their ‘faster’ urban counterparts (Shearmur and Doloreux Citation2016). This might be an advantage during the COVID-19 crisis as (innovative) rural firms might be less affected by workplace, mobility and social distancing restrictions and, thus, be better equipped to engage in or continue RDI activities during lockdowns of varying degrees throughout the waves of the pandemic. At the same time, firms in rural regions are typically smaller than their urban counterparts (North and Smallbone Citation1996; Atterton and Skerratt Citation2017). While no consensus has emerged in the empirical literature on whether small or large firms are generally more innovative, small firms in rural and peripheral locations may face distinct barriers to RDI funding access (McAdam, McConvery, and Armstrong Citation2004). These firms may, however, also have a small size advantage and respond more quickly to changing economic circumstances than their larger counterparts (Sen et al. Citation2022). These remarks pose an interesting open question that is further investigated in this paper:
Does the propensity to attract (higher levels of) public RDI funding during the COVID-19 crisis vary across firms of different type, i.e. small versus large firms, industry and, most notably, across the different geographies, i.e. rural versus urban regions, of a country?
The question is addressed empirically with firm-level data collected from Business Finland RDI funding volumes. We run complementary regression analyses using (1) geo-referenced firm-level data together with information on region types and (2) municipal data based on aggregated RDI funding volumes and other municipal socio-economic characteristics. We estimate firm- and municipal-level regression models over the period 2013–2020. By comparing rural and urban regions, the paper engages with several of the key research questions on ‘peripheral economies’ raised by Pugh and Dubois (Citation2021, 273), namely: do rural firms develop alternative strategies to those in the core urban regions? What benefits and constraints accrue from their rural location, and how can such firms be supported? The results of this paper provide a novel perspective for the study of innovation in rural regions. As discussed, for example, by Eder (Citation2019a) and by Pugh, Schmutzler, and Tsvetkova (Citation2021), thus far, the majority of the research on innovation in general and particularly the research on innovation in times of crisis has – apart from some fragmented case studies (e.g. Cannarella and Piccioni Citation2010) – largely overlooked rural regions. The results of this paper tackle this gap in the existing literature by offering insights on the impact of COVID-19 on firms’ RDI activities in rural Finland.
The remainder of this article is structured as follows. The paper proceeds with a brief overview of the literature on innovation activities and outputs in times of crisis with a particular focus on rural regions, followed by a discussion on public RDI funding during the COVID-19 in Section 2. The third section presents the utilized study design (including the delineation of rural and urban regions, the comparative set-up, the data and associated estimation methods). The main results of the paper are presented in Section 4 followed by a concluding section that summarizes the paper and discusses the implications of the results together with the limitations of this paper, paving the way for future research.
2. Literature review
2.1. Innovation in times of crisis
This paper follows the standard OECD (Citation2005, 47) definition of RDI as: ‘all those scientific, technological, organisational, financial and commercial steps, including investment in new knowledge, which actually lead to, or are intended to lead to, the implementation of innovation’. In turn, innovation is defined as: ‘the implementation of a new or significantly improved product (good or service), or process, a new marketing method, or a new organisational method in business practices, workplace organisation or external relations’ (OECD Citation2005, 46).
Innovation is expected to play a central role in the survival of firms during times of economic upheaval and in generating renewed economic growth following a crisis. Thus, the slowdown of RDI in times of crisis is an ill-suited remedy for firm survival, productivity growth, regional development, etc. However, empirical evidence has shown that severe crises – for example, the financial crisis of 2008/2009 – commonly affect RDI investments in a procyclical and not in a countercyclical way. That is, firms decrease rather than increase their spending on RDI when facing financial constraints caused by a crisis (Filippetti and Archibugi Citation2011). This controversy is commonly discussed through the Schumpeterian (Citation[1911] 2017, Citation1942) concept of creative destruction.
The notion of creative destruction describes a dynamic and competitive business environment in which, due to a major discontinuity such an economic downturn or the COVID-19 pandemic: (1) small firms and new entrants emerge as significant innovators that are able to exploit the turbulence caused by the crisis to grab market share from incumbent firms or to open new markets, (2) collaboration has a heightened importance for innovation, (3) research and development efforts are linked to radical innovations that present a break off from traditional solutions (Archibugi, Filippetti, and Frenz Citation2013b, 1249). This implies that a crisis can create conditions for innovation as firms seek measures to improve their productivity and, therefore, in such circumstances RDI investments could become countercyclical (Aghion et al. Citation2012; Hud and Hussinger Citation2015).
However, innovation is always risky: not all RDI investments and efforts will lead to actual commercial outputs (Roper and Turner Citation2020). As postulated by Archibugi, Filippetti, and Frenz (Citation2013a), in times of crisis firms might focus on survival, rather than on seeking out new opportunities with uncertain outcomes. This leads to a divide between those firms that are able to adopt creatively to changes and those that are merely struggling to survive (Phillipson et al. Citation2004). The situation is worsened by restricted access to credits and public sector RDI funding, which commonly become more limited as the result of a crisis (Aghion et al. Citation2012; Makkonen Citation2013). Therefore, it is likely that while many firms might continue or increase their RDI investments with respect to some of their ongoing RDI projects, they will still pause, abandon or postpone others. This results in an overall drop in RDI investment in times of crisis (Archibugi, Filippetti, and Frenz Citation2013a).
As noted, normally, the response of firms and the public sector to crisis seems to be procyclical. There are, naturally, exceptions, and recent evidence has shown that, indeed, some firms (particularly those engaged with technologies, such as e-commerce, alleviating the impacts of the crisis on businesses) have been able to excel in adapting to the turbulence and changing market requirements caused by the COVID-19 pandemic via innovation (Brem, Viardot, and Nylund Citation2021; Guderian et al. Citation2021). However, crisis situations can hit firms very differently depending, for example, on their: (1) size (Eggers Citation2020) – large firms have more resources, but small firms are commonly seen as more agile; (2) age (Cowling, Liu, and Zhang Citation2018) – the experience inherent in established firms is an advantage, but new firms are commonly seen as more agile; (3) industry – the COVID-19 pandemic has had a different impact on, for example, the online retail (Dannenberg et al. Citation2020) and tourism (Sigala Citation2020) industries and; (4) location (Gherhes, Vorley, and Williams Citation2018). While the firm-related aspects are also addressed in the empirical analysis, the focus of this paper is on location (discussed in greater detail in Section 2.2).
2.2. Innovation in rural regions
It is important to remember that firms themselves assert the key role in the pursuit of their RDI activities. However, while firm-specific factors matter the most (Sternberg and Arndt Citation2001), the location and surrounding business environment (regional factors) of firms have also been shown to influence their innovativeness (Beugelsdijk Citation2007; López-Bazo and Motellón Citation2018). The impact that location can have on firms in times of crisis is commonly discussed through the concept of regional or economic resilience: that is, the ability to adapt to and recover from a shock, typically induced through an exogenous crisis (Christopherson, Michie, and Tyler Citation2010; Gong et al. Citation2020). In this literature, urban regions are generally seen as most resilient to shocks due to favourable agglomeration economies, network density, etc. (Capello, Caragliu, and Fratesi Citation2015). In other words, the larger and denser a city is (in terms of population) and the closer it is to other large cities (i.e. the less ‘peripheral’) the better it is equipped for recovery (Hansen and Winther Citation2018). Contrarily, rural regions are often seen to have defects and, thus, offer limited capacity to adapt to an exogenous shock (Halonen Citation2019) due to organizational or institutional thinness, lack of resources, etc. (Isaksen Citation2014; Brown and Cowling Citation2021). This naturally affects the resilience of the firms located in such places.
Recent evidence by Brown and Cowling (Citation2021) on the impacts of COVID-19 on firm survival indicates weak levels of resilience in rural regions. Nevertheless, Giannakis and Bruggeman (Citation2017) have shown contrary evidence that, in fact, metropolitan regions are less resilient than non-metropolitan ones (Giannakis and Bruggeman Citation2020). Dijkstra, Garcilazo, and McCann (Citation2015) clarify that rural regions close to a city recovered better from the financial crisis of 2008/2009 compared to cities. Contrarily, rural regions farther away from cities were heavily hit by the crisis (for a recent study on regional resilience in Finland after the financial crisis, see Kurikka Citation2021). In other words, the evidence on urban–rural differences in regional resilience is mixed. What is evident is that there are likely differences in RDI activities and the intensity of knowledge usage in times of crisis between firms operating in rural and urban regions. However, apart from some predictive accounts based on the literature on earlier crises (Roper and Turner Citation2020), very little is known about the geography of innovation during the COVID-19 pandemic. The literature discussing the rural–urban divide in innovation can, however, give us some clues.
Shearmur (Citation2015) has suggested that innovators who do not require a high frequency of (face-to-face) interaction and related forms of tacit knowledge exchange are likely to locate in rural regions. Their argumentation posits that whereas market-sourced information is easier to access in urban regions, non-market-sourced (technological and scientific) information is more evenly available in both rural and urban regions. Firms whose innovation relies on slowly decaying technological and scientific information (and, thus, also RDI) and which interact less frequently with other actors tend to locate in rural regions (or at least do not have to locate in an urban region). With survey data from the Canadian province of Quebec, Shearmur and Doloreux (Citation2016) have shown evidence that the above argumentation holds when confronted with empirical data. This discussion provides an interesting viewpoint for studying RDI in times of crisis: firms in rural regions that are accustomed to and depend less on frequent (face-to-face) interaction might prevail better than their urban counterparts during the COVID-19 pandemic and the associated measures to restrict mobility and face-to-face contact. At the same time, it has to be acknowledged that innovation is also largely based on the learning, experience, and internal competence of the innovators and not necessarily directly on public RDI funding. Thus, the metric (RDI funding) utilized in this paper does not capture all innovation in rural areas.
According to empirical evidence, firms in urban regions enjoy a premium when it comes to RDI, and particularly innovation cooperation, due to the co-presence of potential collaboration partners and innovation networks (Aryal et al. Citation2018; Hjaltadóttir, Makkonen, and Mitze Citation2020). In contrast, firms in rural regions have been noted to experience practical challenges to RDI, including availability of skilled workers and access to knowledge, funding, and innovative technologies (Cowie et al. Citation2019). However, recent evidence suggests high loyalty of the (especially RDI) workforce towards their employers in rural regions (once hired they tend to stay with the same employer for long periods), counterbalancing the potential negative impacts of initial recruiting problems (Eder and Trippl Citation2019). Again, rural regions are not completely void of innovation and are the home location of many world-class innovators (hidden champions) in their respective fields (Audretsch and Lehmann Citation2016; Makkonen et al. Citation2020; Vonnahme and Lang Citation2022). Furthermore, earlier literature has shown that firms in rural regions have been extremely flexible in their responses to crises, suggesting that many of them may, in fact, be particularly resilient (Irvine and Anderson Citation2004).
In sum, while most of the literature on the geography of innovation (Florida, Adler, and Mellander Citation2017) points towards the conclusion that urban regions have an absolute and relative advantage over rural regions in the pursuit of innovation activities (i.e. an urban premium), there is also a growing body of evidence supporting a contrary view, namely, a more level distribution of RDI between rural and urban regions (Meili and Shearmur Citation2019; Fritsch and Wyrwich Citation2021). However, as said before, not much is known about the innovativeness of firms in rural regions in times of crisis. This issue will be discussed here with empirical material collected from both rural and urban regions of Finland.
2.3. Public RDI funding during the COVID-19 pandemic
The rationale behind public RDI funding lies in its expected positive impacts on firm innovativeness and the subsequent effects on the local economy. The positive link between public RDI funding, innovation and value creation at the local level is well documented (see Mitze and Makkonen Citation2021). For example, as indicated by Ebersberger (Citation2005), on average, public RDI funding increases both the funded firms’ private investment in RDI as well as their innovation output. According to a recent estimation, 35–55% of major Finnish innovations have been stimulated by public RDI funding (Torregrosa-Hetland et al. Citation2019). Thus, public RDI funding is a decent predictor for actual new products or processes introduced into the market (i.e. product or process innovations).
However, less is known about how well RDI activities capture innovation in rural areas. One the one hand, innovation is expected to be built upon learning, experience and internal capacities of innovative firms resembling the doing-using-interacting mode of innovation (DUI). These kinds of innovation activities are notoriously challenging to operationalize into measurable items via traditional metrics. On the other hand, innovation also very much depends on technological and scientific information resembling the science and technology mode of innovation (STI), which is normally accessed precisely through such standard innovation proxies as RDI (Alhusen et al. Citation2021).
With evidence from rural Canada, Doloreux et al. (Citation2020) have shown how the two types of innovation are connected to different types of innovation: the DUI mode is commonly linked to non-technological innovation and the STI to product (goods and services) innovation. As stated by Davies, Michie, and Vironen (Citation2012), while innovation in particular rural locations is linked to RDI, there are also broader kinds of innovativeness that are only partly linked to RDI. Thus, while acknowledging that the indicator choice (public RDI funding) naturally limits the generalisability of our results to depict the entirety of such a complex concept as innovation, it does provide (at least) a first look into the dynamics of the more STI-driven aspects of rural innovation during the COVID-19 pandemic.
During the contemporary pandemic crisis, the European Union – most notably through the Recovery Assistance for Cohesion and the Territories of Europe (REACT-EU) initiative (European Commission Citation2021) – and many national governments have been active in supporting firms with RDI funding. The previously mentioned Business Finland funding for supporting business development in disruptive circumstances (994 million euros in 2020, Business Finland Citation2021) is a prime example. This additional sum on top of the usual RDI funding is much larger than what Business Finland normally grants for RDI per year (e.g. in 2019: 571 million euros). Overall, RDI funding volumes have tripled in 2020 vis-à-vis 2019. Consequently, also the number of RDI funded firms via this instrument was larger than the number of Business Finland RDI funded firms in 2019. Thus, it seems that the postulated lack of finances for RDI might not be as acute as it has been shown to be in the case of the financial crisis of 2008/2009 (Barajas, Huergo, and Moreno Citation2021).
The main research question of this paper is approached here through RDI funding data collected from Business Finland, which, operating under the Finnish Ministry of Employment and the Economy, is the main funding agency for RDI in Finland. RDI funding is granted for firms to improve, develop and apply new technologies and to transform research-stage ideas into viable businesses (Piekkola Citation2007). The decision criteria for receiving RDI funding for a project from Business Finland are based on the technology to be developed and the resources reserved for it, the estimated effects of the project on competitiveness and collaboration as well as its societal benefits (Takalo, Tanayama, and Toivanen Citation2013). Business Finland RDI funding normally covers up to 40–65% of the total costs of the project depending on the size and organizational type of the applicant.
In the aforementioned Business Finland funding programme for business development in disruptive circumstances, launched in 2020 as a response to the COVID-19 pandemic, RDI funding covers up to 80% of the total costs, of which up to 70% can be paid in advance. This funding is given as (1) preliminary funding for investigating and planning new business, alternative subcontracting chains, and ways to organize production during and after the disruption caused by the coronavirus (max. 10,000 €) and (2) development funding for carrying out development plans to improve the potential for success during and after the disruption caused by the coronavirus via the creation of new product- or production-related solutions (max. 100,000 €). The main criterion for receiving funding was that the solution to be developed or implemented must be new (innovative) to the firm. The application period for the programme ran in 2020 and the programme received almost 30,000 applications of which around 20,000 were funded (Business Finland Citation2020).
An important feature of Business Finland funding is that allocation is spatially blind (Luoto and Virkkala Citation2017) – the funding is based on competition according to the same criteria, irrespective of the location of the firm within Finland (Pelkonen Citation2005). Thus, ideally, since the funding is not allocated per any regional quotas, firms with the most innovative and impactful ideas have received the funding.
3. Study design
3.1. Delineating rural and urban regions
The starting point for answering the posed research question concerning (potential) rural–urban differences in RDI activities would, naturally, be to define what is a rural or an urban region. However, there is no consensus on the academic or practical definition of what exactly constitutes a rural (or urban) region (Woods Citation2005). Labelling regions simply either as rural or urban based on locational advantages or disadvantages does not do justice to the complexity of regional characteristics (Copus Citation2001; Kühn Citation2015). Clear cases are exceptional, as most regions lie somewhere in between purely urban and purely rural: the rural–urban axis is a nuanced and non-uniform continuum (or a gradient) rather than a dichotomy (Cloke Citation1977; Eder Citation2019b).
To avoid some of the pitfalls of the rural–urban dichotomy, the Finnish Environment Institute (SYKE) has produced a categorization of Finnish regions into several different types of rurality and urbanity. The analyses on which the categorization is based have been conducted utilizing geographical information methods and high-precision 250 × 250 m grid data on population, labour, work journeys, buildings, road network and land use (SYKE Citation2013; Citation2020). Based on these data, the Finnish regions have been designated into seven categories (region types): (1) sparsely populated rural areas (SPAs), (2) rural heartland areas (RHAs), (3) rural areas close to urban areas (RACs), (4) local centres in rural areas, (5) peri-urban areas, (6) outer urban areas, and (7) inner urban areas. Since many regions are constituted from more than one of the categories of the rural–urban continuum utilized here, the finer the level of spatial aggregation, the more accurately one can depict the rurality/urbanity of the environment the firm is situated in. Therefore, the spatial level investigated here is the local municipal level, which is the lowest level of spatial aggregation available for the data utilized in this study.
The region type of each Finnish municipality on the rural–urban continuum was assigned based on the SYKE data. As the number of municipalities designated as urban (see categories 5–7 above) is much smaller than the ones designated as rural (categories 1–4), the urban regions are aggregated into a single category (see ). Similarly, as category 4 includes only geographically small local centres (taajama in Finnish) of rural municipalities, this category was included into the other categories of rurality (SPAs, RHAs or RACs) depending on the main category of the countryside surrounding the local centre.
Table 1. Descriptive statistics for the main variables used in the empirical analysis of RDI patterns across region types and firm categories.
Importantly, since the concept of periphery relates to distance, relations, geography and function (Fitzgerald and de Souza Citation2017), peripherality is proxied in the categorization of SYKE with indicators depicting work journeys and the road network. Therefore, the utilized rural–urban categorization can simultaneously be used to describe a (rough) peripherality gradient, SPAs being the most peripheral and RACs the least peripheral of the rural regions.
Finally, the dichotomy between rural and urban regions has been criticized for its lack of analytical and explanatory power (Dymitrow and Brauer Citation2018). Admittedly, the classification of rural regions into the three different groups provided by SYKE is only a minor improvement in this debate. However, for comparative empirical purposes, and due to the lack of better alternatives, the decision was taken to utilize these three types of rurality, plus an additional fourth class for cities.
3.2. Data and estimation method
Panel econometric tools were used to investigate space–time differences in the propensity of individual firms and municipal aggregates to acquire Business Finland RDI funding volumes. For this reason, we constructed two complementary datasets. The first dataset utilizes information on the total population of Finnish firms that have received RDI funding through Business Finland since 2010 as units of analysis. These data were gathered from the data interface of Business Finland. In addition to information on the year and financial amount of public funding received, we also extracted data on basic firm characteristics, such as the size category – micro, small, medium, or large – of each firm and its sectoral affiliation. Moreover, based on geoinformation (addresses) on firm location we were able to associate each firm with one of the above defined region-type categories (SPAs, RHAs, RACs and Cities). This allowed us to specify a first panel regression model to quantify the role that location and other factors play in the firm’s propensity to receive RDI funding over time as
(1)
(1) where
denotes the probability that firm i receives Business Finland RDI funding in year t and
indicates that the model is estimated as a fixed effects (FE) logit model specification with
(Allison Citation2009). Hence, Equation (1) utilizes binary information on whether the individual firm has received competitive public RDI funding or not as a proxy measure for an innovate firm. The included regression coefficients in Equation (1) measure the change in the logit, i.e. the log of the odds, for the probability of RDI funding receipt associated with a unit change in one of the included predictors while holding all other predictors constant. To facilitate the interpretation of regression coefficients from the FE logit model, in the discussion of the empirical results we additionally report the odds ratio, i.e. the exponentiated regression coefficient, as a direct measure of a change in the odds (rather than the log of the odds).
As an alternative to the binary specification, the volume of public RDI funding received by firm i in year t is taken as a metric outcome variable. Given that a considerable fraction of firm-year observations is zero (for details see below), the latter specification is estimated as a FE Poisson model to avoid running into an estimation bias for a non-negative outcome variable that contains a lot of zeros (Wooldridge Citation1999). In analogy to the binary FE Logit model in Equation (1), the FE Poisson model can be estimated as
(2)
(2) where
is the volume of RDI funding received by firm i in year t and the logarithm (log) is used as a link function in the Poisson regressions (Wooldridge Citation2010). This specification allows us to interpret regression coefficients in the FE Poisson model as elasticities, which measure the percentage response in RDI funding volume to a one percent change in a log-transformed continuous variable or to a switch from zero to one in the case of a binary dummy variable. The set of variables used as predictors in Equations (1) and (2) are introduced below.
While we have few time-varying controls at the level of the individual firm, measures the previous success of firm i in receiving Business Finland RDI funding and is specified as the cumulative amount of funding received from 2010 onwards. The log-transformed variable enters Equations (1) and (2) with a one-year lag to avoid endogeneity problems associated with the model’s outcome variable. While
are year fixed effects to control for time differences in funding receipt common to all sample firms,
are firm fixed effects that allow us to control for unobservable time fixed differences across firms (such as age, organizational type etc.). In a less constrained model specification, we replace the firm-level fixed effects with municipal fixed effects
to control for latent differences in funding receipt between otherwise homogeneous firms located in alternative municipalities (for the set of j = 1, … , J municipalities). Finally,
is the model’s error term.
The primary focus of our estimation approach is on quantifying relative changes in the probability of Business Finland RDI funding receipt for distinct groups of firms when comparing firm behaviour during the COVID-19 pandemic in 2020 vis-à-vis pre-COVID time periods. This can be measured through the regression coefficients and
of the included interaction terms
and
in Equation (1). Thereby,
is a binary dummy that takes a value of one in 2020 and is zero otherwise. It thus measures the start of the COVID-19 pandemic in Finland;
and
relate to firm- and region-specific characteristics of interest (where the index j(i) indicates that firm i is located in municipality j), respectively. As such, we are particularly interested in estimating if firm location in different types of regions (i.e. SPAs, RHAs and RACs vis-à-vis cities) has a time heterogeneous effect on RDI funding receipt. This, in turn, helps us to answer the question of whether the geography of innovation has changed during the COVID-19 pandemic. Similarly, regarding firm characteristics
, we seek to investigative if relative changes in RDI funding receipt during the COVID-19 vis-à-vis pre-COVID time periods potentially differ by firm size or sectoral affiliation.
In extended versions of Equations (1) and (2), we also test for potential early-participation effects by replacing the binary dummy with the vector of time fixed effects (
) covering each sample year separately. We do so by adapting the interaction terms to
and
. This allows us to comprehensively estimate whether the correlation between firm- or region-specific characteristics and RDI receipt varies over the sample years (as indicated by the period-specific regression coefficients
and
) and thus to detect group-specific trends, which may have potentially started before the COVID-19 pandemic. However, with the growing complexity of the included interaction terms, also the interpretability of the results becomes more and more challenging. This particularly holds for a triple interaction term of the form
to directly compare the RDI funding receipt of firms of different size groups in alternative locations. Here, we rely on graphical effect visualizations as means of interpretation.
One advantage of firm-level estimates is the large number of observations and the availability of firm-specific information such as size class and sectoral affiliation of the funded firms. The firm-level approach also allows us to control for time constant firm-level heterogeneity by including firm fixed effects (). However, a potential disadvantage of this micro-level approach is that we can only make statements for the sub-sample of municipalities (region types) with at least one firm receiving RDI funding during the sample period. Municipalities without any funding receipt or very few firms receiving funding, either drop out of the sample or get a small weight in the firm-level estimates. This may potentially bias the estimates of the correlation between RDI funding and region-type categories. Another disadvantage of the firm-level dataset is the large fraction of zero firm-year observations when it comes to our outcome variables. Finally, besides
no time-varying controls are available at the firm level, which may also give rise to a potential estimation bias due to omitted variables.
As a complementary regression approach, we have therefore also constructed an aggregate municipal-level dataset to estimate the following FE Poisson panel regression model
(3)
(3) where
is the volume of RDI funding received by municipality j in year t and, as in Equation (2), the logarithm (log) is used as a link function in the Poisson regressions. We control for municipal- and time fixed effects
and additionally include time-varying covariates
at the municipal level, such as firm density, i.e. the number of firms per square kilometre, new firm formation, i.e. the net growth in newly founded business relative to the local stock of firms, the municipal-level unemployment rate and sectoral shares. As outlined above, the included interaction term between the COVID-19 time dummy and regional structural characteristics
allows us to test for significant changes in the RDI funding receipt by region-type category (or alternative municipal characteristics, such as sector structures covered in
) during the COVID-19 pandemic.
Overall, the interaction term specifications in Equations (1) to (3) enable us to investigate if the (potential) differences in RDI funding receipt between region-type categories have converged, stayed the same or grown during the COVID-19 pandemic. All municipal data were gathered from the Stat.Fi database of Statistics Finland. All regression analyses were conducted based on annual data for the time period 2013–2020, where 2013 is the first year with full data availability on all the included variables and 2020 serves as the year indicating the arrival of the COVID-19 pandemic in Finland (the first confirmed coronavirus case in Finland was in January 2020).
4. Results
Before turning to the results of the regression analyses, we present some stylized facts of RDI funding receipt at the individual firm and municipal level. provides descriptive statistics for the main variables used at the micro level. The focus is on describing the distribution of firms having at least once received Business Finland RDI funding since 2010 in the categories ‘region type’ and ‘size class’. As the table shows, approx. 4% of the sample firms are located in a SPA, approx. 8% and 5% belong to an RHA or a RAC, respectively. Most firms are located in cities (approx. 83%). With regard to firm size, about two thirds of firms receiving RDI funding since 2010 are classified as micro firms with less than 10 employees. About 24% of firms are small firms followed by medium-sized and large firms (each 5%). While RDI receipt measured as the share of firms receiving Business Finland funding in each category is more or less equally distributed across region-type categories and size classes (with shares ranging between 15% and 20% per year), shows that firms located in cities receive larger RDI funding volumes, on average, compared to SPA firms (approx. 23,000 versus 10,000 EUR). Even larger differences in average RDI funding volumes can be observed across size classes – ranging from approx. 11,000 EUR for micro firms to 134,000 EUR for large firms (on average). Complementing the information on the cross-sectional distribution of RDI funding across firms given in , plots the temporal development of RDI funding trends across region types and size classes. In all subfigures it can be clearly seen that both the share of firms receiving RDI funding and also the RDI funding volume granted increases with the start of the COVID-19 pandemic in 2020.
The spatial distribution of RDI funding volumes across Finnish municipalities for different temporal snapshots is shown in . Panel A of provides an overview of the different region types as defined above. As shown in panels B and C, prior to the start of the COVID-19 crisis higher RDI funding volumes are mainly allocated to larger cities in the rural–urban continuum of Finnish municipalities. This, however, changes in 2020, with Panel D of showing a more even spatial distribution of RDI funding. This is associated with a general increase in RDI funding levels at the municipal level and a considerably larger share of recipient firms, as already highlighted in . While this may point to a changing geography of innovation during the COVID-19 crisis, mainly driven by strong political incentives to combat the crisis through enhanced RDI activities across all firms in the Finnish economy, we will next explore this issue more systematically with the help of regression analyses.
reports panel regression results for the firm-level data set. The first three columns show results for the probability that firms receive RDI funding using the FE Logit estimator for panel data. In column (1), only industry-level fixed effects are included, while column (2) adds municipal fixed effects and column (3) reports the results for the FE Poisson model with the full vector of firm fixed effects. In addition, column (4) shows the estimation output for a FE Poisson model with RDI funding volumes as the outcome variable. In all model specifications, the included year dummies indicate a statistically significant positive time trend, which peaks in 2020. This is clearly in line with the stylized facts presented in . For instance, the statistically significant coefficient 1.850 in Column 1 for the sample year 2020 indicates in terms of the odds ratio that the probability of receiving RDI funding is exp(1.85) = 6.35 times higher in 2020 compared to the reference year 2014. This highlights the massive increase in RDI funding to fight the economic consequences of the COVID-19 pandemic.
Table 2. Estimates of the geography of RDI funding receipt by Finnish firms before and during COVID-19.
With regard to the included controls, all reported specifications in reveal a negative correlation between the outcome variable (probability of funding receipt or funding volume) and the lagged cumulative RDI funding volume received by the individual firm. The most likely explanation for this negative correlation is that RDI funds are discretely granted to firms over time. Column (1) further shows that, on average, firms in rural areas have a lower probability of receiving funding compared to firms in cities (the underlying odds ratio for SPAs indicates a 32% lower probability, i.e. 1 – exp(−0.393) = 0.32; RHAs and RACs have roughly a 25% lower probability of funding receipt). The reader should note that this effect is partly or fully absorbed by the included fixed effects structure in Column (2) and Column (3). Similarly, SMEs (most notable micro and small firms) are shown to have a generally lower probability of RDI funding receipt compared to large firms during our sample period. The average gap to large firms as a reference group ranges from 25% for medium-sized firms (1 − exp(−0.292) = 0.25) to 58% for micro firms (1 − exp(−0.879) = 0.58).
Of specific interest are the estimated coefficients for relative changes in RDI funding patterns during COVID-19. Here, we find that firms in SPAs show that the largest relative increase in the probability of funding receipt in Columns (1) to (3) and also the largest relative increase in RDI funding volumes as reported in Column (4). This increase is measured relatively to the RDI funding development of firms located in cities as a reference category. Thus, the reported coefficients estimate the additional increase in the outcome variable relative to firms in cities on top of the common time trend measured through the included year dummies. For the exemplary case of Column (1), firms in SPAs show a more than two times stronger increase in the probability of RDI funding receipt during COVID-19 than firms in cities (i.e. exp(0.766) = 2.15); firms located in RHAs or RACs experience a 50% relative increase in RDI funding receipt. The estimated effects become moderately smaller if the regression specification fully controls for latent firm-level heterogeneity in Column (3), e.g. for firms located in SPAs the results in Column (3) still point to a 40% relative increase in RDI funding receipt vis-à-vis firms in cities. With regard to the impact of COVID-19 on the level of RDI funding volume granted to firms in different types of regions, Column (4) indicates that the relative increase in RDI funding is roughly 1.2 times larger for firms in SPAs compared those located to cities; firms in RHAs and RACs experience an additional RDI funding increase relative to those in cities of between 40 and 50%.
A similar time pattern can also be observed for the development of RDI funding by firms of different size categories. All three SME subgroups show larger outcome increases during COVID-19 compared to large firms (reference category). In , micro firms are estimated to experience a four to eight times stronger increase in the probability of receiving funding than large firms when we compare the COVID-19 development to the average pre-COVID funding probability. The relative volume increase of RDI funding for these firms is on average 60% during the COVID-19 period (see Column (4)). While the effects for small firms are of similar size, the additional RDI funding increase during COVID-19 is smaller (and estimated to be statistically insignificant) for medium-sized firms compared to large firms. These trend differences in predicted RDI funding volumes are visualized in for a triple interaction term specification. For ease of presentation, in rural regions (SPAs, RHAs and RACs) are aggregated to one category. Micro and small firms are also pooled together. As the predictions show, small firms in rural regions have, on average, closed the initially large distance to firms in urban regions during COVID-19.
Figure 3. Temporal development of predicted RDI funding volume by region type and size class.
Notes: Estimation results from FE Poisson regression; categorial variables have been merged to rural (=SPAs, RHAs and RACs) and small (=micro and small firms) to increase the readability of the figure. Predicted RDI volume is measured in logarithms; included control variables are measured at their mean values and firm-fixed effects are excluded from the predictions (as they drop out from the likelihood function of the FE Poisson estimator). The reference year for prediction of the effect of the categorial variables on received RDI funding volume is 2014.

We also tested for differences in RDI funding patterns during COVID-19 across sectors. Here, the visualized results in indicate that the sector aggregate I + R (accommodation and food services and arts, culture and recreation) shows a larger increase in RDI funding receipt than most other sectors (with the aggregate A + B, agriculture and mining, serving as reference category). This points to the fact that firms in sectors most affected by the COVID-19 pandemic and subsequent public health restrictions (social distancing, lockdowns, etc.) have been the most active in using RDI funding as a way to cope with this major challenge posed by the COVID-19 crisis.
Figure 4. Sectoral differences in the increase in RDI funding volumes during COVID-19 in 2020.
Notes: Estimation results from FE Poisson regression for coefficient of interaction term composed of COVID-19 time dummy and sectoral aggregates as , where sectoral aggregates are defined as follows: A + B (agriculture and mining), C + F (construction and manufacturing), D + E + H (electricity, water and transportation), G (wholesale and retail trade), I + R (accommodation and food services and arts, culture and recreation), J + M + N (information and communication, professional and scientific services and administrative and support services), K + L + O + P + Q + S (other services). The sector aggregate A+B is used as reference category; firms with other activities or unknown industry have been excluded from the figure. Diamonds indicate point estimates; bars show 95% confidence intervals (relative to A+B as reference cat.).

complements the firm-level results by reporting regression results for RDI funding volume aggregated to the municipal level (descriptive statistics are shown in Appendix ). These aggregated municipal-level results largely support the findings from and point to higher RDI funding receipt in municipalities located in sparsely populated areas (SPAs) during COVID-19. In terms of effect magnitude, the relative increase in RDI funding is roughly 1.2 to 1.4 times larger for SPAs compared to cities. The results are robust for alternative specifications of the outcome variable either as overall RDI volume or as RDI volume per municipal population. Different from the firm-level results, however, we do not find statistically significant differences between the two other rural categories (RHAs and RACs) and the reference group (cities) when we compare the adjustment of RDI funding behaviour during the pandemic. The municipal-level results further show that variations in the level of RDI funding are not significantly correlated with other time-varying factors at the municipal level, such as unemployment rate, firm density, and new firm formation. Overall, the firm- and municipal-level results point to a process of spatial dispersion and a closing of the RDI funding gap between cities and rural areas in the short run.
Table 3. Municipal-level estimates of the geography of RDI funding receipt before and during COVID-19.
5. Conclusions
Drawing from the literature on the geography of innovation, it seems that urban regions do better in terms of innovation compared to their rural counterparts constrained by lack of cooperation partners, agglomeration economies, etc. (Florida, Adler, and Mellander Citation2017). However, the emerging literature on innovation in rural areas posits that rural firms have developed alternative strategies for innovation (Vonnahme and Lang Citation2022) to benefit from their location and that the rural–urban gap in innovation might not be as marked as previously suggested. In times of crisis, the concept of resilience has been brought up as a major factor determining which regions fare better (adapt to and recover from) in the face of abrupt changes in their economy. The evidence on the matter is mixed in relation to rural and urban regions: some studies point to an evident urban premium, whereas others state that rural regions have also done remarkably well in recovering, for example, from the financial crisis of 2008/2009. This literature provides an interesting backdrop to empirically investigate whether the propensity to attract (higher levels of) public RDI funding during the COVID-19 crisis varied across size classes and industry affiliation of the recipient firms and, particularly, across urban and rural areas of Finland?
The results of this paper, based on Business Finland RDI funding data, show that firms located in urban regions have commonly been more successful in applying for competitive public RDI funding than firms located in rural regions. The result is in line with the mainstream geography of innovation literature (Marceau Citation2008). The situation has, however, changed during the COVID-19 pandemic. The most recent data from 2020 on RDI funding aimed precisely at supporting innovation activities in disruptive circumstances shows that the gap between cities and rural regions has narrowed. Controlling for latent firm characteristics, in 2020 rural firms have been at least equally successful as urban firms in attracting publicly funded RDI. The largest relative increase in RDI funding receipt is observed for firms located in SPAs. Similarly, in line with the creative destruction theorem, the gap between large and small firms has been closed. In 2020, small firms became more active in applying for RDI funding during COVID-19 and, overall, closely matched large firms in terms of attracting publicly funded RDI. This is arguably due to the smaller funding amounts of the bulk of the funding granted in 2020, discouraging larger firms to apply. A third point arising from our data and analyses is that sectors heavily affected by COVID-19 – i.e. accommodation and food services and arts, culture and recreation – have been the most active in applying RDI funding during the contemporary crisis.
Public RDI funding has been shown to be beneficial for regional economic growth (Mitze and Makkonen Citation2021). However, the link from innovation to regional development is far from being straightforward, particularly in rural regions. Thus, while not taking a stance on the actual long-term effectiveness of the inspected RDI instrument, the results do have definite implications in terms of innovation policy. Business Finland RDI funding has actually led to an increase in the speed of convergence in innovation activities between rural and urban regions in 2020. Therefore, while not designed as a regional innovation policy tool, the funding has nonetheless led to spatially distributed outcomes. During the COVID-19 pandemic this can be seen as fortunate, since public RDI funding has recently been found to have positive effects on regional structural strength (defined as the presence of growing and/or innovative industries) precisely in economically weak regions and low or even negative impacts in economically strong regions (Brenner and Pudelko Citation2019). That is, while the absorptive capacity of regions naturally affects how well they are able to utilize external funding (Oughton, Landabaso, and Morgan Citation2002), public RDI funding seems to make a more significant impact on regional development precisely in rural rather than in urban regions.
Further, as suggested by Davies, Michie, and Vironen (Citation2012) public sector support is also needed to ensure the necessary infrastructure, higher education facilities and networking opportunities to stimulate innovativeness in rural regions. However, according to the latest information from Business Finland,Footnote1 it seems that only a small portion of the firms that received funding in 2020 from the business development in disruptive circumstances instrument (applicable only in 2020) have applied for (regular) RDI funding again in 2021. Thus, it remains to be seen whether the observed changes in the geography of innovation in 2020 were, in fact, only short-lived and more related to this specific instrument than actual long-term convergence. As such, we might experience a return to the pre-COVID-19 situation in the near future where firms in urban regions have (once again) the premium in terms of RDI funding. However, the decline in RDI funding applications may also reflect that the implementation of innovation projects typically runs for multiple years and that funding demand is thus discontinuous over time. Hence, long-run analyses are needed here to fully answer the question of how far crisis situations lead to a change in the geography of innovation of a country.
The chosen approach has limitations that hopefully will pave the way for further research on the topic. While public RDI funding has been shown to increase the innovativeness of firms (McCann and Ortega-Argilés Citation2016), it is impossible to perfectly predict innovation outputs with data on innovation inputs (Arrow Citation1962). Receiving RDI funding does not imply that the innovation activities firms engage in are automatically successful. That is, the impact of RDI funding on firms’ innovativeness and innovation quality might turn out to be marginal in the long run (Plank and Doblinger Citation2018; Yigitcanlar et al. Citation2018). Therefore, this investigation into the impacts that the COVID-19 pandemic has had on RDI in rural and urban regions of Finland needs to be repeated once data on alternative innovation proxies become available or after the next round of the European Community Innovation Survey is released.
Further, Business Finland is not the only organization administering public RDI funding in Finland. For example, Regional Centres for Economic Development, Transport and the Environment have allocated small RDI subsidies (max. 10,000 €) for micro firms (employing a maximum of five people) during the COVID-19 pandemic (ELY Centre Citation2022). However, the reporting of such data is scattered. Integrating all public RDI funding into a single database would facilitate a more comprehensive analysis on their impacts. Accordingly, comparative data is needed to verify whether the results presented here are specific to the investigated RDI programme and Finland or whether similar patterns have emerged elsewhere. Finally, a longer timeframe is needed to investigate whether the emerged patterns hold in the long run, as the crisis has been prolonged, and, particularly, to analyse what happens once the crisis has ended.
Disclosure statement
No potential conflict of interest was reported by the authors.
Additional information
Funding
Notes
1 Personal communication with Annu Kotiranta (Senior Adviser, Business Finland).
References
- Aghion, P., P. Askenazy, N. Berman, G. Cette, and L. Eymard. 2012. “Credit Constraints and the Cyclicality of R&D Investment: Evidence from France.” Journal of the European Economic Association 10 (5): 1001–1024. doi:10.1111/j.1542-4774.2012.01093.x.
- Alhusen, H., T. Bennat, K. Bizer, U. Cantner, E. Horstmann, M. Kalthaus, T. Proeger, R. Sternberg, and S. Töpfer. 2021. “A New Measurement Conception for the ‘Doing-Using-Interacting’ Mode of Innovation.” Research Policy 50 (4): 104214. doi:10.1016/j.respol.2021.104214.
- Allison, P. D. 2009. Fixed Effects Regression Models. Los Angeles: Sage.
- Archibugi, D., A. Filippetti, and M. Frenz. 2013a. “Economic Crisis and Innovation: Is Destruction Prevailing Over Accumulation?” Research Policy 42 (2): 303–314. doi:10.1016/j.respol.2012.07.002.
- Archibugi, D., A. Filippetti, and M. Frenz. 2013b. “The Impact of the Economic Crisis on Innovation: Evidence from Europe.” Technological Forecasting and Social Change 80 (7): 1247–1260. doi:10.1016/j.techfore.2013.05.005.
- Arrow, K. 1962. “Economic Welfare and the Allocation of Resources for Invention.” In The Rate and Direction of Inventive Activity, edited by R. Nelson, 609–625. Princeton: Princeton University Press.
- Aryal, G., J. Mann, S. Loveridge, and S. Joshi. 2018. “Exploring Innovation Creation across Rural and Urban Firms: Analysis of the National Survey of Business Competitiveness.” Journal of Entrepreneurship and Public Policy 7 (4): 357–376. doi:10.1108/JEPP-D-18-00026.
- Atterton, J., and S. Skerratt. 2017. A Better Future for Europe’s Rural Regions: Final Report for the Council of Europe. Edinburgh: Rural Policy Centre.
- Audretsch, D., and E. Lehmann. 2016. The Seven Secrets of Germany. New York: Oxford University Press.
- Barajas, A., E. Huergo, and L. Moreno. 2021. “The Role of Public Loans in Financing Business R&D Through the Economic Cycle.” Economia Politica 38: 505–538. doi:10.1007/s40888-021-00225-9.
- Beugelsdijk, S. 2007. “The Regional Environment and a Firm’s Innovative Performance: A Plea for a Multilevel Interactionist Approach.” Economic Geography 83 (2): 181–199. doi:10.1111/j.1944-8287.2007.tb00342.x.
- Brem, A., E. Viardot, and P. Nylund. 2021. “Implications of the Coronavirus (COVID-19) Outbreak for Innovation: Which Technologies Will Improve Our Lives?” Technological Forecasting and Social Change 163: 120451. doi:10.1016/j.techfore.2020.120451.
- Brenner, T., and F. Pudelko. 2019. “The Effects of Public Research and Subsidies on Regional Structural Strength.” Journal of Evolutionary Economics 29 (5): 1433–1458. doi:10.1007/s00191-019-00626-x.
- Brown, R., and M. Cowling. 2021. “The Geographical Impact of the COVID-19 Crisis on Precautionary Savings, Firm Survival and Jobs: Evidence from the United Kingdom’s 100 Largest Towns and Cities.” International Small Business Journal: Researching Entrepreneurship 39 (4): 319–329. doi:10.1177/0266242621989326.
- Business Finland. 2020. “Funding for Business Development in Disruptive Circumstances.” Accessed October 3, 2021. https://www.businessfinland.fi/en/for-finnish-customers/services/funding/disruptive-situations-funding.
- Business Finland. 2021. “Häiriötilannerahoituksen Hakemukset Käsitelty.” Accessed October 3, 2021. https://www.businessfinland.fi/ajankohtaista/uutiset/tiedotteet/2021/hairiotilannerahoituksen-hakemukset-kasitelty–kolmannes-yritysten-projekteista-jo-paattynyt.
- Cannarella, C., and V. Piccioni. 2010. “Discouraged Entrepreneurship and Inhibited Innovation in Times of Crisis and Recession: Empirical Observations in Doing Business in a Rural Area in Central Italy.” International Journal of Rural Management 6 (2): 265–288. doi:10.1177/097300521200600204.
- Capello, R., A. Caragliu, and U. Fratesi. 2015. “Spatial Heterogeneity in the Costs of the Economic Crisis in Europe: Are Cities Sources of Regional Resilience?” Journal of Economic Geography 15 (5): 951–972. doi:10.1093/jeg/lbu053.
- Christopherson, S., J. Michie, and P. Tyler. 2010. Regional Resilience: Theoretical and Empirical Perspectives, Cambridge Journal of Regions, Economy and Society 3 (1): 3–10. doi:10.1093/cjres/rsq004
- Cloke, P. 1977. “An Index of Rurality for England and Wales.” Regional Studies 11 (1): 31–46. doi:10.1080/09595237700185041.
- Copus, A. 2001. “From Core–Periphery to Polycentric Development: Concepts of Spatial and Aspatial Peripherality.” European Planning Studies 9 (4): 539–552. doi:10.1080/09654310120049899.
- Cowie, P., P. Tiwasing, J. Phillipson, and M. Gorton. 2019. “Rural Innovation and Small Business Development.” In The Routledge Companion to Rural Planning, edited by M. Scott, N. Gallent, and M. Gkartzios, 192–199. London: Routledge.
- Cowling, M., W. Liu, and N. Zhang. 2018. “Did Firm Age, Experience, and Access to Finance Count? SME Performance After the Global Financial Crisis.” Journal of Evolutionary Economics 28 (1): 77–100. doi:10.1007/s00191-017-0502-z.
- Dannenberg, P., M. Fuchs, T. Riedler, and C. Wiedemann. 2020. “Digital Transition by COVID-19 Pandemic? The German Food Online Retail.” Tijdschrift Voor Economische en Sociale Geografie 111 (3): 543–560. doi:10.1111/tesg.12453.
- Davies, S., R. Michie, and H. Vironen. 2012. “Can Peripheral Regions Innovate?” In Regional Development in Northern Europe, edited by M. Danson, and P. de Souza, 136–151. Abingdon: Routledge.
- Dijkstra, L., E. Garcilazo, and P. McCann. 2015. “The Effects of the Global Financial Crisis on European Regions and Cities.” Journal of Economic Geography 15 (5): 935–949. doi:10.1093/jeg/lbv032.
- Doloreux, D., R. Shearmur, I. Porto-Gomez, and J. Zabala-Iturriagagoitia. 2020. “DUI and STI Innovation Modes in the Canadian Wine Industry: The Geography of Interaction Modes.” Growth and Change 51 (3): 890–909. doi:10.1111/grow.12385.
- Dymitrow, M., and R. Brauer. 2018. Meaningful yet Useless? Factors Behind the Retention of Questionable Concepts in Human Geography. Geografiska Annaler: Series B, Human Geography 100 (3): 195–219. doi:10.1080/04353684.2017.1419071
- Ebersberger, B. 2005. The Impact of Public R&D Funding. Espoo: VTT Technical Research Centre of Finland.
- Ebersberger, B., and A. Kuckertz. 2021. “Hop to It! The Impact of Organization Type on Innovation Response Time to the COVID-19 Crisis.” Journal of Business Research 124: 126–135. doi:10.1016/j.jbusres.2020.11.051.
- Eder, J. 2019a. “Innovation in the Periphery: A Critical Survey and Research Agenda.” International Regional Science Review 42 (2): 119–146. doi:10.1177/0160017618764279.
- Eder, J. 2019b. “Peripheralization and Knowledge Bases in Austria: Towards a New Regional Typology.” European Planning Studies 27 (1): 42–67. doi:10.1080/09654313.2018.1541966.
- Eder, J., and M. Trippl. 2019. “Innovation in the Periphery: Compensation and Exploitation Strategies.” Growth and Change 50 (4): 1511–1531. doi:10.1111/grow.12328.
- Eggers, F. 2020. “Masters of Disasters? Challenges and Opportunities for SMEs in Times of Crisis.” Journal of Business Research 116: 199–208. doi:10.1016/j.jbusres.2020.05.025.
- ELY Centre. 2022. “ELY Centres’ Funding for Companies in the Exceptional Circumstances Caused by the Coronavirus.” Accessed January 19, 2022. https://www.ely-keskus.fi/en/web/ely-en/poikkeustilannerahoitus.
- European Commission. 2021. “REACT-EU: Fostering Crisis repair and Resilience.” Accessed October 3, 2021. https://cohesiondata.ec.europa.eu/stories/s/REACT-EU-Fostering-crisis-repair-and-resilience/26d9-dqzy/.
- Filippetti, A., and D. Archibugi. 2011. “Innovation in Times of Crisis: National Systems of Innovation, Structure, and Demand.” Research Policy 40 (2): 179–192. doi:10.1016/j.respol.2010.09.001.
- Fitzgerald, J., and P. de Souza. 2017. The Rural and Peripheral in Regional Development. Abingdon: Routledge.
- Florida, R., P. Adler, and C. Mellander. 2017. “The City as Innovation Machine.” Regional Studies 51 (1): 86–96. doi:10.1080/00343404.2016.1255324.
- Fritsch, M., and M. Wyrwich. 2021. “Does Successful Innovation Require Large Urban Areas? Germany as a Counterexample.” Economic Geography 97 (3): 284–308. doi:10.1080/00130095.2021.1920391.
- Gherhes, C., T. Vorley, and N. Williams. 2018. “Entrepreneurship and Local Economic Resilience: The Impact of Institutional Hysteresis in Peripheral Places.” Small Business Economics 51 (3): 577–590. doi:10.1007/s11187-017-9946-7.
- Giannakis, E., and A. Bruggeman. 2017. “Determinants of Regional Resilience to Economic Crisis: A European Perspective.” European Planning Studies 25 (8): 1394–1415. doi:10.1080/09654313.2017.1319464.
- Giannakis, E., and A. Bruggeman. 2020. “Regional Disparities in Economic Resilience in the European Union Across the Urban–Rural Divide.” Regional Studies 54 (9): 1200–1213. doi:10.1080/00343404.2019.1698720.
- Gong, H., R. Hassink, J. Tan, and D. Huang. 2020. “Regional Resilience in Times of a Pandemic Crisis: The Case of COVID-19 in China.” Tijdschrift Voor Economische en Sociale Geografie 111 (3): 497–512. doi:10.1111/tesg.12447.
- Guderian, C., P. Bican, F. Riar, and S. Chattopadhyay. 2021. “Innovation Management in Crisis: Patent Analytics as a Response to the COVID-19 Pandemic.” R&D Management 51 (2): 223–239. doi:10.1111/radm.12447.
- Halonen, M. 2019. “The Long-Term Adaptation of a Resource Periphery as Narrated by Local Policy-Makers in Lieksa.” Fennia - International Journal of Geography 197 (1): 40–57. doi:10.11143/fennia.74368.
- Hansen, K., and L. Winther. 2018. Employment Growth in Danish Towns and Regions Since the Crisis: Industrial Structure, City Size and Location, 2008–2013. Geografiska Annaler: Series B, Human Geography 100 (3): 244–262. doi:10.1080/04353684.2017.1405358
- Hjaltadóttir, R. E., T. Makkonen, and T. Mitze. 2020. “Inter-regional Innovation Cooperation and Structural Heterogeneity: Does Being a Rural, or Border Region, or Both, Make a Difference?” Journal of Rural Studies 74: 257–270. doi:10.1016/j.jrurstud.2019.10.008.
- Hud, M., and K. Hussinger. 2015. “The Impact of R&D Subsidies During the Crisis.” Research Policy 44 (10): 1844–1855. doi:10.1016/j.respol.2015.06.003.
- Irvine, W., and A. Anderson. 2004. “Small Tourist Firms in Rural Areas: Agility, Vulnerability and Survival in the Face of Crisis.” International Journal of Entrepreneurial Behavior & Research 10 (4): 229–246. doi:10.1108/13552550410544204.
- Isaksen, A. 2014. “Industrial Development in Thin Regions: Trapped in Path Extension?” Journal of Economic Geography 15 (3): 585–600. doi:10.1093/jeg/lbu026.
- Isaksen, A., and K. Onsager. 2010. “Regions, Networks and Innovative Performance: The Case of Knowledge-Intensive Industries in Norway.” European Urban and Regional Studies 17 (3): 227–243. doi:10.1177/0969776409356217.
- Kühn, M. 2015. “Peripheralization: Theoretical Concepts Explaining Socio-Spatial Inequalities.” European Planning Studies 23 (2): 367–378. doi:10.1080/09654313.2013.862518.
- Kurikka, H. 2021. “Globaali Talouskriisi ja Suomen Alueellinen Resilienssi.” Terra 133 (1): 3–16. doi:10.30677/terra.96014.
- López-Bazo, E., and E. Motellón. 2018. “Innovation, Heterogeneous Firms and the Region: Evidence from Spain.” Regional Studies 52 (5): 673–687. doi:10.1080/00343404.2017.1331296.
- Luoto, I., and S. Virkkala. 2017. Paikkaperustainen Aluekehittäminen Strategisena Ohjenuorana. Helsinki: Ministry of Economic Affairs and Employment.
- Makkonen, T. 2013. “Government Science and Technology Budgets in Times of Crisis.” Research Policy 42 (3): 817–822. doi:10.1016/j.respol.2012.10.002.
- Makkonen, T., S. Gretzinger, R. E. Hjaltadóttir, A. Lorentzen, and R. Shearmur. 2020. “Guest Editorial: Innovation in Peripheries and Borderlands.” Journal of Rural Studies 78: 531–533. doi:10.1016/j.jrurstud.2020.06.030.
- Marceau, J. 2008. “Innovation in the City and Innovative Cities.” Innovation: Management, Policy & Practice 10 (2-3): 136–145. doi:10.5172/impp.453.10.2-3.136.
- Marques, P., and K. Morgan. 2021. “Innovation Without Regional Development? The Complex Interplay of Innovation, Institutions, and Development.” Economic Geography 97 (5): 475–496. doi:10.1080/00130095.2021.1972801.
- McAdam, R., T. McConvery, and G. Armstrong. 2004. “Barriers to Innovation Within Small Firms in a Peripheral Location.” International Journal of Entrepreneurial Behavior & Research 10 (3): 206–221. doi:10.1108/13552550410536780.
- McCann, P., and R. Ortega-Argilés. 2016. “Regional Innovation, R&D and Knowledge Spillovers: The Role Played by Geographical and Non-Geographical Factors.” In Handbook on the Geographies of Innovation, edited by R. Shearmur, C. Carrincazeaux, and D. Doloreux, 22–44. Cheltenham: Edward Elgar.
- Meili, R., and R. Shearmur. 2019. “Diverse Diversities—Open Innovation in Small Towns and Rural Areas.” Growth and Change 50 (2): 492–514. doi:10.1111/grow.12291.
- Mitze, T., and T. Makkonen. 2021. “Can Large-scale R&I Funding Stimulate Post-crisis Recovery Growth? Evidence for Finland during COVID-19.” https://arxiv.org/abs/2112.11562.
- North, D., and D. Smallbone. 1996. “Small Business Development in Remote Rural Areas: The Example of Mature Manufacturing Firms in Northern England.” Journal of Rural Studies 12 (2): 151–167. doi:10.1016/0743-0167(96)00009-5.
- OECD. 2005. Oslo Manual. 3rd ed. Paris: OECD Publishing.
- Oughton, C., M. Landabaso, and K. Morgan. 2002. “The Regional Innovation Paradox: Innovation Policy and Industrial Policy.” The Journal of Technology Transfer 27 (1): 97–110. doi:10.1023/A:1013104805703.
- Pelkonen, A. 2005. “State Restructuring, Urban Competitiveness Policies and Technopole Building in Finland: A Critical View on the Glocal State Thesis.” European Planning Studies 13 (5): 685–705. doi:10.1080/09654310500139319.
- Phillipson, J., K. Bennett, P. Lowe, and M. Raley. 2004. “Adaptive Responses and Asset Strategies: The Experience of Rural Micro-Firms and Foot and Mouth Disease.” Journal of Rural Studies 20 (2): 227–243. doi:10.1016/j.jrurstud.2003.08.006.
- Phillipson, J., M. Gorton, R. Turner, M. Shucksmith, K. Aitken-McDermott, F. Areal, P. Cowie, et al. 2020. “The COVID-19 Pandemic and Its Implications for Rural Economies.” Sustainability 12 (10): 3973. doi:10.3390/su12103973.
- Piekkola, H. 2007. “Public Funding of R&D and Growth: Firm-Level Evidence from Finland.” Economics of Innovation and New Technology 16 (3): 195–210. doi:10.1080/10438590600661897.
- Plank, J., and C. Doblinger. 2018. “The Firm-Level Innovation Impact of Public R&D Funding: Evidence from the German Renewable Energy Sector.” Energy Policy 113: 430–438. doi:10.1016/j.enpol.2017.11.031.
- Pugh, R., and A. Dubois. 2021. “Peripheries Within Economic Geography: Four “Problems” and the Road Ahead of Us.” Journal of Rural Studies 87: 267–275. doi:10.1016/j.jrurstud.2021.09.007.
- Pugh, R., J. Schmutzler, and A. Tsvetkova. 2021. “Taking the Systems Approaches out of Their Comfort Zones: Perspectives from Under Explored Contexts.” Growth and Change 52 (2): 608–620. doi:10.1111/grow.12510.
- Roper, S., and J. Turner. 2020. “R&D and Innovation After COVID-19: What Can We Expect? A Review of Prior Research and Data Trends After the Great Financial Crisis.” International Small Business Journal: Researching Entrepreneurship 38 (6): 504–514. doi:10.1177/0266242620947946.
- Sánchez, A. 1992. “Regional Innovation and Small High Technology Firms in Peripheral Regions.” Small Business Economics 4 (2): 153–168. doi:10.1007/BF00389856.
- Schumpeter, J. 1942. Capitalism, Socialism and Democracy. New York: Harper.
- Schumpeter, J. [1911] 2017. The Theory of Economic Development. Abingdon: Routledge.
- Sen, S., K. Savitskie, R. Mahto, S. Kumar, and D. Khanin. 2022. “Strategic Flexibility in Small Firms.” Journal of Strategic Marketing 1–18. doi:10.1080/0965254X.2022.2036223.
- Shearmur, R. 2012. “Are Cities the Font of Innovation? A Critical Review of the Literature on Cities and Innovation.” Cities 29 (S2): S9–S18. doi:10.1016/j.cities.2012.06.008.
- Shearmur, R. 2015. “Far from the Madding Crowd: Slow Innovators, Information Value, and the Geography of Innovation.” Growth and Change 46 (3): 424–442. doi:10.1111/grow.12097.
- Shearmur, R. 2016. “Why Local Development and Local Innovation Are not the Same Thing: The Uneven Geographic Distribution of Innovation-Related Development.” In Handbook on the Geographies of Innovation, edited by R. Shearmur, C. Carrincazeaux, and D. Doloreux, 432–446. Cheltenham: Edward Elgar.
- Shearmur, R. 2017. “Urban Bias in Innovation Studies.” In The Elgar Companion to Innovation and Knowledge Creation, edited by H. Bathelt, P. Cohendet, S. Henn, and L. Simon, 440–456. Cheltenham: Edward Elgar.
- Shearmur, R., and D. Doloreux. 2016. “How Open Innovation Processes Vary Between Urban and Remote Environments: Slow Innovators, Market-Sourced Information and Frequency of Interaction.” Entrepreneurship & Regional Development 28 (5-6): 337–357. doi:10.1080/08985626.2016.1154984.
- Sigala, M. 2020. “Tourism and COVID-19: Impacts and Implications for Advancing and Resetting Industry and Research.” Journal of Business Research 117: 312–321. doi:10.1016/j.jbusres.2020.06.015.
- Sternberg, R., and O. Arndt. 2001. “The Firm or the Region: What Determines the Innovation Behavior of European Firms?” Economic Geography 77 (4): 364–382. doi:10.2307/3594106.
- SYKE. 2013. “More Accurate Information Available about Urban and Rural Areas.” Accessed October 3, 2021. https://www.syke.fi/en-US/Research__Development/Built_environment/More_accurate_information_available_abou(14636).
- SYKE. 2020. “Kaupunki–maaseutuluokitus päivitetty.” Accessed October 3, 2021. https://www.syke.fi/fi-FI/Avoin_tieto/Kaupunkimaaseutuluokitus_paivitetty_Suom(57423).
- Takalo, T., T. Tanayama, and O. Toivanen. 2013. “Estimating the Benefits of Targeted R&D Subsidies.” Review of Economics and Statistics 95 (1): 255–272. doi:10.1162/REST_a_00280.
- Torregrosa-Hetland, S., A. Pelkonen, J. Oksanen, and A. Kander. 2019. “The Prevalence of Publicly Stimulated Innovations –A Comparison of Finland and Sweden, 1970–2013.” Research Policy 48 (6): 1373–1384. doi:10.1016/j.respol.2019.02.001.
- Vonnahme, L., and T. Lang. 2022. “Hidden Champions in Rural Germany: Innovation Strategies to Compete in Global Markets.” In The Rural Enterprise Economy, edited by B. Leick, S. Gretzinger, and T. Makkonen, 35–52. Abingdon: Routledge.
- Woods, M. 2005. Rural Geography. London: Sage.
- Wooldridge, J. M. 1999. “Distribution-free Estimation of Some Nonlinear Panel Data Models.” Journal of Econometrics 90 (1): 77–97. doi:10.1016/S0304-4076(98)00033-5.
- Wooldridge, J. M. 2010. Econometric Analysis of Cross Section and Panel Data. 2nd ed. Cambridge: MIT Press.
- Yigitcanlar, T., J. Sabatini-Marques, M. Kamruzzaman, F. Camargo, E. Moreira da-Costa, G. Ioppolo, and F. Palandi. 2018. “Impact of Funding Sources on Innovation: Evidence from Brazilian Software Companies.” R&D Management 48 (4): 460–484. doi:10.1111/radm.12323.
Appendix
Table A1. Descriptive statistics for variables in the aggregated municipal-level regressions (2013–2020).