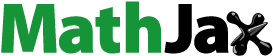
ABSTRACT
Mobility is a global megatrend in our contemporary world as people are constantly crossing nation-state borders for migration, tourism, work and due to mobile transnational lives. Cross-border practices contribute to (re)produce functional border regions between different countries. The current amount of information on the geographies of cross-border mobilities of people, the creation of functional cross-border regions and how these regions change over time is inadequate. As transnational phenomena are fragile to global disruptions and prone to ‘rebordering’ in times of emergency, we lack knowledge of how the COVID-19 crisis affected border practices and functioning regions. We consider mobility to be a tool for understanding society and used big data to examine cross-border mobilities between the five Nordic countries: when and where borders were crossed, how mobilities captured functional border regions, and evaluated the influence of COVID-19 on mobilities and functional border regions from a spatio-temporal perspective. The feasibility of the proposed methodology for monitoring cross-border mobilities and border regions to improve planning and development of border regions and decision-making in future crises is discussed. We studied a 4-year Twitter dataset, including the first year of the pandemic until February 2021. We found that overall cross-border mobility decreased by 68% due to the pandemic, yet with geographical and temporal variations. We showed how the influence of the pandemic on the spatial extent of functional border regions varies for a range of reasons for cross-border mobility. We discuss the feasibility of the proposed approach for monitoring cross-border interactions as the proof-of-concept for capturing functional border regions to improve planning and development of border regions and decision-making in future crises. Finally, we highlight future avenues in enhancing our proposed methodology to improve information on cross-border mobilities derived from social media data such as Twitter.
1. Introduction
Mobility is a global megatrend in our contemporary world as constant mobilities of people transcend nation-state borders and contributes to the network society (Castells, Citation1996; Sheller and Urry, Citation2006). Borders are crossed for migration, leisure tourism, work and education, and seeing family and friends. In particularly, cross-border interactions have been increasing due to transnational people whose daily practices are not confined to one fixed country – including cross-border commuters, multi-local living between different countries and those constantly on the move (Dahinden, Citation2010; Gerber et al., Citation2017; Glick Schiller and Salazar, Citation2013; Hannonen, Citation2020; Järv et al., Citation2021).
These frequent cross-border mobilities and social practices further (re)transform the geographies of social networks and social interactions across borders (Collins, Citation2009). In other words, the bordering practices of people on both sides of a state border contribute to (re)produce new functional transnational spaces (Jackson, Crang, and Dwyer, Citation2004); border regions from different countries forming a functioning system. In Europe, these functional border regions are seen as a key issue towards balanced and sustainable spatial cohesion within the EU (Fries-Tersch, Bradley, and Tugran, Citation2017). The Nordic countries are a prime example in promoting cross-border interactions and integration that has resulted in several well-connected border regions (Schönweitz, Citation2014; Medeiros et al., Citation2021).
However, regardless of its importance, we lack sufficient information on the geographies of habitual and regular cross-border mobilities of people, their temporal dynamics, and trends, and how these form functional cross-border communities (Conradson and Latham, Citation2005; Gerber, Citation2012; Gumy, Drevon, and Kaufmann, Citation2021; Terlouw, Citation2012). The main reason is that traditional methods cannot provide such information on border ignorant socio-spatial phenomena in a timely manner, nor from a longitudinal perspective.
This not only hinders the cross-border planning, development and governance of functional border regions (Allmendinger and Haughton, Citation2009; Moisio, Citation2011) and also causes mismanagement of functional cross-border regions in times of crisis. The COVID-19 pandemic has clearly highlighted how the fragile lifestyles of transnational people and cross-border communities are affected by global disruptions and that they are prone to rebordering by nation-states in times of emergency (Hesse and Rafferty, Citation2020; Järv et al., Citation2021; Klatt, Citation2020; Willi et al., Citation2020). Recent studies indicate how tools using a big data approach could provide timely and valuable information about cross-border mobility and capture the influence of the COVID-19 pandemic and its mitigation measures (Järv et al., Citation2021; Silm, Järv, and Masso, Citation2020; Docquier et al., Citation2021).
To tackle current bottlenecks in knowledge and method in capturing functional cross-border regions, we used a big data approach given that the use of big data as a valid source is thriving in the broad field of social sciences (Kitchin, Citation2014b). We consider digital footprints from mobile devices to be an indication of the mobility of people given that people have adopted mobile devices as a part of their mundane life (Kemp, Citation2019), and the growing literature demonstrates the feasibility of big data sources to reveal human mobility (González, Hidalgo, and Barabási, Citation2008; Järv, Ahas, and Witlox, Citation2014; Silm, Järv, and Masso, Citation2020). In particularly, social media data are suitable for studying cross-border mobility as data are inherently transnational (Hawelka et al., Citation2014).
We further learn from the notion by Kaufmann (Citation2014) that mobility can be used as a tool to understand society and propose that the spatial mobility of people is an indicator for social interaction and that mobilities beyond country borders indicate cross-border interactions. While social interactions form functioning systems (Castells, Citation1996), we can capture functional cross-border regions from mobilities by people across borders.
In this paper, we have sought to contribute to the research on cross-border mobilities and functional border regions through the prism of the spatial mobilities of people with various lifestyles by providing new insights on the changing dimensions in the context of the COVID-19 crisis. For this, we propose a big data approach using Twitter data as a transnational data source and examined the five Nordic countries as the proof of concept for capturing cross-border interaction. With this paper, we aim to (1) provide quantitative evidence on overall cross-border mobilities by revealing when and where borders are crossed; (2) demonstrate how functional cross-border regions can be delineated according to the mobilities of people and (3) evaluate the influence of the COVID-19 crisis on cross-border mobilities and functional border regions from spatial and temporal dimensions.
Finally, we discuss the feasibility of the methodology adopted to implement the monitoring of cross-border mobilities and functional regions, and in the case of the COVID-19 crisis, from a longitudinal perspective. We further discuss how this information can improve the planning and development of border regions in general, and how the knowledge obtained can enhance decision-making in the Nordics.
2. Background
2.1. Mobilities crossing country borders and COVID-19
Human mobility can take many forms (e.g. terrestrial, aerial and digital) from several dimensions (e.g. physical, social and virtual), thus comprising a broad set of human mobilities (Adey, Citation2010; Kellerman, Citation2011). In general, mobilities are affected by several push and pull motivations that result in both an individual's willingness and ability to move, in addition to accessibility–the potential of opportunities for interaction provided by the society (e.g. infrastructure). In this study, we focused on spatial (physical) mobilities and used CitationKaufmann's (Citation2014) proposition about using mobility as a tool to study societies.
From an individual perspective, mobilities are fundamental components of an individual's activity spaces that take place between activity locations (Schönfelder and Axhausen, Citation2010; Järv, Ahas, and Witlox, Citation2014). Thus patterns of spatial mobilities reveal how and where an individual's daily lives take place regarding various mobility forms between certain activity locations. By incorporating time, mobilities reveal the temporal dynamics of people and changes in a life course (Frändberg, Citation2008; Järv, Ahas, and Witlox, Citation2014). The structure of spatial mobilities even reveals the structure and the geography of an individual's social interactions (Axhausen, Citation2007; Puura, Silm, and Masso, Citation2022).
By aggregating individual mobilities, the overall flows of mobilities reveal urban structures, spatial hierarchies of settlement structures, and from the country border context, it reveals the overall structures of mobilities across country borders (Silm, Järv, and Masso, Citation2020). Cross-border mobilities are multi-faceted, including forms of migration (permanent or temporary and forced or voluntary), tourism travel for leisure and work, visiting family and relatives or for daily life practices of transnational people (Hall, Citation2005).
Previous research on the groups crossing borders has mainly relied on surveys, register data and interviews. For example, Möller et al. (Citation2018) combined data from several surveys to reveal the spatial pattern of cross-border commuters between Norway and Sweden and their mobility motivations. Wiesböck et al. (Citation2016) used mixed methods to combine several data sources to examine cross-border commuting in central Europe from the perspective of global crises. Using register databases from the United Nations and the World Bank, Delhey et al. (Citation2019) examined the long-term evolution of migration flows in Europe.
Each form of cross-border mobility can be studied separately to understand each given phenomenon. However, examining the overall cross-border mobilities using continuously evolving data sources and applying Big Data approaches, as in this study, reveals connectedness and overall interactions as an indicator of social interactions between border communities across country borders at a larger scale and with more frequent up-to-date data. Thus detailed characteristics of the overall cross-border mobilities can indicate dominant mobility types. For example, Gabrielli et al. (Citation2019) captured trends in transnational mobility flows and reveals the dominant mobility types between countries using global air passenger traffic data.
The COVID-19 crisis has disrupted all forms of cross-border mobility differently (Järv et al., Citation2021; Docquier et al., Citation2021; Lara-Valencia et al., Citation2020). However, given the measures introduced to tackle the virus spreading in 2020–border closures and strong recommendations not to travel (abroad) – affected the overall cross-border mobilities, in general, and similarly in Europe (Poom et al., Citation2020; Hale et al., Citation2021). Thus disruptions and structural changes in the overall cross-border mobilities per se captures changes within border communities across borders.
2.2. From moblities to functional border regions
The concept of functional regions is old and is inherently linked to spatial mobility (e.g. Berry, Citation1968; Brown and Holmes, Citation1971; Goddard, Citation1970; Philbrick, Citation1957). By proposing an objective approach, the construction of the functional region corresponds to one of the last steps of the regionalization concept as identified by Paasi (Citation1991), after the stages of the territorial, symbolic and institutional region. Based on this reflection and other pioneering work, we consider the functional region to be an organizational structure based on spatial interactions (e.g. social interactions and movements of people), a certain internal autonomy and two founding principles, namely the principle of external separation and the principle of internal cohesiveness (see Klapka and Halás, Citation2016, for a review).
Thus, depending on the spatial interactions involved, the sum of internal flows within the functional region must generally be greater than the sum of external inter-regional or, depending on the context, transnational flows (Jackson, Crang, and Dwyer, Citation2004). In some cases, these transnational or cross-border flows become the majority of regional internal flows. For example, this phenomenon may be linked to a stronger attractiveness on the other side of the border due to wages (Dubois, Citation2017; OECD, Citation2013) or more interesting consumption or appealing leisure practices (Spierings and Van Der Velde, Citation2008). These differentials are often observed in the European context, because the border no longer acts only as a barrier but also as a resource by facilitating cross-border mobility (Sohn and Licheron, Citation2018).
However, the functional border region does not have to be defined solely from the context of intra-national mobility flows (like in gravity models), but it can be defined from the perspective of the border. Here, we utilize the flow approach by van Houtum (Citation2000) as one way to study border regions. Different reasons to move across borders may create different patterns of mobility flows. Considering overall cross-border mobilities, regardless of the reasons for moving or what lifestyles these people have, is another indicator of the general connectedness across borders. In the recent literature, this approach has been seen by other scholars as a solid way for capturing functional border regions (Drevon et al., Citation2018; Durand, Citation2015; Möller et al., Citation2018).
Functional border regions can further reflect the state of spatial integration across borders (Vandermotten, Citation2010; Decoville et al., Citation2013). As we can recall, the recent construction of Europe was mainly envisaged on the basis of a common economic market through the creation of the European Economic Community (EEC) with the Treaty of Rome in 1957. Initially political, this integration process reflects the place left to economic power. Thus with the help of a market that favours and facilitates exchanges and flows between the founding European countries, the decision-makers hoped to accelerate the political and institutional integration of the continent. These exchanges and flows (essentially of goods and people) can be considered as the premises of the functional integration of border regions.
Functional integration in Europe is further accelerated by the creation of European institutional instruments such as the European Regional Development Funds (e.g. via INTERREG; see Cavallaro and Dianin, Citation2019), exchange facilities linked to the creation of a European Grouping of Territorial Cooperation (Schiebel, Omrani, and Gerber, Citation2015), while the European currency being undoubtedly an additional undeniable catalyst. In the European Union, around 390,000 cross-border workers were recorded at the start of the 1990s. Their stable yearly increase rate started to increase rapidly after the consequent enlargement of the EU since the 2000s. In 2015, around 1.7 million cross-border workers were recorded – nearly 1% of the overall EU-28 workforce (Fries-Tersch, Bradley, and Tugran, Citation2017).
However, the question of measuring and managing cross-border flows in the functional region often remains difficult. Certainly, the national borders and local administrative limits usually do not capture the functional extension of an employment cross-border area (Brunet-Jailly, Citation2005). Moreover, transportation jurisdictions are generally smaller than the employment area shaping passenger mobility. Understanding the functional delineation of a region becomes even more complex when it straddles more than one border between two countries, whereas these functional aspects must remain at the heart of territorial management (Thomas et al., Citation2017).
Even if cross-border studies are numerous in some regions, such as Luxembourg, comparable, permanent and reliable cross-border mobility data or surveys remain limited and sometimes require difficult and cumbersome matching (Godefroy et al., Citation2021). It is then possible and appropriate to use other sources of data, which are more easily comparable due to the same collection protocol on both sides of the border (e.g. Schmitz, Drevon, and Gerber, Citation2012; Enaux and Gerber, Citation2014). Thus novel data sources such as Twitter data could be one solution to address this issue.
2.3. Novel data sources to reveal functional border regions
Traditionally, mobility research and cross-border mobility have been studied using official commuter statistics or survey data (Schönfelder and Axhausen, Citation2010). These kinds of data are often times consuming to create, expensive and limited in scope (Silm et al., Citation2020). In the realm of cross-border mobility, traditional data sources are often limited to single jurisdictions and consolidation of data across country borders is a difficult process. As an example, the Nordic Council of Ministers stated in 2021 that the current state of official cross-border statistics 'cannot be used to reflect on the movements across borders' (Nordic Council of Ministers, Citation2021, 12).
Novel big data sources, and especially social media data, can provide up-to-date information spanning country borders and are not limited to a certain group of workers or type of mobility. Big data sources are characterized as being flexible, exhaustive in scope, fine-grained and ever evolving (Kitchin, Citation2014a). These characteristics are some of the reasons behind the increasing use of novel data sources in research. These data sources have been proven to be a reliable source in capturing information on human mobility, amongst others. Crowd size quantification (Botta, Susannah Moat, and Preis, Citation2015), traffic flow analysis (Järv et al., Citation2012) and cross-border movement (Blanford et al., Citation2015; Hawelka et al., Citation2014; Jurdak et al., Citation2015) have all been reliably researched using social media data.
Social media data have also been used in delineating functional regions. Poorthuis (Citation2018) delineated neighbourhoods using Twitter data, and Novak et al. (Citation2013) studied regionalization with mobile phone data. They both exemplify the opportunities that lie within big data to delineate spatial regions from a person-centric mobility focus. However, to our knowledge functional cross-border regions have not previously been revealed in the Nordic context, which we demonstrate in this article, is possible with the data available today.
As with any other data source, Twitter data displays imperfections and limitations that need to be considered when analysing results using such data. For example, in case of representativeness, Tasse and Hong (Citation2014), Hultquist (Citation2020), Mellon and Prosser (Citation2017), all show various aspects of the biased representativeness in Twitter data – predominantly young, male users, yet this may vary by country and across cultures. However, ongoing enhancements in method development (e.g. Hasnat and Hasan, Citation2018; Heikinheimo et al., Citation2022) can provide several avenues to tackle existing limitations of social media data in cross-border research (see Discussion section for further discussion).
3. Case study – the Nordic countries and COVID-19
The Nordic countries are an excellent setting for studying cross-border mobility and the forming of cross-border regions on both sides of country borders that function as one system. The Nordic countries – Denmark, Finland, Iceland, Norway and Sweden – are a connected region within a relatively small geographical area. Through a history of close cooperation, open borders and shared labour markets have been broadened since the inception of the Nordic Council in the 1950s (Perkmann, Citation2003).
To date, the Øresund region between Denmark and southern-Sweden is the densest cross-border region and around 7.5 million motor vehicles crossed the Øresund bridge each year before COVID-19 (CitationØresundsbron, Citationn.d.). With a lesser magnitude in mobility, Tornio-Haparanda in northern parts of Finland-Sweden, and the Norway-Sweden region connecting areas between Oslo and Gothenburg (GO-region) are well-functioning regions. The northern parts of Norway, Sweden and Finland that cover larger areas with scarce population densities, form the Nordkalottrådet region where locals freely move across borders (Schönweitz, Citation2014). Previous research on cross-border mobility in the Nordic region has focused on innovation (Sæther, Terje Andersen, and Hagen, Citation2019), tourism (e.g. Slätmo et al., Citation2019), migration (e.g. Lundborg, Citation1991), while a few studies on cross-border regions (e.g. Löfgren, Citation2008).
With the COVID-19 crisis since March 2020, travel restrictions were among the first measures set in place in the Nordic countries, limiting mobility across borders, requiring testing and isolation upon entry, with different regulations for commuters and logistics in the different countries. However, the countries had different strategies, which also varied over time, as indicated by time series of the stringency index (). The composite index comprising several containment and closure policy indicators (e.g. on internal and international travel) indicates the overall ‘strictness of “lockdown style” policies that primarily restrict people's behaviour’ (Hale et al., Citation2021).
Figure 1. The stringency index of the five Nordic countries during the COVID-19 study period from March 2020 until February 2021. Data obtained from Hale et al. (Citation2021).

In addition to an overall decrease in daily habitual mobility both globally and in the Nordic countries (Santamaria et al., Citation2020), there were also changes in mobility structures due to COVID-19. As an example, people escaped from urban areas to second homes in Finland (Willberg et al., Citation2021) and to a large extent due to the ability to work remotely. Overall, more people started to work remotely, yet it varied significantly by region (Grunfelder, Citation2020), mainly depending on the structure of the regional labour force. Increased remote work and multi-local living meant less mobility to urban centres for shopping, leisure and entertainment and the activities within these centres (Google, Citation2020).
Yet, empirical studies of how and where cross-border mobility takes place in the Nordic region are not well established, and the effects of the COVID-19 pandemic on this mobility is yet to be documented. In this article, we provide insights on empirical findings to shed light on this topic.
4. Methods
Our data were collected using the tools created by Väisänen et al. (Citation2021) and stored in accordance with General Data Protection Regulations (European Council, Citation2016). The study period consists of 4 years from 01.03.2017 to 28.02.2021 and we initially collected a Twitter dataset consisting of 22,805,047 geolocated tweets located within one of the Nordic countries by 405,326 unique users. In our initial data, the tweets were spread across the Nordic countries with 44% of the tweets in Sweden, 23% in Finland, 15% in Norway, 14% in Denmark and 4% in Iceland.
After collecting, processing geo-located tweets and excluding bots in the workflow (), we grouped tweets by user and ordered them chronologically for detecting movements within each year of our study period. As Twitter use is not frequent, especially geotagging Tweets, we used a loose definition of movement criteria–time duration of less than 180 days was considered to be movement between two consecutive tweets. Despite a loose definition and the fact that we may miss additional movements taking place during our ‘movement’, we are confident that this approach revealed the structure of cross-border mobilities as indicated by the existing literature (Hawelka et al., Citation2014; Mazzoli et al., Citation2020).
Finally, we selected only movements that cross country borders – both origin and destination of a movement are within one of the Nordic countries, but not in the same country. We identified a total of 167,130 cross-border movements by 53,252 unique users who crossed country borders during our 4-year period ().
Table 1. Descriptive statistics of initial and weighted data from the study period.
To narrow down the potential influences of external factors such as the temporal fluctuation of social media use and policy changes in Twitter regarding geolocation (Hu and Qian Wang, Citation2020) in our analysis, we weighted the data. We calculated the yearly weight coefficient based on Twitter usage (Tweets per user) and total Tweet count by studied year, so that the derived mobility by year is comparable. We consider the first study year having a weighting coefficient as 1 (). We used weighted data for our analysis by referring to ‘observed’ mobility, in which we considered mobility from the period 03.2017 to 02.2020 as one baseline year (also referred to as BL AVG hereinafter).
We used a kernel density estimation method to delineate functional cross-border regions for each of the time periods based on observed mobility. For that, we selected a cross-border region pair at NUTS 2 level at a time and selected end points of identified observed cross-border mobility. We first selected movement endpoints only from one side of the border and calculated kernel density, and next from the other side, accordingly. We did this for each NUTS 2 level region pair and created four threshold classes indicating areas where mobilities of people are concentrated the densest – 25%, 50%, 75% and 95% of all cross-border mobilities.
For analysis, we first used a difference in differences (DID) research design to reveal the causal effect of the COVID-19 pandemic and its mitigation measures on observed cross-border mobility, in general and for each month. In the DID analysis, we considered our pre-treatment period (t1) as BL 3 (01.03.2019–28.02.2020) and the post-treatment period (t2) as the COVID-19 period (01.03.2020–28.02.2021). We considered our treatment data as the observed (weighted) cross-border mobility data and for capturing the influence of the COVID-19 pandemic. For control data, we used a linear regression model based on the observed mobility from the first three baseline years (01.03.2017–28.02.2020) to obtain ‘estimated’ cross-border mobility for the entire period being studied. We assumed that estimated cross-border mobility would have followed our model estimation in the case that the COVID-19 pandemic had not occurred.
Second, we compared the change in observed mobility between the average baseline period (BL AVG) and the COVID-19 period (03.2020–02.2021). To test whether the structural distribution of cross-border mobility between the Nordic countries and distances travelled were statistically different during the COVID-19 period, we used nonparametric comparative statistics at the level of p<0.01 as our data are not normally distributed. We further compared the temporal dynamics of observed cross-border mobility flows between country pairs by weekday and month.
Finally, we compared functional cross-border regions between the whole baseline period (BL AVG) and the COVID-19 period by measuring the area of the regions by mobility threshold criteria and used a map overlay method to measure proportional differences – how much the geographical extent of a functional region was lost and gained during the COVID-19 period compared to the baseline (BL AVG). All our codes for data collection, data processing and analysis steps are written in the Python programming language and are publicly available at GitHub for the reproducibility or our study and further development of our approach (https://doi.org/10.5281/zenodo.6861319). In the next section, we present the results of our empirical study, while our interpretation of the results is presented and discussed later in the Discussion section.
5. Results
5.1. Changes in spatial patterns
At the overall level, the cross-border mobility between the Nordic countries decreased by 68% due to the COVID-19 crisis, according to our difference in differences calculation (). A significant effect of COVID-19 also varied between country pairs. Decreases in cross-border mobility ranged from −35% to −82%, while the most significant COVID-19 effect was mobility linked to Finland and the least effect was linked to Iceland.
Table 2. Changes in cross-border mobility based on DID analysis between baseline year 3 (BL3) and the COVID-19 period.
To a small degree, the varying effect of the COVID-19 crisis on cross-border mobility changed the spatial structure of overall cross-border mobility flows between the Nordic countries (), although the Wilcoxon signed rank test confirms that the relative distribution of cross-border mobility flows at country level during the COVID-19 period was not statistically different (p = 0.508). Still, as reflected by the results above, while the proportion of mobilities linked to Sweden and Denmark had the largest decrease, then the proportion from overall mobilities decreased the most regarding mobilities between Sweden and Finland (). In contrast, the proportion of mobility between Norway and Finland had the largest increase.
Table 3. The structural distribution of cross-border mobility flows before and after the COVID-19 crisis, and the difference in percentage points.
At more geographically detailed (NUTS 2 region) level we see more variation. The largest decrease (−4.2 percentage points) was between Stockholm (SE11) and Helsinki–Uusimaa (FI1B) – its proportion decreased from 7.9% to 3.7% from the overall mobility in the Nordics. The proportions of cross-border mobility flows increased the most amongst flows that were related to the northern parts of the regions (FI1D and NO07). Also, the busiest mobility flow between NUTS 2 regions, the areas around Copenhagen (DK01) and Malmö (SE22), increased its relative proportion – from 13.5% to 15.4%. The Wilcoxon signed rank test confirms that the relative distribution of cross-border mobility flows at NUTS 2 level during the COVID-19 period is statistically different ().
Changes in functional cross-border regions are also reflected by the changes in the lengths of mobilities across borders between different countries (). Even though Mann–Whitney U-tests confirm that the distribution of overall cross-border mobilities by length during the COVID-19 period remain the same as before (p = 0.247), then the change is still significant between country pairs. Exceptions here are mobilities between Finland–Norway (0.336) and to a lesser extent Iceland-Sweden () and Denmark–Norway (p = 0.014).
Table 4. Changes in cross-border mobilities distances before and during the COVID-19 period.
5.2. Changes in temporal dynamics
Another aspect in examining the effect of the COVID-19 crisis on cross-border mobility is from a temporal perspective. Based on DID analysis for 2020 at the monthly level, we captured the effect of the COVID-19 pandemic on decreasing cross-border mobility since March 2020 while controlling seasonal (monthly) variation (, left). The comparison of yearly proportional distribution of cross-border mobilities by month between the average baseline year (BL AVG) and the COVID-19 period shows deviations from expected seasonal variations. The concentration of mobilities emerged in March as the first large restrictions on travel in the Nordic countries were set in place during and after mid-March 2020 – thus the effect of these restrictions is seen in the drop in mobility from March until July (, right). By increasing restrictions in November 2020 and again in January in Denmark and Sweden show the decrease of cross-border mobilities.
Figure 3. The effect of COVID-19 on cross-border mobility at monthly level based on DID analysis (left). The comparison of yearly proportional distribution of cross-border mobilities by month between the average weighted baseline year and the COVID-19 period (03.2020–02.2021).

The comparison of annual proportional distribution of cross-border mobilities by month between the average baseline year (BL AVG) and the COVID-19 period (03.2020–02.2021) varies between countries (). For example, the monthly distribution of cross-border mobilities between Sweden and Norway did not change significantly, whereas mobilities between Finland–Sweden and Finland–Norway had disrupted patterns (see Discussion section 6.1. for reasoning). Mobilities between Denmark and Sweden were clearly concentrated in July–October. These findings coincide with expected seasonal rhythms found both at aggregated global mobility flows (Gabrielli et al., Citation2019) as well as at individual travel behaviour (Järv, Ahas, and Witlox, Citation2014) resulting in more mobility in the summer linked to the use of second homes and the timing of summer vacations. For a more detailed overview of the mobility between country pairs, see Appendix.
Figure 4. The comparison of yearly proportional distribution of cross-border mobilities by month between the average weighted baseline year and the COVID-19 period (03.2020–02.2021) regarding mobilities between Norway–Sweden, Sweden–Finland, Denmark–Sweden and Finland–Norway.

We also captured changes in weekly rhythms of cross-border mobilities. In general, mobilities across borders during the COVID-19 period are evenly distributed by weekday, whereas before, mobilities were concentrated on Thursdays–Fridays ().
Figure 5. The comparison of weekly proportional distribution of overall cross-border mobilities by weekday between the average weighted baseline year and the COVID-19 period (03.2020–02.2021).

However, weekly rhythms of mobilities change between different countries due to the COVID-19 crisis (). Mobilities between Norway and Sweden still followed the same weekly rhythms, but with higher concentration on Thursday–Friday. Weekly rhythms did not change regarding mobilities between Denmark and Sweden, whereas mobilities between Finland and Sweden shifted from workdays to weekend. Interestingly, the routine weekly rhythm of mobilities between Norway and Finland has been disrupted significantly (see Discussion Section 6.1 for reasoning).
5.3. Changes in functional cross-border regions
We also found spatial changes in the delineations of functional cross-border regions based on the geographical distribution of cross-border mobilities in the Nordic countries. Focusing on three examples, we can see a significant decrease of the extent of the cross-border region around the border cities of Tornio and Haparanda in northern Sweden and Finland (). Based on the distribution of the densest 75% of all mobilities the spatial extent of the region decreased by −77% (−71% on the Swedish side and −83% on the Finnish side), and with 25% and 50% mobilities' threshold levels, the decrease in area were the same (−73% and −80%, accordingly). This coincides with a decrease in movement distances. Mean distance for cross-border mobilities between these regions decreased by half from 197 km to only 98 km during the COVID-19 period. Furthermore, median distance decreased even more, −79% from 173 km to only 36 km, indicating that existing mobilities were concentrated close to the border (see Discussion Section 6.1. for reasoning).
Figure 7. Functional Cross-border Regions between Finland (NUTS 2 region FI1D) and Sweden (NUTS 2 region SE33) before (a), during the pandemic (b), and how region has spatially changed between two time periods (c).

The region between Gothenburg and Oslo experienced a different trend in the COVID-19 period, with a larger increase in spatial extent. In case of the densest 75% of all mobilities, the spatial extent of the region increases by 120% (). While from the Norwegian side it increases by 135% and from the Swedish side it is 112%, this increase mainly surrounds the cities of Oslo and Gothenburg and the areas south of Strömstad in Sweden. Interestingly, both mean and median distance for cross-border mobilities between these regions do not change–initially 222 km and 254 km, and during the COVID-19 period it is 222 km and 246 km, accordingly.
Figure 8. Functional Cross-border Regions between Norway (NUTS 2 region NO08) and Sweden (NUTS 2 region SE23) before (a), during the pandemic (b), and how region has spatially changed between two time periods (c).

In the third example, cross-border mobilities between Denmark and the three southernmost regions of Sweden also indicated a modest increase in cross-border region extent. In case of the densest 75% of all mobilities, the spatial extent of the region increased by 49% (), however, the increase on the Danish side was 141% while on the Swedish side the increase was only 36%. The overall increase was the same at the 50% threshold level (54%), but even more evident when focusing on the 25% threshold level (97%). The mean distance for cross-border mobilities between these regions marginally decreased (−5% from 101 km to 96 km), while median distance (48 km) remained the same during the COVID-19 period.
Figure 9. Functional Cross-border Regions between Denmark and Southern Sweden (NUTS 2 regions SE21, SE22 and SE23) before (a), during the pandemic (b), and how region has spatially changed between two time periods (c).

Across the different region pairings, we can observe that the mobility pattern tends to follow the power-law pattern, with the distances travelled seemingly concentrated around specific geographical centres on either side of the border (Deutschmann, Citation2016). These observations are also in line with the overall mobility changes that Deutschmann notes are rather a ‘ regionalization’ than ‘ globalization’ (Deutschmann, Citation2016, 133).
6. Discussion and conclusion
With this study, we propose a relatively simple and straight forward big data approach to capture and monitor actual mobilities of people and functional regions. Similar approaches have already been showcased in existing literature (Silm, Järv, and Masso, Citation2020), yet we utilize an original angle by considering the given phenomena across country borders. Our empirical study from the Nordic countries has highlighted how this approach can provide new insights by using geo-located Twitter data. Next, we focus on three aspects obtained from the study: (1) addressing key insights captured from the Nordic countries; (2) discussing the feasibility of a big data approach and (3) proposing future directions for this study topic with concluding remarks.
6.1. Capturing mobilities, functional border regions and the influence of the COVID-19 pandemic
By considering mobility as a tool to examine societal processes and phenomena, we revealed the characteristics of interactions between cross-border regions regarding overall quantities, the spatial structure, and distances of mobilities at various spatial (national, regional) scales. This allowed us to delineate functional cross-border regions from individuals' perspectives. Not the least, we revealed the temporal dynamics of cross-border phenomena from various (e.g. weekly and monthly) temporalities, and detected disruptive effect by the COVID-19 crisis affecting the routine rhythms and patterns across borders.
The COVID-19 pandemic affected the flows of cross-border mobilities in the Nordic countries, yet with varying outcomes between countries (). With monthly aggregation levels, we captured the beginning of the crisis in the Nordic countries (, left). The varying levels of decrease in mobilities coincides, to a large degree, with the strength of restricting mobility measures put in place by countries according to the Stringency index (). In case of the whole studied COVID-19 period (March 2020–February 2021), the least decrease was in mobilities related to Iceland due to country's less strict restrictions, whereas the biggest decrease was in mobilities related to Finland due to country's most strict restrictions compared to other countries.
We depicted some structural changes regarding the spatial behaviour in cross-border mobilities between the Nordic countries. From a spatial perspective, we observe less changes in the composition of mobilities at a country level, whereas clear changes at a regional (NUTS 2) level. Restrictions in ferry and flight routes affected the largest proportional decreases in mobilities (e.g. between Finland–Denmark and Finland–Sweden), whereas cross-border mobilities by land decreased the least (e.g. Copenhagen–Malmö connection).
Analysis also revealed the varying cross-border movement restrictions by region within one country affecting mobilities, such as in Finland – the largest increase in the proportion of mobilities crossing borders was between the northern parts of Finland and Norway, whereas the largest decrease was in the southern parts between the Helsinki–Stockholm regions. This also coincides with Finnish border restriction measures. The Finnish government had more flexible restrictions on cross-border working and tourism in the northern regions than elsewhere in the country (Yle, Citation2020b; Järv et al., Citation2021). Also, the border between Norway and Finland re-opened mid-June 2020, while the border between Sweden and Finland remained closed, something that led to a rapid increase of visitors from Finland to Norway in July (Yle, Citation2020a). Thus our approach was able to capture these dynamic changes in border crossings between Finland and Norway.
We analysed overall cross-border mobilities, however, some robust assumptions about reasons behind cross-border mobility flows could be made. While leisure travels have a clear seasonal rhythm and concentrating on weekends, in general, then work-related travelling occurs evenly in workdays throughout the year while weekly commuting is likely to happening from Monday to Thursday or Friday. The overall mobilities distribution by weekday from the whole study region shows a decrease of workday mobilities, thus suggesting less work-related travels than before the pandemic. This links to the overall trend, where people shifted to remote work in the Nordic countries (Grunfelder, Citation2020).
More precisely, for example, rather evenly distributed Norway–Sweden mobilities in weekdays before the pandemic, concentrated to travels on Mondays and Fridays during the pandemic – possibly indicating less weekend leisure travel and a shift from daily commuting to a pattern of more workweek commuting (). The weekly mobilities pattern between Finland–Sweden and Finland–Norway showing mobilities concentration in weekends might indicate that work-related mobility was affected more by the pandemic than weekend leisure travelling. In contrast, the weekly mobilities patterns between Denmark and Sweden suggest equal effect of the crisis on mobility types.
The three examples of functional cross-border regions revealed how the geographical interactions within the Nordic region changed due to the pandemic – the functional region around Tornio–Haparanda shrunk significantly, yet the regions around Oslo–Gothenburg and Copenhagen–Malmö expanded. Differences could possibly be explained by varying mobility types and use of transport mode. In the Tornio–Haparanda region (), all mobilities crossing borders are done by land transport and thus the region does not have regional variations by transport mode due to varying mobility restrictions. Yet, the drastic decline in median travel distances () indicate that local travel remained while long-distance (leisure-oriented) travels ceased to exist during the pandemic.
In contrast, mobilities between Denmark and Southern Sweden (i.e. mainly the Øresund region) did not change regarding travel distances, thus indicating flows of mobilities had no structural changes during the pandemic. Yet, the increase of spatial extent of most cross-border mobilities () indicates the overall avoidance of core urban centres (Willberg et al., Citation2021; Google, Citation2020) and thus having spatially more dispersed cross-border interactions than before. The increased spatial extent of the region between Oslo and Gothenburg could be further linked to varying mobility restrictions by transport mode – with the reduction in flight connections between the two urban centres meaning less dominant cross-border interactions concentrating to these cities. Interpreting the changes regarding distances between different country pairs () remains challenging without a more enhanced methodology. Yet, factors like the geographical setting (border on land or water) and settlement structure (distance between the main urban hubs across the border) of a border region, in addition to the dominant mode of transport and mobility type certainly affect these spatial changes in mobility.
Overall, our results show that functional structures vary in time and space and reflecting that the relativity of flows of people as an indicator for interaction can reflect the (de)construction of the attractiveness of cities and territories. Changes in spatial structures of cross-border mobilities – e.g. urban–rural and border city-catchment area nexus, and varying changes by country – indicate changes in social, economic, and political interactions within cross-border communities. Hence, changes in spatial structures of cross-border mobilities will, in turn, impact various cross-border phenomena such as policies and regulations, individual habits and daily routines (e.g. teleworking, transport mode) and overall interactions within cross-border communities, especially during and after the COVID-19 pandemic (Dijst et al., Citation2021). Big data approach can help in monitoring these structural changes in cross-border regions, and potentially help in situating and contextualizing the events of the next global crisis from the cross-border perspective in near-real time. Our findings about the spatial structure of cross-border mobility flows tend to follow a power-law pattern, as found in the case of broad transnational mobility (see Deutschmann, Citation2016), and could be further examined in future research.
6.2. The feasibility of a big data approach – strengths and limitations
This study highlights the need for cross-border (mobilities) research to overcome existing challenges like the lack of timely data, the cost of collecting appropriate data and geographically and temporally limited study scopes. As such, our simple and robust methodology focusing on overall cross-border mobilities already highlights the feasibility of using a big data approach and social media data (e.g. Twitter) in providing efficient and up-to-date insights. The methodology can be used both for a monitoring tool to evaluate long-term changes and trends of cross-border phenomena, and also detect any abnormalities and disruptions in a timely manner. With the ease of processing data and timeliness in providing information, the methodology used is both low-cost and easy to replicate and reproduce in other cross-border areas globally. That said, the proposed approach has its limitations and biases generic to social media data as well as specific to our current methodology that should be acknowledged and tackled in future studies.
The first challenge with the current methodological framework is its straightforward generic approach focusing on overall flows of cross-border mobilities. This does not allow us to reveal the reason for the observed mobilities nor distinguish people with various lifestyles to specify certain types of mobility flows. A more detailed framework combining an individual activity space approach (Järv, Ahas, and Witlox, Citation2014) can reveal the reasons behind mobilities by following the concept of ‘make big data small and meaningful’ (Poorthuis and Zook, Citation2017). First, this helps to detect residences of individuals (Heikinheimo et al., Citation2022) and second, their spatio-temporal behaviour over a longer period can reveal routine patterns and interpret reasons for specific mobility. Thus, characterizing individuals' repeated mobility frequencies, duration and timing can distinguish between daily commuting, weekend leisure travelling, seasonal tourism and second home mobility in a more activity-based approach. Not only can this provide insights into specific lifestyle mobilities, per se, but offers a holistic understanding of functional border regions -- what are the reasons for people interacting across borders and how different (multi-layered) functional regions are formed by people with different lifestyle mobilities.
The second challenge is the representativeness of data due to the biases inherent in social media regarding the features of the people we study – for example, place of residence, gender, age, socio-economic and cultural background (Mellon and Prosser, Citation2017; Toivonen et al., Citation2019). The relevance of the issue increases when studying more specific topics and/or at geographically very detailed (e.g. neighbourhood) level. It has been shown that representativeness of findings from Twitter data can be improved, to some extent, by deriving demographic and socio-economic characteristics from social media content and users' profiles (Sloan et al., Citation2015; Huang and Wong, Citation2016). Residence locations detection (e.g. Heikinheimo et al., Citation2022) enables people to be distinguished in categories such as local people from border regions, other locals (e.g. people from the Nordic countries) and foreigners, which also helps to interpret their reasons for mobility. The implementation of these improvements requires additional contextualized data processing models that to our knowledge have not yet been developed for the Nordic countries. Thus, we are unable to use these enhancements in this study, but we address the key enhancements for improving the representativeness of such data in Section 6.3.
The third challenge, as with any big data source, is the issue of privacy and data availability. The latter depends on existing regulations (e.g.GDPR in the EU) and largely on the policies of data a provider company (Hu and Qian Wang, Citation2020). However, even if open access to some social media data platform will be terminated, then digital data still exists in databases and could possibly be used through other arrangements for societal good (e.g. public–private partnership). Regarding the former, preserving privacy is one inherent challenge when working with individual level social media data and thus the paramount cornerstone for any big data analytics. By following responsible big data analytics (Zook et al., Citation2017) and presenting geographical data at aggregated (e.g. NUTS 2 region) level, the proposed approach is feasible without harming individuals' privacy for societal good (Poom et al., Citation2020).
6.3. Conclusion and perspectives
This study highlights the need for cross-border (mobilities) research to overcome challenges like timeliness and temporally limited scope of existing data collection and analysis, while showing the potential of a big data approach to mitigate this research gap. By efficiently capturing cross-border mobilities, social media data provides a promising opportunity to understand functional cross-border regions based on actual interactions of people and further contribute to policy and decision-making.
Nevertheless, to understand specific cross-border mobility due to different lifestyles (e.g. commuting, second home tourism, shopping) and individual-level interactions between communities across borders in a more nuanced way, the research focus needs to move beyond the overall cross-border mobilities. For that, several developments will be necessary, especially from a methodological point of view to implement the enhancements mentioned above (see Section 6.2). We suggest four avenues to improve information on cross-border mobilities derived from social media data such as Twitter.
First, capturing users' place of residence by deriving their residence from spatio-temporal behaviour (or from user profiles and social content) would enable distinguishing locals from foreign tourists (Hasnat and Hasan, Citation2018). Second, extending the study period would enable better capturing of individual's' spatio-temporal behaviour to infer reasons behind mobilities, such as recurring leisure visits to given locations (Järv, Ahas, and Witlox, Citation2014). Third, enriching social media data with other data sources could further help to infer reasons for mobilities and provide a nuanced division of the mobilities within each cross-border region. For example, contextual information about the location where Tweets were posted (e.g. land use; characteristics of a municipality) combined with users spatio-temporal behaviour could provide better possibilities to profile mobility types and forms (Huang and Wong, Citation2016).
Fourth, deriving user background information from social media posts using content analysis has the best potential to reveal socio-demographic information behind cross-border mobilities such as age groups, gender, indicate lifestyle and occupation type, yet it is the most challenging to obtain (e.g. Huang, Ebert, and Rider, Citation2017). The implementation of the four advancements (especially the last) could contribute to improve the representativeness of the research and enable more multi-layered modelling of cross-border mobilities by people with different lifestyles. Further, our attempt to capture functional cross-border regions based on observed cross-border interactions of people could be further enhanced by including non-border crossing mobilities within countries in the analysis. This way, the complete mobilities perspective would enable a better contextualization around cross-border mobilities and thus delineate functional cross-border regions using a traditional OD-matrix approach. This, along with the other method developments mentioned above, would open avenues for capturing socio-economic patterns and disparities within and between cross-border regions, and how people from different socio-economic backgrounds and lifestyles are affected in times of border closures.
Interestingly, systematic research with comparable transnational surveys (and registers) focusing on cross-border workers (and daily lives of local cross-border people) have so far received little attention in the Nordic countries and the official statistics is not in a suitable state for analysis, compared to for example Luxembourg and its cross-border region (Gerber et al., Citation2020). A big data approach could offer a basis for providing systematic information about border regions in the Nordic countries. But also, to evaluate the impact of specific infrastructure developments on the cross-border interactions of people in a border region, such as building the Tornio–Haparanda rail line to restore passenger train connection between Finland and Sweden (Yle, Citation2021).
To conclude, regardless of the challenges entailed by using a big data approach, the key to successfully employing the potential of social media data in providing better understanding of cross-border mobilities and border regions depends on two aspects. First, transparency and reproducibility of research regarding both used data and methodology. Second, how to link with and make it compatible with other existing data sources and statistics. We believe these aspects can be achieved through an open science initiative, and thus we share openly our codes for data collection, processing, and analysis to foster research on cross-border mobilities and border regions, in general (https://doi.org/10.5281/zenodo.6861319).
Disclosure statement
No potential conflict of interest was reported by the authors.
Additional information
Funding
References
- Adey, P. 2010. Mobility. London and New York: Routledge. https://www.routledge.com/Mobility/Adey/p/book/9781138949010
- Allmendinger, P., and G. Haughton. 2009. “Soft Spaces, Fuzzy Boundaries, and Metagovernance: the New Spatial Planning in the Thames Gateway.” Environment and Planning A 41 (3): 617–633.
- Axhausen, K. W. 2007. “Activity Spaces, Biographies, Social Networks and Their Welfare Gains and Externalities: Some Hypotheses and Empirical Results.” Mobilities 2 (1): 15–36. doi:10.1080/17450100601106203
- Berry, B. J. L. 1968. “A Synthesis of Formal and Functional Regions Using a General Field Theory of Spatial behaviour.” In Spatial Analysis, edited by B. J. L Berry and D. F. Marble, 419–428. Englewood Cliffs: Prentice-Hall.
- Blanford, J. I., Z. Huang, A. Savelyev, and A. M. MacEachren. 2015. “Geo-Located Tweets. Enhancing Mobility Maps and Capturing Cross-Border Movement.” PLoS One 10 (6): 1–16. doi:10.1371/journal.pone.0129202.
- Botta, F., H. Susannah Moat, and T. Preis. 2015. “Quantifying Crowd Size with Mobile Phone and Twitter Data.” Royal Society Open Science 2 (5): Article ID 150162. doi:10.1098/rsos.150162
- Brown, L. A, and J. Holmes. 1971. “The Delimitation of Functional Regions, Nodal Regions, and Hierarchies by Functional Distance Approaches.” Ekistics; Reviews on the Problems and Science of Human Settlements 32 (192): 387–391.
- Brunet-Jailly, E. 2005. “Theorizing Borders: An Interdisciplinary Perspective.” Geopolitics 10 (4): 633–649. doi:10.1080/14650040500318449
- Castells, M. 1996. The Rise of the Network Society. The Information Age: Economy, Society and Culture. 1st ed. Malden Mass: Blackwell Publishers.
- Cavallaro, F., and A. Dianin. 2019. “Cross-border Commuting in Central Europe: Features, Trends and Policies.” Transport Policy 78: 86–104.
- Collins, F. L. 2009. “Transnationalism Unbound: Detailing New Subjects, Registers and Spatialities of Cross-Border Lives.” Geography Compass 3 (1): 434–458. doi:10.1111/j.1749-8198.2008.00187.x
- Conradson, D., and A. Latham. 2005. “Transnational Urbanism: Attending to Everyday Practices and Mobilities.” Journal of Ethnic and Migration Studies 31 (2): 227–233. doi:10.1080/1369183042000339891
- Dahinden, J. 2010. “The Dynamics of Migrants' Transnational Formations.” In Diaspora and Transnationalism, edited by Rainer Bauböck and Thomas Faist, Concepts, Theories and Methods, 51–72. Amsterdam University Press. www.jstor.org/stable/j.ctt46mz31.6.
- Decoville, A., F. Durand, C. Sohn, and O. Walther. 2013. “Comparing Cross-border Metropolitan Integration in Europe: Towards a Functional Typology.” Journal of Borderlands Studies 28 (2): 221–237. doi:10.1080/08865655.2013.854654
- Delhey, J., M. Verbalyte, A. Aplowski, and E. Deutschmann. 2019. “Global Mobilities Project View Project Post-socialist Societies: From Marx to Markets View Project Chapter 4 Free to Move: The Evolution of the European Migration Network, 1960–2017”.
- Deutschmann, E. 2016. “The Spatial Structure of Transnational Human Activity.” Social Science Research 59: 120–136.
- Dijst, M., C. D'Ambrosio, V. Van Acker, A. Albanese, C. M. de Chardon, S. Cosaert, I. Gewinner, et al. 2021. SEI -- Socio-Economic Impacts of Covid-19: Collecting the Data. Technical Report March.
- Docquier, F., N. Golenvaux, S. Nijssen, P. Schaus, and F. Stips. 2021. “Cross-Border Mobility Responses to Covid-19 in Europe: New Evidence from Facebook Data.” Globalization and Health 18 (41): 1–17.
- Drevon, G., P. Gerber, O. Klein, and C. Enaux. 2018. “Measuring Functional Integration by Identifying the Trip Chains and the Profiles of Cross-Border Workers: Empirical Evidences from Luxembourg.” Journal of Borderlands Studies 33 (4): 549–568.
- Dubois, Y. 2017. “Cross-Border Life in Europe: Daily Mobility in the Trinational Metropolis of Basel.” Cuadernos Europeos de Deusto 56 (56): 133–160. https://ced.revistas.deusto.es/article/view/1289
- Durand, F. 2015. “Theoretical Framework of the Cross-border Space Production–The Case of the Eurometropolis Lille–Kortrijk-Tournai.” Journal of Borderlands Studies 30 (3): 309–328.
- Enaux, C., and P. Gerber. 2014. “Beliefs About Energy, a Factor in Daily Ecological Mobility?.” Journal of Transport Geography 41: 154–162.
- European Council. 2016. “General Data Protection Regulation (EU) 2016/679.” Official Journal of the European Union 59 (119): 1–88.
- Frändberg, L. 2008. “Paths in Transnational Time-space: Representing Mobility Biographies of Young Swedes.” Geografiska Annaler, Series B: Human Geography 90 (1): 17–28.
- Fries-Tersch, E., H. Bradley, and T. Tugran. 2017. 2016 Annual Report on intra-EU Labour Mobility. Technical Report.
- Gabrielli, L., E. Deutschmann, F. Natale, E. Recchi, and M. Vespe. 2019. “Dissecting Global Air Traffic Data to Discern Different Types and Trends of Transnational Human Mobility.” EPJ Data Science 8 (26): 1–24.
- Gerber, P. 2012. “Advancement in Conceptualizing Cross-Border Daily Mobility: the Benelux Context in the European Union.” European Journal of Transport and Infrastructure Research 12 (2): 178–197.
- Gerber, P., T. Y. Ma, O. Klein, J. Schiebel, and S. Carpentier-Postel. 2017. “Cross-border Residential Mobility, Quality of Life and Modal Shift: A Luxembourg Case Study.” Transportation Research Part A: Policy and Practice 104: 238–254.
- Gerber, P., M. Thériault, C. Enaux, and S. Carpentier-Postel. 2020. “Links Between Attitudes, Mode Choice, and Travel Satisfaction: A Cross-Border Long-Commute Case Study.” Sustainability 12 (21): 9203. https://www.mdpi.com/2071-1050/12/21/9203
- Glick Schiller, N., and N. B. Salazar. 2013. “Regimes of Mobility Across the Globe.” Journal of Ethnic and Migration Studies 39 (2): 183–200. doi:10.1080/1369183X.2013.723253
- Goddard, J. B. 1970. “Functional Regions Within the City Centre: A Study by Factor Analysis of Taxi Flows in Central London.” Transactions of the Institute of British Geographers 49 (49): 161–182.
- Godefroy, S., J. Delloy, S. Klein, and C. Bredel. 2021. Exploitation harmonisée des enquêtes de déplacements sur le périmètre MMUST. Technical Report.
- González, M. C., C. A. Hidalgo, and A. Barabási. 2008. “Understanding Individual Human Mobility Patterns.” Nature 453 (7196): 779–782. doi:10.1038/nature06958
- Google. 2020. COVID-19 Community Mobility Reports. Technical Report. https://www.google.com/covid19/mobility/.
- Grunfelder, J. 2020. Mobility Changes due to COVID-19 — Nordregio. Technical Report. https://nordregio.org/maps/mobility-changes-due-to-covid-19/.
- Gumy, A., G. Drevon, and V. Kaufmann. 2021. “Inequalities in Access to Cross-border Resources? An Analysis Based on Spatio-temporal Behaviours in the Cross-border Area of Greater Geneva:.” European Urban and Regional Studies 29 (1): 85–106. doi:10.1177/09697764211026716
- Hale, T., N. Angrist, R. Goldszmidt, B. Kira, A. Petherick, T. Phillips, and S. Webster, et al. 2021. “A Global Panel Database of Pandemic Policies (Oxford COVID-19 Government Response Tracker).” Nature Human Behaviour 5 (4): 529–538. https://www.nature.com/articles/s41562-021-01079-8
- Hall, C. M. 2005. “Reconsidering the Geography of Tourism and Contemporary Mobility.” Geographical Research 43 (2): 125–139. doi:10.1111/j.1745-5871.2005.00308.x
- Hannonen, O. 2020. “In Search of a Digital Nomad: Defining the Phenomenon.” Information Technology & Tourism 22 (3): 335–353. doi:10.1007/s40558-020-00177-z
- Hasnat, Md M., and S. Hasan. 2018. “Identifying Tourists and Analyzing Spatial Patterns of Their Destinations from Location-based Social Media Data.” Transportation Research Part C: Emerging Technologies 96: 38–54.
- Hawelka, B., I. Sitko, E. Beinat, S. Sobolevsky, P. Kazakopoulos, and C. Ratti. 2014. “Geo-located Twitter as Proxy for Global Mobility Patterns.” Cartography and Geographic Information Science 41 (3): 260–271.
- Heikinheimo, V., O. Järv, H. Tenkanen, T. Hiippala, and T. Toivonen. 2022. “Detecting Country of Residence from Social Media Data: A Comparison of Methods.” International Journal of Geographical Information Science, 1–22.
- Hesse, M., and M. Rafferty. 2020. “Relational Cities Disrupted: Reflections on the Particular Geographies of COVID-19 for Small But Global Urbanisation in Dublin, Ireland, and Luxembourg City, Luxembourg.” Tijdschrift Voor Economische En Sociale Geografie 111 (3): 451–464. doi:10.1111/tesg.12432
- Hu, Y., and R. Qian Wang. 2020. “Understanding the Removal of Precise Geotagging in Tweets.” Nature Human Behaviour 4: 1219–1221. doi:10.1038/s41562-020-00949-x.
- Huang, A., D. Ebert, and P. Rider. 2017. “You Are What You Tweet: A New Hybrid Model for Sentiment Analysis.” In Lecture Notes in Computer Science (Including Subseries Lecture Notes in Artificial Intelligence and Lecture Notes in Bioinformatics) 10358 LNAI: 403–416.
- Huang, Q., and D. W. S. Wong. 2016. “Activity Patterns, Socioeconomic Status and Urban Spatial Structure: What Can Social Media Data Tell Us?.” International Journal of Geographical Information Science 30 (9): 1873–1898. doi:10.1080/13658816.2016.1145225
- Hultquist, C. 2020. “Representation in Geosocial Data: Grappling with Uncertainty in Digital Traces of Human Activity.” International Journal of Humanities and Arts Computing 14 (1–2): 218–234.
- Jackson, P., P. Crang, and C. Dwyer. 2004. Transnational Spaces. London: Routledge.
- Järv, O., R. Ahas, E. Saluveer, B. Derudder, and F. Witlox. 2012. “Mobile Phones in a Traffic Flow: A Geographical Perspective to Evening Rush Hour Traffic Analysis Using Call Detail Records.” PloS One 7 (11): 1–11.
- Järv, O., R. Ahas, and F. Witlox. 2014. “Understanding Monthly Variability in Human Activity Spaces: A Twelve-month Study Using Mobile Phone Call Detail Records.” Transportation Research Part C: Emerging Technologies 38: 122–135. doi:10.1016/j.trc.2013.11.003
- Järv, O., A. Tominga, K. Müürisepp, and S. Silm. 2021. “The Impact of COVID-19 on Daily Lives of Transnational People Based on Smartphone Data: Estonians in Finland.” Journal of Location Based Services 15 (3): 169–197. doi:10.1080/17489725.2021.1887526
- Jurdak, R., K. Zhao, J. Liu, M. AbouJaoude, M. Cameron, and D. Newth. 2015. “Understanding Human Mobility From Twitter.” PLoS One 10 (7): 1–16. doi:10.1371/journal.pone.0131469.
- Kaufmann, V. 2014. “Mobility as a Tool for Sociology.” Sociologica 8 (1): 1–17.
- Kellerman, A. 2011. “Mobility or Mobilities: Terrestrial, Virtual and Aerial Categories or Entities?.” Journal of Transport Geography 19 (4): 729–737.
- Kemp, S. 2019. Digital 2019: Global digital report. Technical Report. https://datareportal.com/.
- Kitchin, R. 2014a. “Big Data, New Epistemologies and Paradigm Shifts.” Big Data & Society 1 (1): 1–14. doi:10.1177/2053951714528481
- Kitchin, R. 2014b. The Data Revolution: Big Data, Open Data, Data Infrastructures & Their Consequences. Los Angeles, CA: SAGE Publications.
- Klapka, P., and M. Halás. 2016. “Conceptualising Patterns of Spatial Flows: Five Decades of Advances in the Definition and Use of Functional Regions.” Moravian Geographical Reports 24 (2): 2–11.
- Klatt, M. 2020. “What Has Happened to Our Cross-border Regions? Corona, Unfamiliarity and Transnational Borderlander Activism in the Danish-German Border Region.” Borders in Perspective. UniGR-CBC Thematic Issue. Bordering in Pandemic Times: Insights Into the COVID-19 Lockdown 4: 43–47.
- Lara-Valencia, F., J. Agulló, H. García-Pérez, X. Oliveras, and J. M. Trillo. 2020. COVID-19 and Cross-Border Mobility. Technical Report. Tempe, Arizona: Transborder Policy Lab, Arizona State University.
- Löfgren, O. 2008. “Regionauts: The Transformation of Cross-border Regions in Scandinavia.” European Urban and Regional Studies 15 (3): 195–209. doi:10.1177/0969776408090418
- Lundborg, P. 1991. “Determinants of Migration in the Nordic Labor Market.” The Scandinavian Journal of Economics 93 (3): 363–375. https://www.jstor.org/stable/3440180
- Mazzoli, M., B. Diechtiareff, A. Tugores, W. Wives, N. Adler, P. Colet, and J. J. Ramasco. 2020. “Migrant Mobility Flows Characterized with Digital Data.” PloS One 15 (3): Article ID e0230264. https://journals.plos.org/plosone/article?id=10.1371/journal.pone.0230264
- Medeiros, E., M. Guillermo Ramírez, C. Dellagiacoma, and G. Brustia. 2021. “Will Reducing Border Barriers via the EU's B-solutions Lead Towards Greater European Territorial Integration?.” Regional Studies 56 (3): 504–517. doi:10.1080/00343404.2021.1912724.
- Mellon, J., and C. Prosser. 2017. “Twitter and Facebook Are Not Representative of the General Population: Political Attitudes and Demographics of British Social Media Users:.” Research & Politics4 (3): 1–9. doi:10.1177/2053168017720008.
- Moisio, S. 2011. “Geographies of Europeanization: The EU's Spatial Planning as a Politics of Scale.” In Europe in the World: EU Geopolitics and the Making of European Space, 19–39.
- Möller, C., E. Alfredsson-Olsson, B. Ericsson, and K. Overvåg. 2018. “The Border as an Engine for Mobility and Spatial Integration: A Study of Commuting in a Swedish–Norwegian Context.” Norsk Geografisk Tidsskrift -- Norwegian Journal of Geography 72 (4): 217–233.
- Nordic Council of Ministers. 2021. Nordic Cross-border Statistics: The results of the Nordic Mobility project 2016–2020. Technical Report. Nordic Council of Ministers. https://pub.norden.org/nord2021-006/.
- Novak, J., R. Ahas, A. Aasa, and S. Silm. 2013. “Application of Mobile Phone Location Data in Mapping of Commuting Patterns and Functional Regionalization: A Pilot Study of Estonia.” Journal of Maps 9 (1): 10–15.
- OECD. 2013. Regions and Innovation: Collaborating Across Borders -- OECD Reviews of Regional Innovation. https://www.oecd.org/innovation/regions-and-innovation-collaborating-across-borders.htm.
- Paasi, A. 1991. “Deconstructing Regions: Notes on the Scales of Spatial Life.” Environment & Planning A 23 (2): 239–256. doi:10.1068/a230239
- Perkmann, M. 2003. “Cross-border Regions in Europe: Significance and Drivers of Regional Cross-border Co-operation.” European Urban and Regional Studies 10 (2): 153–171.
- Philbrick, A. K. 1957. “Areal Functional Organization in Regional Geography.” Papers in Regional Science 3 (1): 87–98. doi:10.1111/j.1435-5597.1957.tb01589.x
- Poom, A., O. Järv, M. Zook, and T. Toivonen. 2020. “COVID-19 Is Spatial: Ensuring that Mobile Big Data Is Used for Social Good.” Big Data and Society 7 (2): 1–7.
- Poorthuis, A. 2018. “How to Draw a Neighborhood? The Potential of Big Data, Regionalization, and Community Detection for Understanding the Heterogeneous Nature of Urban Neighborhoods.” Geographical Analysis 50 (2): 182–203.
- Poorthuis, A., and M. Zook. 2017. “Making Big Data Small: Strategies to Expand Urban and Geographical Research Using Social Media.” Journal of Urban Technology 24 (4): 115–135. doi:10.1080/10630732.2017.1335153
- Puura, A., S. Silm, and A. Masso. 2022. “Identifying Relationships Between Personal Social Networks and Spatial Mobility: A Study Using Smartphone Tracing and Related Surveys.” Social Networks 68: 306–317.
- Øresundsbron. n.d. “Traffic Statistics — The Oresund Bridge.” Accessed 15 November 2021. https://www.oresundsbron.com/en/traffic-stats.
- Santamaria, C., F. Sermi, S. Spyratos, S. Maria Iacus, A. Annunziato, D. Tarchi, and M. Vespe. 2020. “Measuring the Impact of COVID-19 Confinement Measures on Human Mobility Using Mobile Positioning Data. A European Regional Analysis.” Safety Science 132 (April): Article ID 104925. doi:10.1016/j.ssci.2020.104925
- Schiebel, J., H. Omrani, and P. Gerber. 2015. “Border Effects on the Travel Mode Choice of Resident and Crossborder Workers in Luxembourg.” European Journal of Transport and Infrastructure Research15 (4): 570–596. https://journals.open.tudelft.nl/ejtir/article/view/3098
- Schmitz, F., G. Drevon, and P. Gerber. 2012. “La mobilité des Frontaliers du Luxembourg: Dynamiques et Perspectives.” Les Cahiers Du CEPS/INSTEAD https://statistiques.public.lu/catalogue-publications/cahiers-CEPS/2012/hors-serie-FR.pdf.
- Schönfelder, S., and K. W. Axhausen 2010. Urban Rhythms and Travel Behaviour: Spatial and Temporal Phenomena of Daily Travel. Transport and Society. Ashgate. https://books.google.fi/books?id=mFLQikBXgm8C.
- Schönweitz, M. 2014. “Cross-border Cooperation of Urban Regions in the Baltic Sea Area.” PhD diss., Humboldt Universität zu Berlin. https://edoc.hu-berlin.de/bitstream/handle/18452/17993/schoenweitz.pdf.
- Sheller, M., and J. Urry. 2006. “The New Mobilities Paradigm.” Environment and Planning A 38 (2): 207–226. http://www.envplan.com/abstract.cgi?id=a37268
- Silm, S., O. Järv, and A. Masso. 2020. “Tracing Human Mobilities Through Mobile Phones.” In Handbook of Research Methods and Applications for Mobilities, edited by Monika Büscher, Malene Freudendal-Pedersen, Sven Kesselring, and Nikolaj Grauslund Kristensen, 182–192. Edward Elgar Publishing.
- Silm, S., J. S. Jauhiainen, J. Raun, and M. Tiru. 2020. “Temporary Population Mobilities Between Estonia and Finland Based on Mobile Phone Data and the Emergence of a Cross-Border Region.” European Planning Studies 29 (4): 699–719. doi:10.1080/09654313.2020.1774514.
- Slätmo, E., L. Ormstrup Vestergård, J. Lidmo, and E. Turunen. 2019. Urban–Rural Flows from Seasonal Tourism and Second Homes: Planning Challenges and Strategies in the Nordics. Technical Report. Stockholm: Nordregio.
- Sloan, L., J. Morgan, P. Burnap, and M. Williams. 2015. “Who Tweets? Deriving the Demographic Characteristics of Age, Occupation and Social Class from Twitter User Meta-Data.” PLoS One 10 (3): 1–20. doi:10.1371/journal.pone.0115545.
- Sohn, C., and J. Licheron. 2018. “The Multiple Effects of Borders on Metropolitan Functions in Europe.” Regional Studies 52 (11): 1512–1524. doi:10.1080/00343404.2017.1410537
- Spierings, B., and M. Van Der Velde. 2008. “Shopping, Borders and Unfamiliarity: Consumer Mobility in Europe.” Tijdschrift voor economische en sociale geografie 99 (4): 497–505. doi:10.1111/j.1467-9663.2008.00484.x
- Sæther, B., B. T. Andersen, and E. Hagen. 2019. “Cross-Border Functional Regions and Innovation: Cases from Inner Scandinavian Regions.” In Urban Book Series, 91–109. Springer.
- Tasse, D., and J. I. Hong. 2014. Using Social Media Data to Understand Cities. Technical Report. Carnegie Mellon University.
- Terlouw, K. 2012. “Border Surfers and Euroregions: Unplanned Cross-Border Behaviour and Planned Territorial Structures of Cross-Border Governance.” Planning Practice & Research 27 (3): 351–366. doi:10.1080/02697459.2012.670939
- Thomas, I., J. Jones, G. Caruso, and P. Gerber. 2017. “City Delineation in European Applications of LUTI Models: Review and Tests.” Transport Reviews 38 (1): 6–32. doi:10.1080/01441647.2017.1295112
- Toivonen, T., V. Heikinheimo, C. Fink, A. Hausmann, T. Hiippala, O. Järv, H. Tenkanen, and E. Di Minin. 2019. “Social Media Data for Conservation Science: A Methodological Overview.” Biological Conservation 233: 298–315.
- Väisänen, T., S. Sirkiä, T. Hiippala, O. Järv, and T. Toivonen. 2021. “DigitalGeographyLab/tweetsearcher: A Python Tool to Download Tweets Using Academic Research Credentials.” https://zenodo.org/record/4723337.
- Vandermotten, C. 2010. “les Aires Urbaines Transfrontalières En Europe.” Mosella : Revue du Centre d'études géographiques de Metz 32 (1–4): 37.
- van Houtum, H. 2000. “III European Perspectives on Borderlands.” Journal of Borderlands Studies 15 (1): 56–83.
- Wiesböck, L., R. Verwiebe, C. Reinprecht, and R. Haindorfer. 2016. “The Economic Crisis As a Driver of Cross-Border Labour Mobility? A Multi-Method Perspective on the Case of the Central European Region.” Journal of Ethnic and Migration Studies 42 (10): 1711–1727. doi:10.1080/1369183X.2016.1162354
- Willberg, E., O. Järv, T. Väisänen, and T. Toivonen. 2021. “Escaping from Cities During the COVID-19 Crisis: Using Mobile Phone Data to Trace Mobility in Finland.” ISPRS International Journal of Geo-Information 10 (2): 103. https://www.mdpi.com/2220-9964/10/2/103
- Willi, Y., G. Nischik, D. Braunschweiger, and M. Pütz. 2020. “Responding to the COVID-19 Crisis: Transformative Governance in Switzerland.” Tijdschrift voor economische en sociale geografie 111 (3): 302–317. doi:10.1111/tesg.12439
- Yle. 2020b, May. “Why Northern Residents Pushed Back Against Border Closure — News — Yle Uutiset.” Accessed 29 November 2021 https://yle.fi/news/3-11349310.
- Yle. 2021. “Work Set to Begin on Cross-Border Finland to Sweden Rail Line — News — Yle Uutiset.” Accessed 29 November 2021. https://yle.fi/news/3-12208368.
- Yle, June. 2020a. “Norway Opens Borders to Visitors from Finland.” https://yle.fi/uutiset/osasto/news/norway_opens_borders_to_visitors_from_finland/11401226.
- Zook, M., S. Barocas, D. Boyd, K. Crawford, E. Keller, S. P. Gangadharan, and A. Goodman, et al. 2017. “Ten Simple Rules for Responsible Big Data Research.” PLoS Computational Biology 13 (3): Article ID e1005399. https://journals.plos.org/ploscompbiol/article?id=10.1371/journal.pcbi.1005399