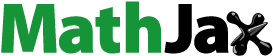
Abstract
Remote sensing is key for large-scale forest mapping, yet the limited integration of LiDAR sensors restricts the spatial coverage of forest attribute estimation. Our study aimed to accurately map Foliage Height Diversity (FHD) in five key North American regions, vital for ongoing research in ecosystem dynamics in western North America. We used a combination of GEDI data and LVIS data, incorporating measures of forest complexity and relative forest heights (RH25, RH50, RH75, and RH98), to predict FHD with a random forest regression in the Kluane region, southwest Yukon—a northernmost site where GEDI data are not available. This method was designed to overcome spatial coverage limitations of different sensors, enabling the production of consistent, precise, multi-temporal FHD maps across all sites. We found strong agreement between predicted and observed FHD values estimated from Airborne Laser Scanning in the Yukon (R2 = 0.72; RMSE = 0.46). Additionally, we upscaled GEDI FHD predictions in all sites by integrating Landsat imagery, ALOS PALSAR, and topographical data, resulting in high accuracy (R2 = 0.85; RMSE = 0.26). Our findings demonstrate that by harmonizing full-wave form LiDAR sensors, we can significantly expand the coverage of LiDAR data, allowing for consistent broad-scale analyses of forest attributes.
RÉSUMÉ
La télédétection est essentielle pour la cartographie des forêts à grande échelle, cependant l’intégration limitée des capteurs LiDAR restreint la couverture spatiale de l’estimation des attributs forestiers. Notre étude visait à cartographier avec précision la Foliage Height Diversity (FHD) dans cinq régions clés d’Amérique du Nord, cruciale pour la recherche en cours sur la dynamique des écosystèmes dans l’ouest de l’Amérique du Nord. Nous avons utilisé une combinaison de données GEDI et de données LVIS, en intégrant des mesures de la complexité forestière et des hauteurs forestières relatives (RH25, RH50, RH75 et RH98), pour prédire la FHD à l’aide d’une régression de forêts aléatoires dans la région de Kluane, au sud-ouest du Yukon—un site le plus septentrional où les données GEDI ne sont pas disponibles. Cette méthode a été conçue pour surmonter les limitations de couverture spatiale des différents capteurs, permettant la production de cartes FHD précises, cohérentes et multi-temporelles sur tous les sites. Nous avons trouvé une forte corrélation entre les valeurs de FHD prédites et observées estimées à partir du balayage laser aéroporté au Yukon (R2 = 0.72; RMSE = 0.46). De plus, nous avons étendu les prédictions de FHD de GEDI sur tous les sites en intégrant des images Landsat, ALOS PALSAR et des données topographiques, ce qui a abouti à une haute précision (R2 = 0.85; RMSE = 0.26). Nos résultats démontrent qu’en harmonisant les capteurs LiDAR à forme d’onde complète, nous pouvons significativement étendre la couverture des données LiDAR, permettant des analyses à grande échelle et cohérentes des attributs forestiers.
Introduction
Forests play a pivotal role in maintaining global ecological functioning and services (Edwards-Jones et al. Citation2011; Foley et al. Citation2000), serving as rich reservoirs of biodiversity (Brockerhoff et al. Citation2017; Thompson et al. Citation2011) and acting as vital sinks in the global carbon cycle (Pan et al. Citation2011). Forest dynamics are important for climate regulation (Bonan Citation2008), water purification, and habitat provisioning (Gauthier et al. Citation2015). Accurately quantifying forest attributes has become a high priority, not only to understand their current state but also to predict and mitigate potential threats to their sustainability (Goetz et al. Citation2009). This makes forests central to remote sensing investigations and methodological refinements, ranging from high-resolution forest mapping and species identification (Akujärvi et al. Citation2016; Hermosilla et al. Citation2022) to biomass estimation and change detection over time (Ferraz et al. Citation2016; Sun et al. Citation2011).
LiDAR (Light Detection and Ranging) has emerged as an important tool for surveying forests due to its ability to capture the structural characteristics of trees, including canopy height, biomass, and structural complexity (Coops et al. Citation2021; Zolkos et al. Citation2013). Recently, LiDAR-derived metrics have been leveraged to provide a detailed understanding of forest structure and its relationship with biodiversity (Bergen et al. Citation2009; Carrasco et al. Citation2019; Goetz et al. Citation2010; Guo et al. Citation2017). One fundamental metric derived from LiDAR, particularly the GEDI sensor, is Foliage Height Diversity (FHD) (Crockett et al. Citation2023; Killion et al. Citation2023). This feature represents forest vertical stratification and canopy complexity, allowing it to inform on the diverse habitats within the forest canopy that host a rich variety of flora and fauna (Hardiman et al. Citation2011; Kacic et al. Citation2021; Smith et al. Citation2022; Wood et al. Citation2012). The use of FHD in forest ecology research underscores the unique ability of LiDAR to capture forest structure and complexity, aiding in the prediction of habitat changes over time.
The measurement of FHD from remotely sensed data is subject to sensor-specific differences. GEDI measures FHD through the vertical distribution of Leaf Area Index (LAI) profiles (Tang and Armston Citation2019). It employs Shannon’s Diversity Index to evaluate the complexity of LAI, resulting in a metric where a higher FHD value reflects a more complex vertical forest structure (MacArthur and Horn Citation1969). In contrast, when using discrete return LiDAR, a common method involves organizing the collected point cloud data into voxels (three-dimensional pixels) to estimate the forest’s vertical structure (Carrasco et al. Citation2019). This voxelization allows for an estimation of vertical complexity and, consequently, an assessment of FHD. While one provides a broad, and standardized evaluation of vertical forest structure at large scales, the other provides a more detailed, localized analysis by dividing the three-dimensional forest structure into small cubes or voxels, providing flexibility in the scale of analysis.
While LiDAR is highly effective in measuring forest attributes, it faces significant constraints in terms of spatial and temporal coverage. This limitation poses challenges for applying it to broader regions or for ongoing forest monitoring purposes. To address this, data fusion techniques that merge detailed individual LiDAR plot data with satellite imagery expand the spatial and temporal reach of forest structure assessments (Ahmed et al. Citation2014; Pascual et al. Citation2010; Pflugmacher et al. Citation2014). However, understanding continental-scale forest dynamics still presents challenges due to these inherent limitations in spatial coverage. Recent advancements, such as the introduction of spaceborne LiDAR systems like GEDI (Global Ecosystem Dynamics Investigation) (Dubayah et al. Citation2020) and ICESat (Ice, Cloud, and land Elevation Satellite) (Markus et al. Citation2017), have significantly widened the geographical extent. These technologies enable more continuous and extensive monitoring of forest dynamics, ice sheets, and other terrestrial features (Roy et al. Citation2021; Sun et al. Citation2022; Verhelst et al. Citation2021). Nevertheless, limitations persist; for example, GEDI does not cover areas beyond 51.6°N and 51.6°S latitudes, thereby excluding high-latitude boreal forests and certain polar zones. Such omissions limit our ability to develop a regional or global understanding of forest dynamics and structure. These gaps, however, can be mitigated by integrating GEDI data with other full waveform LiDAR datasets, like LVIS (Land, Vegetation, and Ice Sensor). The LVIS sensor, having been flown in a variety of campaigns including the Artic-Boreal Vulnerability Experiment (ABoVE), may be used to extend the spatial extent beyond GEDI’s latitudinal limitations, thereby ensuring a more extensive coverage in vital regions like the northern boreal forest of North America. LVIS is preferred over sensors like ICESat-2 because it can measure multiple relative heights within the canopy, capturing the diversity in forest heights which is essential for assessing foliage height diversity. However, it is essential that efforts to combine these remote sensing products be approached with proper validation before they receive broad application.
Our first objective is to combine LVIS and GEDI data to develop regression models that are trained where both datasets overlap. These models can be applied locally in northern regions where only LVIS data is available. Our second objective is to upscale the FHD measurements across large regions by incorporating machine learning with features from Landsat imagery, ALOS PALSAR (Advanced Land Observing Satellite Phased Array type L-band Synthetic Aperture Radar), and topographical information from the Copernicus Digital Elevation Model. Our efforts are concentrated on five northern regions of North America, four of which have GEDI data, and one exclusively covered by LVIS. All these sites are critical for recent or ongoing studies on Canada Lynx (Lynx canadensis) and Snowshoe Hares (Lepus americanus) (e.g., Jensen et al. Citation2021; Studd et al. Citation2021). Our study showcases the effectiveness of merging different remote sensing technologies and machine learning to create dependable, multi-temporal forest structure maps.
Methods
Study regions
Each study region was a bounding box created based on Lynx movement and mainly comprised of montane forest, with a range of wetlands, lakes and limited urban development (Appendix A). In Washington and British Columbia, the forest composition was characterized by a diversity of tree species including abundant evergreen fir (Abies spp.), with some maples (Acer spp.) and birches (Betula spp.). In Montana, coniferous forests include Western Larch (Larix occidentalis), known for its seasonal needle shedding, Ponderosa Pine (Pinus ponderosa) and Douglas Fir (Pseudotsuga menziesii), with some Engelmann Spruce (Picea engelmannii) and Lodgepole Pine (Pinus contorta). The Alberta site is comprised of coniferous forests with White Spruce (Picea glauca), Lodgepole Pine (Pinus contorta), and Subalpine Fir (Abies lasiocarpa), along with deciduous trees like Aspen (Populus spp.) and Balsam Poplar (Populus balsamifera). The Kluane region in the Yukon is dominated by White Spruce (Picea glauca) accompanied by Trembling Aspen (Populus tremuloides).
Data analysis
We integrated GEDI data with LVIS (Land, Vegetation, and Ice Sensor) data to predict GEDI FHD in the Kluane region (hereafter Yukon) where GEDI data were unavailable. We included Complexity, RH25, RH50, RH75, and RH98 from the LVIS L3 2019 data obtained from https://daac.ornl.gov/ABOVE/guides/ABoVE_LVIS_VegetationStructure.html. Complexity represents the number of surfaces detected in the waveform, which provides insights into the forest’s structural complexity including canopy relative heights, offering information on the vertical distribution of vegetation. The RH25, RH50, RH75, and RH98 are specific percentiles of relative height derived from LVIS data.
We clipped the LVIS data to align spatially and temporally with GEDI FHD data from May to August of 2019 (Appendix A). We retained only the GEDI footprints with Level 2 A and Level 2B quality flag = 1. This filtering process ensured that our dataset consisted of the highest quality GEDI footprints, which were then used as reliable training and test samples for developing accurate models (Smith et al. Citation2022). In addition, we collected forest/non-forest data for the year 2019 from the Global 4-class ALOS PALSAR FNF4 dataset (Shimada et al. Citation2014). Using this information, we applied a mask to the LVIS image, differentiating between dense and non-dense forest pixels. Combining these classifications, we created a composite mask that excluded non-forested areas to predict FHD in only forested areas.
To obtain a representative sample of reference sites, we randomly generated sampling points across the region of interest to sample both GEDI and LVIS, resulting in approximately 11,000 samples. The resulting sample was split into training (70%) and validation (30%) subsets, with merged LVIS predictors used to train a random forest regression with 150 trees, and a bag fraction of 0.8 (Breiman Citation2001). This decision was based on observing that increasing the number of trees beyond 150 and altering the bag fraction values did not significantly enhance the model’s performance We then applied the regression and clipped the result to the regions of interest. We assessed model fit using the corresponding R2 and RMSE values for the validation data (Bolton et al. Citation2018; Potapov et al. Citation2021).
For model validation, we used our northernmost site in the Yukon, where GEDI FHD data were unavailable. Using the same predictor variables (Complexity, RH25, RH50, RH75, and RH98), we applied our previous model to predict FHD, which was then substituted into the LVIS footprint allowing us to estimate FHD for the LVIS data in the absence of GEDI measurements (). To assess model accuracy, we compared predicted FHD values to observed FHD measurements derived from a commercially available Airborne Laser Scanning (ALS) sensor (DJI L1) during August 2022. The sensor was flown at a height of 80 m, at a speed of 10 m/s and an overlap of 50%. The LiDAR data were voxelized with a voxel size of 1 meter to divide the LiDAR data into smaller volumetric units (voxels), from which we calculated the Leaf Area Index (LAI), providing information on the amount of leaf area within each voxel. To calculate FHD, we computed Shannon’s diversity index using the voxalized LAI to provide a measure of the variability in foliage heights within the study area, to mimic GEDI’s FHD calculation (Dubayah et al. Citation2020).
Figure 1. Illustration of the application of predictor variables (Complexity, RH25, RH50, RH75, and RH98) from the random forest regression to predict FHD values. These values were subsequently integrated into the LVIS footprint, facilitating an estimation of FHD in the absence of GEDI measurements.

The LiDAR FHD was computed using the formula:
where
is the proportion of the Leaf Area Index (LAI) within a specific vertical layer of the canopy. LAI, a measure of leaf canopy, is defined for a vertical interval from z
to z
+1. The calculation of
is given by:
Where Δz is the height difference between the consecutive vertical layers, which in this context is 1 meter due to the voxelization of the LiDAR data.
To evaluate the model’s performance, we selected areas where both LVIS-derived and our ALS-derived FHD measurements were available in the Yukon. Within these areas, we sampled 1,000 random points. We then calculated the mean FHD values for the observed ALS-derived dataset within 30-meter buffers. This buffer size is intended to account for spatial discrepancies between the two datasets. To visualize the relationship between observed and predicted FHD values, we created a scatter plot and used R2 and RMSE metrics to quantify model performance. By employing these validation procedures, we assessed the accuracy of our model’s predictions for our 5th site (Yukon region).
In the spatial extrapolation phase, we used Landsat 8 and Landsat 5, Level 2, Collection 2, Tier 1 surface reflectance collections to continuously predict FHD across our study sites. For each Landsat collection, we filtered images taken during summer months relative to the year of desired data extrapolation and with < 50% cloud cover, and we created a median summer composite for each year of interest. Next, we calculated a series of vegetation indices including the Normalized Difference Vegetation Index (NDVI), Enhanced Vegetation Index (EVI), Soil Adjusted Vegetation Index (SAVI), Normalized Difference Water Index (NDWI), Modified Normalized Difference Water Index (MNDWI), Normalized Burn Ratio (NBR), and the Tasseled Cap transformation indices including brightness, greenness, wetness, and angle indices. We also included Bands 1 through 7 from the Landsat 8 archive. Additionally, we incorporated PALSAR yearly mosaics, using the HH and HV polarizations. The HH polarization captured radar backscatter under horizontally polarized transmit and receive conditions, while the HV variable measured the HV polarization backscattering coefficient under horizontal transmit and vertical receive conditions. We also included the HH:HV ratio as an additional predictor, as it may include unique and complementary information to the individual HH and HV coefficients. To account for topographical variation, we incorporated a Digital Elevation Model (DEM) from the Copernicus collection, from which we derived terrain attributes such as slope, aspect, and roughness (). We also constructed an image with longitude and latitude to account for variation in forest structure according to location.
Table 1. Independent remote sensing variables used for the machine learning model to predict forest structure in southwestern Yukon.
All predictor variables were colelcted with approximately 23,000 sampling points. Data were then filtered to a density of a single point per 500 m to avoid spatial autocorrelation (Queinnec et al. Citation2021). Next, we carried out a correlation analysis on the variables to identify any redundancy between our predictors. Although random forest regressions are generally robust against multicollinearity, we aimed to develop a parsimonious model that maintained model generality while also having high predictive power and variable independence. Variable pairs with r > 0.75 were identified as redundant, and the final model included variables with strong associations with forest structure based on established research findings. This selection process ensured the retention of the most relevant and informative predictors.
The retained variables were used to construct separate FHD models for each year of interest. Preliminary analysis indicated stable model accuracy regardless of an increase in sampling points. Therefore, we selected a random subset of 15,000 data points for model training. For both the backcasting and extrapolation phases, we allocated 70% of our dataset for model training purposes and reserved the remaining 30% for validating the model. The model included 150 trees and a bag fraction of 0.9 as they demonstrated the highest accuracy. For each year of reconstruction, we assumed that the relationship between predictors and FHD was constant (Matasci et al. Citation2018). Due to their geographical proximity, we consolidated Washington and British Columbia study areas into a unified research site. For this combined site, we predicted FHD for the years 2007, 2010, and 2017. In Montana, we reconstructed FHD for the years 2007, 2008, and 2015. At the Alberta site, which is beyond the coverage of the GEDI footprint, we calculated FHD for 2008. We modified our sampling approach by selecting points approximately 7 km to the south of the site, working under the assumption that forest structure remained consistent within that range, given our chosen predictors. The predicted FHD images for each year were masked using the corresponding PALSAR data to include only forested areas. Model performance was evaluated by comparing observed and predicted GEDI data on the validation dataset for the year 2019 with R2 and RMSE and then extrapolated using the final model. Additionally, variable importance was assessed using the random forest model to identify the features that had greatest influence on the model’s predictions.
Results
Our harmonized model predicted GEDI FHD accurately with the selected parameters and predictor variables. Based on random forest regression, the most-to-least important variable ranking was: RH98, RH75, RH50, Complexity, and RH25. RH98 was approximately 1.5 times more important than the least important variable, RH25 (Appendix A). During the model validation phase, we observed strong agreement between the LVIS predicted FHD and the FHD estimates obtained from our commercial drone sensor (R2 = 0.72; RMSE = 0.46) ().
Figure 2. Scatter plot showcasing the relationship between point cloud estimated FHD and the estimated GEDI FHD values for the Yukon region of the validation phase.

During the feature selection, we observed considerable redundancy among chosen predictors. Only the orthogonal or independent variables remained uncorrelated, as shown in . The Tasseled Cap (TC) indices were prominently correlated with all other indices and bands. Specifically, Greenness exhibited strong correlations with NDVI, SAVI, and EVI; Wetness was notably linked with MNDWI and NBR; and Brightness was correlated with Bands 2 through 7. The ALOS PALSAR polarizations and terrain metrics did not show any significant correlations with other variables.
The extrapolation conducted with remaining predictors also revealed strong concordance between predicted and observed values (R2 = 0.85; RMSE = 0.26). Nonetheless, our model has a vertical clump of points at the lower end of the FHD values, suggesting that GEDI might have a lower limit of detection sensitivity for FHD values. Measurements falling below this threshold appear indistinguishable, resulting in their aggregation at a minimum detectable value, leading to a clumping of data points at the lower end of the observed FHD scale (). The strong relationship between observed and predicted values enabled a continuous spatial prediction of FHD across our study sites for multiple years as shown in . Brightness was the most important predictor, followed by the HV polarization and TC Wetness. Longitude demonstrated more importance than latitude, terrain elevation, and TC greenness had comparable importance. The remaining terrain metrics including roughness, slope, and aspect had the least importance (Appendix A).
Discussion
There have been a few initiatives to merge different LiDAR products to increase spatial coverage. Our study is the first where LVIS and GEDI datasets have been combined to predict GEDI FHD in areas lacking GEDI data. Our harmonization model demonstrates the feasibility of this approach with a high degree of accuracy, corroborated by the relationship between LVIS predicted FHD and FHD estimated by our LiDAR data. This aligns with the findings of Vogeler et al. (Citation2023), who observed a similar pattern using satellite imagery and GEDI for forest structure modeling across multiple study sites in the Western United States (Colorado, Wyoming, Idaho, Oregon, Washington, and Montana). They reported a strong correspondence between ALS-derived FHD and GEDI FHD (R2 = 0.61, RMSE = 2.14). In contrast, a study by Ilangakoon et al. (Citation2021) estimated FHD at a 10-meter scale in Idaho found that ALS FHD estimates are less agreeable with GEDI FHD (R2 = 0.41, RMSE = 2.45). Our model’s precision surpasses these results, underscoring the effectiveness of our approach in harmonizing LVIS and GEDI data.
The discrepancies in FHD estimates could be attributed to differences in the scale of analysis and the specific methods used for FHD calculation. Vogeler et al. (Citation2023) did not clearly state the spatial resolution or the exact formula they used to estimate FHD, but their ALS data encompassed extensive areas, ranging from 150 to 450 km2. On the other hand, our analysis was conducted on a considerably smaller scale, encompassing around 200 ha. Such a stark contrast in spatial scale can impact the accuracy of FHD estimates, with larger areas tending to have more varied forest structures and compositions (Ren et al. Citation2023; Schneider et al. Citation2023; Yu et al. Citation2022), potentially leading to varying levels of precision in FHD measurements. In contrast, Ilangakoon et al. (Citation2021) estimated ALS FHD at the plot scale 10 × 10 m. The finer scale allows for more detailed, localized measurements, whereas our method, with its 30-meter averaging, might capture a more generalized view of forest structure. This discrepancy in scale could be a key factor influencing differences observed in our respective findings. Moreover, we sought to closely replicate GEDÍs FHD estimates with our chosen mathematical formula and voxelization approach by mirroring GEDI’s method for estimating FHD (see Ilangakoon et al. Citation2021). We use voxelization to enable better comparisons between full-waveform LiDAR systems (such as GEDI) and discrete systems used in drones. This technique improves how our results align with GEDI’s FHD estimates despite not comparing GEDI measurements directly with our LiDAR data. Instead, we compare FHD predictions made using LVIS data in areas where GEDI data are lacking, which reinforces the reliability of our harmonization model by filling gaps in regions lacking GEDI coverage.
Our harmonization model agrees with ecological expectations, with RH98 being the most significant predictor variable. This emphasizes the critical influence of the upper layers of forest canopy on FHD estimates, reinforcing prior research that suggests the vertical complexity of a forest is predominantly determined by the characteristics of its upper canopy (Ma et al. Citation2022; Zimble et al. Citation2003). However, in future applications of our approach, researchers should use a greater diversity of predictor variables, beyond relative heights. Sole reliance on these variables can overestimate FHD, especially in high-altitude regions as we observed in our model output. To reduce this bias, we incorporated measures of canopy complexity into our model, which had little effect on its accuracy. This suggests that our estimates of FHD in the mountainous terrains of the Yukon might have been overestimated. Future models could benefit from including terrain features such as slope and roughness by allowing for a more explicit assessment of forest vertical complexity, thereby enhancing the model’s ability to generalize across different ecological zones and topographical variations.
We focused our FHD validation efforts on ALS data collected from the valley regions within the Kluane area, omitting areas at higher elevations. Consequently, evaluating FHD values in these elevated terrains was not within the scope of our study and the observed overestimation may reflect the ecological characteristics of the sites selected for harmonization. For instance, at higher elevations montane and subalpine forests are comprised of diverse tree species composition, including Engelmann spruce (Picea engelmannii), Douglas-fir (Pseudotsuga menziesii), and subalpine fir (Abies lasiocarpa) in Washington State and southern B.C. The inclusion of these species should increase measures of forest structural complexity (Holbrook et al. Citation2017). Conversely, in the Yukon, we anticipate less complexity at higher elevations due to a decrease in density and size of predominant white spruce. This variation emphasizes the need to consider the ecological context of the areas used for model development and to ensure that selected predictor variables align with the ecological characteristics of the targeted regions. Our model’s success at predicting FHD in the Yukon demonstrates its robustness in the specific context of our study; adaptations might be necessary for its application in regions with different structural features.
Similar care is required when choosing the voxel size in the validation phase, as the FHD estimated by ALS consistently shows higher values than those predicted by the random forest regression. Potential causes for this discrepancy include our choice of small voxels and the comparison to large scale full-wave form LiDAR data. Specifically, the smaller voxel size may exaggerate averaging effects across the 30-meter scale, particularly if there are outlier data points. However, despite these disparities, the constant linear correlation across the range of both datasets ensures a consistent FHD measurement across the Yukon region.
In the extrapolation or upscaling phase of our study, we attained a slightly better predictive performance than that achieved in similar research. For instance, Smith et al. (Citation2022) reported an R2 of 0.66 and an RMSE of 0.42 in their study focusing on upscaling FHD using only Landsat vegetation indices and individual spectral bands. Vogeler et al. (Citation2023) achieved an R2 of 0.74 and an RMSE of 0.39 when extrapolating FHD with Sentinel and Landsat spectral indices. The slight improvement in model accuracy in our study likely reflects our efforts to reduce bias by spacing sampling points to 500 m to mitigate against spatial autocorrelation. Indeed, using training data with lower spatial clumping enhances model performance and generality (Queinnec et al. Citation2021). Our model also benefited from having initially filtered redundant features, thereby avoiding suboptimal decision splits in the regression tree (Hastie et al. Citation2009). Nonetheless, our model has a vertical clump of points at the lower end of the FHD values, suggesting that GEDI might have a lower limit of detection sensitivity for FHD values. Measurements falling below this threshold appear indistinguishable, resulting in their aggregation at a minimum detectable value, leading to a clumping of data points at the lower end of the observed FHD scale.
The strong agreement between predicted and observed values in our model highlight the benefit of integrating SAR and Landsat indices to infer GEDI FHD data. The role of HV (horizontal-vertical) backscatter in our model’s predictions aligns with previous studies using Alos Palsar to reveal HV polarization’s strong sensitivity to forest structural parameters (Ji et al. Citation2020; Santoro et al. Citation2009; Viet Nguyen et al. Citation2016; Watanabe et al. Citation2006). Similarly, the relevance of all Tasseled Cap Indices in our study echoes findings from prior investigations into forest structure (Gómez et al. Citation2011; Healey et al. Citation2005; Lastovicka et al. Citation2020; Zald et al. Citation2016). Notably, the importance of the Tasseled Cap Brightness index corroborated observations made by Smith et al. (Citation2022). This index, along with the Tasseled Cap wetness, were important predictors due to their ability to capture unique, non-redundant aspects of surface reflectance directly related to vegetation characteristics (Crist and Cicone Citation1984). Additionally, considering the diverse ecological characteristics of our five study areas, we anticipated that incorporating geographic coordinates would improve the model’s accuracy, as the broad spatial extent of this study means that variations in geographic coordinates correspond with differences in the local environment, history of climatic conditions, and, consequently, forest structure (Ehbrecht et al. Citation2017).
While the existing literature is rich with different data fusion methods, including those involving LiDAR sensor fusion as detailed in studies by Duncanson et al. (Citation2020), Fekry et al. (Citation2022), Silva et al. (Citation2021) and Wang et al. (Citation2023), we are not aware of studies that harmonize full-waveform LiDAR systems to address data gaps in areas lacking measurements of one sensor. Although our model revealed promising results, we highlight those differences in the temporal acquisition of datasets, sensor specifications, predictor variables of choice or forest conditions can weaken model fit. The Random Forest approach was successful in integrating LVIS and GEDI data to predict FHD in areas above GEDI’s footprint, and we encourage further research to test whether other GEDI products can be spatially extended with LVIS variables across diverse forest ecosystems. In addition, further investigation should address whether GEDI, LVIS, or alternative LiDAR sensors can be used to overcome spatial coverage limitations. Given the often-limited spatial coverage of many drone-operated surveys, we also advocate for using these methods with large-footprint full-waveform LiDAR systems to facilitate extrapolation over broader spatial areas.
Disclosure statement
No conflict of interest was reported by the author(s).
Additional information
Funding
References
- Ahmed, O.S., Franklin, S.E., and Wulder, M.A. 2014. “Interpretation of forest disturbance using a time series of Landsat imagery and canopy structure from airborne lidar.” Canadian Journal of Remote Sensing, Vol. 39(No. 6): pp. 1–15. doi:10.5589/m14-004.
- Akujärvi, A., Lehtonen, A., and Liski, J. 2016. “Ecosystem services of boreal forests – Carbon budget mapping at high resolution.” Journal of Environmental Management, Vol. 181: pp. 498–514. doi:10.1016/j.jenvman.2016.06.066.
- Baig, M.H.A., Zhang, L., Shuai, T., and Tong, Q. 2014. “Derivation of a tasselled cap transformation based on Landsat 8 at-satellite reflectance.” Remote Sensing Letters, Vol. 5(No. 5): pp. 423–431. doi:10.1080/2150704X.2014.915434.
- Bergen, K.M., Goetz, S.J., Dubayah, R.O., Henebry, G.M., Hunsaker, C.T., Imhoff, M.L., Nelson, R.F., Parker, G.G., and Radeloff, V.C. 2009. “Remote sensing of vegetation 3-D structure for biodiversity and habitat: Review and implications for lidar and radar spaceborne missions.” Journal of Geophysical Research, Vol. 114(No. G2): 13 pages. doi:10.1029/2008JG000883.
- Bolton, D.K., White, J.C., Wulder, M.A., Coops, N.C., Hermosilla, T., and Yuan, X. 2018. “Updating stand-level forest inventories using airborne laser scanning and Landsat time series data.” International Journal of Applied Earth Observation and Geoinformation, Vol. 66: pp. 174–183. doi:10.1016/j.jag.2017.11.016.
- Bonan, G.B. 2008. “Forests and climate change: Forcings, feedbacks, and the climate benefits of forests.” Science, Vol. 320(No. 5882): pp. 1444–1449. doi:10.1126/science.1155121.
- Breiman, L. 2001. “Random forests.” Machine Learning, Vol. 45(No. 1): pp. 5–32. doi:10.1023/A:1010933404324.
- Brockerhoff, E.G., Barbaro, L., Castagneyrol, B., Forrester, D.I., Gardiner, B., González-Olabarria, J.R., Lyver, P.O.B., et al. 2017. “Forest biodiversity, ecosystem functioning and the provision of ecosystem services.” Biodiversity and Conservation, Vol. 26(No. 13): pp. 3005–3035. doi:10.1007/s10531-017-1453-2.
- Carrasco, L., Giam, X., Papeş, M., and Sheldon, K. 2019. “Metrics of lidar-derived 3D vegetation structure reveal contrasting effects of horizontal and vertical forest heterogeneity on bird species richness.” Remote Sensing, Vol. 11(No. 7): pp. 743. doi:10.3390/rs11070743.
- Coops, N.C., Tompalski, P., Goodbody, T.R.H., Queinnec, M., Luther, J.E., Bolton, D.K., White, J.C., Wulder, M.A., van Lier, O.R., and Hermosilla, T. 2021. “Modelling lidar-derived estimates of forest attributes over space and time: A review of approaches and future trends.” Remote Sensing of Environment, Vol. 260: pp. 112477. doi:10.1016/j.rse.2021.112477.
- Crist, E.P. 1985. “A TM Tasseled Cap equivalent transformation for reflectance factor data.” Remote Sensing of Environment, Vol. 17(No. 3): pp. 301–306. doi:10.1016/0034-4257(85)90102-6.
- Crist, E.P., and Cicone, R.C. 1984. “A physically-based transformation of thematic mapper data—The TM Tasseled cap.” IEEE Transactions on Geoscience and Remote Sensing, Vol. GE-22(No. 3): pp. 256–263. doi:10.1109/TGRS.1984.350619.
- Crockett, E.T.H., Atkins, J.W., Guo, Q., Sun, G., Potter, K.M., Ollinger, S., Silva, C.A., et al. 2023. “Structural and species diversity explain aboveground carbon storage in forests across the United States: Evidence from GEDI and forest inventory data.” Remote Sensing of Environment, Vol. 295: pp. 113703. doi:10.1016/j.rse.2023.113703.
- Dubayah, R., Blair, J.B., Goetz, S., Fatoyinbo, L., Hansen, M., Healey, S., Hofton, M., et al. 2020. “The global ecosystem dynamics investigation: High-resolution laser ranging of the Earth’s forests and topography.” Science of Remote Sensing, Vol. 1: pp. 100002. doi:10.1016/j.srs.2020.100002.
- Duncanson, L., Neuenschwander, A., Hancock, S., Thomas, N., Fatoyinbo, T., Simard, M., Silva, C.A., et al. 2020. “Biomass estimation from simulated GEDI, ICESat-2 and NISAR across environmental gradients in Sonoma County, California.” Remote Sensing of Environment, Vol. 242: pp. 111779. doi:10.1016/j.rse.2020.111779.
- Edwards-Jones, G., Cross, P., Foley, N., Harris, I., Kaiser, M., Vay, L.L., Rayment, M., Scowen, M., and Waller, P. 2011. “Chapter 15: Provisioning Services.” In Nano Letters, (Vol. 7): pp. 598–631. doi:10.1021/nl071254m.
- Ehbrecht, M., Schall, P., Ammer, C., and Seidel, D. 2017. “Quantifying stand structural complexity and its relationship with forest management, tree species diversity and microclimate.” Agricultural and Forest Meteorology, Vol. 242: pp. 1–9. doi:10.1016/j.agrformet.2017.04.012.
- Fekry, R., Yao, W., Cao, L., and Shen, X. 2022. “Ground-based/UAV-LiDAR data fusion for quantitative structure modeling and tree parameter retrieval in subtropical planted forest.” Forest Ecosystems, Vol. 9: pp. 100065. doi:10.1016/j.fecs.2022.100065.
- Ferraz, A., Saatchi, S., Mallet, C., Jacquemoud, S., Gonçalves, G., Silva, C., Soares, P., Tomé, M., and Pereira, L. 2016. “Airborne lidar estimation of aboveground forest biomass in the absence of field inventory.” Remote Sensing, Vol. 8(No. 8): pp. 653. doi:10.3390/rs8080653.
- Foley, J.A., Levis, S., Costa, M.H., Cramer, W., and Pollard, D. 2000. “Incorporating dynamic vegetation cover within global climate models.” Ecological Applications, Vol. 10(No. 6): pp. 1620–1632. doi:10.1890/1051-0761(2000)010[1620:IDVCWG]2.0.CO;2.
- Gauthier, S., Bernier, P., Kuuluvainen, T., Shvidenko, A.Z., and Schepaschenko, D.G. 2015. “Boreal forest health and global change.” Science, Vol. 349(No. 6250): pp. 819–822. doi:10.1126/science.aaa9092.
- Goetz, S.J., Baccini, A., Laporte, N.T., Johns, T., Walker, W., Kellndorfer, J., Houghton, R.A., and Sun, M. 2009. “Mapping and monitoring carbon stocks with satellite observations: A comparison of methods.” Carbon Balance and Management, Vol. 4(No. 1): pp. 2. doi:10.1186/1750-0680-4-2.
- Goetz, S.J., Steinberg, D., Betts, M.G., Holmes, R.T., Doran, P.J., Dubayah, R., and Hofton, M. 2010. “Lidar remote sensing variables predict breeding habitat of a Neotropical migrant bird.” Ecology, Vol. 91(No. 6): pp. 1569–1576. doi:10.1890/09-1670.1.
- Gómez, C., White, J.C., and Wulder, M.A. 2011. “Characterizing the state and processes of change in a dynamic forest environment using hierarchical spatio-temporal segmentation.” Remote Sensing of Environment, Vol. 115(No. 7): pp. 1665–1679. doi:10.1016/j.rse.2011.02.025.
- Guo, X., Coops, N.C., Tompalski, P., Nielsen, S.E., Bater, C.W., and John Stadt, J. 2017. “Regional mapping of vegetation structure for biodiversity monitoring using airborne lidar data.” Ecological Informatics, Vol. 38: pp. 50–61. doi:10.1016/j.ecoinf.2017.01.005.
- Hardiman, B.S., Bohrer, G., Gough, C.M., Vogel, C.S., and Curtis, P.S. 2011. “The role of canopy structural complexity in wood net primary production of a maturing northern deciduous forest.” Ecology, Vol. 92(No. 9): pp. 1818–1827. doi:10.1890/10-2192.1.
- Hastie, T., Tibshirani, R., and Friedman, J. H. 2009. “The elements of statistical learning: Data mining, inference, and prediction, second edition (Springer series in statistics).” In The Mathematical Intelligencer, Vol. 20(2).
- Healey, S.P., Cohen, W.B., Zhiqiang, Y., and Krankina, O.N. 2005. “Comparison of Tasseled Cap-based Landsat data structures for use in forest disturbance detection.” Remote Sensing of Environment, Vol. 97(No. 3): pp. 301–310. doi:10.1016/j.rse.2005.05.009.
- Hermosilla, T., Bastyr, A., Coops, N.C., White, J.C., and Wulder, M.A. 2022. “Mapping the presence and distribution of tree species in Canada’s forested ecosystems.” Remote Sensing of Environment, Vol. 282: pp. 113276. doi:10.1016/j.rse.2022.113276.
- Holbrook, J.D., Squires, J.R., Olson, L.E., Lawrence, R.L., and Savage, S.L. 2017. “Multiscale habitat relationships of snowshoe hares (Lepus americanus) in the mixed conifer landscape of the Northern Rockies, USA: Cross-scale effects of horizontal cover with implications for forest management.” Ecology and Evolution, Vol. 7(No. 1): pp. 125–144. doi:10.1002/ece3.2651.
- Huete, A., Didan, K., Miura, T., Rodriguez, E.P., Gao, X., and Ferreira, L.G. 2002. “Overview of the radiometric and biophysical performance of the MODIS vegetation indices.” Remote Sensing of Environment, Vol. 83(No. 1-2): pp. 195–213. doi:10.1016/S0034-4257(02)00096-2.
- Huete, A.R. 1988. “A soil-adjusted vegetation index (SAVI).” Remote Sensing of Environment, Vol. 25(No. 3): pp. 295–309. doi:10.1016/0034-4257(88)90106-X.
- Ilangakoon, N., Glenn, N.F., Schneider, F.D., Dashti, H., Hancock, S., Spaete, L., and Goulden, T. 2021. “Airborne and spaceborne lidar reveal trends and patterns of functional diversity in a semi-arid ecosystem.” Frontiers in Remote Sensing, Vol. 2: pp. 743320. doi:10.3389/frsen.2021.743320.
- Jensen, P.O., Meddens, A.J.H., Fisher, S., Wirsing, A.J., Murray, D.L., and Thornton, D.H. 2021. “Broaden your horizon: The use of remotely sensed data for modeling populations of forest species at landscape scales.” Forest Ecology and Management, Vol. 500: pp. 119640. doi:10.1016/j.foreco.2021.119640.
- Ji, Y., Huang, J., Ju, Y., Guo, S., and Yue, C. 2020. “Forest structure dependency analysis of L-band SAR backscatter.” PeerJ., Vol. 8: pp. e10055. doi:10.7717/peerj.10055.
- Kacic, P., Hirner, A., and Da Ponte, E. 2021. “Fusing sentinel-1 and-2 to model gedi-derived vegetation structure characteristics in gee for the paraguayan chaco.” Remote Sensing, Vol. 13(No. 24): pp. 5105. doi:10.3390/rs13245105.
- Key, C.H., Benson, N.C. 2006. Landscape Assessment (LA) sampling and analysis methods. USDA Forest Service - General Technical Report RMRS-GT R (164 RMRS-GT R).
- Killion, A.K., Honda, A., Trout, E., and Carter, N.H. 2023. “Integrating spaceborne estimates of structural diversity of habitat into wildlife occupancy models.” Environmental Research Letters, Vol. 18(No. 6): pp. 065002. doi:10.1088/1748-9326/acce4d.
- Lastovicka, J., Svec, P., Paluba, D., Kobliuk, N., Svoboda, J., Hladky, R., and Stych, P. 2020. “Sentinel-2 data in an evaluation of the impact of the disturbances on forest vegetation.” Remote Sensing, Vol. 12(No. 12): pp. 1914. doi:10.3390/rs12121914.
- Ma, Q., Su, Y., Hu, T., Jiang, L., Mi, X., Lin, L., Cao, M., et al. 2022. “The coordinated impact of forest internal structural complexity and tree species diversity on forest productivity across forest biomes.” Fundamental Research. doi:10.1016/j.fmre.2022.10.005.
- MacArthur, R. H., and Horn, H. S. 1969. ““Foliage profile by vertical measurements.” Ecology, Vol. 50(No. 5): pp. 802–804. doi:10.2307/1933693
- Markus, T., Neumann, T., Martino, A., Abdalati, W., Brunt, K., Csatho, B., Farrell, S., et al. 2017. “The ice, cloud, and land elevation satellite-2 (ICESat-2): Science requirements, concept, and implementation.” Remote Sensing of Environment, Vol. 190: pp. 260–273. doi:10.1016/j.rse.2016.12.029.
- Matasci, G., Hermosilla, T., Wulder, M.A., White, J.C., Coops, N.C., Hobart, G.W., Bolton, D.K., Tompalski, P., and Bater, C.W. 2018. “Three decades of forest structural dynamics over Canada’s forested ecosystems using Landsat time-series and lidar plots.” Remote Sensing of Environment, Vol. 216: pp. 697–714. doi:10.1016/j.rse.2018.07.024.
- McFeeters, S.K. 1996. “The use of the normalized difference water index (NDWI) in the delineation of open water features.” International Journal of Remote Sensing, Vol. 17(No. 7): pp. 1425–1432. doi:10.1080/01431169608948714.
- Pan, Y., Birdsey, R.A., Fang, J., Houghton, R., Kauppi, P.E., Kurz, W.A., Phillips, O.L., et al. 2011. “A large and persistent carbon sink in the world’s forests.” Science, Vol. 333(No. 6045): pp. 988–993. doi:10.1126/science.1201609.
- Pascual, C., García-Abril, A., Cohen, W.B., and Martín-Fernández, S. 2010. “Relationship between LiDAR-derived forest canopy height and Landsat images.” International Journal of Remote Sensing, Vol. 31(No. 5): pp. 1261–1280. doi:10.1080/01431160903380656.
- Pflugmacher, D., Cohen, W.B., Kennedy, R.E., and Yang, Z. 2014. “Using Landsat-derived disturbance and recovery history and lidar to map forest biomass dynamics.” Remote Sensing of Environment, Vol. 151: pp. 124–137. doi:10.1016/j.rse.2013.05.033.
- Potapov, P., Li, X., Hernandez-Serna, A., Tyukavina, A., Hansen, M.C., Kommareddy, A., Pickens, A., et al. 2021. “Mapping global forest canopy height through integration of GEDI and Landsat data.” Remote Sensing of Environment, Vol. 253: pp. 112165. doi:10.1016/j.rse.2020.112165.
- Queinnec, M., Tompalski, P., Bolton, D.K., and Coops, N.C. 2021. “FOSTER—An R package for forest structure extrapolation.” PLOS One, Vol. 16(No. 1): pp. e0244846. doi:10.1371/journal.pone.0244846.
- Ren, C., Jiang, H., Xi, Y., Liu, P., and Li, H. 2023. “Quantifying Temperate forest diversity by integrating GEDI LiDAR and multi-temporal sentinel-2 imagery.” Remote Sensing, Vol. 15(No. 2): pp. 375. doi:10.3390/rs15020375.
- Rosen, P. A., Hensley, S., and Chen, C. 2010. Measurement and mitigation of the ionosphere in L-band Interferometric SAR data. IEEE National Radar Conference – Proceedings. IEEE. doi:10.1109/RADAR.2010.5494385.
- Rouse, J. W., Haas, R. H., Schell, J. A., and Deeering, D. W. 1973. Monitoring vegetation systems in the Great Plains with ERTS (Earth Resources Technology Satellite). Third Earth Resources Technology Satellite-1 Symposium, 1.
- Roy, D.P., Kashongwe, H.B., and Armston, J. 2021. “The impact of geolocation uncertainty on GEDI tropical forest canopy height estimation and change monitoring.” Science of Remote Sensing, Vol. 4: pp. 100024. doi:10.1016/j.srs.2021.100024.
- Santoro, M., Fransson, J.E.S., Eriksson, L.E.B., Magnusson, M., Ulander, L.M.H., and Olsson, H. 2009. “Signatures of ALOS PALSAR L-band backscatter in Swedish Forest.” IEEE Transactions on Geoscience and Remote Sensing, Vol. 47(No. 12): pp. 4001–4019. doi:10.1109/TGRS.2009.2023906.
- Schneider, F.D., Longo, M., Paul-Limoges, E., Scholl, V.M., Schmid, B., Morsdorf, F., Pavlick, R.P., Schimel, D.S., Schaepman, M.E., and Moorcroft, P.R. 2023. “Remote sensing-based forest modeling reveals positive effects of functional diversity on productivity at local spatial scale.” Journal of Geophysical Research, Vol. 128(No. 6): pp. e2023JG007421. doi:10.1029/2023JG007421.
- Shimada, M., Itoh, T., Motooka, T., Watanabe, M., Shiraishi, T., Thapa, R., and Lucas, R. 2014. “New global forest/non-forest maps from ALOS PALSAR data (2007–2010).” Remote Sensing of Environment, Vol. 155: pp. 13–31. doi:10.1016/j.rse.2014.04.014.
- Silva, C.A., Duncanson, L., Hancock, S., Neuenschwander, A., Thomas, N., Hofton, M., Fatoyinbo, L., et al. 2021. “Fusing simulated GEDI, ICESat-2 and NISAR data for regional aboveground biomass mapping.” Remote Sensing of Environment, Vol. 253: pp. 112234. doi:10.1016/j.rse.2020.112234.
- Smith, A.B., Vogeler, J.C., Bjornlie, N.L., Squires, J.R., Swayze, N.C., and Holbrook, J.D. 2022. “Spaceborne LiDAR and animal-environment relationships: An assessment for forest carnivores and their prey in the Greater Yellowstone Ecosystem.” Forest Ecology and Management, Vol. 520: pp. 120343. doi:10.1016/j.foreco.2022.120343.
- Studd, E.K., Derbyshire, R.E., Menzies, A.K., Simms, J.F., Humphries, M.M., Murray, D.L., and Boutin, S. 2021. “The Purr-fect catch: Using accelerometers and audio recorders to document kill rates and hunting behaviour of a small prey specialist.” Methods in Ecology and Evolution, Vol. 12(No. 7): pp. 1277–1287. doi:10.1111/2041-210X.13605.
- Sun, G., Ranson, K.J., Guo, Z., Zhang, Z., Montesano, P., and Kimes, D. 2011. “Forest biomass mapping from lidar and radar synergies.” Remote Sensing of Environment, Vol. 115(No. 11): pp. 2906–2916. doi:10.1016/j.rse.2011.03.021.
- Sun, M., Cui, L., Park, J., García, M., Zhou, Y., Silva, C.A., He, L., Zhang, H., and Zhao, K. 2022. “Evaluation of NASA’s GEDI Lidar observations for estimating biomass in temperate and tropical forests.” Forests, Vol. 13(No. 10): pp. 1686. doi:10.3390/f13101686.
- Tang, H., and Armston, J. 2019. Algorithm theoretical basis document (ATBD) for GEDI L2B footprint canopy cover and vertical profile metrics. Greenbelt, MD: Goddard Space Flight Center.
- Thompson, I.D., Okabe, K., Tylianakis, J.M., Kumar, P., Brockerhoff, E.G., Schellhorn, N.A., Parrotta, J.A., and Nasi, R. 2011. “Forest biodiversity and the delivery of ecosystem goods and services: Translating science into policy.” BioScience, Vol. 61 (No. 12):pp. 972–981. doi:10.1525/bio.2011.61.12.7.
- Verhelst, K., Gou, Y., Herold, M., and Reiche, J. 2021. “Improving forest baseline maps in tropical wetlands using gedi-based forest height information and sentinel-1.” Forests, Vol. 12(No. 10): pp. 1374. doi:10.3390/f12101374.
- Viet Nguyen, L., Tateishi, R., Thanh Nguyen, H., C. Sharma, R., Trong To, T., and Mai Le, S. 2016. “Estimation of Tropical Forest Structural Characteristics Using ALOS-2 SAR Data.” Advances in Remote Sensing, Vol. 05(No. 02): pp. 131–144. doi:10.4236/ars.2016.52011.
- Vogeler, J.C., Fekety, P.A., Elliott, L., Swayze, N.C., Filippelli, S.K., Barry, B., Holbrook, J.D., and Vierling, K.T. 2023. “Evaluating GEDI data fusions for continuous characterizations of forest wildlife habitat.” Frontiers in Remote Sensing, Vol. 4: pp. 1196554. doi:10.3389/frsen.2023.1196554.
- Wang, S., Liu, C., Li, W., Jia, S., and Yue, H. 2023. “Hybrid model for estimating forest canopy heights using fused multimodal spaceborne LiDAR data and optical imagery.” International Journal of Applied Earth Observation and Geoinformation, Vol. 122: pp. 103431. doi:10.1016/j.jag.2023.103431.
- Watanabe, M., Shimada, M., Rosenqvist, A., Tadono, T., Matsuoka, M., Romshoo, S.A., Ohta, K., Furuta, R., Nakamura, K., and Moriyama, T. 2006. “Forest structure dependency of the relation between L-band σ 0 and biophysical parameters.” IEEE Transactions on Geoscience and Remote Sensing, Vol. 44(No. 11): pp. 3154–3165. doi:10.1109/TGRS.2006.880632.
- Wood, E.M., Pidgeon, A.M., Radeloff, V.C., and Keuler, N.S. 2012. “Image texture as a remotely sensed measure of vegetation structure.” Remote Sensing of Environment, Vol. 121: pp. 516–526. doi:10.1016/j.rse.2012.01.003.
- Xu, H. 2006. “Modification of normalised difference water index (NDWI) to enhance open water features in remotely sensed imagery.” International Journal of Remote Sensing, Vol. 27(No. 14): pp. 3025–3033. doi:10.1080/01431160600589179.
- Yu, Y., Pan, Y., Yang, X., and Fan, W. 2022. “Spatial Scale Effect and Correction of Forest Aboveground Biomass Estimation Using Remote Sensing.” Remote Sensing, Vol. 14 ((No. 12):pp. 2828. doi:10.3390/rs14122828.
- Zald, H.S.J., Wulder, M.A., White, J.C., Hilker, T., Hermosilla, T., Hobart, G.W., and Coops, N.C. 2016. “Integrating Landsat pixel composites and change metrics with lidar plots to predictively map forest structure and aboveground biomass in Saskatchewan, Canada.” Remote Sensing of Environment, Vol. 176: pp. 188–201. doi:10.1016/j.rse.2016.01.015.
- Zimble, D.A., Evans, D.L., Carlson, G.C., Parker, R.C., Grado, S.C., and Gerard, P.D. 2003. “Characterizing vertical forest structure using small-footprint airborne LiDAR.” Remote Sensing of Environment, Vol. 87(No. 2–3): pp. 171–182. doi:10.1016/S0034-4257(03)00139-1.
- Zolkos, S.G., Goetz, S.J., and Dubayah, R. 2013. “A meta-analysis of terrestrial aboveground biomass estimation using lidar remote sensing.” Remote Sensing of Environment, Vol. 128: pp. 289–298. doi:10.1016/j.rse.2012.10.017.
Appendix A
Figure A1. Map of the study sites including Montana, Washington, British Columbia, Alberta, and Yukon.

Figure A2. Map of the areas where LVIS and GEDI were sampled for the summer of 2019 to harmonize both sensors.
