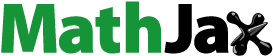
Abstract
Canopy height is a fundamental parameter for describing forest ecosystems. GEDI is a spaceborne LiDAR system that was designed to measure vegetation’s vertical structure at a global scale. This study evaluates the accuracy of GEDI-derived canopy height estimates over complex tropical forests in Mayotte Island (Overseas France) characterized by moderate height and biomass levels as well as a relatively steep terrain. The influence of GEDI signal and environmental parameters (canopy height, beam sensitivity and slope) on height estimates was assessed. Linear as well as non-linear approaches were implemented using the GEDI L2A product to estimate canopy height. Empirical models were trained on reference data derived from airborne LiDAR scanning. The results showed that using regression models built on multiple GEDI metrics yielded improved accuracies compared to a direct estimation from a single GEDI height metric. Canopy height, beam sensitivity and terrain slope were found to have a significant impact on the height metrics derived from GEDI waveforms. Conversely, both linear and non-linear regression models produced unbiased and stable estimates.
RÉSUMÉ
La hauteur de la canopée est un paramètre fondamental pour décrire les écosystèmes forestiers. GEDI est un système LiDAR spatial conçu pour mesurer la structure verticale de la végétation à l’échelle mondiale. Cette étude évalue la précision des estimations de la hauteur de la canopée à partir de GEDI sur des forêts tropicales complexes de l’île de Mayotte (France d’outre-mer) caractérisées par des hauteurs et des niveaux de biomasse modérés ainsi que par un terrain relativement escarpé. L’influence du signal GEDI et des paramètres environnementaux (hauteur de la canopée, sensibilité du faisceau laser et pente du terrain) sur les estimations de hauteur a été évaluée. Des approches linéaires et non-linéaires ont été mises en œuvre en utilisant le produit GEDI L2A pour estimer la hauteur de la canopée. Des modèles empiriques ont été entraînés sur des données de référence issues d’acquisitions par LiDAR aéroporté. Les résultats ont montré que l’utilization de modèles de régression construits à partir de plusieurs métriques GEDI permettait d’améliorer la précision par rapport à une estimation directe à partir d’une seule métrique de hauteur GEDI. La hauteur de la canopée, la sensibilité du faisceau et la pente ont eu un impact significatif sur les métriques de hauteur dérivées des formes d’onde GEDI. Par ailleurs, les modèles de régression linéaire et non-linéaire ont produit des estimations stables et sans biais.
Introduction
Tropical moist forests play a critical role in maintaining natural balances by serving as global carbon storage reservoirs. They are natural carbon dioxide sinks and account for more than 40% of the world’s terrestrial carbon stock (Pan et al. Citation2011). Measuring the standing aboveground biomass (AGB) in these forests is a fundamental step in assessing their carbon sequestration potential. AGB refers to the quantity of living vegetation above the soil, including stem, stump, branches, bark, seed and foliage. A number of studies have established allometric relationships that link the measurable structural characteristics of a forest to its AGB amounts (Asner and Mascaro Citation2014; Chave et al. Citation2005). Canopy height is a crucial parameter for quantifying biomass, as most allometric equations rely on it to derive AGB (Lefsky et al. Citation2005). Indeed, multiple authors have found that including canopy height in AGB prediction models can significantly reduce estimation errors and improve accuracy (Lima et al. Citation2012; Feldpausch et al. Citation2012), and that allometric relationships based on canopy height only may be used to predict AGB (Lefsky et al. Citation2005).
Remote sensing data has been proven to be an effective means of estimating forest characteristics at both regional and global scales over large areas that would be difficult to study otherwise (Boyd and Danson Citation2005). Light detection and ranging (LiDAR) technology is particularly well-suited for characterizing forest height and vertical structures. By emitting laser pulses and retrieving return signals, LiDAR can measure the three-dimensional structure of the environment with precision. In particular, full waveform (FW) systems are able to record the complete profiles of the return signals by sampling them in constant time intervals (Wehr and Lohr Citation1999). These systems provide geolocated one-dimensional temporal signals (referred to as return or received waveforms) describing the vertical structure of the vegetation at specific geolocations, from which a variety of forest parameters can be extracted. Airborne and spaceborne LiDARs are the two primary systems used to acquire data to describe forest vertical structures. The main advantage of airborne sensors is their high resolution (i.e., the number of returned points over a given surface), but they come at a high financial cost for data users which restricts their usage to limited areas and dates. Conversely, spaceborne LiDAR data are freely accessible and provide global coverage, but they produce low density information (spatial coverage of about 4% of the Earth’s surface for the latest system) and their high operational altitudes make them more sensitive to difficult atmospheric conditions (Dubayah et al. Citation2020; Baghdadi et al. Citation2014). The latest operating spaceborne LiDAR system providing data at a global scale is the Global Ecosystem Dynamics Investigation (GEDI) embedded on the International Space Station (ISS). By emitting laser pulses that pass through the atmosphere and interact with the objects on the Earth’s surface, GEDI records the return signals resulting from backscattering. These received waveforms are a direct proxy of the vegetation’s vertical structure and descriptive metrics can then be extracted to characterize vegetation parameters.
In tropical biomes, characterizing forest parameters from GEDI data poses important challenges due to the dense and complex nature of these ecosystems. To address these issues, statistical methods have been developed and implemented to predict canopy heights from GEDI data (Dorado-Roda et al. Citation2021; Adrah et al. Citation2022; Gupta and Sharma Citation2022). Accurately describing the vertical structure of forests from top-of-canopy to the ground using GEDI depends directly on the system’s ability to penetrate through the vegetation all the way to the ground. The assessment of GEDI’s capability to measure canopy height has highlighted the importance of signal parameters such as beam sensitivity and intensity when dealing with densely vegetated areas (Adam et al. Citation2020; Ngo et al. Citation2022). Wang et al. (Citation2022) concluded that the higher the waveform sensitivity, the lower the errors in GEDI canopy height estimation. More precisely, Fayad et al. (Citation2022) found that GEDI shots with beam sensitivity greater than 98% exhibited a significantly greater ability to detect the ground and canopy tops in a tropical context. Moreover, terrain configuration has a strong impact on the waveforms acquired by GEDI and the effects of slope on the waveforms need to be accounted for and compensated, especially over steep and rugged areas. Topographic slope is indeed a critical factor affecting the precision of canopy height estimates using spaceborne LiDAR GEDI (Liu et al. Citation2021; Fayad et al. Citation2021a). For example, the waveform extent may stretch over terrain with significant elevation differences, causing an overestimation of the relevant vegetation features (Chen Citation2010; Kutchartt et al. Citation2022). Dhargay et al. (Citation2022) investigated the impact of terrain slope on GEDI-derived canopy height in complex forest ecosystems of south-eastern Australia, revealing an increasing trend in estimation error with steeper slopes. Liu et al. (Citation2021) noted that regions characterized by dense canopy cover or steep slopes pose significant challenge for retrieving accurate canopy height information from GEDI. Similarly, in a study over multiple Eucalyptus plantations, Fayad et al. (Citation2021b) observed a 14% increase in RMSE for the estimation of dominant height in areas with slopes exceeding 20% compared to slopes ranging between 10 and 20%. Finally, the vegetation structure may also affect GEDI measurements and, in particular, tree heights and cover fraction have a direct impact on the metrics extracted from GEDI waveforms. Schlund et al. (Citation2022) built empirical models from GEDI data to estimate vegetation heights and noted that structural attributes like the canopy height itself and forest cover had a significant effect on the accuracies of height estimates, with higher and denser vegetation generally resulting in higher errors. Reciprocally, when dealing with lower tree heights, the ground return may blend with the vegetation, also causing errors in canopy height estimates. For example, Ilangakoon et al. (Citation2018) observed that using GEDI waveforms to differentiate bare ground from low-height vegetation such as shrubs could be challenging because the waveforms share the same characteristics.
This paper aims at evaluating the impact of vegetation height, beam sensitivity and slope on the estimation of canopy heights over tropical forests in Mayotte Island (Overseas France), a particularly complex context for GEDI measurements due to its spatial heterogeneity, terrain slope (mean slope of 20°), and relatively small tree height with an average height of 15 m (Dupuy et al. Citation2013). The first objective is to understand how these parameters impact the data retrieved by the GEDI sensor and, consequently, the height metrics derived from the received waveforms. The context of Mayotte Island is particularly interesting as it is one of the last islands to have forest complexes in this part of the western Indian Ocean. Secondly, in the light of this understanding, the second objective is to evaluate how these parameters can be integrated as input of prediction models to improve canopy height estimates from GEDI data. Based on the results of this analysis, we aim at offering valuable recommendations to GEDI users on how to utilize their data effectively depending on their specific work configurations, goals and objectives. The information contained in GEDI products are indeed abundant and can be leveraged in various ways to derive indicators that describe the structure of forest ecosystems. In order to do so, GEDI-derived canopy height estimates are compared to reference values from a canopy height model (CHM) obtained from airborne LiDAR scanning (ALS) data.
Materials and methods
Study area
The study was conducted over two forests in the island of Mayotte. Mayotte Island (Comoro Archipelago) is an overseas department of France located in the Indian Ocean. It is one of the few remaining islands in the western Indian Ocean with forest ecosystems. These ecosystems are primarily located in five forested areas. The areas of interest considered in this study are located over the Dapani state forest and the Majimbini departmental forest ().
Figure 1. Location of the two study sites in Mayotte Island (ESRI Satellite®) and GEDI footprints over ALS canopy height.

Dapani forest is a vital natural ecosystem that provides numerous ecological, economic and social benefits to the region. Located in the southern part of the island, the forest covers an area of approximately 340 hectares, on a relatively hilly terrain that ranges from sea level to 500 m above sea level (asl). The forest is a unique habitat characterized by a rich diversity of endemic and endangered plant and animal species, including several species of lemurs, bats and birds (Gargominy Citation2003). The climate in Dapani forest is typically tropical (group Aw according to Köppen climate classification), with two distinct seasons: the dry season from June to October and the wet season from November to May. The average annual rainfall in the southern regions of Mayotte amounts to about 1,000 mm (Lachassagne et al. Citation2014). The forest is dominated by shallow to deep soil and the vegetation is characterized by a dense canopy of evergreen trees and shrubs that provide vital carbon sequestration and oxygen production services (Pascal and Labat Citation2002).
Majimbini Forest, situated on the northeast part of the island, is also a crucial ecosystem for biodiversity conservation in the region. The forest covers an area of approximately 1,200 hectares and is characterized by a variety of vegetation types, including dense forests, shrublands and grasslands (Pascal and Labat Citation2002). Over 500 plant species are recorded in the area, including several endemic species (Gargominy Citation2003). Majimbini forest is subject to a tropical climate (group Aw according to Köppen climate classification), although more humid than Dapani forest with an average annual rainfall of approximately 2,000 mm in the northern parts of Mayotte Island (Lachassagne et al. Citation2014). The topography of Majimbini forest is characterized by a series of rolling hills, with the highest point in the forest reaching approximately 500 m asl.
The two study sites correspond to zones with similar structural properties in terms of average heights and biomass levels, with an average canopy height of 15 m and a mean aboveground biomass (AGB) ranging between 100 and 150 Mg/ha (Santoro et al. Citation2021). Even though global AGB maps such as the ones we considered are produced with quite large uncertainties (error between 50 and 100 Mg/ha in average), they still allow drawing conclusions on the relatively moderate AGB levels that characterize Mayotte’s forest ecosystems and that are significantly lower than the known saturation levels of LiDAR spaceborne sensors (Duncanson et al. Citation2022; Shendryk Citation2022). For the purpose of this study, all the data related to each site were merged together to produce a single database describing forests in Mayotte’s tropical context.
Data and processing
GEDI data
GEDI is a FW LiDAR sensor that was specifically designed to measure the vertical structure of forest ecosystems (Dubayah et al. Citation2020). Between April 2019 and April 2023, GEDI has been gathering data using three 1064 nm lasers that emit 242 pulses per second. One laser is split into coverage beams and the other two remain as power beams. All the beams are then slightly dithered to create eight parallel tracks of observations over a 4.2 km swath on the ground. The beams illuminate circular footprints of 25 m in diameter on the Earth’s surface and the waveforms of the return signals are recorded to measure the vertical structure of the vegetation. The profiles of the return signals are sampled at a fixed time interval of 1 ns which corresponds to a 15 cm sampling distance.
The GEDI data used in this study were processed by NASA’s Land Processes Distributed Active Archive Center (LP DAAC). The datasets consist of two processing levels, namely L1B (Level 1) and L2A (Level 2). The L1B product provides geolocated and smoothed waveforms along with their ancillary parameters, with an expected geolocation accuracy of about 10 m (Roy et al. Citation2021). The L2A product provides elevation and height metrics at the footprint-level, such as ground elevation, canopy top height and relative height (RH) metrics. The L2A product is derived from the received waveforms in the L1B product using six possible signal processing configurations known as algorithm setting groups. These groups determine the thresholds and smoothing settings used to interpret the received waveforms, which in turn affects the height metrics computed in the L2A product. In the context of this study, we utilized the L2A metrics that were computed using algorithm setting group number 2.
GEDI shots (i.e., L1B and L2A data associated with a footprint on the ground) acquired in the period between April 2019 and March 2023 were downloaded over the two areas of interest considered in this study. To ensure the validity of the measurements obtained by the GEDI sensor and considering the potential negative impact of atmospheric conditions, various filters were applied to remove unusable and irrelevant shots before conducting the analysis:
Shots with no mode detected (num_detectedmodes = 0) were removed. A signal with no mode is just pure noise and does not contain any information related to the vertical structure of the forest.
Shots with a null SNR (SNR = 0) were removed. These shots also correspond to pure noise.
Shots with an incorrect detection of the ground were removed. The quality assessment of the ground detection from GEDI data are performed using the corresponding Shuttle Radar Topography Mission (SRTM) digital elevation model (DEM). Even though a more accurate ALS DEM is available, we chose to use the SRTM product to place our study in an operational context where accurate products are not always available for users. Moreover, this filtering step does not require a very accurate DEM product and the filtering performed with the ALS DEM did not change the output. If the absolute difference between the elevation of the GEDI lowest mode (elev_lowestmode) and the SRTM DEM (digital_elevation_model_srtm) is greater than 100 m, then the shot is discarded.
Shots with an incomplete waveform were removed. An incomplete waveform is a partial signal that does not have a sufficient number of bins to be interpretable. If the end location of the usable portion of the waveform (search_end) is equal to the total number of bins in the waveform (rx_sample_count), then the waveform is considered incomplete.
Shots with a distance between the canopy top and the ground return (rh_100) lower than 3 m were removed. GEDI shows certain insensitivity to estimate the height of short objects. In fact, GEDI’s lasers fire shots with a pulse width of about 15 ns, which corresponds to a distance between the first emitted photons and the last of approximately 4.5 m. In an ideal scenario (perfect reflectance and no atmospheric perturbations), the returned waveform over a bare ground would have a distance between the toploc (first returned photons) and botloc (last returned photons) of approximately 4.5 m, and the derived rh_100 would amount to about 2.25 m (distance between toploc and the ground which is located in the middle between toploc and botloc). Due to noise and other sources of perturbations, this insensitivity of GEDI may increase to around 3 m.
After the filtering steps, and considering the availability of ground truth data, a total of 3384 GEDI footprints were retained out of a total of 19,272 shots (about 17%) for the combined analysis of the two study sites.
ALS data
In order to evaluate the accuracy of GEDI data in predicting canopy heights, a CHM derived from ALS data was utilized as ground truth. ALS data were acquired through surveys conducted in October 2008 by the French National Geographic Institute (IGN) in the context of the Litto3D project (Dupuy et al. Citation2013). All the LiDAR echoes were recorded and IGN then used automatic and interactive filtering to extract the first and last returns from the point clouds. The first returns are typically associated with the canopy top in forested areas, while the last returns usually indicate the ground, even though it is not always the case in densely vegetated areas. Therefore, IGN also conducted significant interactive processing to verify and reclassify points. A digital surface model (DSM) and a digital terrain model (DTM) at a resolution of 1 m were finally produced using TerraScan software (Terrasolid Ltd., Finland). The CHM used in this study was obtained by firstly calculating the difference between the DSM and the DTM, and then applying a rank-order operator median filter to generate the final CHM product (Dupuy et al. Citation2013).
Dapani and Majimbini forests are located in protected areas and consist mainly of mature old-growth and secondary woodlands. Nevertheless, given the time span between the acquisition of ground truth data and the GEDI mission, vegetation growth and changes occurred. There are no in-situ measurements of forest growth based on permanent inventory plots for these forests, which makes it impossible for a precise evaluation of changes in canopy height. Considering the rough estimate of forest AGB increase of about 1% per year in Mayotte Island (Requena Suarez et al. Citation2019), we expect an average height growth of 1 to 2 m in a ten-year time span considering simple allometric relationships for tropical forests (Chave et al. Citation2014). Some studies have investigated canopy dynamics over extended time periods in old-growth tropical rainforest landscapes. Dubayah et al. (Citation2010) utilized two LiDAR datasets from 1998 and 2005 acquired over La Selva, a tropical biome in Costa Rica (Central America), and reported a net height loss of −0.33 m for old-growth forests and a net gain of 2.08 m for secondary forests over a 7-year time span. Similarly, Kellner et al. (Citation2009) analyzed two LiDAR datasets acquired over La Selva (in 1997 and 2006) and found a mean height change of −0.32 m for old-growth landscapes over an 8.5-year period. Although the height did not vary significantly in average, local variations can still occur. For example, Dubayah et al. (Citation2010) reported local variations of approximately +/−3 m for old-growth forests over a 7-year time span.
To summarize, in the present study, the discrepancies between GEDI-derived heights and ALS CHM include the possible evolutions of the forests between the acquisition dates, together with various other factors, such as GEDI geolocation accuracy and measurement uncertainties. In the following section, we describe a filter that was applied to exclude GEDI footprints over areas where disturbances (i.e., height decrease) occurred in the period between ALS and GEDI acquisitions.
Ancillary data
Even though Dapani and Majimbini forests are located in protected areas, they are still under threat from human activities such as logging, agriculture and urbanization. Moreover, intense climatic events such as cyclones, typhoons or fires can also cause significant degradations to these ecosystems. Areas where such significant changes in height occurred need to be removed from the analysis to produce a relevant comparison between GEDI data and reference heights.
The dataset produced by the European Commission’s Joint Research Center (JRC) on forest cover change in tropical moist forests (TMF dataset) is a highly structured and comprehensive record of forest cover and land use change in these ecosystems (Vancutsem et al. Citation2021). It is compiled from optical satellite imagery spanning several decades and covers a vast area of the tropics, including the western Indian Ocean and Mayotte Island. The dataset includes information on forest area, forest cover change, and forest loss and degradation, as well as data on human activities and infrastructure that may be contributing to these changes. For the purpose of this study, we focused on the zones of the Dapani and Majimbini forests that remained undisturbed since the year 2008. An undisturbed zone is defined as an area that did not undergo any degradation or deforestation since a given year. In this way, our study focuses on old-growth and secondary undisturbed forested areas only and, therefore, the dispersion that will be observed between GEDI-derived heights and the reference CHM cannot be linked to potential major disturbances in forest cover during the time span between acquisitions.
The TMF dataset allowed restricting the study sites to the extent of undisturbed forested areas and, out of the 3384 GEDI shots that were firstly retained after the preliminary filters, a total of 2397 footprints are finally available for the assessment of canopy height estimates performed in this analysis.
Methods
The study presented herein has two main objectives. First, using a single GEDI height metric as a direct proxy of canopy height, a comparative assessment of GEDI’s capabilities in predicting canopy heights is performed. Canopy height, beam sensitivity and slope are studied independently from each other in order to evaluate their specific influence on GEDI data. Through this analysis, we aim at understanding how signal physical parameters as well as environmental configuration can impact the waveforms acquired through GEDI and thus the canopy height estimates resulting from their processing. Next, in the light of this preliminary analysis, we evaluate several regression approaches based on multiple GEDI metrics to improve the accuracy of canopy height prediction models. The aforementioned GEDI signal and environmental parameters are also included in the construction of these models to assess how their impact can be taken into account and how they can contribute to reaching better accuracies. Specifically for slope, a number of studies have been carried out to address this particular issue and to integrate slope information in estimation models. Some works retrieve terrain indices that describe the elevation configuration and directly utilize them as inputs in prediction models (Chen Citation2010; Xing et al. Citation2010). Others rely on the computation of slope-corrected waveforms to minimize the effects of slope and produce simulated metrics that are later used in prediction models (Wang et al. Citation2019; Fayad et al. Citation2021a). In the context of this study, a multilinear regression model and Random Forest (RF) estimators are implemented to take advantage of the information contained in GEDI waveforms. Specifically, to investigate the possible outcomes related to the integration of slope information, we build a RF model based on actual GEDI metrics on one hand, and a RF model trained on simulated metrics on the other hand.
GEDI and ALS canopy heights
To determine the potential of GEDI for estimating canopy heights, a single metric from the L2A product was selected as the GEDI-derived height (referred to as GEDI-CHM). From all the available values, three relative heights were identified as potential candidates to be a direct proxy of canopy height: rh_100, rh_98 or rh_95. Relative height metrics indicate the height relative to the ground at which a specific percentile of returned energy is attained. For instance, rh_95 denotes the height at which 95% of the waveform energy is reached. Relative heights of high percentiles are therefore considered as good indicators of canopy heights. In this study, we assessed the use of rh_100, rh_98 and rh_95 as direct proxies of canopy height in order to select the most adapted one in accordance with our results and previous assumptions in the literature review (Potapov et al. Citation2021; Dorado-Roda et al. Citation2021).
The accuracy of GEDI estimates is determined by comparing the relative height metric value with the corresponding reference canopy height, which is obtained from the reference CHM raster. The geolocation of the received GEDI waveforms is used to overlay GEDI footprints with the CHM raster. For each usable footprint in the GEDI L2A product, zonal statistics of the CHM raster cells contained within the extent of the footprint (a 25 m circle) are extracted. We identified two statistical values as potential candidates to represent the reference canopy height (referred to as ALS-CHM): the maximum (als100) or the 95th percentile (als95). High statistics of the CHM raster cells were chosen because they are theoretically closer to the top-of-canopy signal obtained from GEDI waveforms. Given the point density of the ALS acquisition (2 points/m2) and the CHM raster resolution (1 m), these statistics were directly computed from the raster. Adam et al. (Citation2020) supported the use of the maximum value by the fact that received waveforms begin at the highest point of vegetation within the footprints. In another study, Hilbert and Schmullius (Citation2012) obtained better correlations between ALS reference heights and spaceborne LiDAR-derived height estimates when using the maximum rather than the mean value. Specifically in this study, we noted a significant difference between als100 and als95 for some GEDI shots, especially the ones over spatially fragmented and heterogeneous canopies. Therefore, we also assessed the use of each of these two statistics to select the one most correlated with the height information contained in GEDI waveforms.
In the end, the accuracy of GEDI estimates is achieved by comparing GEDI-derived heights (GEDI-CHM) with ALS reference heights (ALS-CHM). To perform statistical analysis and evaluate the accuracies of this approach, we calculated the root mean square error (RMSE) as well as the difference between GEDI-CHM and ALS-CHM (referred to as CHM-Differences). The rh_95 metric proved to be the most suitable indicator of canopy height and the maximum als100 appeared to be the most suited value for reference canopy height.
Influence of signal physical parameters
The performances of GEDI’s canopy height estimation depend on the laser’s ability to penetrate the vegetation and detect the ground. To estimate canopy heights using a relative height metric, it is crucial to identify the ground peak in the waveform signal. Incorrect ground peak detection could be due to the laser’s inability to reach the ground or to a difficulty in isolating the ground mode from the background noise that characterizes every numeric signal (Adam et al. Citation2020). In this prospective, beam sensitivity is an important parameter that can help understand and characterize these situations. Sensitivity is defined as the maximum canopy cover that can be penetrated considering the SNR of the waveform. A high sensitivity allows penetrating denser canopies and thus reaching the ground. This signal physical parameter is therefore of paramount importance when extracting canopy heights from GEDI data and its influence was assessed to understand how it may affect the obtained results.
Influence of environmental parameters
The forest variables and height metrics measured by the backscattered GEDI signals may contain uncertainties due to the distortion caused by the topographic conditions within the footprints. In order to quantify the influence of terrain on canopy height estimates, a slope raster was derived from the DTM raster and resampled to a 5 m resolution. This upsampling was done to minimize the impact of extreme slope values (Wang et al. Citation2019; Adam et al. Citation2020). The mean slope value within the extent of the footprint was then calculated for each GEDI waveform. To better visualize the influence of slope on GEDI measurement accuracies, three slope classes were created to later group accuracy metrics by these categories: inferior to 15°, between 15 and 25°, superior to 25°. Furthermore, vegetation and canopy characteristics can also play a role in the shape of GEDI measurements. Consequently, in order to highlight the impact of tree height, the accuracy of GEDI’s canopy height estimates was assessed depending on two classes of heights: inferior to 15 m and superior to 15 m.
Regression models for canopy height estimation
After assessing the use of a single metric to derive canopy height, we built regression models between the ALS-CHM reference height data and GEDI L2A waveform metrics, as well as acquisition and environmental parameters.
In this study, three models were trained and evaluated: a Multilinear Regression (MRH), a RF regressor (RFH) based on GEDI metrics and a RF regressor (sRFH) based on simulated metrics computed from simulated ground returns (Wang et al. Citation2019; Fayad et al. Citation2021a). presents a list of the models along with the metrics used for their implementation. The goal is to understand how the parameters that impact canopy height estimation can be integrated to improve the obtained accuracies. To evaluate the performances of the models, a ten-fold cross validation was used, and the RMSE and CHM-Differences were calculated.
Table 1. List of the models used for the estimation of canopy heights and input data.
Firstly, a MRH was implemented to predict canopy heights from a set of predictive explanatory variables. In this approach, a linear relationship is built between the target variable and the predictors. Secondly, canopy heights were also estimated through non-linear non-parametric regressions using Random Forest (RF) regressors. The RF method is a machine learning algorithm that leverages ensemble of trees through a bagging strategy to predict the target variable. Compared to MRH, RFH and sRFH have the advantage of being able to model non-linear relationships between the predictors and the variable to predict. The importance of the predictors used as input variables can be quantified in order to understand the most contributing factors in the estimation task. In this analysis, RFH and sRFH differ by the way slope information is integrated to the model: the former is built on GEDI relative height metrics and mean slope of the footprints while the latter relies on metrics extracted from simulated waveforms that already take into account the slope effects. To generate new metrics from simulated waveforms, we used a method developed by Wang et al. (Citation2019). To begin with, for each GEDI footprint of the dataset, we simulated a waveform over a bare ground with the same slope value as the actual waveform acquired over forested area. The simulated waveform is based on a Gaussian signal such as the laser pulses emitted by FW LiDAR sensors (Fayad et al. Citation2021a). Over steep terrain, the waveform extent increases with slope and the ground peak exhibits a broadening of its width. To account for this, the standard deviation used in the Gaussian function to simulate a bare ground return is broadened to reflect the slope of the terrain. Once the shape of the waveform over a bare ground with known slope is determined, the simulated ground return is finally overlaid on the actual waveform by aligning the positions of the signal end of both waveforms. The superposition of the simulated ground return and the original waveform allows for the computation of new waveform metrics defined as follows:
HT_n: height between the signal end and the position at which n% of the original waveform energy is reached.
sim_HG_n: height between the signal end and the position at which n% of the simulated ground return energy is reached.
sim_RHT_n: difference between HT_n and sim_HG_n.
Results
GEDI and ALS canopy heights
We first analyzed the simple correlation between GEDI-derived heights and ALS reference data. The results presented in are scatter plots of GEDI relative height metrics (rh_100, rh_98 and rh_95) and ALS-CHM (als100). All RH metrics exhibit a quite limited linear correlation, with R2 coefficients around 0.3. We note a tendency for rh_100 to overestimate canopy heights, while rh_98 and rh_95 appear less biased and result in point distributions that are more centered on the bisector line. These observations are also confirmed by the accuracy metrics given in . In the light of these results, we chose for the next steps of this study to select rh_95 as the best metric to derive GEDI-CHM.
Figure 2. GEDI-CHM estimates from rh_100 (a), rh_98 (b) and rh_95 (c) as a function of ALS-CHM (als100).

Table 2. Accuracy of GEDI-CHM estimates (rh_100, rh_98 and rh_95) against ALS-CHM (als100 and als95).
Regarding the value used to retrieve ALS-CHM from the reference raster data, we first compared CHM-Differences with the difference between als100 and als95 for all GEDI footprints. Two main observations can be drawn from the results presented in . On one hand, for GEDI shots with a relatively low difference between als100 and als95 (inferior to 7 m, mostly corresponding to shots over homogeneous areas), CHM-Differences remain stable, and the comparison between GEDI-CHM and ALS-CHM exhibits a null bias and an RMSE of 6.3 m. On the other hand, when considering GEDI footprints with a higher difference between als100 and als95 (superior to 7 m, corresponding to shots over spatially fragmented areas), CHM-Differences increase in absolute value, with a significantly stronger bias of −3.6 m and an increased RMSE of 7.7 m. Therefore, the horizontal heterogeneity of the vegetation proves to have an impact on the accuracy of canopy height estimates from GEDI data and ALS-CHM needs to be chosen accordingly.
Figure 3. CHM-Differences as a function of the difference between als100 and als95. The difference between als100 and als95 is an indicator of spatial heterogeneity.

Similarly to what was done for GEDI-CHM, displays scatter plots of GEDI-CHM and the ALS-CHM candidates (als100 and als95). The use of als95 for reference data induces a tendency to overestimate canopy heights, even though it shows a slightly better correlation than when als100 is utilized. In terms of accuracy metrics, using the maximum value (i.e., als100) to calculate ALS-CHM allows for smaller errors and a less biased estimation, as confirmed by the results reported in .
Based on these findings, we decided to extract the maximum canopy height value als100 within a given GEDI footprint as the ALS-CHM used for reference in this study.
Influence of canopy height, sensitivity and slope
Focusing on the influence of beam sensitivity, GEDI shots with a mean slope superior to 15° were removed in order to suppress slope effects and thus concentrate on the impact of sensitivity.
Boxplots in describe the distributions of CHM-Differences (i.e., the difference between GEDI-CHM and ALS-CHM) depending on four sensitivity classes. We note that the overall CHM-Differences as well as the median value increase in absolute value when beam sensitivity decreases. Classes [0.90, 0.95] and [0.95, 0.98] display a similar behavior while GEDI shots with a sensitivity inferior to 0.90 appear to clearly underestimate canopy heights. Beam sensitivity values superior to 0.98 outperform all other classes in terms of accuracy and, contrary to them, do not show a tendency to underestimate canopy heights. These findings are confirmed by the performance metrics given in . All classes inferior to a sensitivity value of 0.98 tend to underestimate canopy heights, with bias values ranging from −3.2 to −1.8 m. Conversely, for sensitivity values superior to 0.98, rh_95 produces relatively unbiased estimates and allow reaching the lowest error, with an RMSE value of 5.8 m. Sensitivity is linked to signal penetration and ground detection: a higher sensitivity allows for better detecting the ground and in the end produces better height estimates.
Table 3. Accuracy of GEDI-CHM estimates depending on beam sensitivity, tree height and mean slope.
Similarly, to study the influence of tree height, we also kept GEDI footprints over steep areas (mean slope superior to 15°) out of the study. provides visual representations to understand the relationship between CHM-Differences and ALS-CHM. The main observation that can be drawn is that CHM-Differences increase significantly in absolute value with tree height. Moreover, we also observe two distinct behaviors depending on tree height class. On one hand, for heights ranging between 0 and approximately 15 m, rh_95 generates unbiased estimates, with a moderate bias of 0.6 m and an RMSE value of 4.7 m (see ). On the other hand, for ALS-CHM values superior to 15 m, estimates through rh_95 are negatively biased and GEDI strongly underestimates canopy heights, with a bias of −4.4 m and an RMSE of 7.6 m (see ). This underestimation for heights superior to 15 m is still clearly observed when considering only high-sensitivity shots (sensitivity superior to 0.98).
Figure 6. CHM-Differences as a function of ALS-CHM (a) and boxplots of CHM-Differences depending on ALS-CHM class (b).

Regarding the impact of slope, and in the light of our previous findings, we excluded GEDI footprints with sensitivity values inferior to 0.98 as well as those with an ALS-CHM superior to 15 m. In that way, we can focus on the impact of slope only, without adding the other effects that were previously studied. exhibits the relation between CHM-Differences and mean slope. As highlighted by the trendline, CHM-Differences increase with mean slope and steeper slopes result in an overestimation of canopy heights. Indeed, when performing the analysis by slope classes, boxplots in show that the overall CHM-Differences mostly correspond to positive values for slopes superior to 15° (i.e., an overestimation of canopy heights). On the contrary, GEDI footprints acquired over relatively flat terrain (mean slope inferior to 15°) allow reaching unbiased and more accurate height estimates, as confirmed by the accuracy metrics displayed in .
Regression models
In order to improve the accuracy of canopy height estimates, we implemented several regression models based on GEDI waveform metrics, signal parameters and terrain conditions. In this section, we assess the performances of these models and how the integration of additional data to GEDI height metrics can help reach better estimations.
Multilinear regression for height estimation (MRH)
The MRH model allows linking ALS-CHM with previously selected explanatory variables through a linear relation. When considering all the available input data (i.e., 2397 GEDI footprints), the relation built by the MRH model is as follows:
(1)
(1)
MRH produces an unbiased estimation with an RMSE of 5.7 m (see ). These results highlight the fact that using a multilinear regression built on waveform metrics allows for improving the accuracy of the estimation task compared to the direct method when using only rh_95. More importantly, when the analysis is carried out according to the signal and terrain parameters that were previously assessed (sensitivity, slope and tree height), we note that the impact of these factors is greatly lessened and that canopy height estimates are no longer dependent on them as well as completely unbiased. When considering CHM-Differences, boxplots in highlight that MRH displays the same behavior for all four sensitivity classes. Similarly, and show that the results given by MRH do not vary with slope and tree height.
Figure 8. Boxplots of CHM-Differences depending on sensitivity class (a) and CHM-Differences as a function of slope (b) and ALS-CHM (c) for MRH and rh_95.

Table 4. Accuracy of GEDI-CHM estimates for rh_95 and the three regression models.
Random Forest for height estimation (RFH and sRFH)
Both RF models also produce unbiased canopy height estimates with an RMSE of 5.7 m (see ). Similarly to MRH, building RF models based on waveform metrics results in a performance gain compared to the use of rh_95 only. All three estimators assessed in this study display equivalent accuracies in terms of bias and error (see ). Additionally, RF regressors are also capable of lessening and erasing the impact of signal and terrain parameters. In the same spirit as what is observed for MRH, and highlight the fact that canopy height estimates produced through RF models are not dependent on sensitivity. The same conclusion can be drawn regarding slope and tree height, as exhibited in and .
Figure 9. Boxplots of CHM-Differences depending on sensitivity class (a) and CHM-Differences as a function of slope (b) and ALS-CHM (c) for RFH and rh_95.

Figure 10. Boxplots of CHM-Differences depending on sensitivity class (a) and CHM-Differences as a function of slope (b) and ALS-CHM (c) for sRFH and rh_95.

The analysis of importance informs about which input variables have the most predictive power (). In the case of RFH, when looking at the ten most important predictors, relative height metrics of high percentages appear as the most important variables, with rh_95 conveying the highest contribution to the estimation task. Slope and sensitivity also bring an interesting added value as input variables when estimating CHM through a RF model. Regarding sRFH, we note that the simulated RHT metrics (which contain slope information) all appear as the most contributing predictors whereas sRFH do not seem able to leverage the information given by the simulated HG metrics. Similarly, sensitivity emerges as a contributing factor in the estimation task through sRFH.
Figure 11. Importance of input variables (ten most important) using mean decrease Gini (%IncNodePurity) for RFH (a) and sRFH (b).

Overall, when comparing estimates with ALS reference data for the whole dataset, we note that both models struggle to predict canopy heights at the edges, with an overestimation of low heights and an underestimation of high heights as shown in .
Discussion
In the scope of our study, various methods were evaluated for estimating canopy heights from GEDI data. The results indicate that utilizing waveform metrics-based prediction models yield unbiased and relatively accurate height estimates, with a best RMSE value of around 5.7 m (38%).
The most straightforward approach for estimating canopy heights from GEDI data consists in using a specific L2A level metric as a direct indicator of CHM. The metric selected for this purpose is rh_95, which represents the 95th percentile of energy return height relative to the ground. Upper relative height metrics, such as the ones we considered in our analysis, are associated with the top of forest canopies. Previous studies have observed that rh_95 showed the highest correlation with ALS-derived reference heights in tropical forest biomes, while rh_100 tended to overestimate canopy height (Potapov et al. Citation2021). Nonetheless, other works in the literature have also advocated for the use of rh_98 to derive forest heights, although in different contexts such as Mediterranean forests (Dorado-Roda et al. Citation2021) and African savannas (Li et al. Citation2023). Given the forest type of Mayotte Island and in the light of the results we obtained, rh_95 was finally selected in this study to retrieve GEDI-CHM.
The reference heights used to assess GEDI accuracies and to build regression models were derived from a CHM obtained from ALS data. In this approach, each GEDI footprint is characterized by a distinct reference height, which is compared to the canopy height derived from rh_95. In this regard, the maximum CHM value within the footprint’s extent proves to have a stronger correlation with GEDI height metrics, as the top location of the waveform (i.e., the location where the highest detected return in the waveform occurs) is directly linked to the first interaction of the laser signal with the highest object within the footprint (Hilbert and Schmullius Citation2012; Adam et al. Citation2020). However, considering the uncertainty of GEDI geolocation, extracting relevant reference heights from CHM rasters can be particularly challenging for canopies that exhibit fragmented spatial distributions or possess heterogeneous three-dimensional structures at scales similar to the 25 m GEDI footprint dimension. For example, Roy et al. (Citation2021) observed that secondary forests in the western Democratic Republic of Congo are particularly likely to produce unreliable canopy height retrievals associated with geolocation uncertainty because of their spatially fragmented and heterogeneous three dimensional structure. Specifically in this study, we note that a significant difference between als100 and als95 corresponds to GEDI shots over heterogeneous canopies, isolated trees and forest edges, amongst other cases. These shots prove to give less reliable canopy height estimates, with a stronger bias and a larger RMSE. The error increases with heterogeneity because the value extracted as reference data are probably off and do not really correspond to the associated GEDI waveform due to the geolocation uncertainty. The impact of this uncertainty is lessened when dealing with homogeneous forests because the spatial variation of tree heights is smoother. On average, using als100 (i.e., the maximum height value within the footprint) still remains the better option, even though it may give less reliable results locally. All things considered, as highlighted before in this study, the dispersion that is observed between GEDI-derived heights and ALS CHM is linked to many factors, including GEDI geolocation accuracy.
Like any remote sensing instrument, GEDI is subject to geolocation errors than can impact the accuracy of its measurements. Geolocation uncertainties in the context of GEDI refer to inaccuracies in determining precisely the spatial location of the laser footprint on the Earth’s surface. These errors can arise from various sources, including satellite orbital inaccuracies, instrumental calibration imperfections and atmospheric effects that impact the propagation of the laser beam through the atmosphere (Roy et al. Citation2021). The quantification and mitigation of geolocation errors are critical for ensuring the reliability of GEDI’s data products and various approaches were developed in order to tackle this particular issue (Hancock et al. Citation2019; Shannon et al. Citation2022; Schleich et al. Citation2023; Tang et al. Citation2023). The GEDI geolocation requirement as provided in the version 2 of the data products is that each 25 m footprint center is horizontally georeferenced to within 10 m, assuming normally distributed geolocation errors with a 0 m mean and a 10 m standard deviation (Dubayah et al. Citation2021). To address geolocation errors in our dataset and understand how they could impact our results, an investigation was undertaken to quantify the contribution of geolocation uncertainties in the dispersion and the errors observed in our study. The process involved a controlled spatial perturbation of each GEDI footprint center, systematically shifting them within both the X and Y directions across a range from −10 m to 10 m with a 5 m step size. This shifting process resulted in the creation of a comprehensive grid encompassing 25 distinct spatial locations for each footprint. Subsequently, for each possible spatial location within the grid, the associated ALS-CHM value was extracted from the reference canopy height raster. The optimal corrected spatial location and the corresponding reference ALS-CHM value were chosen based on the closest match to rh_95 relative height metric. As expected, this approach significantly reduced the canopy height estimation errors (). More interestingly, we quantified an improvement of RMSE value from 6.6 m for the initial geolocations to 3.8 m for the corrected geolocations, confirming a substantial positive impact in our analysis of geolocation errors on the use of rh_95 as a direct proxy of canopy height. Moreover, the tendencies observed regarding the influence of key parameters (sensitivity, canopy height and slope) persisted when using corrected spatial locations, albeit with reduced errors (not shown). Additionally, the implementation of regression models demonstrated a notable decrease in errors when using corrected geolocations. The RMSE value, which initially stood at 5.7 m (38%) decreased significantly to 3.2 m (21%) for both MRH and RFH. This new error on height estimation, calculated by attempting to remove the geolocation error of GEDI shots, is similar in order of magnitude to the one observed in another study over Eucalyptus plantations in Brazil (Fayad et al. Citation2021b), where GEDI geolocation uncertainty is less problematic given the fact these plantations present homogeneous heights on large areas. The enhancements we observed provide confirmation of the impact of geolocation uncertainties on canopy height estimates. They also offer insights into the maximum performance improvements achievable with refined geolocation. However, these enhancements may not be attainable within an operational context where ALS data are not available to correct GEDI spatial locations. Therefore, we retained the uncorrected results as the primary findings of this study.
Figure 13. GEDI-CHM estimates as a function of ALS-CHM when using initial geolocations (a) and corrected geolocations (b).

The ability of a laser signal to penetrate through forest cover and reach the ground is directly linked to the laser’s physical properties. The interpretation of waveform data and the subsequent computation of metrics are heavily reliant on the quality and shape of the signal. This study highlights the impact of beam sensitivity and shows that it is a crucial parameter for forest height estimation, with lower sensitivities generally resulting in an underestimation of canopy heights while higher sensitivities favor a better penetration through vegetation and thus allow a better detection of the ground. In densely vegetated areas, the ground reflection that is recorded by GEDI sensors can be weak or mixed with the background noise. In that case, footprints characterized by high sensitivities are better suited for ground detection since they can penetrate denser canopies. Fayad et al. (Citation2022) observed that footprints with sensitivities greater than 98% exhibited a deeper penetration of vegetation cover, with an average increase of 5 m in the rh_100 values compared to footprints with a sensitivity value inferior to 98%. Although a different height metric was considered in this study, the strong correlation between rh_95 and rh_100 suggests that the same conclusion can be drawn regardless of the metric used to derive heights. Several other studies have also advocated for the use of only high-sensitivity data when dealing with tall, dense or complex forests. The thresholds vary depending on the forest type and the characteristics of the study site. Rajab Pourrahmati et al. (Citation2023) indicated that removing shots with sensitivity inferior to 0.96 increased the accuracy of canopy height estimates over broadleaf and coniferous forests in Germany. A threshold of 0.95 was proposed by Dhargay et al. (Citation2022) in the context of high-density complex forests located in the Central Highlands region of Victoria in Australia. Rishmawi et al. (Citation2021) also used the same threshold in their study on key forest structure attributes of large-extent forests across the United States.
This study also assessed the impact of slope on the information derived from GEDI waveforms. When estimating canopy heights from a relative height metric such as rh_95, increasing slopes lead to an increased waveform extent, which leads to an increase of RH metrics and therefore results in an overestimation of canopy heights (Adam et al. Citation2020). Indeed, on steep forested terrain, LiDAR returns from the vegetation and the ground can coincide at the same height and, consequently, this effect leads to an overestimation of the vertical structure of the vegetation (Fayad et al. Citation2021a). The results presented in this study show that for slopes greater than 15°, rh_95 produces positively biased canopy height estimates with relatively higher errors than when considering slope values inferior to 15°. Some studies proposed a geometric correction of slope effects by applying an offset to GEDI RH metrics (Yang et al. Citation2011). This offset is computed from the mean slope value as well as the footprint diameter, in order to account for the additional height seen in the increased waveform extents. shows the improvements obtained when applying this simple correction to the rh_95 metric. We note that the dependency to slope is reduced and that the overall accuracies of canopy height estimates are improved. However, this approach also appears to over-correct slope effects, especially for steep slopes, and shows a tendency to underestimate canopy heights in general. The way slope information is integrated in prediction models is a key element toward reaching better accuracies for canopy height estimation.
Figure 14. CHM-Differences as a function of slope (a) and boxplots of CHM-Differences (b) depending on whether a simple geometric correction is applied or not.

In general, the accuracy achieved by using rh_95 as a direct substitute for GEDI-CHM is insufficient and cannot be deemed satisfactory. Other studies obtained similar accuracies in terms of errors when using a single GEDI metric to derive canopy height. For example, Dorado-Roda et al. (Citation2021) reported rRMSE values of up to 41% depending on tree species in Mediterranean forests. In their study over African savannas, Li et al. (Citation2023) presented rRMSE values between 29.8% and 40.9% depending on leaf conditions. In a tropical context, V.C. Oliveira et al. (Citation2023) obtained a percent error of 36% when using rh_95 to derive canopy height over the Brazilian Amazon forest and, similarly, in an analysis conducted over a study site in the western part of Brazil, Urbazaev et al. (Citation2021) presented an RMSE value of 7 m when estimating canopy height from GEDI data. Nevertheless, rRMSE was found to be much lower in the particular case of Eucalyptus plantations in Brazil (Fayad et al. Citation2021b), which are characterized by a very large and homogeneous cover in terms of height, an absence of gaps as well as the availability of precise field measurements. In this prospective, statistical approaches that rely on GEDI L2A metrics have been found to lead to significant improvements in CHM estimates (Lahssini et al. Citation2022). Instead of relying on a single metric, these approaches utilize multiple predictors as inputs to create and train empirical models. By doing so, the richness of the information contained in the waveforms can be leveraged, allowing for taking advantage of the interplay between all the predictors to estimate the target variable. The predictors selected in this study include height metrics that describe the canopy tops, the ground elevation and the vertical structure of the forest, which are known to be correlated with tree heights and are frequently used in canopy height estimation. In addition, signal physical characteristics and terrain configuration were taken into account through the inclusion of beam sensitivity and slope as input variables, since they were found to have a significant impact on direct CHM estimation via rh_95.
Implementing regression models demonstrates that waveform metrics combined with other external parameters have the potential to yield improved accuracies for CHM estimates. The RMSE values remain significant (5.7 m, 38%) but are nonetheless consistent with observations made in other studies over tropical ecosystems. In the global forest canopy height map for the year 2019 released by Potapov et al. (Citation2021), RMSE values of 6.6 m and 9.1 m were documented in validations against GEDI validation data and ALS data, respectively. In another study over tropical forest ecosystems in French Guiana and Gabon, Ngo et al. (Citation2023) reported errors of about 5 m on canopy height estimation. Conversely, on the same Eucalyptus dataset (Fayad et al. Citation2021b), several linear and non-linear approaches based on GEDI metrics produced much lower rRMSE values ranging between 7.8% and 12.4% depending on the model implemented. In our study, all three competitors assessed exhibit similar performances and prove to remain stable regarding all the parameters of influence that were considered. MRH uses pre-selected predictors and produces a linear relationship linking explanatory variables with canopy height. RF is commonly used for the prediction of forest stand attributes when reference in-situ data are available for model training. In the context of this study, both RFH and sRFH show a robust ability to utilize the available inputs to generate accurate CHM estimates. These models differ by the way terrain information is integrated to account for slope effects. Examining the importance reveals which variables possess the most substantial predictive power. Regarding RFH, high-percentile relative heights emerge as important indicators of canopy height (see ). These RH metrics are linked with the characterization of canopy tops and are logically selected as major factors of CHM variability. Beam sensitivity and slope, which prove to have a direct impact on the interpretability of GEDI waveforms, also appear in the most contributing input variables for RFH. When integrating terrain parameters through simulated ground returns, sRFH is capable of leveraging the slope information to produce similar estimates to RFH in terms of accuracy. Simulated RHT metrics of high percentiles are the most contributing factors in the estimation task and beam sensitivity is still an interesting variable to consider for model training and validation.
When looking at the performances of regression models depending on tree height, the scatter plots in highlight two specific tendencies for canopy height estimates through RF models. The same observations can be made for all the estimators assessed in this study. On one hand, for relatively high heights (i.e., superior to 25 m), GEDI tends to underestimate CHM. This is due to the fact this tree height range usually corresponds to higher AGB levels and denser vegetation covers. As discussed before, the laser penetration through vegetation is of paramount importance to measure the vertical structure of the forest, and it is more challenging for the signal to penetrate denser canopies. In these conditions, the ground peak in the waveform is extracted at a higher height than the actual ground, resulting in an underestimation of canopy height (Potapov et al. Citation2021; Lang et al. Citation2022; Lahssini et al. Citation2022). Conversely, for lower heights (i.e., inferior to 5 m), GEDI exhibits a tendency to overestimate CHM in a quite significant way. This overestimation of low heights is due to the waveform extent and the natural broadening of the ground return, especially over steep terrain. Indeed, if we consider a bare soil with no vegetation, the resulting echoed waveform will present a single peak with a width of about 3 m (Fayad et al. Citation2021a), even though the height for this specific footprint should be 0 m. This effect is exacerbated over steep terrain, leading to an even bigger overestimation of heights. Using RH metrics to derive canopy heights for low vegetated areas is therefore more challenging because of the impact of the ground peak width on the waveform extent.
Conclusions
In this study, we explored different methods for estimating canopy height from GEDI data while examining the impact of GEDI acquisition and environmental parameters on the precision of canopy height estimates.
In a heterogeneous forest environment, accurately assessing the precision of GEDI for height estimation poses challenges, primarily due to the geolocation error of GEDI data, even though geolocation uncertainty can be considered low. Thus, in the context of Mayotte’s forests, we posit that about half of the error in height estimation is attributable to the geolocation of GEDI shots. Taking all factors into consideration, the accuracy of canopy height estimates from GEDI data are strongly influenced by signal parameters and environmental features. The issue of LiDAR beam penetration is crucial for canopy height estimation, particularly for dense canopies such as the ones that characterize tropical ecosystems. In this study, we illustrate a reduction in laser beam penetration depth starting at approximately 15 m, in contrast to findings from other studies that reported full penetration of the LiDAR waveform up to heights of about 30 m. Hence, LiDAR beam penetration capability is strongly dependent on forest characteristics and penetration depth can differ between forests with the same height and biomass levels. Similar conclusions have been well documented in the literature regarding the penetration of L-band SAR data, with studies reporting different saturation levels of the radar signal for different forest types. Terrain slope also proves to significantly impact the received waveforms that are retrieved by GEDI sensors and needs to be accounted for when dealing with data that was acquired over steep areas.
In general, using rh_95 as a direct indicator of CHM resulted in relatively low accuracies. However, rh_95 can still be utilized to estimate canopy heights under certain conditions, particularly when reference canopy height data are unavailable. In that case, it is recommended to only use high-sensitivity data, as it yielded better results compared to using all available footprints. When reference data are available (in our case ALS data), implementing regression models can improve canopy height estimation accuracies, especially when GEDI beam sensitivity and terrain slope are accounted for in the models. The primary requirement for building empirical models is having enough data for model training. GEDI information can be utilized in various ways to estimate canopy heights, depending on data availability, operational application and the expected accuracies.
Acknowledgments
The authors would like to thank the GEDI team and NASA’s LP DAAC for providing the GEDI data. This research received funding from the French Space Study Center (CNES, TOSCA 2022 project) and the National Research Institute for Agriculture, Food and the Environment (INRAE).
Disclosure statement
No potential conflict of interest was reported by the author(s).
References
- Adam, M., Urbazaev, M., Dubois, C., and Schmullius, C. 2020. “Accuracy assessment of GEDI terrain elevation and canopy height estimates in European temperate forests: Influence of environmental and acquisition parameters.” Remote Sensing, Vol. 12(No. 23): p. 1. doi:10.3390/rs12233948.
- Adrah, E., Wan Mohd Jaafar, W.S., Omar, H., Bajaj, S., Leite, R.V., Mazlan, S.M., Silva, C.A., et al. 2022. “Analyzing canopy height patterns and environmental landscape drivers in tropical forests using NASA’s GEDI spaceborne LiDAR.” Remote Sensing, Vol. 14(No. 13): pp. 3172. doi:10.3390/rs14133172.
- Asner, G.P., and Mascaro, J. 2014. “Mapping tropical forest carbon: Calibrating plot estimates to a simple LiDAR metric.” Remote Sensing of Environment, Vol. 140 pp. 614–22. doi:10.1016/j.rse.2013.09.023.
- Baghdadi, N.N., El Hajj, M., Bailly, J.-S., and Fabre, F. 2014. “Viability statistics of GLAS/ICESat data acquired over tropical forests.” IEEE Journal of Selected Topics in Applied Earth Observations and Remote Sensing, Vol. 7(No. 5): pp. 1658–1664. doi:10.1109/JSTARS.2013.2273563.
- Boyd, D.S., and Danson, F.M. 2005. “Satellite remote sensing of forest resources: Three decades of research development.” Progress in Physical Geography: Earth and Environment, Vol. 29(No. 1): pp. 1–26. doi:10.1191/0309133305pp432ra.
- Chave, J., Andalo, C., Brown, S., Cairns, M.A., Chambers, J.Q., Eamus, D., Fölster, H., et al. 2005. “Tree allometry and improved estimation of carbon stocks and balance in tropical forests.” Oecologia, Vol. 145(No. 1): pp. 87–99. doi:10.1007/s00442-005-0100-x.
- Chave, J., Réjou-Méchain, M., Búrquez, A., Chidumayo, E., Colgan, M.S., Delitti, W.B.C., Duque, A., et al. 2014. “Improved allometric models to estimate the aboveground biomass of tropical trees.” Global Change Biology, Vol. 20(No. 10): pp. 3177–3190. doi:10.1111/gcb.12629.
- Chen, Q. 2010. “Retrieving vegetation height of forests and woodlands over mountainous areas in the Pacific Coast region using satellite laser altimetry.” Remote Sensing of Environment, Vol. 114(No. 7): pp. 1610–1627. doi:10.1016/j.rse.2010.02.016.
- Dhargay, S., Lyell, C.S., Brown, T.P., Inbar, A., Sheridan, G.J., and Lane, P.N.J. 2022. “Performance of GEDI space-borne LiDAR for quantifying structural variation in the temperate forests of south-eastern Australia.” Remote Sensing, Vol. 14(No. 15): p. 3615. doi:10.3390/rs14153615.
- Dorado-Roda, I., Pascual, A., Godinho, S., Silva, C., Botequim, B., Rodríguez-Gonzálvez, P., González-Ferreiro, E., and Guerra-Hernández, J. 2021. “Assessing the accuracy of GEDI data for canopy height and aboveground biomass estimates in Mediterranean forests.” Remote Sensing, Vol. 13(No. 12): p. 2279. doi:10.3390/rs13122279.
- Dubayah, R., Blair, J.B., Goetz, S., Fatoyinbo, L., Hansen, M., Healey, S., Hofton, M., et al. 2020. “The Global Ecosystem Dynamics Investigation: High-resolution laser ranging of the Earth’s forests and topography.” Science of Remote Sensing, Vol. 1: p. 100002. doi:10.1016/j.srs.2020.100002.
- Dubayah, R., Hofton, M., Blair, J., Armston, J., Tang, H., and Luthcke, S. 2021. GEDI L2A Elevation and Height Metrics Data Global Footprint Level V002. NASA EOSDIS Land Processes Distributed Active Archive Center. doi:10.5067/GEDI/GEDI02_A.002.
- Dubayah, R.O., Sheldon, S.L., Clark, D.B., Hofton, M.A., Blair, J.B., Hurtt, G.C., and Chazdon, R.L. 2010. “Estimation of tropical forest height and biomass dynamics using lidar remote sensing at La Selva, Costa Rica.” Journal of Geophysical Research: Biogeosciences, Vol. 115(No. G2): p. G00E09. doi:10.1029/2009JG000933.
- Duncanson, L., Kellner, J.R., Armston, J., Dubayah, R., Minor, D.M., Hancock, S., Healey, S.P., et al. 2022. “Aboveground biomass density models for NASA’s Global Ecosystem Dynamics Investigation (GEDI) lidar mission.” Remote Sensing of Environment, Vol. 270: p. 112845. doi:10.1016/j.rse.2021.112845.
- Dupuy, S., Lainé, G., Tassin, J., and Sarrailh, J.-M. 2013. “Characterization of the horizontal structure of the tropical forest canopy using object-based LiDAR and multispectral image analysis.” International Journal of Applied Earth Observation and Geoinformation, Vol. 25: pp. 76–86. doi:10.1016/j.jag.2013.04.001.
- Fayad, I., Baghdadi, N., Alcarde Alvares, C., Stape, J.L., Bailly, J.S., Scolforo, H.F., Cegatta, I.R., Zribi, M., and Le Maire, G. 2021a. “Terrain slope effect on forest height and wood volume estimation from GEDI data.” Remote Sensing, Vol. 13(No. 11): p. 2136. doi:10.3390/rs13112136.
- Fayad, I., Baghdadi, N., and Lahssini, K. 2022. “An assessment of the GEDI lasers’ capabilities in detecting canopy tops and their penetration in a densely vegetated, tropical area.” Remote Sensing, Vol. 14(No. 13): p. 2969. doi:10.3390/rs14132969.
- Fayad, I., Baghdadi, N.N., Alvares, C.A., Stape, J.L., Bailly, J.S., Scolforo, H.F., Zribi, M., and Maire, G.L. 2021b. “Assessment of GEDI’s LiDAR data for the estimation of canopy heights and wood volume of eucalyptus plantations in Brazil.” IEEE Journal of Selected Topics in Applied Earth Observations and Remote Sensing, Vol. 14: pp. 7095–7110. doi:10.1109/JSTARS.2021.3092836.
- Feldpausch, T.R., Lloyd, J., Lewis, S.L., Brienen, R.J.W., Gloor, M., Monteagudo Mendoza, A., Lopez-Gonzalez, G., et al. 2012. “Tree height integrated into pantropical forest biomass estimates.” Biogeosciences, Vol. 9(No. 8): pp. 3381–3403. doi:10.5194/bg-9-3381-2012.
- Gargominy, O. 2003. Biodiversité et conservation dans les collectivités françaises d’outre-mer. Paris: Comité français pour l’UICN.
- Gupta, R., and Sharma, L.K. 2022. “Mixed tropical forests canopy height mapping from spaceborne LiDAR GEDI and multisensor imagery using machine learning models.” Remote Sensing Applications: Society and Environment, Vol. 27: p. 100817. doi:10.1016/j.rsase.2022.100817.
- Hancock, S., Armston, J., Hofton, M., Sun, X., Tang, H., Duncanson, L.I., Kellner, J.R., and Dubayah, R. 2019. “The GEDI simulator: A large-footprint waveform lidar simulator for calibration and validation of spaceborne missions.” Earth and Space Science (Hoboken, N.J.), Vol. 6(No. 2): pp. 294–310. doi:10.1029/2018EA000506.
- Hilbert, C., and Schmullius, C. 2012. “Influence of surface topography on ICESat/GLAS forest height estimation and waveform shape.” Remote Sensing, Vol. 4(No. 8): pp. 2210–2235. doi:10.3390/rs4082210.
- Ilangakoon, N.T., Glenn, N.F., Dashti, H., Painter, T.H., Mikesell, T.D., Spaete, L.P., Mitchell, J.J., and Shannon, K. 2018. “Constraining plant functional types in a semi-arid ecosystem with waveform lidar.” Remote Sensing of Environment, Vol. 209: pp. 497–509. doi:10.1016/j.rse.2018.02.070.
- Kellner, J.R., Clark, D.B., and Hubbell, S.P. 2009. “Pervasive canopy dynamics produce short-term stability in a tropical rain forest landscape.” Ecology Letters, Vol. 12(No. 2): pp. 155–164. doi:10.1111/j.1461-0248.2008.01274.x.
- Kutchartt, E., Pedron, M., and Pirotti, F. 2022. “Assessment of canopy and ground height accuracy from GEDI LIDAR OVER steep mountain areas.” ISPRS Annals of the Photogrammetry, Remote Sensing and Spatial Information Sciences, Vol. 3: pp. 431–438. doi:10.5194/isprs-annals-V-3-2022-431-2022.
- Lachassagne, P., Aunay, B., Frissant, N., Guilbert, M., and Malard, A. 2014. “High-resolution conceptual hydrogeological model of complex basaltic volcanic islands: A Mayotte, Comoros, case study.” Terra Nova, Vol. 26(No. 4): pp. 307–321. doi:10.1111/ter.12102.
- Lahssini, K., Baghdadi, N., Le Maire, G., and Fayad, I. 2022. “Influence of GEDI acquisition and processing parameters on canopy height estimates over tropical forests.” Remote Sensing, Vol. 14(No. 24): p. 6264. doi:10.3390/rs14246264.
- Lang, N., Kalischek, N., Armston, J., Schindler, K., Dubayah, R., and Wegner, J.D. 2022. “Global canopy height regression and uncertainty estimation from GEDI LIDAR waveforms with deep ensembles.” Remote Sensing of Environment, Vol. 268: p. 112760. doi:10.1016/j.rse.2021.112760.
- Lefsky, M.A., Harding, D.J., Keller, M., Cohen, W.B., Carabajal, C.C., Del Bom Espirito-Santo, F., Hunter, M.O., and de Oliveira, R. 2005. “Estimates of forest canopy height and aboveground biomass using ICESat.” Geophysical Research Letters, Vol. 32(No. 22): p. L22S02. doi:10.1029/2005GL023971.
- Li, X., Wessels, K., Armston, J., Hancock, S., Mathieu, R., Main, R., Naidoo, L., Erasmus, B., and Scholes, R. 2023. “First validation of GEDI canopy heights in African savannas.” Remote Sensing of Environment, Vol. 285: p. 113402. doi:10.1016/j.rse.2022.113402.
- Lima, A.J.N., Suwa, R., de Mello Ribeiro, G.H.P., Kajimoto, T., dos Santos, J., da Silva, R.P., de Souza, C.A.S., et al. 2012. “Allometric models for estimating above- and below-ground biomass in Amazonian forests at São Gabriel da Cachoeira in the upper Rio Negro, Brazil.” Forest Ecology and Management, Vol. 277: pp. 163–172. doi:10.1016/j.foreco.2012.04.028.
- Liu, A., Cheng, X., and Chen, Z. 2021. “Performance evaluation of GEDI and ICESat-2 laser altimeter data for terrain and canopy height retrievals.” Remote Sensing of Environment, Vol. 264: p. 112571. doi:10.1016/j.rse.2021.112571.
- Ngo, Y.-N., Ho Tong Minh, D., Baghdadi, N., and Fayad, I. 2023. “Tropical forest top height by GEDI: From sparse coverage to continuous data.” Remote Sensing, Vol. 15(No. 4): pp. 975. doi:10.3390/rs15040975.
- Ngo, Y.-N., Huang, Y., Minh, D.H.T., Ferro-Famil, L., Fayad, I., and Baghdadi, N. 2022. “Tropical forest vertical structure characterization: From GEDI to P-band SAR tomography.” IEEE Geoscience and Remote Sensing Letters, Vol. 19: pp. 1–5. doi:10.1109/LGRS.2022.3208744.
- Oliveira, P.V., Zhang, X., Peterson, B., and Ometto, J.P. 2023. “Using simulated GEDI waveforms to evaluate the effects of beam sensitivity and terrain slope on GEDI L2A relative height metrics over the Brazilian Amazon Forest.” Science of Remote Sensing, Vol. 7:p. 100083. doi:10.1016/j.srs.2023.100083.
- Pan, Y., Birdsey, R.A., Fang, J., Houghton, R., Kauppi, P.E., Kurz, W.A., Phillips, O.L., et al. 2011. “A large and persistent carbon sink in the world’s forests.” Science (New York, N.Y.), Vol. 333(No. 6045): pp. 988–993. doi:10.1126/science.1201609.
- Pascal, O., and Labat, J.-N. 2002. Plantes et forêts de Mayotte. Paris: Muséum national d’histoire naturelle, Institut d’écologie et de gestion de la biodiversité, Service du patrimoine naturel.
- Potapov, P., Li, X., Hernandez-Serna, A., Tyukavina, A., Hansen, M.C., Kommareddy, A., Pickens, A., et al. 2021. “Mapping global forest canopy height through integration of GEDI and Landsat data.” Remote Sensing of Environment, Vol. 253: p. 112165. doi:10.1016/j.rse.2020.112165.
- Rajab Pourrahmati, M., Baghdadi, N., and Fayad, I. 2023. “Comparison of GEDI LiDAR Data Capability for Forest Canopy Height Estimation over Broadleaf and Needleleaf Forests.” Remote Sensing, Vol. 15(No. 6): p. 1522. doi:10.3390/rs15061522.
- Requena Suarez, D., Rozendaal, D.M.A., De Sy, V., Phillips, O.L., Alvarez-Dávila, E., Anderson-Teixeira, K., Araujo-Murakami, A., et al. 2019. “Estimating aboveground net biomass change for tropical and subtropical forests: Refinement of IPCC default rates using forest plot data.” Global Change Biology, Vol. 25(No. 11): pp. 3609–3624. doi:10.1111/gcb.14767.
- Rishmawi, K., Huang, C., and Zhan, X. 2021. “Monitoring Key Forest Structure Attributes across the Conterminous United States by Integrating GEDI LiDAR Measurements and VIIRS Data.” Remote Sensing, Vol. 13(No. 3): p. 442. doi:10.3390/rs13030442.
- Roy, D.P., Kashongwe, H.B., and Armston, J. 2021. “The impact of geolocation uncertainty on GEDI tropical forest canopy height estimation and change monitoring.” Science of Remote Sensing, Vol. 4: p. 100024. doi:10.1016/j.srs.2021.100024.
- Santoro, M., Cartus, O., Carvalhais, N., Rozendaal, D.M.A., Avitabile, V., Araza, A., De Bruin, S., et al. 2021. “The global forest above-ground biomass pool for 2010 estimated from high-resolution satellite observations.” Earth System Science Data, Vol. 13(No. 8): pp. 3927–3950. doi:10.5194/essd-13-3927-2021.
- Schleich, A., Durrieu, S., Soma, M., and Vega, C. 2023. “Improving GEDI footprint geolocation using a high-resolution digital elevation model.” IEEE Journal of Selected Topics in Applied Earth Observations and Remote Sensing, Vol. 16: pp. 7718–7732. doi:10.1109/JSTARS.2023.3298991.
- Schlund, M., Wenzel, A., Camarretta, N., Stiegler, C., and Erasmi, S. 2022. “Vegetation canopy height estimation in dynamic tropical landscapes with TanDEM-X supported by GEDI data.” Methods in Ecology and Evolution, Vol. 14 (No. 7): pp. 1639–1656. doi:10.1111/2041-210X.13933.
- Shannon, E.S., Finley, A.O., Hayes, D.J., Noralez, S.N., Weiskittel, A.R., Cook, B.D., and Babcock, C. 2024. “Quantifying and correcting geolocation error in spaceborne LiDAR forest canopy observations using high spatial accuracy ALS: A Bayesian model approach.” Environmetrics: p. e2840. https://doi.org/10.1002/env.2840.
- Shendryk, Y. 2022. “Fusing GEDI with earth observation data for large area aboveground biomass mapping.” International Journal of Applied Earth Observation and Geoinformation, Vol. 115: p. 103108. doi:10.1016/j.jag.2022.103108.
- Tang, H., Stoker, J., Luthcke, S., Armston, J., Lee, K., Blair, B., and Hofton, M. 2023. “Evaluating and mitigating the impact of systematic geolocation error on canopy height measurement performance of GEDI.” Remote Sensing of Environment, Vol. 291: p. 113571. doi:10.1016/j.rse.2023.113571.
- Urbazaev, M., Hess, L., Sato, L., Ometto, J., Thiel, C., Dubois, C., Adam, M., and Schmullius, C. 2021. “Accuracy assessment of terrain and canopy height estimates from ICESat-2 and GEDI LiDAR missions in temperate and tropical forests: First results.” In Proceedings of the Silvilaser. TU Wien. doi:10.34726/WIM.1984.
- Vancutsem, C., Achard, F., Pekel, J.-F., Vieilledent, G., Carboni, S., Simonetti, D., Gallego, J., Aragão, L.E.O.C., and Nasi, R. 2021. “Long-term (1990–2019) monitoring of forest cover changes in the humid tropics.” Science Advances, Vol. 7(No. 10): p. eabe1603. doi:10.1126/sciadv.abe1603.
- Wang, C., Elmore, A.J., Numata, I., Cochrane, M.A., Shaogang, L., Huang, J., Zhao, Y., and Li, Y. 2022. “Factors affecting relative height and ground elevation estimations of GEDI among forest types across the conterminous USA.” GIScience & Remote Sensing, Vol. 59(No. 1): pp. 975–999. doi:10.1080/15481603.2022.2085354.
- Wang, Y., Ni, W., Sun, G., Chi, H., Zhang, Z., and Guo, Z. 2019. “Slope-adaptive waveform metrics of large footprint lidar for estimation of forest aboveground biomass.” Remote Sensing of Environment, Vol. 224: pp. 386–400. doi:10.1016/j.rse.2019.02.017.
- Wehr, A., and Lohr, U. 1999. “Airborne laser scanning—An introduction and overview.” ISPRS Journal of Photogrammetry and Remote Sensing, Vol. 54(No. 2–3): pp. 68–82. doi:10.1016/S0924-2716(99)00011-8.
- Xing, Y., De Gier, A., Zhang, J., and Wang, L. 2010. “An improved method for estimating forest canopy height using ICESat-GLAS full waveform data over sloping terrain: A case study in Changbai mountains, China.” International Journal of Applied Earth Observation and Geoinformation, Vol. 12(No. 5): pp. 385–392. doi:10.1016/j.jag.2010.04.010.
- Yang, W., Ni-Meister, W., and Lee, S. 2011. “Assessment of the impacts of surface topography, off-nadir pointing and vegetation structure on vegetation lidar waveforms using an extended geometric optical and radiative transfer model.” Remote Sensing of Environment, Vol. 115(No. 11): pp. 2810–2822. doi:10.1016/j.rse.2010.02.021.