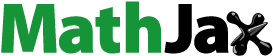
Abstract
The transition kernel of an ℝn-valued diffusion or jump diffusion process {Xt} is known to satisfy the Feller property if {Xt} is the solution of an SDE whose coefficients are Lipschitz continuous. This Lipschitz route to Feller falls short if {Xt} is the solution of an SDE whose coefficients depend on a state-dependent regime-switching process {θt}. In this paper it is shown that pathwise uniqueness and the Feller property are satisfied under mild conditions for a regime-switching jump diffusion process {Xt, θt} with hybrid jumps, i.e. jumps in {Xt} that occur simultaneously with {θt} switching.
1. Introduction
Continuous-time Markov processes with hybrid state space have proven their great value in modeling complex stochastic systems. Applications range from finance [Citation1] to air traffic [Citation2, Citation3], and to biology [Citation4]. Lygeros and Prandini [Citation5] review literature on processes that involve an ℝn-valued component {Xt} and a discrete-valued component {θt}, and where both components may interact with each other, i.e. {θt} influences the dynamics of {Xt} and vice versa. Foundations for the study of hybrid state Markov processes with a two-sided interaction between the dynamics of {Xt}and {θt} have been laid by Davis [Citation6, Citation7] for Piecewise Deterministic Markov Processes (PDMP) and by Skorohod [Citation8] and Ghosh et al. [Citation9] for state-dependent regime-switching diffusions. In contrast to the latter, PDMP involves hybrid jumps, i.e. a jump in {Xt} that occurs simultaneously with {θt} switching. Processes that involve Brownian motion and hybrid jumps have been studied from a Markov automaton perspective [Citation10–Citation15] and as pathwise unique solutions of Itô-Skorohod SDEs on a hybrid state space [Citation16 –Citation18].
A natural condition of a Markov process is that the transition kernel satisfies the Feller property, i.e. the transition kernel transforms a continuous function into a continuous function. For diffusion and jump-diffusion processes, the Feller property has shown to be satisfied if the coefficients of the corresponding SDEs satisfy Lipschitz conditions [Citation19–Citation21]. However, this Lipschitz route to the Feller property does not exist for the Markov transition kernel of a regime-switching diffusion, let alone for a regime-switching jump-diffusion that involves hybrid jumps. To improve the situation, this paper studies the Feller property of the transition kernel of a regime-switching jump-diffusion process {Xt, θt}, which is the solution of the pair of Itô-Skorohod SDEs of [Citation18]:
(1.1)
(1.1)
(1.2)
(1.2)
with {Wt} standard Brownian motion, q1(dt, dv) a martingale random measure with intensity measure μ1(dv) on mark space V, and p2(dt, du) a Poisson random measure with intensity measure μ2(du)on mark space U.
and p2(dt, du)are independent of each other and of (X0, θ0). The coefficient
in (Equation1.2
(1.2)
(1.2) ) is defined in such a way that {θt}assumes values in
, according to Xt-conditional transition rates Πij(Xt):
(1.3)
(1.3)
with
.
For specific versions of (Equation1.1(1.1)
(1.1) )-(Equation1.2
(1.2)
(1.2) ), the Feller property of the corresponding transition kernel has been shown by [Citation22–Citation27]; an overview is given in . Yin and Zhu [Citation22] study the Feller property if
, which simplifies (Equation1.1
(1.1)
(1.1) ) to the regime-switching diffusion:
(1.4)
(1.4)
Table 1. Conditions imposed by
[Citation22–Citation27] on key elements in (Equation1.1(1.1)
(1.1) )-(Equation1.2
(1.2)
(1.2) ) for the Feller property.
Yin and Zhu [Citation22, Lemma 2.14] first remind that the Feller property is satisfied, if Πij(x) is x-invariant. Subsequently, Yin and Zhu [Citation22, Theorem 2.18] develop a novel approach in proving that the Feller property is satisfied if Πij(x) is continuous in x. Shao [Citation23] and Zhang [Citation24] extend the results of [Citation22] to situations of countable . Xi et al. [Citation25] and Kunwai and Zhu [Citation26] extend the Feller property results of [Citation22, Citation23] to regime-switching jump-diffusions of type (Equation1.1
(1.1)
(1.1) )-(Equation1.2
(1.2)
(1.2) ) with locally Lipschitz
. [Citation22–Citation26] have
in common, i.e. there are no hybrid jumps.
In unpublished report [Citation27], Feller property is proven in case of hybrid jumps and finite M under the conditions that Πij(x) is Lipschitz and is bounded. This proof makes use of a stochastic continuity result by Gihman and Skorohod [Citation28] for a non-switching jump-diffusion solution of (Equation1.1
(1.1)
(1.1) ) for
The objective of this paper is to relax the Lipschitz and bound conditions that [Citation27] imposes on Πij(x) and to continuity and linear growth conditions respectively. To make this feasible, section 2 introduces a partition of the mark space U, that differs from the common partition [Citation9, Citation16–Citation18, Citation22–Citation27]. In addition, the following generalizations of the mark space U and the mapping
are adopted. Firstly, a multi-dimensional mark space is assumed, i.e.
. Secondly, the measure μ2 is assumed to be a product of a Lebesque measure on ℝ and probability measure on ℝd. Thirdly, while only one mark component of u influences coefficient
in (Equation1.2
(1.2)
(1.2) ), all mark components of u influence coefficient
in (Equation1.1
(1.1)
(1.1) ).
The novel mark space partition and the generalization of U and imply the need of a novel proof of the existence of a pathwise unique solution of (1.1–1.2), as well as a novel route in using the stochastic continuity result of [Citation28] in the derivation of the Feller property for the corresponding transition kernel.
This paper is organized as follows. Section 2 starts presenting the novel partitioning and extension of the mark space U and definitions of and
. Subsequently, Section 2 develops relevant characterizations and an illustrative hybrid jump example. Section 3 starts with the background of pathwise uniqueness of a jump-diffusion solution of a non-switching Itô-Skorohod SDE of type (Equation1.1
(1.1)
(1.1) ) if
. Subsequently, Section 3 derives the existence of pathwise unique solutions of Itô-Skorohod SDEs (Equation1.1
(1.1)
(1.1) )-(Equation1.2
(1.2)
(1.2) ) under the novel mark space partition and generalization of U and
. Finally, Section 3 characterizes the corresponding Markov transition kernel. Section 4 proves that this Markov transition kernel satisfies the Feller property. Section 5 provides a discussion of results.
2. Composition and characterization of p2(dt, du) generated discontinuities
2.1. Composition
Throughout the paper all processes are defined on a stochastic basis with (Ω, ℱ) a measurable space that is equipped with a collection
of increasing right-continuous sub σ-algebra’s ℱt of ℱ; P is a probability measure defined on the σ-algebra ℱ.
Itô-Skorohod SDEs (Equation1.1(1.1)
(1.1) )-(Equation1.2
(1.2)
(1.2) ) evolve on hybrid space ℝn×𝕄, where
is a finite set of unit vectors
. We assume Borel measurable mappings
,
,
,
,
, with
; and that {Wt}, q1(dt, dv), and p2(dt, du) satisfy (A1)-(A4):
(A1) {Wt} is an m−dimensional standard Wiener process;
(A2) q1(dt, dv) is a martingale random measure on a Blackwell space {V, 𝒱}, associated to a homogeneous Poisson random measure p1(dt, dv) with intensity dt×μ1(dv), where μ1(dv) is a positive σ-finite measure on {V, 𝒱};
(A3) p2(dt, du) is a homogeneous Poisson random measure on a Blackwell space {U, 𝒰} with intensity dt×μ2(du), where μ2(du) is a positive σ-finite measure on {U, 𝒰};
(A4) Wiener process {Wt} and Poisson random measures p1(dt, dv) and p2(dt, du) are mutually independent, and are also independent of initial condition .
The right-continuous sub σ-algebra ℱt is assumed to be endowed with the restriction to [0,t] of Wiener process {Wt} and Poisson random measures p1(dt, dv) and p2(dt, du).
Composition of the p2(dt, du) related measure μ2(du) and mappings and
are specified in (C0) below:
(C0) Conditions imposed on μ2(du), and on coefficients and
Measure μ2(du) satisfies:
with m(du0) Lebesque measure on ℝ and
a probability measure on ℝd. Mappings
and
are composed as follows:
with measurable mappings
; and where Δij(x),
, are the following intervals on the real line:
with constant Cλ < ∞, and measurable mappings
.
Remark 2.1.
The μ2(du) product composition in (2.1a) and the composition in (2.1c) follow [Citation16]. The composition of
in (2.1b) is similar to the one in [Citation22–Citation27]. However, the Δij(x)’s defined by (2.1d) differ from those in [Citation16, Citation22–Citation27] on the following three aspects. Firstly, in addition to the sets
, (2.1d) also defines non-empty sets Δii(x). Secondly, Δij(x) in (2.1d) has an x-invariant left-hand-side boundary. Thirdly for i≠k the sets Δij(x) and Δkj(x) in (2.1d) have overlap.
2.2. Characterizations
Condition (C0) leads to the following characterizations.
Characterization 2.2: Thanks to the Δij(x) definition in (2.1d), for all ei∈𝕄, :
(2.2)
(2.2)
The non-overlap property in (Equation2.2(2.2)
(2.2) ) will be used in proving existence of pathwise uniqueness of solutions of (Equation1.1
(1.1)
(1.1) )-(Equation1.2
(1.2)
(1.2) ) and subsequently in proving the Feller property of the Markov kernel.
Characterization 2.3:If eq. (1.2) admits a pathwise unique solution {θt} that assumes values in then {θt} is an {Xt}-dependent N−state switching process with N×N transition rate matrix
, with
and off-diagonal components satisfying:
(2.3)
(2.3)
Characterization 2.4: (Equation1.1(1.1)
(1.1) )-(Equation1.2
(1.2)
(1.2) ) includes the special case that the {θt} transition rate matrix is x-invariant, i.e. Π(x) = Π. Then p2(dt, du) in (Equation1.1
(1.1)
(1.1) )-(Equation1.2
(1.2)
(1.2) ) can still generate simultaneous jumps in {Xt} and in {θt}, as a result of which the process {θt} is not independent of the process {Xt}. Because in this case the term
in (2.1b-c) is x-invariant, pathwise uniqueness and Feller property of the joint solution of (Equation1.1
(1.1)
(1.1) )-(Equation1.2
(1.2)
(1.2) ) follows from the classical reasoning under the additional conditions that λii(x) is x-invariant and φij(x) satisfies a local Lipschitz condition.
Characterization 2.5: If p2(dt, du) generates a joint random mark at moment τ, and if
and
, then the jump in {Xt, θt} satisfies:
(2.4)
(2.4)
where the last equality follows from substituting eq. (2.1b,c). From eq. (2.4) can be seen when the different types of jumps in {Xt, θt} happen:
Jump in {Xt} only: if u0∈Δkk(x) and
;
Jump in {θt} only: if
such that u0∈Δkj(x) and
;
Hybrid jump: if
such that u0∈Δkj(x) and
;
No jump: if none of conditions 1) - 3) hold true.
2.3. Hybrid jump example of (1.1)-(1.2) under (C0)
In air traffic, spontaneous changes of aircraft dynamics pose safety risk. For example, the aircraft flight mode may suddenly switch from level flight to climb or to descent. Consider a discrete-valued state space consisting of three vertical flight modes: Level flight (i = 1), Climb (i = 2), and Descent (i = 3). The vertical flight mode process evolves according to SDE (Equation1.2
(1.2)
(1.2) ), with
satisfying (2.1b) and (2.1d), with non-zero rates λ12(x), λ13(x), λ21(x) and λ31(x). Because an aircraft undergoes a finite number of discontinuities on a finite time interval, Xt is modeled by (Equation1.1
(1.1)
(1.1) ) with
, where the Xt components in vertical direction are position Xy, t and velocity Xv, t. The evolution in vertical position satisfies
, i.e.
and
. In between two successive mode switching’s, the evolution in vertical velocity satisfies
, i.e.
and
, with fθ a feedback factor to keep the aircraft near the desired vertical velocity vθ under mode θ, and βθ the level of Brownian motion disturbance in vertical velocity under mode θ.
At moments of mode switching the random measure p2(dt, du) also generates a jump in vertical velocity. This is modeled using eq. (2.1c) with , and with
(2.5)
(2.5)
where the first two lines capture a reset of the vertical velocity to zero simultaneously with a mode switching from Climb or from Descent to Level flight. The third and fourth lines capture a jump in the vertical velocity to random value
or
from probability measure
, simultaneously with a mode switching from Level flight to Climb or to Descent.
3. Existence of pathwise unique solutions
Characterization 2.4 explains that the classical route to pathwise unique joint solution of (Equation1.1(1.1)
(1.1) )-(Equation1.2
(1.2)
(1.2) ) falls short if one or more of the following three conditions apply: 1) Π(x)≠Π; 2) λii(x)≠λii, for some i; and 3)
is non-Lipschitz in x, for some i,j. To address these cases, in subsection 3.1 a non-Lipschitz route in literature for an Itô-Skorohod SDE on a Euclidean space is presented. Next, in subsection 3.2, the existence of a pathwise unique solution of (Equation1.1
(1.1)
(1.1) )-(Equation1.2
(1.2)
(1.2) ) under (C0) is proven under weak conditions, by elaboration of the mapping of Itô-Skorohod SDEs and pathwise uniqueness conditions from Euclidean space to hybrid space.
3.1. Itô-Skorohod SDE on Euclidean space
The Itô-Skorohod SDE considered is:
(3.1)
(3.1)
with
measurable mappings, and with {Wt}, q1(dt, dv) and p2(dt, du) satisfying (A1)-(A4). Moreover, the coefficients
, and
satisfy conditions (L1)-(L3):
(L1) There exists a constant cG < ∞ such that for all :
(L2) For each positive integer r there is constant such that for all
:
(L3) There is a constant cJ < ∞ such that .
If , then conditions for existence of pathwise unique cadlag solution of (Equation3.1
(3.1)
(3.1) ) are well known under (L1) and (L2), e.g. [Citation20, Citation21]. If
, (L3) assures that p2(dt, du) generates a finite number of additional discontinuities in {ξt} on a finite interval, as a result of which no Lipschitz condition on
is needed to prove existence of pathwise unique solution of (Equation3.1
(3.1)
(3.1) ) [Citation28–Citation30].
Proposition 3.1.
Let assumptions (A1)-(A4) hold true and let the coefficients of Itô-Skorohod SDE (Equation3.1(3.1)
(3.1) ) satisfy conditions (L1)-(L3). Then for each F0-measurable square integrable
-valued random variable η, SDE (Equation3.1
(3.1)
(3.1) ) with initial condition ξ0 = η admits a pathwise unique Ft-measurable cadlag solution
, satisfying
. Moreover, for each
there exists a random measurable function
, such that
In having shown existence and pathwise uniqueness of a solution of an SDE driven by Brownian motion and Poisson random measure, the classical approach in showing stochastic continuity of this solution is to make use of Lipschitz continuity for all terms in (Equation3.1(3.1)
(3.1) ). Because Proposition 3.1 does not assume Lipschitz continuity on the term involving
, another proof is needed to show that the solution process of (Equation3.1
(3.1)
(3.1) ) is stochastically continuous. Such proof has been given by Gihman and Skorohod [Citation28, pp. 249–254], the result of which is stated in Proposition 3.2 below.
Proposition 3.2.
Let, in addition to the assumptions and conditions of Proposition 3.1, be continuous in ξ, for almost all u in measure μ2(du). Then for any converging sequence {ηκ} that is independent of {Wt}, p1(dt, dv), p2(dt, du) and ξ0, and has limit
3.2. Itô-Skorohod SDE on a hybrid space
To prove existence of a pathwise unique solution of (Equation1.1(1.1)
(1.1) )-(Equation1.2
(1.2)
(1.2) ), in addition to condition (C0), we adopt the following conditions (C1)-(C3):
(C1) There exists a constant ℓG such that for all ei∈𝕄 and all x∈ℝn:
(C2) For each positive integer r there exists a constant such that for all ei∈𝕄 and all
:
(C3) There exists a constant ℓφ < ∞ such that for all and all x∈ℝn:
To prove existence of a pathwise unique solution, the system of equations (1.1)-(Equation1.2(1.2)
(1.2) ) is mapped on (Equation3.1
(3.1)
(3.1) ). Subsequently it is verified that conditions (C0)-(C3) imply that conditions (L1)-(L3) in Proposition 3.1 hold true. Hence, Proposition 3.1 yields existence of a pathwise unique solution of (Equation1.1
(1.1)
(1.1) )-(Equation1.2
(1.2)
(1.2) ).
Theorem 3.3.
Let assumptions (A1)-(A4) and conditions (C0)-(C3) hold true. Then for each F0-measurable square integrable Rn×M-valued random variable η, SDE (Equation1.1(1.1)
(1.1) )-(Equation1.2
(1.2)
(1.2) ) with initial condition
, admits a pathwise unique Ft-measurable cadlag solution
, satisfying
. Moreover, for each
there exists a random measurable function
, such that
Proof.
See Appendix A, which also explains differences with the proof in [Citation27]. ▪
A well-known consequence of Theorem 3.3 is that joint solutions of (Equation1.1(1.1)
(1.1) )-(Equation1.2
(1.2)
(1.2) ) define a Markov transition kernel, e.g. [Citation19, Citation20].
Corollary 3.4.
Theorem 3.3’s pathwise unique Ft-measurable SDE solution process is a time-homogeneous Markov process with respect to F = {Ft}, with Markov transition kernel
,
, forming a semigroup, i.e.
(3.2)
(3.2)
Remark 3.5.
Conditions (C0) through (C3) adopted in Theorem 3.3 mean that for the existence of a pathwise unique solution of (Equation1.1(1.1)
(1.1) )-(Equation1.2
(1.2)
(1.2) ) it is sufficient for λij(x) and
to be measurable only. In the next section it will be shown that the Feller property holds true if λij(x) and
are continuous in x, for each
4. Feller property of the Markov transition kernel
Let denote the space of bounded functions on ℝn×𝕄 that are continuous on ℝn for each value in 𝕄. Following [Citation19], transition kernel
,
,
is said to satisfy the Feller property if
, for each
.
The objective is to prove that the Markov transition kernel of Corollary 3.4 satisfies the Feller property. This is accomplished by adopting additional conditions (C4)-(C5):
(C4) λij(x) is continuous in x for all
(C5) is continuous in x, for every
, for all
Under these additional assumptions, it will be proven in Theorem 4.2 that solution of (Equation1.1
(1.1)
(1.1) )-(Equation1.2
(1.2)
(1.2) ) is stochastically continuous in
. To prepare, we first prove in Lemma 4.1 that
and
are continuous in x, for almost all u in measure μ2(du).
Lemma 4.1.
Under (C0), (C4) and (C5), the mapping
(4.1)
(4.1)
is continuous in x, for almost all u in measure μ2(du).
Proof.
Substitution of (2.1b,c) in (Equation4.1(4.1)
(4.1) ) yields:
Due to (C5), is continuous in x∈ℝn for every
and every
Hence, for the completion of the proof, it remains to be shown that the process
is continuous in x, for almost all u0 in measure m(du0). To prove the latter, for each sequence
that is independent of {Wt}, p1(dt, dv), p2(dt, du) and ξ0, and which converges to x∈ℝn, i.e.
, we evaluate:
Thanks to non-overlap property (Equation2.2(2.2)
(2.2) ), there is only one non-zero term in each of the summations over j. Hence the summation can be moved outside
. Due to Fubini’s theorem, the summation can subsequently be moved outside the integral. These two moves yield:
Hence
Following (C4), λij(x) is continuous in x, which means . Hence
The latter implies that the mapping is continuous in x for every
and almost all u0 in measure m(du0). ▪
Remark 4.2.
There are two differences with [Citation27]. The first difference is that under the common partition, non-overlap property (Equation2.2(2.2)
(2.2) ) does not hold true. Therefore [Citation27; Appendix C] cannot shift the summation outside
, which makes the proof more demanding and needs Lipschitz λij(x). The second difference is that instead of proving, [Citation27; Remark 2.4] assumes that
is continuous in x for almost all u in measure μ2(du).
Next, Proposition 3.2 and Lemma 4.1 are used to prove in Theorem 4.3 that solution of (Equation1.1
(1.1)
(1.1) )-(Equation1.2
(1.2)
(1.2) ) is stochastically continuous in
. From this stochastic continuity result the Feller property follows in Theorem 4.4.
Theorem 4.3.
Let in addition to the assumptions and conditions of Theorem 3.3, conditions (C4) and (C5) hold true. Then solution of (Equation1.1
(1.1)
(1.1) )-(Equation1.2
(1.2)
(1.2) ) is stochastically continuous in
, i.e. for any converging sequence {ηκ},
, that is independent of {Wt}, p1(dt, dv), p2(dt, du) and ξ0, and that converges to
:
(4.2)
(4.2)
Proof.
From the proof of Theorem 3.3 we know that SDE (Equation1.1(1.1)
(1.1) )-(Equation1.2
(1.2)
(1.2) ) has a pathwise unique ℱt-measurable solution for each initial value in ℝn×𝕄. From Lemma 4.1 we know that
is continuous in x, for almost all
in measure μ2(du). Then, from Proposition 3.2 follows for all r > 0 and ε > 0:
(4.3)
(4.3)
Hence solutions of SDE (Equation3.1(3.1)
(3.1) ) are stochastically continuous. Because SDE (Equation3.1
(3.1)
(3.1) ) embeds SDE (Equation1.1
(1.1)
(1.1) )-(Equation1.2
(1.2)
(1.2) ), limit (Equation4.3
(4.3)
(4.3) ) also holds true for (Equation1.1
(1.1)
(1.1) )-(Equation1.2
(1.2)
(1.2) ). ▪
Theorem 4.4.
Under assumptions and conditions of Theorem 4.3, the Markov transition kernel Qt(ξ;B) satisfies the Feller property.
Proof.
Because solutions of (Equation1.1
(1.1)
(1.1) )-(Equation1.2
(1.2)
(1.2) ) are stochastically continuous w.r.t.
(see Theorem 4.3), for all
the process
also is stochastically continuous w.r.t.
. This means that
for all
. Because we know
this implies
, i.e. the Feller property holds true for
. ▪
Corollary 4.5.
Under the assumptions and conditions of Theorem 4.3, the time-homogeneous Markov process solution of (Equation1.1
(1.1)
(1.1) )-(Equation1.2
(1.2)
(1.2) ) is a strong Markov process, i.e. for any stopping time τ with
, for every
and
:
Proof.
See Friedman [Citation19, Theorem 2.4]. ▪
5. Discussion of results
This paper has studied Feller property of the Markov transition kernel of regime-switching jump diffusion processes with hybrid jumps. The processes considered evolve in a hybrid state space, as solutions of SDE’s that are driven by Brownian motion and Poisson random measures. The results obtained significantly enhance Feller results of [Citation22–Citation27] for SDE’s that involve regime-switching.
These results also open directions for relevant follow-on research. One is the extension to a countable number of modes [Citation23–Citation26]. Another extension is to further relax growth and local Lipschitz conditions for the mode-dependent drift coefficient [Citation22, Citation25, Citation26]. Thirdly, [Citation11–Citation15, Citation17, Citation18] show relevant larger classes of continuous-time hybrid state Markov processes; the study of the Feller property of their Markov transition kernels forms another direction for relevant follow-on research.
Acknowledgement
The author would like to thank anonymous reviewers for comments and suggestions that were very helpful in improving the paper.
Disclosure statement
No potential conflict of interest was reported by the author.
Additional information
Funding
References
- Glasserman, P., (2004). Monte Carlo Methods in Financial Engineering. New York: Springer Science & Business Media.
- De Santis, E., Di Benedetto, M.D., Di Gennaro, S., D’Innocenzo, A., Pola, G. (2006). Critical observability of a class of hybrid systems and applications to air traffic management. In: Blom H.A.P., Lygeros, J., eds. Stochastic Hybrid Systems, Theory and Safety Critical Applications. Berlin: Springer, p. 141–170.
- Blom, H.A.P., Krystul, J., Bakker, G.J., Klompstra, M.B., Klein Obbink, B. (2007). Estimation of collision risk in free flight air traffic by sequential Monte Carlo simulation. In: Cassandras, C.G., Lygeros, J., eds. Stochastic Hybrid Systems. Boca Raton: Taylor & Francis/CRC Press, pp. 249–281.
- Li, X., Omotere, O., Qian, L., Dougherty, E.R. (2017). Review of stochastic hybrid systems with applications in biological systems modeling and analysis. Eurasip J. on Bioinformatics and Systems. 8:1–12.
- Lygeros, J., Prandini, M. (2010). Stochastic Hybrid Systems: A Powerful Framework for Complex, Large Scale Applications. European Journal of Control. 16(6):583–594. doi:10.3166/ejc.16.583-594.
- Davis, M.H.A. (1984). Piecewise-Deterministic Markov Processes: A General Class of Non-Diffusion Stochastic Models. Journal of the Royal Statistical Society: Series B (Methodological). 46(3):353–388. doi:10.1111/j.2517-6161.1984.tb01308.x.
- Davis, M.H.A., (1993). Markov Models and Optimization, London: Chapman & Hall.
- Skorohod, A.V. (1989). Asymptotic Methods in the Theory of Stochastic Differential Equations, Providence, RI: Amer. Math. Soc.
- Ghosh, M.K., Arapostathis, A., Marcus, S.I. (1993). Optimal Control of Switching Diffusions with Application to Flexible Manufacturing Systems. SIAM J. Control Optim. 31(5):1183–1204. doi:10.1137/0331056.
- Hespanha, J.P. (2005). A model for stochastic hybrid systems with application to communication networks. Nonlinear Analysis: Theory, Methods & Applications. 62(8):1353–1383. doi:10.1016/j.na.2005.01.112.
- Pola, G., Bujorianu, M., Lygeros, J., Di Benedetto, M.D. (2003). Stochastic hybrid models: An overview with applications to air traffic management. Analysis and Design of Hybrid Systems (ADHS), Proc. IFAC Conf. Saint Malo, Brittany, France. Oxford: Elsevier, pp. 45–50.
- Bujorianu, M.L. (2004). Extended stochastic hybrid systems and their reachability problem, In: Alur R., Pappas, G., eds. Hybrid Systems: Computation and Control LNCS 2993. Berlin: Springer, pp. 234–249.
- Bujorianu, M.L., Lygeros, J. (2006). Towards a general theory of stochastic hybrid systems. In: Blom H.A.P., Lygeros, J., eds. Stochastic Hybrid Systems, Theory and Safety Critical Applications. Berlin: Springer, pp. 3–30.
- Cassandras, C.G., Lygeros, J. (2007). Stochastic Hybrid Systems Research Issues and Areas. Stochastic Hybrid Systems: Recent Developments and Research Trends. Boca Raton: Taylor & Francis, pp. 1–14.
- Bect, J. (2010). A unifying formulation of the Fokker–Planck–Kolmogorov equation for general stochastic hybrid systems. Nonlinear Anal.: Hybrid Syst. 4(2):357–370. doi:10.1016/j.nahs.2009.07.008.
- Blom, H.A.P. (2003). Stochastic hybrid processes with hybrid jumps. In: Analysis and Design of Hybrid Systems. Proc. IFAC Conf. Saint-Malo, Brittany, France. Oxford: Elsevier, pp. 319–324.
- Ghosh, M.K., Bagchi, A. (2004). Modeling stochastic hybrid systems. In: Cagnol J., Zolèsio, J.P., eds. System Modeling and Optimization. Proc. 21st IFIP TC7 Conference, Sophia, Antipolis, France. New York: Springer, pp. 269–279.
- Krystul, J., Blom, H.A.P., Bagchi, A. (2007). Stochastic differential equations on hybrid state spaces, Stochastic Hybrid Systems: Recent Developments and Research Trends, C. G. Cassandras and J. Lygeros. Boca Raton: Taylor & Francis/CRC Press, pp. 15–45.
- Friedman, A.V. (1975). Stochastic Differential Equations and Applications, Vol. 1, New York: Academic Press.
- Protter, P.E. (2003). Stochastic Integration and Differential Equations, 2nd ed. Berlin: Springer.
- Oksendal, B., Sulem, A. (2005). Applied Stochastic Control of Jump Diffusions. Berlin: Springer.
- Yin, G.G., Zhu, C. (2010). Hybrid Switching Diffusions; Properties and Applications. New York: Springer.
- Shao, J. (2015). Strong Solutions and Strong Feller Properties for Regime-Switching Diffusion Processes in An Infinite State Space. SIAM J. Control Optim. 53(4):2462–2479. doi:10.1137/15M1013584.
- Zhang, S.-Q. (2020). Regime-switching diffusion processes: strong solutions and strong Feller property. Stochastic Analysis and Applications. 38(1):97–123. doi:10.1080/07362994.2019.1650066.
- Xi, F., Yin, G., Zhu, C. (2019). Regime-switching jump diffusions with non-Lipschitz coefficients and countably many switching states: Existence and uniqueness, Feller, and strong Feller properties, Modeling, Stochastic Control, Optimization, and Applications, Yin G., Zhang Q., p. 571–599. Cham: Springer.
- Kunwai, K., Zhu, C. (2020). On Feller and strong Feller properties and irreducibility of regime-switching jump diffusion processes with countable regimes. Nonlinear Anal.: Hybrid Syst. 38:100946. doi:10.1016/j.nahs.2020.100946.
- Krystul, J., Bagchi, A., Blom, H.A.P. (2011). On strong Markov property of solutions to stochastic differential equations on hybrid state spaces, Report, University of Twente. https://repository.tudelft.nl/islandora/object/uuid:948fc4dd-66f5-42bc-82e6-8770d371add3.
- Gihman, I.I., Skorohod, A.V. (1982). Stochastic differential equations and their applications (in Russian). Kiev: Naukova Dumka.
- Lepeltier, J.P., Marchal, B. (1976). Probleme des martingales et equations differentielles stochastiques associees a un operateur integro-differentiel. Ann. Inst. Henri Poincare B. 12:43–103.
- Ikeda, N., Watanabe, S. (1981). Stochastic Differential Equations and Diffusion Processes. Amsterdam: North-Holland.
Appendix A
Proof of Theorem 3.3
SDEs (Equation1.1(1.1)
(1.1) )-(Equation1.2
(1.2)
(1.2) ) on ℝn×𝕄 are mapped on SDE (Equation3.1
(3.1)
(3.1) ) on
, with
and:
where ON and ON×m denote zero N-vector and zero N×m-matrix respectively, and αi(z) is an elevated cosine on the set
and zero outside this set, i.e.
This implies that αi(z) is Lipschitz continuous and that αi(z)αj(z) = 0 for each .
We have to verify that posing conditions (C0)-(C3) on coefficients a(x, ei), b(x, ei), ,
, and
, for
, imply that conditions (L1)-(L3) of Proposition 3.1 hold true for
and
for
. This verification is demonstrated in subsections A.1, A.2and A.3 for (L1), (L2), and (L3) respectively.
During this verification we point to the ei that is at smallest distance from z∈ℝN by using the pointer . This pointer is of specific use if
such that
; in such case
.
Remark A: [Citation27] also maps (Equation1.1(1.1)
(1.1) )-(Equation1.2
(1.2)
(1.2) ) on (Equation3.1
(3.1)
(3.1) ) in a way similar as done above, and subsequently verifies that conditions imposed on (Equation1.1
(1.1)
(1.1) )-(Equation1.2
(1.2)
(1.2) ) imply those imposed on (Equation3.1
(3.1)
(3.1) ) for the existence of a pathwise unique solution. In doing so, there are two differences. The first difference is that [Citation27; Appendix B] restricts the mapping on (Equation3.1
(3.1)
(3.1) ) to
, which means
This restricted mapping makes the verification of growth and Lipschitz conditions simpler, though also requires λij(x) to be Lipschitz. The second difference is that [Citation27, Appendix A] assumes that
in (Equation3.1
(3.1)
(3.1) ) is bounded; this asks
also to be bounded.
A.1. Verification that (C0), (C1) and (C3) imply condition (L1) in Proposition 3.1
For we get:
where ℓG is the constant in (C1), and the last term follows from (2.1b,c) of (C0). Thanks to (2.1d) of (C0) we can make use of (Equation2.2
(2.2)
(2.2) ), which implies that there is only one non-zero term in the summation over j. Hence the summation can be moved outside
. Due to Fubini’s theorem this summation can also be moved outside the integral, i.e.
This implies: Hence (L2) is satisfied by setting
where ℓG and ℓφ are the constants in (C1) and (C3).
A.2. Verification that (C1) and (C2) imply condition (L2) in Proposition 3.1
For ,
we define:
Evaluation of for
yields:
Evaluation of for
yields:
Taking the two bounds together yields for :
Hence with ,
we get:
Hence, (L2) is satisfied by setting .
A.3. Verification that (C0) implies (L3) in Proposition 3.1
Using we get:
Due to equations (2.1b,c) of (C0) we know: .
Hence: .
By using (2.1a) and (2.1d) from (C0) we get:
Due to (C0) we also know for all i,j,x; hence
The latter verifies (L3) by setting cJ = NCλ.