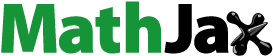
Abstract
A growing research body shows that victimization increases victims’ fear of crime. Typically, this research estimates average effects, which may conceal that people respond differently to victimization or that ecological contexts shape the experience of crime. The current study is among the few that attempt to uncover potential heterogeneities in victimization effects by ecological contexts. It explores whether the victims’ neighborhood conditions (levels of crime, disorder, and concentrated disadvantage) moderate the impact of violent victimization close to home on fear of crime. For this purpose, longitudinal multilevel models were estimated using data from a two-wave panel survey of about 3300 adult respondents nested in 140 neighborhoods in two large German cities. The results suggest that individuals from more disadvantaged neighborhoods tend to increase their fear of crime more strongly after victimization than those from more advantaged areas. For the other neighborhood conditions, victimization effects were estimated to be more homogenous.
Introduction
Scholars have studied the causes and consequences of fear of crime for individuals and communities for decades (see Hale, Citation1996; Lee & Mythen, Citation2018). For obvious reasons, the victimization perspective from early on has identified becoming the victim of crime as a major cause of fear of crime (e.g. Garofalo, Citation1979; Janoff-Bulman & Frieze, Citation1983; Skogan, Citation1987). Over time, an abundance of empirical evidence has been accumulated showing that victimization indeed increases fear of crime (even though other factors than crime also have been found to foster fear; for a meta-analysis, see Collins, Citation2016). However, most of this research was based on data from cross-sectional designs, which typically cannot account for issues of unobserved individual heterogeneity and causal time order, questioning the internal validity of the findings (for critique, see Ditton et al., Citation2003; Mahuteau & Zhu, Citation2016; Skogan, Citation1987). Only in recent years have more prospective panel studies emerged that address these internal validity threats by surveying the same individuals of a general population—including both victims and non-victims—repeatedly over time. Starting with the seminal study of Skogan (Citation1987), this work produced more trustworthy evidence that victimization experiences increased fear of crime on average (e.g. Janssen et al., Citation2021; Mahuteau & Zhu, Citation2016; Russo & Roccato, Citation2010; Salm & Vollaard, Citation2021; for more mixed results, however, see Braakmann, Citation2012; Bunch et al., Citation2014).
Despite this undeniable progress, scholars have emphasized the need to look also at the heterogeneity underlying these victimization effects. People do not respond uniformly to criminal victimization—they cope differently depending on resilience-related psychological and social characteristics (e.g. Agnew, Citation1985; Denkers & Winkel, Citation1998; Skogan, Citation1987). Following the suggestions of these scholars, a growing empirical literature explores the factors that may account for the heterogeneity of victimization effects. Its findings imply that the impact of victimization on fear of crime may differ depending on, amongst others, the victim’s age, gender, ethnicity, previous victimization experience, or victimization propensity (e.g. Borooah & Carcach, Citation1997; Karakus et al., Citation2010; Köber, Citation2018; Logan & Walker, Citation2021; Mahuteau & Zhu, Citation2016; May, Citation2001; Russo & Roccato, Citation2010; Scherg & Ejrnæs, Citation2022; Skogan, Citation1987; Turanovic, Citation2019; Weitzer & Kubrin, Citation2004).
While most of this research has concentrated on individual victim characteristics as moderators, less research has investigated to what extent victimization effects differ by social contexts on various spatial levels, such as countries (e.g. Abbott & McGrath, Citation2017; Chon & Wilson, Citation2016; Ejrnæs & Scherg, Citation2020), cities (e.g. Bennett & Flavin, Citation1994; Stein, Citation2014; Valente et al., Citation2022), neighborhoods (e.g. Brunton-Smith & Sturgis, Citation2011; Jing et al., Citation2021; Wilcox Rountree & Land, Citation1996a), or schools (e.g. Baek et al., Citation2019; Coyle et al., Citation2022; Kollerová & Smolík, Citation2016; Swartz et al., Citation2011). Research on neighborhoods, which have been found to be powerful contextual determinants of fear of crime in general (e.g. Brunton-Smith & Sturgis, Citation2011; Wilcox Rountree & Land, Citation1996a, Citation1996b), has typically studied whether neighborhood conditions, such as crime rates or levels of disorder moderate the impact of victimization on fear. Overall, this relatively sparse literature has produced mixed findings: While several studies have found that victimization has a larger impact on fear in more disordered or crime-ridden neighborhoods (e.g. Borooah & Carcach, Citation1997; Jing et al., Citation2021; Weitzer & Kubrin, Citation2004), others have reported more equivocal findings (e.g. Abdullah et al., Citation2015; Brunton-Smith & Sturgis, Citation2011; Roccato et al., Citation2011; Wilcox Rountree & Land, Citation1996a, Citation1996b).
However, this extant research on heterogenous victimization effects on fear of crime is limited in various ways. First, as with the general literature on the victimization–fear link, most studies used cross-sectional designs, and their results thus typically suffer from low internal validity. As argued above, panel studies with a control (non-victim) group are necessary to explore causal (victimization) effects more adequately (see Gangl, Citation2022; Shadish et al., Citation2002; Skogan, Citation1987). To our knowledge, however, so far, no study has investigated the moderating role of neighborhood conditions on the victimization–fear link longitudinally. Second, most studies measure neighborhood conditions by aggregating individual survey responses (e.g. Box et al., Citation1988; Jing et al., Citation2021; Roccato et al., Citation2011; for a notable exception, see Brunton-Smith & Sturgis, Citation2011). The aggregation of individual perceptual measures may be affected by sample bias, endogeneity issues, and correlated measurement errors, as is well-known from neighborhood and school effects research (Brunton-Smith & Sturgis, Citation2011; Duncan & Raudenbush, Citation1999; Sampson et al., Citation1997). Third, most research on the moderating force of neighborhoods lacks theoretical depth (for an earlier critique, see Wilcox Rountree & Land, Citation1996a). Typically, studies discuss no (e.g. Brunton-Smith & Sturgis, Citation2011; Wilcox Rountree & Land, Citation1996a, Citation1996b) or only one (e.g. Jing et al., Citation2021; Roccato et al., Citation2011) theoretical perspective on how and why victimization effects may differ depending on neighborhood conditions, although various theoretically reasonable (opposing) mechanisms exist.
The current study aims to overcome some limitations of previous research by investigating heterogeneous victimization effects on fear of crime using two-wave panel survey data from 140 neighborhoods in two German cities. It supplements previous work with the same data that already found that victimization—and here especially violent rather than property crime victimization—increases fear of crime on average (Janssen et al., Citation2021). The current paper extends this work and goes beyond the analysis of average effects in the following ways. First, it introduces four theoretical perspectives that differ in their explanation of how and why victimization effects on fear of crime may vary by neighborhood contexts. Second, it links panel survey data from a general population sample with neighborhood-level external information to test two opposing hypotheses that can be derived from the theoretical perspectives. In the analyses, we regard fear of crime as an umbrella term for a multidimensional concept spanning cognitive, emotional, and behavioral responses to subjectively perceived threats which calls for a measurement reflecting this multidimensionality (Hart et al., Citation2022; Jackson, Citation2006; Rader et al., Citation2007).
Neighborhood-Specific Victimization Effects
To explain why the fear of crime may change differentially after a victimization experience depending on the residential neighborhood context, we draw on four theoretical perspectives: (1) the cumulative disadvantage thesis, (2) the disorder highlighting thesis, (3) the disadvantage saturation thesis, and (4) the resilience thesis. The former two theses predict that victimization has a stronger impact on fear for individuals living in more crime-ridden, disadvantaged, or disorderly neighborhoods. The latter two theses, in contrast, allow for the opposite prediction that victimization has a stronger impact on those living in less crime-ridden, disadvantaged, or disordered areas. Empirically, our data only allow us to test the two broader predictions but not the more detailed underlying mechanisms. Accordingly, we formalize the two competing predictions as hypotheses to be tested in the remainder of the article and leave it to future research to explore the mechanisms producing the predictions.
The cumulative disadvantage thesis posits that experiences of adversity and disadvantage have a kind of snowballing effect (see Scherg & Ejrnæs, Citation2022; Turanovic, Citation2019). According to this perspective, adverse events beget adverse events, and the resulting permanent exposure to adversity undermines psychological and social resources that affected individuals may otherwise employ to mitigate the detrimental consequences of further negative experiences (Aquino et al., Citation2022; Carr & Umberson, Citation2013; Hobfoll, Citation1989; Turner & Lloyd, Citation1995; Winkel et al., Citation2003). As a result, individuals who face such continuous stress due to their exposure to ecological disadvantage can cope less efficiently with a victimization experience and suffer stronger increases of fear than individuals residing in better-off neighborhoods who do not (or less frequently) have to endure such adversity and thus have more resources available to shield against the detrimental effect.
Neighborhoods may contribute to cumulative disadvantage in multiple ways. A plethora of research has produced evidence for the adverse effects of neighborhood conditions on many aspects of well-being and life chances, ranging from social cohesion and social capital (Dinesen et al., Citation2020; Sampson et al., Citation1997), educational achievement and labor market success (Chetty et al., Citation2016; Sharkey & Elwert, Citation2011), to mental health (Klijs et al., Citation2016), and physical health and mortality (Meijer et al., Citation2012; Schüle & Bolte, Citation2015). Concentrated poverty, mostly in combination with the segregation of ethnic minorities, has often been viewed as the root cause of adverse neighborhood outcomes, either directly or mediated by poor housing, disorder, crime, or related adverse features of urban life, which are deemed as harsh and stressful living conditions (Galster & Sharkey, Citation2017; Pepper & Nettle, Citation2017; Sharkey & Faber, Citation2014). Scholars have proposed various social and psychological mechanisms to account for the negative effects of concentrated disadvantages, such as alienation, feelings of powerlessness, lack of trust, social contagion and peer influences, or physical environment (de Courson et al., Citation2023; Leventhal & Brooks-Gunn, Citation2000; Ross et al., Citation2001; Sampson, Citation2022). Indirect victimization, the knowledge of victim experiences by others through personal networks, which often include people living in the vicinity, may be one mechanism that has been shown to increase fear of crime (Drakulich, Citation2015; Keel et al., Citation2022; Russo et al., Citation2013; Xie & Mcdowall, Citation2008). In sum, residents of disadvantaged neighborhoods may be exposed to more adverse experiences and harsher living conditions than residents of other urban neighborhoods, and some scholars assume exponential or threshold effects of adverse neighborhood conditions (Galster, Citation2014). According to the cumulative disadvantage thesis, these continuous adversities will deplete the coping resources of individuals, who will then have a more difficult time dealing with victimization than individuals from more advantaged neighborhoods.
Some studies found support for the assumption that adverse neighborhood conditions reinforce the effect of victimization on fear. However, all these studies were based on cross-sectional data, whose findings may have questionable internal validity. Of the cross-sectional studies using officially recorded crime rates, Weitzer and Kubrin (Citation2004) suggested that property victimization had only a fear-inducing impact among those living in districts with higher crime rates, and Brunton-Smith and Sturgis (Citation2011) found that the effect of a single (but not multiple) violent victimization on fear was reinforced in neighborhoods with more recorded crime.
More studies investigated the impact of perceived neighborhood crime and disorder on the effects of victimization on fear (e.g. Box et al., Citation1988; Jing et al., Citation2021; Roccato et al., Citation2011), often showing that the association between victimization and fear increases with higher levels of perceived crime and disorder. However, this finding may reflect that more fearful respondents also perceive and report more crime and disorder independent of objective levels of crime and disorder, as has been shown in the seminal work by Ralph Taylor and colleagues (Perkins & Taylor, Citation2002; cf. O’Brien et al., Citation2019). In a previous analysis of the survey data used in this paper, Janssen et al. (Citation2022) found that violent victimization was by far the strongest predictor of the disorder perception bias in a model controlling for objectively measured disorder. Thus, a reinforcing effect of perceived disorder on the association between victimization and fear is likely to be an artifact of correlated measurement errors and should not be interpreted as a substantive finding.
Yet, exactly this research finding could be interpreted in favor of a second theoretical perspective, which we will call the disorder highlighting thesis. This thesis focuses on the symbolic dimensions of neighborhood disorder and argues that experiences of adversity may work as a highlighter of problematic features of residential contexts (see Roccato et al., Citation2011). Neighborhoods are a particularly salient context for the disorder highlighting thesis given that they constitute people’s immediate residential environments. According to this perspective, individuals confronted with a harmful experience will subsequently scan their social environment for signs of disorder and use those as warning signs to infer the likelihood of further critical incidents, especially if the experience was made close to their homes. If persons live in crime-ridden, disadvantaged, and disordered neighborhoods, they are more likely to find environmental cues to support and exacerbate their risk perceptions. In better-off and less disordered contexts, in contrast, victimization experiences will be matched less often with corresponding environmental cues. Consequently, a person will be more likely to dismiss the event as unfortunate and unlikely to occur again (Agnew, Citation1985); hence, an increase in fear of crime is less likely than in crime-ridden, disadvantaged, and disordered neighborhoods. As noted above, disorder perceptions are highly subjective and closely linked to feelings of insecurity (Perkins & Taylor, Citation2002; Ward et al., Citation2017), and victimization experiences may be one of the factors fueling a sense of disorder (LaGrange et al., Citation1992). However, in contrast to studies relying solely on survey-based measures of subjective perceptions of disorder, we maintain that independently measured data on disorder is necessary to substantiate this second hypothesis.
Both the cumulative disadvantage thesis and the disorder highlighting thesis lead to the following hypothesis:
H1: Victimization experiences will increase fear of crime more strongly if the victim lives (and is victimized) in a neighborhood characterized by more crime, disadvantage, and disorder.
Finally, the resilience thesis takes this reasoning one step further and posits that individuals develop coping skills when they face adverse experiences and are thus, in the long run, better able to deal with future adversities (see Scherg & Ejrnæs, Citation2022; Seery et al., Citation2010, Citation2013; Updegraff & Taylor, Citation2000; Winkel et al., Citation2003). The psychological concept of posttraumatic growth investigates how individuals develop positive coping strategies through adverse experiences (Calhoun & Tedeschi, Citation2006; Linley & Joseph, Citation2005; Roepke & Seligman, Citation2015). This concept can be applied to adaptation processes in the face of repeated observed or experienced adversities in disadvantaged neighborhoods. Ethnographic research, furthermore, has stressed the importance of becoming “streetwise” to successfully navigate the risks of living in disadvantaged neighborhoods (Anderson, Citation1990; Jones, Citation2009). These perspectives suggest that resilience may grow stronger in neighborhoods that confront their residents with adverse experiences. Individuals, in contrast, who are rarely exposed to difficult circumstances will not develop the skills to encounter adversities efficiently. Criminal victimization will thus impact residents of crime-ridden, disadvantaged, and disordered neighborhoods, who are rich in negative experiences, less compared to others residing in better-off neighborhoods who lack such experience.
Both the disadvantage saturation thesis and the resilience thesis lead to the following hypothesis:
H2: Victimization experiences will increase fear of crime more strongly if the victim lives (and is victimized) in a neighborhood characterized by less crime, disadvantage, and disorder.
Methods
Data
The data are from a two-wave panel survey on crime and insecurity in urban neighborhoods in Cologne and Essen, two large cities in North Rhine-Westphalia (Germany) with populations of one million and slightly more than half a million, respectively. Both cities rank high nationally in terms of police-recorded violent and property crime rates but have very low homicide rates (0.6 resp. 0.5 per 100.000), as does Germany as a whole. In a two-stage cluster design, 140 neighborhoods were randomly drawn with an oversampling of the most disadvantaged areas. Neighborhoods are small administrative units with a mean area size of 0.56 square kilometers (SD = 0.55) and a mean population of 2900 (SD = 2100). Within these areas, respondents aged between 25 and 89 years were randomly drawn from a population register, with an oversampling of 60–89 old persons. This restricted age range reflects the purpose of the original study, which focused on the security perceptions of older adults and excluded younger adults many of whom are still in tertiary education and whose residential patterns are more unstable. Because young adults relocate more frequently, neighborhood conditions may be less salient for them. Based on previous analyses of the same survey data focusing on age heterogeneity in victimization effects on fear (Köber, Citation2018, p. 107), it can indeed be assumed that younger age groups are less susceptible to adverse effects than older age groups (within the range of 25 to 89 years). If this finding can be extrapolated to young adults below the age of 25 and had we included this age group in our analyses, we would expect on average slightly weaker effect estimates.
The first wave (T1) was conducted in spring 2014 and the second wave (T2) 18 months later in autumn 2015, both using a mail survey mode and self-administered paper-and-pencil questionnaires. The response rate at T1 was 41.1%. Using information from the official population register on the citizenship of both respondents and non-respondents, the response rate of minority citizens was computed as roughly half of that of German citizens, indicating a strong response bias (Gerstner & Oberwittler, Citation2016). However, the neighborhood-level correlation between register- and survey-based percentages of minority citizens in T1 was r = 0.87 in Cologne and 0.78 in Essen, and the correlation between register- and survey-based percentages of welfare recipients was 0.88 in Cologne and 0.90 in Essen, hinting at a satisfactory ecological quality of the survey sample.
Of n = 6565 participants in T1, n = 3746 (57%) participated also in T2. The largest source of panel attrition was due to respondents who indicated in T1 that they did not want to be contacted again in response to a consent question proscribed by data protection rules.
One of the problems of a panel postal survey is the possibility that different persons from the same household may complete the questionnaire at different measurement occasions (even though the participants were personally addressed), precluding the analysis of individual changes over time. To exclude such cases across waves, we checked for the identity of gender and year of birth across waves and found 345 non-identical cases, reducing the sample to 3401 valid cases for the panel analysis.
In our models, we included only respondents with full information on all assessed variables, leaving 3325 respondents eligible for our analyses.Footnote1 An additional indicator category was included for missing values on categorical variables. Comparing our final sample (n = 3325) to the original T1 sample (n = 6565), we found signs of selective attrition (Janssen & Gerstner, Citation2016; see also Online Supplementary Material, Table S22). The final sample included fewer individuals with an immigrant background and with lower socio-economic status, and these respondents were also less likely to reside in disadvantaged neighborhoods. The final sample scored slightly lower on feelings of neighborhood unsafety, worry about crime, and avoidance behavior, and scored slightly higher on positive affect, generalized trust, neighborhood satisfaction, life satisfaction, contact with neighbors, self-rated health, and going out. Thus, sample attrition resulted in a disproportional loss of respondents with lower social status, lower well-being, and higher fear of crime. However, the final sample did not differ in terms of victimization rate compared to the larger T1 sample.
Measures
In this section, we introduce the measurements of the current study. For a full list of the descriptive statistics of these measures, see Table S1 in the Online Supplementary Material.
DV: Fear of Crime Indicators
Since its beginnings in the 1960s and 1970s, survey-based research on fear of crime has evolved from relying on rather crude single items to employing multi-item and multidimensional measurements of fear (Hart et al., Citation2022). Fear of crime is commonly conceptualized as a threat perception that consists of cognitive, affective (or emotional), and behavioral dimensions (Chataway & Bourke, Citation2020; Erčulj, Citation2022; Gabriel & Greve, Citation2003; Jackson, Citation2006; Rader et al., Citation2007). The affective component has a central position in conceptual models, which assume that cognitive risk perceptions are combined with appraisals of one’s coping resources and vulnerabilities “to form an overall appraisal of threat that then shapes worry” (Jackson, Citation2004, p. 949), which subsequently influences behaviors intended to reduce victimization risks, such as avoiding scary spaces (Erčulj, Citation2022). Consequently, the affective dimension is often measured by asking respondents how much or how often they “worry” about becoming the victim of specific crimes, and this question has become a standard instrument in victimization surveys, such as the British Crime Survey (now Crime Survey for England and Wales) since the 1990s (Farrall et al., Citation1997; Hough, Citation2004; Jackson, Citation2005). Jackson (Citation2005) and Erčulj (Citation2022) have used confirmatory factor analyses to demonstrate the distinctiveness of the cognitive, affective, and behavioral dimensions of fear crime.
An important stream of research has supported the notion that fear of crime, in particular in urban contexts, is fundamentally rooted in place (Brunton-Smith & Jackson, Citation2012). Fear levels vary distinctively between urban neighborhoods, reflecting subjective perceptions of local crime and disorder but also wider concerns about “the social and moral make-up of one’s community” (cf., Girling et al., Citation2000; Jackson, Citation2004, p. 946). Questions about insecurity feelings in local areas and about avoidance behavior, in particular, have shown large neighborhood variations (Brunton-Smith & Sturgis, Citation2011; Lorenc et al., Citation2012; Oberwittler, Citation2008; Rader et al., Citation2007).
We used three familiar survey scales, two of which (feelings of unsafety in the neighborhood and worry about crime) tap into the affective dimension and one (avoidance behavior) taps into the behavioral dimension of fear of crime. A fourth scale measuring cognitive fear was not included in the questionnaire of the second survey wave and therefore cannot be considered. Exploratory factor analyses of all items suggested that a 3-factor solution explains the relation between the items well (see Online Supplementary Material, Table S23). The results indicated that feelings of unsafety in the neighborhood are more closely related to avoidance behavior than to worry about crime, probably because both scales address the ecological dimension of fear. This view is supported by the high shares of between-neighborhood variance of both feelings of unsafety and avoidance behavior, in contrast to worry about crime, which has a lower share of neighborhood-level variance (see Online Supplementary Material, Table S25).
Feelings of unsafety in the neighborhood (in short: “feel unsafe”) were measured by two items asking the respondents “How safe do you feel—or would you feel—if you” (a) “walk alone in your area after dark?”, and (b) “walk alone in your area during day time?”. Answering categories ranged from 0 (very safe) to 3 (very unsafe); the polychoric correlation between the two items was r = .79.
Worry about crime (in short: “worry”) was measured by asking respondents to indicate on a scale ranging from 0 (not at all worried) to 3 (very worried) how worried they were about: “having your home broken into and something stolen?”, “being mugged and robbed?”, “being physically attacked by strangers?”, “being victim of a fraud?”. Cronbach’s alpha (measured at T1, as for the following scales) of this scale was .83. Both questions on unsafety and worry are standard measurements of affective fear of crime adopted from the Crime Survey for England and Wales (Office For National Statistics, Citation2022).
Avoidance behavior (in short: “avoidance”) is a summary construct adopted from Lüdemann (Citation2006). It indicates how many different measures to protect themselves against crime respondents have taken during the last 12 months (response categories: 0 = no; 1 = yes): “avoid certain streets or places in my neighborhood during daytime”, “avoid certain streets or places in my neighborhood at dark”, “take the car or a taxi rather than walk in my neighborhood at dark”, “leave the house at dark only in company”, and “avoid public transport at dark”. Cronbach’s alpha is .76.Footnote2
All outcome scales have been z-standardized (mean of 0 and standard deviation of 1).
IV: Violent Victimization Experiences
The survey asked about personal victimization experiences both in the respondents’ residential neighborhoods and elsewhere during the last 2 years (T1) and since the first wave, i.e. during the last 18 months (T2), respectively. Because we are interested in the moderation effects of neighborhoods on the victimization–fear link, we concentrated on violent victimization experienced in the respondents’ own neighborhoods using three categorical items indicating no, single, or multiple victimizations for each occasion: “I was beaten and injured or physically assaulted in any other kind”, “somebody harassed or threatened me”, “I was sexually assaulted”. These items were combined into two binary variables indicating whether respondents had experienced any violent victimization before T1 (0 = no, 1 = yes) or between T1 and T2 (0 = no, 1 = yes) in their neighborhood. For analysis of the victimization effects, we finally generated a categorical variable from these two variables, assigning respondents to one of the following four violent victimization groups: no victim (n = 2504; 75.3%), T1-only victim (n = 307; 9.2%), T2-only victim (n = 238; 7.2%), T1+T2 victim (n = 276; 8.3%).
We focus on victimization in the victims’ neighborhood for two reasons. On the one hand, research suggests that victimizations in locations closer to home have more detrimental effects on victims than victimizations further away (Dugan, Citation1999; Janssen et al., Citation2021; Plassa et al., Citation2023). On the other hand, it is more consistent to study the moderation effects of neighborhood conditions on the victimization–fear link with a victimization measure referring to the neighborhood area. A methodological issue that arises when focusing on victimization close to home is how to deal with individuals who reported victimization outside their neighborhood. Should they be excluded completely, or included in the non-victim group? For our main analysis, we choose a conservative approach by including these individuals as non-victims. Yet, since victimization outside of one’s neighborhood will probably still increase fear of crime, this approach might reduce our effect estimates. To guard against a potential downward bias, we conducted sensitivity analyses, in which we excluded these individuals from modeling. The effect estimates of these robustness analyses are basically indistinguishable from our main results (see Online Supplementary Material, Tables S7–S10). Similar findings suggest that it is primarily victimization in the neighborhood that drives fear of crime and that victimization further away from home has less impact on fear (see Dugan, Citation1999; Janssen et al., Citation2021; Plassa et al., Citation2023).
Moderators: Neighborhood Characteristics
For the measurement of neighborhood conditions, we used register-based socio-demographic data, police-recorded crime data, and systematic social observations of social and physical disorder, thus avoiding the problem of correlated measurement errors, which has plagued many studies based on respondents’ perceptions aggregated to the neighborhood level (O’Brien et al., Citation2019).
Concentrated disadvantage is a composite index of two continuous neighborhood variables: the percentage of welfare recipients and a diversity index of nine nationality groups defined by citizenship. In the absence of standard sociodemographic variables, such as ethnicity, educational status, or income, these register-based information are commonly used in neighborhood research in Germany (Schaeffer, Citation2013). The Pearson correlation of r = .78 indicates a very close association between poverty and diversity in neighborhoods, which is typical for ethnically and socially segregated cities in Germany. As an illustration of the level of concentrated disadvantage in the two cities, the share of welfare-dependent residents was 35% at the top decile of the most disadvantaged neighborhoods.
Crime rate is the number of police-recorded crimes per 100,000 inhabitants, including various violent and property offensesFootnote3 averaged for the years 2012–2014.
The systematic social observation (SSO) of physical and social disorder was carried out by trained undergraduate students equipped with GPS-enabled tablets in Spring and Summer 2015 during afternoon and evening daylight hours in all 140 neighborhoods, randomly selecting about 40% of face-blocks (one side of a street segment). Two different observers went to each neighborhood independently to reduce bias. For each face block, the observers assessed the frequency of 17 different types of physical and 17 different types of social incivilities, in most cases by counting. The list of incivilities was based on previous studies (Häfele, Citation2013; Sampson & Raudenbush, Citation1999) and on pretesting. The items were summed to create an overall index of incivilities, standardized by the length of observed face-blocks and log-transformed to account for the highly skewed distribution (see more detailed information in Janssen et al., Citation2022).
Other Covariates
As covariates, we included a rich list of individual-level variables that may account for potential confounding. Next to property crime victimization experiences,Footnote4 this list encompasses various socio-demographic and socio-economic indicators (age, gender, migration background, education level, employment status, marital status, and subjective income), and negative life events (financial loss, severe illness, and partner loss). While the socio-economic and socio-demographic indicators were all measured at T1, the data on negative life events came from T2, and property victimization was operationalized to bridge both T1 and T2 in line with the violent victimization measure.
Analytical Procedure
To estimate whether neighborhood characteristics moderate the causal effects of victimization on the various indicators of fear of crime (the outcomes), we proceeded as follows. First, we defined our causal effects (CEs) as the averageFootnote5 differences in the changes in the outcomes (Ys) from T1 to T2 comparing those individuals who reported a victimization experience between the panel waves (VictimT2 = 1) to those who did not (VictimT2 = 0). That is, we assumed that differential changes in an outcome can be attributed to the victimization experience. Behind this assumption is the idea that without the victimization experience, the change would have been the same in the victimized and non-victimized groups (the so-called “common [parallel] trend assumption”; see Gangl, Citation2022).
Since we acknowledge that victimization effects may differ depending on whether a person was already a victim before T1 (i.e. whether a person was re-victimized; see Russo & Roccato, Citation2010; Scherg & Ejrnæs, Citation2022), we then distinguished two types of causal effects. The first group of effects identifies the impact victimization between T1 and T2 had on the outcomes for individuals who had not been victimized before T1 (VictimT1 = 0). On the other hand, the second group of effects identifies the impact on individuals who had already been victimized before T1 (VictimT1 = 1).
Our analysis differentiates these causal effects even further given that we want to explore whether victimization effects differ across neighborhoods. To do this, we first calculated how victimization influenced individuals living in areas with different scores (e.g. at the 0.05 percentile, P0.05, or the 0.95 percentile, P0.95) along the dimension of a specific neighborhood characteristic (NC; e.g. concentrated disadvantage).
Finally, we compared the neighborhood-specific effects to test our moderation hypotheses that the victimization effects differ depending on neighborhood characteristics. To do this, we computed the (second) differences in the causal effect estimates for individuals with the same T1 victimization history but different values on neighborhood characteristics.
To calculate the aforementioned causal effects and their (second) differences, we estimated linear longitudinal multilevel models within a random effect modeling framework (Bell & Jones, Citation2015; Hoffman, Citation2015). This modeling framework considers the nested structure of our observations, which are occasions (level 1) within individuals (level 2) within neighborhoods (level 3). It has the advantage of accounting more accurately for the uncertainty in our estimates when modeling the changes in our outcomes over time. Within these models, we also controlled for other potential sources of confounding by including various other covariates as additional predictors. Overall, our analytical approach resembles methods discussed as difference-in-difference estimation (Gangl, Citation2022). Within these methods, differences (or changes) in outcome variables over time are compared between treatment (here: victims) and control (here: non-victim) groups. The random effect models were estimated with the mixed command in Stata 17.0 using restricted maximum likelihood estimation (StataCorp, Citation2021).
Given the complexity of our models, below we show a conceptual equation that lists the categories of predictors for the outcome ytij at time point t for individual i in neighborhood j as
Within this model specification, ytij refers to the respective outcome studied (e.g. worry about crime) and Wavetij is the binary indicator for panel wave (0 = T1, 1 = T2). All individual-level predictors were centered at their neighborhood means (i.e. cwc = centered within-context) so as to estimate purely within-neighborhood fixed effects (see Yaremych et al., Citation2023). Victim_status_cwcij is a vector of six binary predictors, indicating whether a person experienced a violent victimization (3 dummies) or property victimization (3 dummies) only before T1, only between T1 and T2, or both before T1 and between T1 and T2 (with the reference categories of no violent victimization or no property victimization, respectively), and NCj is a vector of the three studied time-stable neighborhood characteristics (e.g. concentrated disadvantage). Next to these key variables, the model also included Life_events_t2_cwcij as a vector of three other negative life events (e.g. a financial loss) that occurred between T1 and T2, as well as X_cwcij as a vector of seven respondent characteristics measured at T1 (e.g. gender).
Lastly, as warranted by the sampling design (and following Hoffman, Citation2015, ch. 11), the model includes a complex heterogeneous variance structure that is multivariate by wave across both level-2 individuals and level-3 neighborhoods, which allows the intraclass correlation of individuals from the same neighborhoods to differ by wave. To do so, the model included a separate level-3 random intercept for neighborhood mean differences at T1 and at T2, a single level-2 random intercept for individual mean differences, and separate level-1 residuals for the remaining outcome deviations at T1 and at T2. Not shown in the model formula but also estimated was the covariance between the level-3 random intercepts.
Our central variable for calculating causal victimization effects is the binary Wavetij variable, which identifies the change in the studied outcome between T1 and T2. A complex set of interactions is necessary to allow this change to differ between individuals who vary in their victimization status (Victim_status_cwcij) and in their neighborhood characteristics (NCj). This specification includes the three-way-interaction term (Wavetij*Victim_status_cwcij*NCj) along with all lower-level interactions and main effects. Only by including all of these terms is it possible to allow the outcomes to change freely over time within all of the various subgroups (with some restriction in terms of the linear nature of the moderation by the neighborhood characteristics; see Hainmueller et al., Citation2019).
To infer all the neighborhood-specific causal estimates and their differences from the pure regression estimates, it is necessary to calculate average marginal effects (AMEs) from the model (see Mize 2019).Footnote6 In our case, the AMEs depict average changes in the outcomes from T1 to T2 calculated across each observation in a subsample (e.g. among T1+T2 victims). In the current study, differences in the AMEs between individuals with and without a T2 victimization mirror our causal effects (e.g. CE0a, CE0b, …). We estimated these causal effects for particular percentiles (P0.05, P0.5, P0.95) of the moderating neighborhood conditions (see and ) and more continuously over the whole distribution of the neighborhood dimensions (see ). The neighborhood-specific differences of the causal effects (e.g. CE0b–0a) finally depict the actual moderation effects—that is, they reflect whether victims with different scores on a neighborhood dimension differ in how strongly they are affected by victimization. For all main and moderation effects, we tested whether they were significantly different from null using an alpha level of 0.05 (depicted by bolded numbers in the tables).
Figure 1. Causal effect of violent victimization between T1 and T2 on (a) feeling unsafe and (b) worry about crime conditional on neighborhood concentrated disadvantage for individuals who already reported violent victimization before T1.
Note: The black lines and their gray ribbons reflect the main models’ effect estimates and 95% confidence intervals. The error bars depict the effect estimates and confidence intervals of sensitivity analyses, in which neighborhood concentrated disadvantage is split into quintiles (the x-axis values of the error bars reflect the mean in the specific quintile groups). The density plots at the bottom depict the distribution of concentrated disadvantage among T1-only victims (dashed line; the “control” group) and T1+T2 victims (solid line; the “treatment” group). The y-axis shown only reflects the values of the conditional victimization effects, while the plot does not include an additional y-axis for the density distribution of concentrated disadvantage.
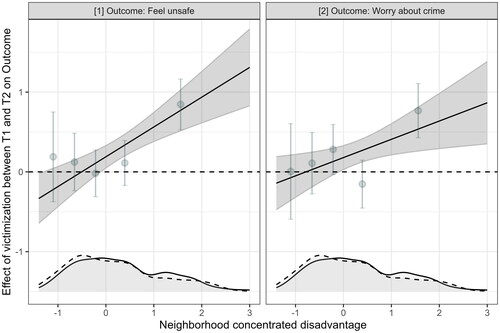
Table 2. Causal effects of T2 violent victimization by neighborhood concentrated disadvantage.
Table 4. Causal effects of T2 violent victimization by neighborhood crime rate.
Table 5. Causal effects of T2 violent victimization by neighborhood disorder.
Results
In line with findings previously obtained with these data (Janssen et al., Citation2021), the current analysis indicates that violent victimization between T1 and T2 had a fear-inducing impact on average for all three fear of crime measures. However, this impact depended to some degree on whether a person reported having been victimized before (see ). For all victims, violent victimization between T1 and T2 substantially increased feelings of being unsafe (CET2-only: 0.22; CET1+T2: 0.28) and worry about crime (CET2-only: 0.18; CET1+T2: 0.24). For example, re-victimization between T1 and T2 was estimated to increase the change in feeling unsafe from T1 to T2 by 0.28 standard deviations (all outcomes are z-standardized). Behavioral effects (i.e. on avoidance), instead, were only found among first-time victims—the individuals who had not reported a violent victimization in the two years before T1 (CET2-only: 0.27). In contrast, re-victimization had no impact on the avoidance behavior of individuals who had already reported victimization before T1 (CET1+T2: 0.02).
Table 1. Causal effects of T2 violent victimization by victimization status.
We now turn to our key effect estimates: the neighborhood-specific causal effects and their differences. These estimates indicate that concentrated disadvantage seems to be the neighborhood condition most decisive for the victimization effects (see and ). Most (4 out of 6) of the moderation effects (i.e. the differences between the CEs) are clearly positive, indicating that victimization increased the fear of crime of individuals living in neighborhoods with higher levels of concentrated disadvantage more strongly, with the rest of the moderation estimates oscillating closely around zero.
Table 6. Summary–moderation of victimization effects by neighborhood conditions.
The strongest moderation estimates suggest that neighborhood concentrated disadvantage shapes the effects on affective fear (“feel unsafe” and “worry”), particularly among individuals who had already experienced victimization before T1 (see , lower part). For these “experienced” victims, the fear-inducing impact of violent re-victimization is estimated to be moderate to strongFootnote7 if they live in more disadvantaged neighborhoods, while it loses its detrimental effect in more advantaged areas. In particular, victimization is estimated to increase feelings of unsafety and worry about crime by about 0.88 and 0.61 standard deviations, respectively, for individuals living in neighborhoods high in concentrated disadvantage (i.e. with a score at the 95%-percentile). It had, in contrast, barely any effect on those living in the least disadvantaged neighborhoods (5%-percentile; CEfeel_unsafe: −0.27; CEworry: −0.10). These differences in the victimization effects between neighborhoods low and high in concentrated disadvantage are substantial and statistically significant (differencefeel_unsafe: 1.15; differenceworry: 0.71). These substantial moderation results are also depicted in over the whole concentrated disadvantage dimension (see black lines and surrounding gray ribbons). This figure also includes two density plots at the bottom showing the distributional overlap in concentrated disadvantage across individuals victimized at T1 and T2 (“treatment” group; solid line) and individuals only victimized at T1 (“control” group; dashed line). The plots indicate that the distributions overlap sufficiently in both groups, indicating that our effects estimates are not (or only to some small extent) based on extrapolation.
Plotting the average predicted changes in feeling unsafe and worrying about crime (see ) shows that the strong re-victimization impact among those living in highly disadvantaged neighborhoods comes about due to two processes. First, individuals from disadvantaged areas who had not been victimized again between T1 and T2 seemed to have reduced their affective fear of crime (see dashed lines, , column 2). In contrast, the fear remained relatively stable (yet on a considerably lower level) among those from more advantaged neighborhoods (see dashed lines, , column 1). Second, if individuals from disadvantaged areas had experienced a re-victimization between T1 and T2, the fear of crime increased even further (see solid lines, , column 2), while this was not the case for those from less disadvantaged neighborhoods, whose fear remained on the same level between T1 and T2 (see solid lines, , column 1). Thus, re-victimization among residents of disadvantaged neighborhoods may thwart the “recovery process” leading to reductions in fear (that otherwise seems to set in) after some time without victimization and even triggers further increases in affective fear.
Figure 2. Average predictions of (a) feeling unsafe and (b) worry about crime for individuals who reported violent victimization only at T1 or at both T1 and T2 conditional on neighborhood concentrated disadvantage.
Note: The y-axis refers to the predicted values of z-standardized outcomes (i.e. outcomes with a mean of 0 and a standard deviation of 1).

Apart from the moderation effect, which is the main focus of our analysis, the prediction plots also illuminate the strong “main effect” of neighborhood concentrated disadvantage on fear levels in general; this effect is much more pronounced than that of the other investigated neighborhood conditions (see ). On average, residents of disadvantaged neighborhoods report substantially more fear of crime (in particular, feeling unsafe in the neighborhood) than those from more advantaged areas, which is in line with previous research findings (e.g. Brunton-Smith & Sturgis, Citation2011; Camacho Doyle et al., Citation2022).
Table 3. Average marginal effects of neighborhood conditions.
We now turn to the effects of the other neighborhood characteristics: crime rates and systematically observed disorder. Compared to concentrated disadvantage, these neighborhood conditions moderate the fear-related effects of victimization less strongly or not at all (see and ). As one of the notable and statistically significant exceptions, the crime rate moderated the victimization impact on worry about crime among individuals who reported their first violent victimization between T1 and T2 (see ): Victimization increased worry only (or particularly) among those who lived in neighborhoods with a low crime rate (5%-percentile; CE: 0.53). In contrast, for residents of more crime-ridden areas (95%-percentile), a victimization experience did not increase worry (CE = −0.01). Contrasting these effect estimates indicates that, for residents of areas with low crime rates, worry about crime was substantially more affected by victimization than the worry of their counterparts residing in neighborhoods with higher crime rates (difference: −0.54).
Similar to the crime rate, neighborhood disorder had only little impact on the victimization–fear link (see ). The only statistically significant moderation effect was found for feelings of unsafety of individuals reporting violent victimization both in T1 and T2. If those individuals lived in neighborhoods with low disorder (5%-percentile), the effect of re-victimization was quite substantive (CE = 0.66). Individuals from neighborhoods with high disorder (95%-percentile), on the other hand, were not affected by a renewed violent victimization (CE = 0.01). Contrasting between the neighborhoods low and high in disorder shows that these victimization effects differ significantly (difference: −0.65).
The findings reported above all rest on the assumption that neighborhood conditions shape the victimization–fear link in a linear fashion. Since this is not necessarily the case (see Hainmueller et al., Citation2019), we conducted sensitivity analyses in which we introduced the variables representing neighborhood characteristics in a categorical fashion, using quintile groups with the lowest group as the reference category. This alternative model specification dispenses with the assumption of a linear moderation and allows for non-linear moderation effects. The results do in fact question the linearity assumption and instead suggest a threshold for the moderating effects of concentrated disadvantage. The error bars in represent the estimated effects of all quintiles of neighborhood social disadvantage and show that the fear-inducing effect of re-victimization was distinctly stronger in the highest quintile of concentrated disadvantage compared to the much smaller and non-significant effects in all four other quintiles (cf. Online Supplementary Material, Table S11). This finding could indicate that concentrated disadvantage starts to amplify the fear-related consequences of victimization only beyond a certain level (i.e. only among individuals who live in neighborhoods with a percentage of welfare recipients starting around 30%). While threshold effects of concentrated disadvantage on various outcomes have long been a focal interest of research on neighborhood effects, findings have been rather inconclusive, in particular in European countries (Crane, Citation1991; de Courson et al., Citation2023; Galster, Citation2014; Hipp & Yates, Citation2011).
For the neighborhood crime rate (but not for disorder), the alternative model specification furthermore leads to more consistent moderation effects than found in the main analysis (see Online Supplementary Material, Tables S12–S14). These effect estimates indicate that (first-time) victimization increased fear of crime substantially only among individuals living in the neighborhoods with the lowest crime rates. For residents of more crime-ridden neighborhoods, in contrast, victimization had no substantial fear-inducing impact.
Discussion
Using panel survey data from two German cities, we analyzed whether neighborhood conditions moderate the impact of (violent) victimization on three components of fear of crime. Our study adds to the scarce empirical research exploring heterogeneous victimization effects by modeling within-person changes. Focusing on the role of urban neighborhoods, this is, to our knowledge, the first longitudinal study to explore how various facets of ecological adversity (concentrated disadvantage, crime, and disorder) moderate victimization effects on fear of crime. It is also the first to examine the moderating effects of concentrated disadvantage—a neighborhood dimension overlooked by previous cross-sectional studies investigating the heterogeneous impact of neighborhoods on the victimization–fear link.Footnote8 The current study, thus, addresses some of the limitations that have plagued previous cross-sectional research.
Applying random effects modeling with various interaction terms, our analyses produced the following key findings: First, in line with previous longitudinal research (e.g. Janssen et al., Citation2021; Mahuteau & Zhu, Citation2016; Skogan, Citation1987), our estimates suggest that violent victimization increases fear of crime on average, measured by both affective and behavioral indicators. While the affective components of fear of crime (feeling unsafe, worry about crime) were similarly elevated in respondents who became either first-time victims after the first wave (T2-only victims) or else reported repeated victimization experiences (T1+T2 victims), an increase in avoidance behavior was only observed in respondents who reported their first violent victimization after T1 (T2-only victims). It seems that only “novice” victims of violence become more cautious, while more “experienced” victims do not increase their avoidance behavior further (but still become more fearful) after a re-victimization. This finding could reflect that individuals use protective behavior as a coping mechanism to deal with first-time victimization. However, if individuals become victims repeatedly, they may be frustrated about their failure to protect themselves and put little hope in even more cautious behavior (see Janoff-Bulman & Frieze, Citation1983). This differential behavioral effect of victimization depending on victimization history is a novel finding in the longitudinal research on the victimization–fear link. Before, only a few longitudinal studies had analyzed similar differential effects on affective fear. They produced mixed evidence on whether those with more or less victimization experience showed more substantial increases in fear of crime after current victimization (Russo & Roccato, Citation2010; Scherg & Ejrnæs, Citation2022).
Second, our analysis yielded mixed results regarding our key hypotheses on how neighborhood conditions moderate the fear-inducing impact of victimization. Among the neighborhood conditions, concentrated disadvantage turned out to have the strongest moderation effects on the victimization–fear link, as well as the strongest neighborhood-level association with fear. Yet, no consistent pattern of moderation effects extending to other neighborhood conditions (disorder and crime) in support of one hypothesis over the other emerged. Instead, our models indicate that the neighborhood conditions under investigation shaped the victimization–fear link in different directions or had no or only little moderating force (see for a summary).
In more detail, all significant moderation effects of concentrated disadvantage support hypothesis H1: Residents of more disadvantaged neighborhoods become more fearful of crime after violent victimization, with the strongest moderation effect for those who had already been victims before T1. In contrast, a (re-)victimization had little effect on the fear of residents of better-off neighborhoods. An alternative model specification of concentrated disadvantage as a categorial variable suggested a non-linear moderation effect, with victimization showing a distinctly stronger effect in the highest quintile of disadvantaged neighborhoods than in all other neighborhoods. We cautiously interpret this finding as a possible threshold effect of neighborhood disadvantage on the victimization–fear link; yet, considering the dearth of research in this field, more studies are needed before one can come to a definite conclusion. This also applies to the question as to which perceptional processes and social mechanisms involving the neighborhood level (such as stigma, reputation, or lack of trust and cohesion) may account for the effects (Pepper & Nettle, Citation2017; Ross et al., Citation2001; Sampson, Citation2009). Nevertheless, the relatively strong moderation effects together with the likewise strong “main effect” of concentrated disadvantage on neighborhood levels of fear lend support to the cumulative disadvantage hypothesis and tie into a large stream of research on urban inequalities and the negative consequences on the well-being of living in concentrated disadvantage (Massey, Citation2016; Sampson, Citation2012). We regard it as an important take-away of this study that concentrated disadvantage should be considered not only as a relevant predictor of fear of crime but also as a potentially strong moderator of the victimization–fear link.
In contrast to concentrated disadvantage, our main analyses indicate that neighborhood crime rates and systematically observed disorder had little impact on the victimization–fear link. Most moderation estimates were statistically insignificant (10 out of 12) and typically smaller than those for concentrated disadvantage. The few significant estimates point in the opposite direction of the moderation results obtained for the disadvantage dimension. That is, individuals from less crime-ridden or disordered neighborhoods seem to have increased their affective fear of crime more strongly than individuals from more crime-ridden or disordered neighborhoods. This lack of moderating power of the level of crime and disorder in neighborhoods aligns well with the rather mixed and often insignificant findings on these dimensions produced by previous research (e.g. Brunton-Smith & Sturgis, Citation2011; Jing et al., Citation2021; Roccato et al., Citation2011; Wilcox Rountree & Land, Citation1996a, Citation1996b), and in particular the contested role of disorder (Janssen et al., Citation2022; O’Brien et al., Citation2019). This finding contradicts the disorder highlighting hypothesis—at least with regard to independently observed signs of disorder (but not with regard to disadvantage cues)—which assumes that residents become more sensitive toward disorder after victimization experiences.
In light of these overall mixed results, we succeeded only partially in evaluating the four perspectives introduced in the theoretical section. While the results for the neighborhood concentrated disadvantage condition seem overall supportive of hypothesis H1 and thus may be read as evidence in favor of the cumulative disadvantage thesis, the results of the other neighborhood conditions provide some contradicting support and mostly suggest that victimization effects are relatively homogenous.
Despite its strength in design, the current study has some limitations. First, it is like most research on heterogenous effects hampered by relatively low statistical power (see Skogan, Citation1987). Despite a fairly large sample size and rather large variation in neighborhood conditions, our highly interactional analyses could only detect moderation effects that are at least moderate in size. Second, our sample is affected by panel attrition. This attrition could bias our effect estimates if systematically related to the key concepts of victimization, fear of crime, and neighborhood conditions (Enders, Citation2013). However, we think that a substantial bias is unlikely because T1 measures of victimization were not strongly related to attrition (see Online Supplementary Material, Table S22). Third, our victimization measure is relatively coarse, including victimizations in the last 18 months, without any information on the exact timing of the event. This rather long period could diminish our victimization (and moderation) effect estimates, as previous research has shown that the psychological consequences of victimization are often relatively short-lived (Mahuteau & Zhu, Citation2016; Russo & Roccato, Citation2010) and adaption quite imminent (Janssen et al., Citation2021). Fourth, and relatedly, we study a rather broad measure of violent victimization, including crimes as diverse as physical assault, sexual assault, and provocation or harassment. Such an inclusive measure, however, may blur the effect of victimization, which is probably more crime-specific, with more severe crimes typically having stronger effects (see Abbott & McGrath, Citation2017; Chadee et al., Citation2020; Shapland & Hall, Citation2007), which may go along with larger moderation effects. Fifth, it was repeatedly shown that the frequency of victimization in a specific period is essential for its effect size (e.g. Janssen et al., Citation2021; Skogan, Citation1987). Although our survey data provide such frequency (repeat victimization) information, we refrained from reporting the results produced with these data since they suffer (even) more severely in terms of statistical power (but see Online Supplementary Material, Tables S15–S18). Sixth, our findings for avoidance behavior might be affected by reverse causality (see Braakmann, Citation2012), because it is, other than the other two outcomes, measured retrospectively (over the last year) and thus overlaps temporally with the victimization measure (over last 18 months). Our estimates might thus reflect a mixture of the actual impact of victimization on behavior and the reverse effects of behavior on victimization. It is difficult to know in what direction such reverse causality influences our findings, given that research on the impact of avoidance behavior on victimization is sparse (e.g. Miethe et al., Citation1990; Norris & Kaniasty, Citation1992). Seventh, the parallel trend assumption of our panel models does not necessarily hold. The change (trend) in fear of crime of non-victims may not accurately mirror the (counterfactual) change of fear in victims if these would not have been victimized. If these trends indeed differ, for example, due to some unmeasured differences between victims and non-victims, our estimates will be biased (Gangl, Citation2022). Finally, the comparison of victimization effects across neighborhoods may not reflect pure contextual moderation. Rather, it may also capture that individuals with different (unmeasured) coping-related social and psychological characteristics select into different areas (Sharkey & Faber, Citation2014).
Overall, the current study, which is the first longitudinal analysis on whether and how neighborhood conditions may moderate the impact of victimization on fear of crime, produced mixed results. While individuals from more disadvantaged neighborhoods tend to increase their fear of crime more strongly after violent victimization than individuals living in more advantaged areas, with hints at a threshold effect at the top end of concentrated disadvantage, other neighborhood conditions that are often assumed to activate fear—disorder and crime—seem to have less moderation power or moderate the victimization effects in the opposite direction. More longitudinal research is needed to elucidate the processes that can explain how collective neighborhood conditions—and here especially, concentrated disadvantage—contribute to residents’ insecurity perceptions.
Supplemental Material
Download (7.1 KB)Supplemental Material
Download PDF (726.8 KB)Supplemental Material
Download MS Word (65 KB)Acknowledgment
This research was supported by the German Federal Ministry of Education and Research, Civil Security Research Programme (Grant: 13N12980). We thank the anonymous reviewers and the editors of Justice Quarterly for their helpful comments, which helped us to improve our manuscript.
Disclosure statement
No potential conflict of interest was reported by the author(s).
Notes
1 To be more precise, the number of respondents varies slightly between the models (from n = 3318 to n = 3325), depending on the missingness in the dependent variables (see Online Supplementary Material, Table S2).
2 Since the indicators are binary, we also conducted a sensitivity analysis with an IRT scale of avoidance behavior. The results are very similar to our main findings (see Online Supplementary Material, Tables S19–S21).
3 Violent offenses include assault, robbery, sexual offenses, coercion, and homicide; property offenses include burglary, property damage, theft of/from vehicles, and pickpocketing.
4 In addition to using property victimization as control variable, we also examined its effect on fear of crime in some supplementary analyses. In these analyses, property victimization had much weaker average effects and fewer significant moderation effects compared to violence (see Online Supplementary Material, Tables S3–S6).
5 E[.] refers to the expectation operator from probability theory. In this article, the expectations are average changes in the outcomes of interest between T1 and T2.
6 In the Online Supplementary Material, Table S2, we also report the “pure” regression coefficients.
7 As our outcomes are z-standardized, the causal effects have a similar meaning to Cohen’s d (Lipsey & Wilson, Citation2001). Accordingly, we call effects strong if they have a value of about 0.8, moderate if the value is about 0.5, and weak if the value is about 0.2.
8 Brunton-Smith and Sturgis (Citation2011) are exceptional in including neighborhood disadvantage indicators; however, they did not report how these indicators moderated the victimization–fear link.
References
- Abbott, J., & McGrath, S. A. (2017). The effect of victimization severity on perceived risk of victimization: Analyses using an international sample. Victims & Offenders, 12(4), 587–609. https://doi.org/10.1080/15564886.2016.1208130
- Abdullah, A., Hedayati Marzbali, M., Maghsoodi Tilaki, M. J., & Bahauddin, A. (2015). Territorial features, disorder and fear of crime in residential neighbourhoods in Malaysia: Testing for multigroup invariance. Global Crime, 16(3), 197–218. https://doi.org/10.1080/17440572.2015.1019611
- Agnew, R. S. (1985). Neutralizing the impact of crime. Criminal Justice and Behavior, 12(2), 221–239. https://doi.org/10.1177/0093854885012002005
- Anderson, E. (1990). Streetwise: Race, class, and change in an urban community. University of Chicago Press.
- Aquino, T., Brand, J. E., & Torche, F. (2022). Unequal effects of disruptive events. Sociology Compass, 16(4), e12972. https://doi.org/10.1111/soc4.12972
- Baek, H., Andreescu, V., & Rolfe, S. M. (2019). Bullying and fear of victimization: Do supportive adults in school make a difference in adolescents’ perceptions of safety? Journal of School Violence, 18(1), 92–106. https://doi.org/10.1080/15388220.2017.1387133
- Bell, A., & Jones, K. (2015). Explaining fixed effects: Random effects modeling of time-series cross-sectional and panel data. Political Science Research and Methods, 3(1), 133–153. https://doi.org/10.1017/psrm.2014.7
- Bennett, R. R., & Flavin, J. M. (1994). Determinants of fear of crime: The effect of cultural setting. Justice Quarterly, 11(3), 357–381. https://doi.org/10.1080/07418829400092311
- Borooah, V. K., & Carcach, C. A. (1997). Crime and fear: Evidence from Australia. British Journal of Criminology, 37(4), 635–657. https://doi.org/10.1093/oxfordjournals.bjc.a014202
- Box, S., Hale, C., & Andrews, G. (1988). Explaining fear of crime. The British Journal of Criminology, 28(3), 340–356. https://doi.org/10.1093/oxfordjournals.bjc.a047733
- Braakmann, N. (2012). How do individuals deal with victimization and victimization risk? Longitudinal evidence from Mexico. Journal of Economic Behavior & Organization, 84(1), 335–344. https://doi.org/10.1016/j.jebo.2012.04.001
- Brunton-Smith, I., & Jackson, J. (2012). Urban fear and its roots in place. In V. Ceccato (Ed.), The urban fabric of crime and fear (pp. 55–82). Springer. https://doi.org/10.1007/978-94-007-4210-9_3
- Brunton-Smith, I., & Sturgis, P. (2011). Do neighborhoods generate fear of crime? An empirical test using the British Crime Survey. Criminology, 49(2), 331–369. https://doi.org/10.1111/j.1745-9125.2011.00228.x
- Bunch, J., Clay-Warner, J., & Mcmahon-Howard, J. (2014). The effects of victimization on routine activities. Criminal Justice and Behavior, 41(5), 574–592. https://doi.org/10.1177/0093854813508286
- Calhoun, L. G., & Tedeschi, R. G. (2006). Handbook of posttraumatic growth: Research and practice. Lawrence Erlbaum Associates.
- Camacho Doyle, M., Gerell, M., & Andershed, H. (2022). Perceived unsafety and fear of crime: The role of violent and property crime, neighborhood characteristics, and prior perceived unsafety and fear of crime. Deviant Behavior, 43(11), 1347–1365. https://doi.org/10.1080/01639625.2021.1982657
- Carr, D., & Umberson, D. (2013). The social psychology of stress, health, and coping. In J. DeLamater & A. Ward (Eds.), Handbook of social psychology (pp. 465–487). Springer. https://doi.org/10.1007/978-94-007-6772-0_16
- Chadee, D., Williams, D., & Bachew, R. (2020). Victims’ emotional distress and preventive measures usage: Influence of crime severity, risk perception, and fear. Journal of Community & Applied Social Psychology, 30(1), 14–30. https://doi.org/10.1002/casp.2418
- Chataway, M., & Bourke, A. (2020). Fear of crime, disorder, and quality of life. In K. M. Lersch & J. Chakraborty (Eds.), Geographies of behavioural health, crime, and disorder: The intersection of social problems and place (pp. 137–163). Springer. https://doi.org/10.1007/978-3-030-33467-3_7
- Chetty, R., Hendren, N., & Katz, L. F. (2016). The effects of exposure to better neighborhoods on children: New evidence from the moving to opportunity experiment. The American Economic Review, 106(4), 855–902. https://doi.org/10.1257/aer.20150572
- Cho, W., & Ho, A. T. (2018). Does neighborhood crime matter? A multi-year survey study on perceptions of race, victimization, and public safety. International Journal of Law, Crime and Justice, 55, 13–26. https://doi.org/10.1016/j.ijlcj.2018.08.002
- Chon, D. S., & Wilson, M. (2016). Perceived risk of burglary and fear of crime: Individual- and country-level mixed modeling. International Journal of Offender Therapy and Comparative Criminology, 60(3), 308–325. https://doi.org/10.1177/0306624X14551257
- Collins, R. E. (2016). Addressing the inconsistencies in fear of crime research: A meta-analytic review. Journal of Criminal Justice, 47, 21–31. https://doi.org/10.1016/j.jcrimjus.2016.06.004
- Coyle, S., Weinreb, K. S., Davila, G., & Cuellar, M. (2022). Relationships matter: The protective role of teacher and peer support in understanding school climate for victimized youth. Child & Youth Care Forum, 51(1), 181–203. https://doi.org/10.1007/s10566-021-09620-6
- Crane, J. (1991). The epidemic theory of ghettos and neighborhood effects on dropping out and teenage childbearing. American Journal of Sociology, 96(5), 1226–1259. https://doi.org/10.1086/229654
- de Courson, B., Frankenhuis, W. E., Nettle, D., & van Gelder, J.-L. (2023). Why is violence high and persistent in deprived communities? A formal model. Proceedings. Biological Sciences, 290(1993), 20222095. https://doi.org/10.1098/rspb.2022.2095
- Denkers, A. J. M., & Winkel, F. W. (1998). Crime victims’ well-being and fear in a prospective and longitudinal study. International Review of Victimology, 5(2), 141–162. https://doi.org/10.1177/026975809800500202
- Di Tella, R., Freira, L., Gálvez, R. H., Schargrodsky, E., Shalom, D., & Sigman, M. (2019). Crime and violence: Desensitization in victims to watching criminal events. Journal of Economic Behavior & Organization, 159, 613–625. https://doi.org/10.1016/j.jebo.2017.10.005
- Dinesen, P. T., Schaeffer, M., & Sønderskov, K. M. (2020). Ethnic diversity and social trust: A narrative and meta-analytical review. Annual Review of Political Science, 23(1), 441–465. https://doi.org/10.1146/annurev-polisci-052918-020708
- Ditton, J., Chadee, D., & Khan, F. (2003). The stability of global and specific measures of the fear of crime: Results from a two wave Trinidadian longitudinal study. International Review of Victimology, 10(1), 49–70. https://doi.org/10.1177/026975800301000103
- Drakulich, K. M. (2015). Social capital, information, and perceived safety from crime: The differential effects of reassuring social connections and vicarious victimization. Social Science Quarterly, 96(1), 176–190. https://doi.org/10.1111/ssqu.12096
- Dugan, L. (1999). The effect of criminal victimization on a household’s moving decision. Criminology, 37(4), 903–930. https://doi.org/10.1111/j.1745-9125.1999.tb00509.x
- Duncan, G. J., & Raudenbush, S. W. (1999). Assessing the effects of context in studies of child and youth development. Educational Psychologist, 34(1), 29–41. https://doi.org/10.1207/s15326985ep3401_3
- Eaton, W. W. (1978). Life events, social supports, and psychiatric symptoms: A re-analysis of the New Haven data. Journal of Health and Social Behavior, 19(2), 230–234. https://doi.org/10.2307/2136537
- Ejrnæs, A., & Scherg, R. H. (2020). The impact of victimization on feelings of unsafety in different welfare regimes. European Journal of Criminology, 19(6), 1304–1326. https://doi.org/10.1177/1477370820960025
- Enders, C. K. (2013). Dealing with missing data in developmental research. Child Development Perspectives, 7(1), 27–31. https://doi.org/10.1111/cdep.12008
- Erčulj, V. (2022). The ‘young and the fearless’: Revisiting the conceptualisation of fear of crime. Quality & Quantity, 56(3), 1177–1192. https://doi.org/10.1007/s11135-021-01171-1
- Farrall, S., Bannister, J., Ditton, J., & Gilchrist, E. (1997). Questioning the measurement of the ‘fear of crime’: Findings from a major methodological study. British Journal of Criminology, 37(4), 658–679. https://doi.org/10.1093/oxfordjournals.bjc.a014203
- Gabriel, U., & Greve, W. (2003). The psychology of fear of crime: Conceptual and methodological perspectives. British Journal of Criminology, 43(3), 600–614. https://doi.org/10.1093/bjc/43.3.600
- Galster, G. (2014). Nonlinear and threshold aspects of neighborhood effects. Kölner Zeitschrift für Soziologie und Sozialpsychologie, 66(S1), 117–133. https://doi.org/10.1007/s11577-014-0268-3
- Galster, G., & Sharkey, P. (2017). Spatial foundations of inequality: A conceptual model and empirical overview. The Russell Sage Foundation Journal of the Social Sciences, 3(2), 1–33. https://doi.org/10.7758/rsf.2017.3.2.01
- Gangl, M. (2022). Longitudinal designs and models for causal inference. In Handbook of sociological science (pp. 287–308). Edward Elgar Publishing.
- Garofalo, J. (1979). Victimization and the fear of crime. Journal of Research in Crime and Delinquency, 16(1), 80–97. https://doi.org/10.1177/002242787901600107
- Gerstner, D., & Oberwittler, D. (2016). Bevölkerungsbefragung “Zusammenleben und Sicherheit in Köln/Essen”: Methodenbericht (SENSIKO Working Paper 1). Max-Planck-Institut für Ausländisches und Internationales Strafrecht.
- Girling, E., Loader, I., & Sparks, R. (2000). Crime and social change in middle England: Questions of order in an English town. Routledge.
- Häfele, J. (2013). Die Stadt, das Fremde und die Furcht vor Kriminalität. Springer. https://doi.org/10.1007/978-3-531-94201-8
- Hainmueller, J., Mummolo, J., & Xu, Y. (2019). How much should we trust estimates from multiplicative interaction models? Simple tools to improve empirical practice. Political Analysis, 27(2), 163–192. https://doi.org/10.1017/pan.2018.46
- Hale, C. (1996). Fear of crime: A review of the literature. International Review of Victimology, 4(2), 79–150. https://doi.org/10.1177/026975809600400201
- Hart, T. C., Chataway, M., & Mellberg, J. (2022). Measuring fear of crime during the past 25 years: A systematic quantitative literature review. Journal of Criminal Justice, 82, 101988. https://doi.org/10.1016/j.jcrimjus.2022.101988
- Hipp, J. R., & Yates, D. K. (2011). Ghettos, thresholds, and crime: Does concentrated poverty really have an accelerating increasing effect on crime? Criminology, 49(4), 955–990. https://doi.org/10.1111/j.1745-9125.2011.00249.x
- Hobfoll, S. E. (1989). Conservation of resources: A new attempt at conceptualizing stress. The American Psychologist, 44(3), 513–524. https://doi.org/10.1037/0003-066X.44.3.513
- Hoffman, L. (2015). Longitudinal analysis: Modeling within-person fluctuation and change. Routledge.
- Hough, M. (2004). Worry about crime: Mental events or mental states? International Journal of Social Research Methodology, 7(2), 173–176. https://doi.org/10.1080/1364557042000194559
- Jackson, J. (2004). Experience and expression: Social and cultural significance in the fear of crime. British Journal of Criminology, 44(6), 946–966. https://doi.org/10.1093/bjc/azh048
- Jackson, J. (2005). Validating new measures of the fear of crime. International Journal of Social Research Methodology, 8(4), 297–315. https://doi.org/10.1080/13645570500299165
- Jackson, J. (2006). Introducing fear of crime to risk research. Risk Analysis, 26(1), 253–264. https://doi.org/10.1111/j.1539-6924.2006.00715.x
- Janoff-Bulman, R., & Frieze, I. H. (1983). A theoretical perspective for understanding reactions to victimization. Journal of Social Issues, 39(2), 1–17. https://doi.org/10.1111/j.1540-4560.1983.tb00138.x
- Janssen, H. J., & Gerstner, D. (2016). An attrition analysis in the SENSIKO survey (waves 1 and 2) (SENSIKO Working Paper 3). Max-Planck-Institut für Ausländisches und Internationales Strafrecht.
- Janssen, H. J., Oberwittler, D., & Gerstner, D. (2022). Dissecting disorder perceptions: Neighborhood structure and the moderating role of interethnic contact and xenophobic attitudes. International Criminal Justice Review, 32(4), 429–456. https://doi.org/10.1177/1057567719896020
- Janssen, H. J., Oberwittler, D., & Koeber, G. (2021). Victimization and its consequences for well-being: A between- and within-person analysis. Journal of Quantitative Criminology, 37(1), 101–140. https://doi.org/10.1007/s10940-019-09445-6
- Jing, F., Liu, L., Zhou, S., & Feng, J. (2021). How does crime-specific victimization impact fear of crime in urban China? The role of neighborhood characteristics. International Journal of Offender Therapy and Comparative Criminology, 306624X211066829. https://doi.org/10.1177/0306624X211066829
- Jones, N. (2009). Between good and ghetto: African American girls and inner-city violence. Rutgers University Press.
- Karakus, O., McGarrell, E. F., & Basibuyuk, O. (2010). Fear of crime among citizens of Turkey. Journal of Criminal Justice, 38(2), 174–184. https://doi.org/10.1016/j.jcrimjus.2010.02.006
- Keel, C., Wickes, R., & Benier, K. (2022). The vicarious effects of hate: Inter-ethnic hate crime in the neighborhood and its consequences for exclusion and anticipated rejection. Ethnic and Racial Studies, 45(7), 1283–1303. https://doi.org/10.1080/01419870.2021.1930094
- Klijs, B., Kibele, E. U. B., Ellwardt, L., Zuidersma, M., Stolk, R. P., Wittek, R. P. M., Mendes de Leon, C. M., & Smidt, N. (2016). Neighborhood income and major depressive disorder in a large Dutch population: Results from the LifeLines Cohort study. BMC Public Health, 16(1), 773. https://doi.org/10.1186/s12889-016-3332-2
- Köber, G. (2018). A dynamic and relational perspective on vulnerability and fear of crime: The role of physical, psychological, and social factors as well as life events and neighborhood contexts using a between-within person approach [Dissertation]. University of Freiburg.
- Kollerová, L., & Smolík, F. (2016). Victimization and its associations with peer rejection and fear of victimization: Moderating effects of individual-level and classroom-level characteristics. The British Journal of Educational Psychology, 86(4), 640–656. https://doi.org/10.1111/bjep.12129
- LaGrange, R. L., Ferraro, K. F., & Supancic, M. (1992). Perceived risk and fear of crime: Role of social and physical incivilities. Journal of Research in Crime and Delinquency, 29(3), 311–334. https://doi.org/10.1177/0022427892029003004
- Lee, M., & Mythen, G. (2018). The Routledge international handbook on fear of crime. Routledge.
- Leventhal, T., & Brooks-Gunn, J. (2000). The neighborhoods they live in: The effects of neighborhood residence on child and adolescent outcomes. Psychological Bulletin, 126(2), 309–337. https://doi.org/10.1037/0033-2909.126.2.309
- Linley, P. A., & Joseph, S. (2005). The human capacity for growth through adversity. The American Psychologist, 60(3), 262–264. https://doi.org/10.1037/0003-066X.60.3.262b
- Lipsey, M. W., & Wilson, D. B. (2001). Practical meta-analysis. Sage.
- Logan, T., & Walker, R. (2021). The gender safety gap: Examining the impact of victimization history, perceived risk, and personal control. Journal of Interpersonal Violence, 36(1–2), 603–631. https://doi.org/10.1177/0886260517729405
- Lorenc, T., Clayton, S., Neary, D., Whitehead, M., Petticrew, M., Thomson, H., Cummins, S., Sowden, A., & Renton, A. (2012). Crime, fear of crime, environment, and mental health and wellbeing: Mapping review of theories and causal pathways. Health & Place, 18(4), 757–765. https://doi.org/10.1016/j.healthplace.2012.04.001
- Lüdemann, C. (2006). Kriminalitätsfurcht im urbanen Raum: Eine Mehrebenenanalyse zu individuellen und sozialräumlichen Determinanten verschiedener Dimensionen von Kriminalitätsfurcht. Kölner Zeitschrift für Soziologie und Sozialpsychologie, 58(2), 285–306. https://doi.org/10.1007/s11575-006-0056-z
- Mahuteau, S., & Zhu, R. (2016). Crime victimisation and subjective well-being: Panel evidence from Australia. Health Economics, 25(11), 1448–1463. https://doi.org/10.1002/hec.3230
- Massey, D. S. (2016). Residential segregation is the linchpin of racial stratification. City & Community, 15(1), 4–7. https://doi.org/10.1111/cico.12145
- May, D. C. (2001). The effect of fear of sexual victimization on adolescent fear of crime. Sociological Spectrum, 21(2), 141–174. https://doi.org/10.1080/02732170119080
- Meijer, M., Röhl, J., Bloomfield, K., & Grittner, U. (2012). Do neighborhoods affect individual mortality? A systematic review and meta-analysis of multilevel studies. Social Science & Medicine, 74(8), 1204–1212. https://doi.org/10.1016/j.socscimed.2011.11.034
- Miethe, T. D., Stafford, M. C., & Sloane, D. (1990). Lifestyle changes and risks of criminal victimization. Journal of Quantitative Criminology, 6(4), 357–376. https://doi.org/10.1007/BF01066676
- Norris, F. H., & Kaniasty, K. (1992). A longitudinal study of the effects of various crime prevention strategies on criminal victimization, fear of crime, and psychological distress. American Journal of Community Psychology, 20(5), 625–648. https://doi.org/10.1007/BF00941775
- Oberwittler, D. (2008). Armut macht Angst: Ansätze einer sozialökologischen Interpretation der Kriminalitätsfurcht. In A. Groenemeyer & S. Wieseler (Eds.), Soziologie sozialer Probleme und sozialer Kontrolle (pp. 215–230). VS Verlag für Sozialwissenschaften. https://doi.org/10.1007/978-3-531-90879-3_11
- O’Brien, D. T., Farrell, C., & Welsh, B. C. (2019). Looking through broken windows: The impact of neighborhood disorder on aggression and fear of crime is an artifact of research design. Annual Review of Criminology, 2(1), 53–71. https://doi.org/10.1146/annurev-criminol-011518-024638
- Office For National Statistics (2022). Crime survey for England and Wales, 1996–2020: Secure access (Version 11) [dataset]. UK Data Service. https://doi.org/10.5255/UKDA-SN-7280-11
- Pepper, G. V., & Nettle, D. (2017). The behavioural constellation of deprivation: Causes and consequences. The Behavioral and Brain Sciences, 40, e314. https://doi.org/10.1017/S0140525X1600234X
- Perkins, D. D., & Taylor, R. B. (2002). Ecological assessments of community disorder: Their relationship to fear of crime and theoretical implications. In T. A. Revenson, A. R. D’Augelli, S. E. French, D. L. Hughes, D. Livert, E. Seidman, M. Shinn, & H. Yoshikawa (Eds.), Ecological research to promote social change: Methodological advances from community psychology (pp. 127–170). Springer. https://doi.org/10.1007/978-1-4615-0565-5_6
- Plassa, W., Bernardelli, L. V., & Kortt, M. A. (2023). Victimisation and fear of crime in Brazil: The effect on the desire to move. Journal of Criminology, 56(2–3), 194–212. https://doi.org/10.1177/26338076231168403
- Rader, N. E., May, D. C., & Goodrum, S. (2007). An empirical assessment of the “threat of victimization:” Considering fear of crime, perceived risk, avoidance, and defensive behaviors. Sociological Spectrum, 27(5), 475–505. https://doi.org/10.1080/02732170701434591
- Roccato, M., Russo, S., & Vieno, A. (2011). Perceived community disorder moderates the relation between victimization and fear of crime. Journal of Community Psychology, 39(7), 884–888. https://doi.org/10.1002/jcop.20470
- Roepke, A. M., & Seligman, M. E. P. (2015). Doors opening: A mechanism for growth after adversity. The Journal of Positive Psychology, 10(2), 107–115. https://doi.org/10.1080/17439760.2014.913669
- Ross, C. E., Mirowsky, J., & Pribesh, S. (2001). Powerlessness and the amplification of threat: Neighborhood disadvantage, disorder, and mistrust. American Sociological Review, 66(4), 568–591. https://doi.org/10.2307/3088923
- Russo, S., & Roccato, M. (2010). How long does victimization foster fear of crime? A longitudinal study. Journal of Community Psychology, 38(8), 960–974. https://doi.org/10.1002/jcop.20408
- Russo, S., Roccato, M., & Vieno, A. (2013). Criminal victimization and crime risk perception: A multilevel longitudinal study. Social Indicators Research, 112(3), 535–548. https://doi.org/10.1007/s11205-012-0050-8
- Salm, M., & Vollaard, B. (2021). The dynamics of crime risk perceptions. American Law and Economics Review, 23(2), 520–561. https://doi.org/10.1093/aler/ahab012
- Sampson, R. J. (2009). Disparity and diversity in the contemporary city: Social (dis)order revisited. The British Journal of Sociology, 60(1), 1–31. https://doi.org/10.1111/j.1468-4446.2009.01211.x
- Sampson, R. J. (2012). Great American city: Chicago and the enduring neighborhood effect. University of Chicago Press.
- Sampson, R. J. (2022). Legacies of inequality, legacy lead exposures, and improving population well-being. Proceedings of the National Academy of Sciences of the United States of America, 119(14), e2202401119. https://doi.org/10.1073/pnas.2202401119
- Sampson, R. J., & Raudenbush, S. W. (1999). Systematic social observation of public spaces: A new look at disorder in urban neighborhoods. American Journal of Sociology, 105(3), 603–651. https://doi.org/10.1086/210356
- Sampson, R. J., Raudenbush, S. W., & Earls, F. (1997). Neighborhoods and violent crime: A multilevel study of collective efficacy. Science, 277(5328), 918–924. https://doi.org/10.1126/science.277.5328.918
- Schaeffer, M. (2013). Can competing diversity indices inform us about why ethnic diversity erodes social cohesion? A test of five diversity indices in Germany. Social Science Research, 42(3), 755–774. https://doi.org/10.1016/j.ssresearch.2012.12.018
- Scherg, R. H., & Ejrnæs, A. (2022). Heterogeneous impact of victimization on sense of safety: The influence of past victimization. Victims & Offenders, 17(3), 395–420. https://doi.org/10.1080/15564886.2021.1943091
- Schüle, S. A., & Bolte, G. (2015). Interactive and independent associations between the socioeconomic and objective built environment on the neighbourhood level and individual health: A systematic review of multilevel studies. PLOS One, 10(4), e0123456. https://doi.org/10.1371/journal.pone.0123456
- Seery, M. D., Holman, E. A., & Silver, R. C. (2010). Whatever does not kill us: Cumulative lifetime adversity, vulnerability, and resilience. Journal of Personality and Social Psychology, 99(6), 1025–1041. https://doi.org/10.1037/a0021344
- Seery, M. D., Leo, R. J., Lupien, S. P., Kondrak, C. L., & Almonte, J. L. (2013). An upside to adversity? Moderate cumulative lifetime adversity is associated with resilient responses in the face of controlled stressors. Psychological Science, 24(7), 1181–1189. https://doi.org/10.1177/0956797612469210
- Shadish, W. R., Cook, T. D., & Campbell, D. T. (2002). Experimental and quasi-experimental designs for generalized causal inference. Wadsworth Cengage Learning.
- Shapland, J., & Hall, M. (2007). What do we know about the effects of crime on victims? International Review of Victimology, 14(2), 175–217. https://doi.org/10.1177/026975800701400202
- Sharkey, P., & Elwert, F. (2011). The legacy of disadvantage: Multigenerational neighborhood effects on cognitive ability. American Journal of Sociology, 116(6), 1934–1981. https://doi.org/10.1086/660009
- Sharkey, P., & Faber, J. W. (2014). Where, when, why, and for whom do residential contexts matter? Moving away from the dichotomous understanding of neighborhood effects. Annual Review of Sociology, 40(1), 559–579. https://doi.org/10.1146/annurev-soc-071913-043350
- Skogan, W. G. (1987). The impact of victimization on fear. Crime & Delinquency, 33(1), 135–154. https://doi.org/10.1177/0011128787033001008
- StataCorp (2021). Stata statistical software: Release 17 [Computer software]. StataCorp LLC.
- Stein, R. E. (2014). Neighborhood residents’ fear of crime: A tale of three cities. Sociological Focus, 47(2), 121–139. https://doi.org/10.1080/00380237.2014.883860
- Swartz, K., Reyns, B. W., Henson, B., & Wilcox, P. (2011). Fear of in-school victimization: Contextual, gendered, and developmental considerations. Youth Violence and Juvenile Justice, 9(1), 59–78. https://doi.org/10.1177/1541204010374606
- Turanovic, J. J. (2019). Heterogeneous effects of adolescent violent victimization on problematic outcomes in early adulthood. Criminology, 57(1), 105–135. https://doi.org/10.1111/1745-9125.12198
- Turner, R. J., & Lloyd, D. A. (1995). Lifetime traumas and mental health: The significance of cumulative adversity. Journal of Health and Social Behavior, 36(4), 360–376. https://doi.org/10.2307/2137325
- Updegraff, J., & Taylor, S. (2000). From vulnerability to growth: Positive and negative effects of stressful life events. In J. H. Harvey & Eric. D. Miller (Eds.), Loss and trauma: General and close relationship perspectives (pp. 3–28). Brunner-Routledge.
- Valente, R., Valera Pertegas, S., & Guàrdia Olmos, J. (2022). Feeling unsafe in Italy’s biggest cities. European Journal of Criminology, 19(4), 849–867. https://doi.org/10.1177/1477370820932075
- Ward, J. T., Link, N. W., & Taylor, R. B. (2017). New windows into a broken construct: A multilevel factor analysis and DIF assessment of perceived incivilities. Journal of Criminal Justice, 51, 74–88. https://doi.org/10.1016/j.jcrimjus.2017.06.004
- Weitzer, R., & Kubrin, C. E. (2004). Breaking news: How local TV news and real-world conditions affect fear of crime. Justice Quarterly, 21(3), 497–520. https://doi.org/10.1080/07418820400095881
- Wilcox Rountree, P., & Land, K. C. (1996a). Burglary victimization, perceptions of crime risk, and routine activities: A multilevel analysis across Seattle neighborhoods and census tracts. Journal of Research in Crime and Delinquency, 33(2), 147–180. https://doi.org/10.1177/0022427896033002001
- Wilcox Rountree, P., & Land, K. C. (1996b). Perceived risk versus fear of crime: Empirical evidence of conceptually distinct reactions in survey data. Social Forces, 74(4), 1353–1376. https://doi.org/10.1093/sf/74.4.1353
- Winkel, F. W., Blaauw, E., Sheridan, L., & Baldry, A. C. (2003). Repeat criminal victimization and vulnerability for coping failure: A prospective examination of a potential risk factor. Psychology, Crime & Law, 9(1), 87–95. https://doi.org/10.1080/10683160308137
- Wright, E. M., & Fagan, A. A. (2013). The cycle of violence in context: Exploring the moderating roles of neighborhood disadvantage and cultural norms. Criminology: An Interdisciplinary Journal, 51(2), 217–249. https://doi.org/10.1111/1745-9125.12003
- Xie, M., & Mcdowall, D. (2008). Escaping crime: The effects of direct and indirect victimization on moving. Criminology, 46(4), 809–840. https://doi.org/10.1111/j.1745-9125.2008.00133.x
- Yaremych, H. E., Preacher, K. J., & Hedeker, D. (2023). Centering categorical predictors in multilevel models: Best practices and interpretation. Psychological Methods, 28(3), 613–630. https://doi.org/10.1037/met0000434