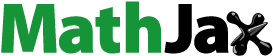
Abstract
Multiple animals and in vitro studies have demonstrated that perfluoroalkyl and polyfluoroalkyl substances (PFASs) exposure causes liver damage associated with fat metabolism. However, it is lack of population evidence for the correlation between PFAS exposure and nonalcoholic fatty liver disease (NAFLD). A cross-sectional analysis was performed of 1150 participants aged over 20 from the US. Liver ultrasound transient elastography was to identify the participants with NAFLD and multiple biomarkers were the indicators for hepatic steatosis and hepatic fibrosis. Logistics regression and restricted cubic splines models were used to estimate the association between PFASs and NAFLD. PFASs had not a significant association with NAFLD after adjustment. The hepatic steatosis indicators including fatty liver index, NAFLD liver fat score, and Framingham steatosis index were almost not significantly correlated with PFASs exposure respectively. But fibrosis indicators including fibrosis-4 index (FIB-4), NAFLD fibrosis score, and Hepamet fibrosis score were positively correlated with each type of PFASs exposure. After adjustment by gender, age, race, education, and poverty income rate, there was also a significant correlation between PFOS and FIB-4 with 0.07 (0.01, 0.13). The mixed PFASs were associated with FIB-4, with PFOS contributing the most (PIP = 1.000) by the Bayesian kernel machine regression model. The results suggested PFASs exposure appeared to be more closely associated with hepatic fibrosis than steatosis, and PFOS might be the main cause of PFASs associated with hepatic fibrosis.
Current exposure doses of PFAS did not significantly change the risk of developing NAFLD.
PFASs exposure appeared to be more closely associated with hepatic fibrosis than steatosis.
PFOS might be the main cause of PFASs associated with hepatic fibrosis.
Key messages
Introduction
Due to the rapid development of the economy and the change of lifestyle, nonalcoholic fatty liver disease (NAFLD) has become the main cause of liver disease in the world, which may develop into steatohepatitis, cirrhosis and even liver cancer, threatening human health [Citation1]. The estimated global prevalence of NAFLD was about 30% in recent years, and it has gradually increased and become a major concern [Citation2,Citation3]. Exposure to environmental pollutants is also an important cause of NAFLD as the liver is the most important metabolic organ.
Perfluoroalkyl and polyfluoroalkyl substances (PFASs) are not only widely found in food packaging [Citation4], nonstick cookware [Citation5], outdoor apparel [Citation6], and other consumer products because of their chemical inertness and high-temperature resistance, but also in surface water [Citation7] and aquatic biota [Citation8] due to their strong resistance to degradation. The Centers for Disease Control and Prevention of the U.S. added them to the routine health testing items decades ago, and 98% of U.S. adults had residual PFASs in their blood in 2014 [Citation9].
PFAS exposure and NAFLD may have a link. A cross-sectional study from National Health and Nutrition Examination Survey (NHANES) (2011 ∼ 2014) on US adults, pointed out that perfluorooctane sulfonic acid (PFOS), perfluorohexane sulfonic acid (PFHxS) and perfluorononanoic acid (PFNA) serum concentrations were positively associated with alanine aminotransferase (ALT) and gamma-glutamyl transferase (GGT) in obese participants aged ≥20 years [Citation10]. Another study with 200 participants aged between 40 to 70 from six water districts were contaminated with perfluorooctanoic acid (PFOA) in the mid-Ohio Valley of Ohio and West Virginia from 2005 to 2006 indicated that cytokeratin 18 M30, a promising serologic NAFLD biomarker, positively associated with PFHxS, PFOA and PFNA in serum [Citation11]. All these indirectly indicate a potential association between PFASs exposure and NAFLD. The trials in vivo and in vitro also provide biological evidence that PFAS exposure led to NAFLD. Dietary treatment of mice with 0.002% (w/w) PFOA or 0.005% (w/w) PFOS for 10 days resulted in significant reductions in serum levels of cholesterol and triglyceride (TG), a moderate increase in the serum activity of alkaline phosphatase and hepatomegaly [Citation12]. PFOA and PFOS have been shown showed to induce hepatocyte peroxisome proliferation, liver hypertrophy, vacuolization and hyperplasia in vitro [Citation13]. In addition, long-term feeding of low concentrations of PFASs to mice, such as 0.0001% (w/w) PFOA for 8 weeks and 0.0001% (w/w) PFOS for 6 weeks might have a protective effect against fatty diet-induced hepatic steatosis [Citation14,Citation15]. But the mechanisms behind this paradoxical phenomenon are not yet clear.
Therefore, whether different exposure levels of PFASs in the US population have a direct impact on the occurrence of NAFLD needs further study. In this cross-sectional study, the generalized linear model (GLM), nonlinear restricted cubic spline (RCS) model, and Bayesian kernel machine regression (BKMR) model were used to assess the single effect, nonlinear dose-response relationship, and overall effects of PFASs on NAFLD-related indicators.
2 Methods
2.1. Data source
NHANES is a large cross-sectional survey, that combines interviews and physical examinations to assess the health and nutritional status of adults and children in the United States (https://www.cdc.gov/nchs/nhanes/index.htm). The NHANES 2017–2018 survey is the only one that includes the liver ultrasound transient elastography examination which use to identify participants with fatty liver.
2.2. Study participants
There were 9254 participants in NHANES 2017–2018, of whom 5492 (59.3%) had a completed liver ultrasound transient elastography examination. The 983 participants were excluded for age under 20, and 181 were excluded for viral hepatitis (43 for HCV RNA+; 27 for HBsAg+) and 73 for excess alcohol (more than two drinks per day in women and more than three drinks per day in men) [Citation16]. At last, 99 participants were excluded for body mass index (BMI) < 18.5, as the link between undernutrition and fatty liver was more complex and less understood [Citation17]. showed the flowchart of participant selection. The tests of HBV surface antigen and HCV RNA were detailed in the supplementary document.
2.3. Vibration-Controlled transient elastography (VCTE)
VCTE involves an ultrasound machine (FibroScan model 502 V2 Touch) with a handheld transducer that taps, in rapid succession, on the participant’s abdomen. The controlled attenuation parameter (CAP) could be quantified liver fat [Citation18]. CAP values ranged from 100 to 400 dB/m, with higher values indicating higher amounts of liver fat [Citation19]. Participants with NAFLD were diagnosed as having a median CAP score of ≥285 dB/m–optimized sensitivity of 80% and specificity of 77% for detecting hepatic steatosis [Citation20]. Although not as reliable as liver biopsy, VCTE has been used as an identification method for NAFLD in several studies because it is noninvasive and has higher accuracy than common liver color ultrasounds [Citation17,Citation21–23].
2.4. PFAS detection
The blood samples were collected, and serum specimens were stored at −30 °C until being detected. Online Solid Phase Extraction-High Performance Liquid Chromatography- Turbo Ion Spray-Tandem Mass Spectrometry (online SPE-HPLC-TIS- MS/MS) was used for the quantitative detection of PFAS. A total of 5 PFAS with the highest rate of detection (>80%) in the US population were considered, including perfluorodecanoic acid (PFDeA), PFHxS, PFNA, PFOA and PFOS. The concentration of PFOA was the sum of the concentrations of linear PFOA and the branched PFOA isomers. The concentration of PFOS was the sum of the linear PFOS and monomethyl-branched PFOS isomers. The lower limit of detection (LLOD in) for each PFAS was 0.10 ng/mL and replaced with LLOD divided by the square root of 2, which was 0.07 ng/mL [Citation24]. Total relative standard deviations were ≤ 15% (CV ≤ 15%).
2.5. Hepatic steatosis-related biomarkers detection
2.5.1. Fatty liver index (FLI)
FLI was to predict hepatic steatosis, performed by BMI, waist circumference, TG and GGT. The area under the ROC curve (AUROC curve) was 0.84 with ultrasonography as the standard in diagnosing fatty liver [Citation25]. The equation for FLI is:
2.5.2. NAFLD liver fat score (LFS)
NAFLD LFS was used to estimate liver fat based on metabolic syndrome and type 2 diabetes, serum insulin, aspartate aminotransferase (AST) and the AST/ALT ratio. The AUROC curve was 0.86 with proton magnetic resonance spectroscopy as the standard in diagnosing fatty liver [Citation26]. The equation for LFS is:
The detailed definition of metabolic syndrome is in the supplementary materials.
2.5.3. Framingham steatosis index (FSI)
FSI was used to identify hepatic steatosis. The AUROC curve of FSI was 0.845 with abdominal Computed Tomography scans as the standard in diagnosing fatty livers [Citation27]. Through constructing a stepwise regression model, FSI was derived from the indexes which were strongly associated with steatosis including age, sex, BMI, TG, hypertension, diabetes and the ALT/AST ratio. The equation for FSI is:
2.6. Hepatic fibrosis-related biomarkers
2.6.1. Fibrosis-4 index (FIB-4)
The FIB-4 was originally developed for patients co-infected with HCV/HIV in 2006 [Citation28]. As a widely used non-invasive biomarker, FIB-4 used four indicators associated with liver fibrosis including platelets, ALT, AST and age to predict the level of liver fibrosis in many liver diseases including NAFLD [Citation17,Citation29,Citation30]. The overall statistical consistency with liver biopsy in the classification of hepatic fibrosis was 0.87 [Citation28]. The equation for FIB-4 is [Citation28]:
2.6.2. NAFLD fibrosis score (NFS)
NFS was constructed based on age, hyperglycemia, BMI, platelet count, albumin, and AST/ALT ratio to separate NAFLD patients with and without advanced fibrosis in 2007. The statistical consistency with liver biopsy in diagnosing advanced fibrosis was 0.82 [Citation31]. The equation for NFS is:
2.6.3. Hepamet fibrosis score (HFS)
HFS is a new fibrosis scoring system, developed in 2020. Variables used to determine HFS were sex, age, homeostatic model assessment score, presence of diabetes, levels of aspartate aminotransferase, and albumin, and platelet counts. HFS discriminated between patients with and without advanced fibrosis with an AUROC curve value of 0.85 [Citation32]. The equation for HFS is:
Homeostatic model assessment of insulin resistance (HOMA-IR) was calculated based on insulin and glucose [fasting insulin(µU/mL) x fasting glucose(mg/dL)/405].
The detailed methods for the detection of biomarkers related to NAFLD, including BMI, blood pressure (BP), ALT, AST, GGT, albumin, TG, high-density lipoprotein (HDL), fasting plasma glucose (FPG), insulin and platelet count are in the supplementary materials.
2.7. Covariates selection
A directed acyclic graph (DAG) was made based on the results of baseline characteristics comparison and prior knowledge. And the DAGitty v3.0 was used to visualize DAG and calculated the minimal sufficient adjustment sets to recognize the covariates.
The baseline characteristics included socio-economic factors (gender, age, race, education, marital status, poverty income rate (PIR)), pathophysiological factors (cancer, hypertensive, diabetic, liver diseases, creatinine level which might be harmful to the livers and affect chemical concentrations among participants), and behavior factors (drug use, smoking status and physical activity). The methods for information collection of baseline characteristics are shown in the supplementary materials.
2.8. Statistical analysis
2.8.1. Description of distribution
The total population was divided into NAFLD and non-NAFLD. The PFASs value was divided into quartiles. To compare the distribution differences of baseline characters, the Chi-square tests were used for categorical data and the Kruskal-Wallis tests were used for continuous data. Kruskal-Wallis tests were used to compare the distribution of NAFLD-related biochemical values in quartiles of PFASs.
2.8.2. The association between PFASs exposure and NAFLD
Two logistic models were used to study the association between PFASs exposure and NAFLD. Model 1 was a univariate model, and model 2 was a multivariate model adjusted for covariates. The PFASs levels were divided into quartiles and compared three other quartiles with the first to calculate the odds ratio (OR) and 95% confidence interval (95% CI) to estimate the risk of NAFLD in the population exposed to PFASs at each quantile level. Besides, the median exposure level of each group of elements was taken as a continuous variable to conduct a trend test. For models with significant trends, RCS was employed to reveal the dose-response relationship between PFASs and NAFLD. RCS was suitable for nonlinear models and could supplement the shortcomings of GLM [Citation33]. The generation of RCS is detailed in the supplementary material.
2.8.3. Correlations among PFASs and between PFASs and NAFLD-related biomarkers
Logarithmic transformation of PFASs values was performed to approximate the normal distribution, as shown in the quantile-quantile plot (Figure S1). The Spearman’s test was used to analyze the correlation between PFASs and NAFLD-related biomarkers values. And Pearson’s test was used to analyze the correlation among PFASs.
2.8.4. Association of FIB-4 with PFAS
Two GLMs included a univariate model and a multivariate model adjusted for covariates to estimate the increased risk of FIB-4 in the population exposed to single PFASs.
And BKMR was used to explore the mixed exposure effect [Citation34]. The BMKR model is Yi = ℎ(PFDeA, PFHxS, PFNA, PFOA, PFOS) + βxi + εi, where denotes the FIB-4 for individual
,
denotes the unknown exposure-response function to be estimated,
represents the effect of the covariates (
is a vector), and
is the residual. The covariates were consistent with those mentioned earlier. The number of iterations of the Markov chain Monte Carlo sampler was 10,000. A BMKR model without variable selection and a BMKR model with gamma prior distribution was fitted to demonstrate the robustness of the results [Citation35].
To account for the complex survey sampling, the strata (sdmvstra), primary sampling units (sdmvpsu) information, and the weight was used to adjust the results of logistics regression. The R ‘Survey’ package was used to adjust the results according to the NHANES’s analytic guideline (https://wwwn.cdc.gov/nchs/nhanes/ analyticguidelines.aspx). The statistically significant difference was defined as a two-sided p < 0.05.
3 Results
3.1. Effect of PFASs exposure on the risk of developing NAFLD
Among those with completed ultrasound transient elastography of the liver, those who were younger than 20 years of age, with excessive alcohol consumption, hepatitis B/C, and a BMI of less than 18.5 were excluded. A total of 4267 people were included and divided into non-alcoholic liver patients and healthy controls. According to NHANES analytic guidelines, the samples were weighted, and then the weighted sample represented over 197.6 million Americans with 36.8% NAFLD, as shown in .
Table 1. Baseline characteristics among the healthy control and NAFLD.
The baseline features were compared between normal and NAFLD group, including socio-economic factors (gender, age, race, education, marital status, PIR), pathophysiological factors (cancer, hypertensive, diabetic, liver diseases), behavior factors (drug use, smoking status and physical activity) and urine creatine level. There were statistical differences in socio-economic characteristics including gender (p < 0.001), age (p < 0.001), race (p < 0.001), education levels (p = 0.035) and marital status (p < 0.001) between normal and NAFLD group while there was no significant difference in PIR. In pathophysiological characteristics, there were significant differences in the history of cancer (p = 0.015), hypertension (p < 0.001), diabetes (p < 0.001), and liver disease (p = 0.002), but not urinary creatinine levels. And in behavior characteristics, there were significant differences in smoking (p = 0.013), and physical activity status (p < 0.001) between NAFLD and normal while there was no significant difference in drug use. Males, aging, Mexicans, married or living with a partner, lower education level, a history of cancer, hypertension, diabetes, liver diseases, smoking and lower physical activity were more common among NAFLD patients.
A total of 1318 samples were successfully merged with PFASs laboratory data. The populations were grouped according to quartile of PFAS exposure concentration and compared baseline characteristics. As shown in , there were significant differences in the distribution of gender, age, race and PIR in people exposed to different concentrations of the same PFASs. The concentrations of PFNA and PFOA exposure differed between those with and without a college education. And the concentrations of PFOS exposure differed between those with and without a history of hypertension. Marital status, history of cancer, diabetes, liver disease, urinary creatinine levels, drug use, smoking, and physical activity were not associated with PFASs exposure. The details were shown in Table S2.
Table 2. The P value of Chi-square tests conducted by PFASs quartile grouping.
The DAG graphs were formed through baseline characteristic comparison results and prior knowledge, as shown in . The covariates were recognized as Gender, Age, Race, Education and PIR.
Figure 2. DAG was made based on the results of baseline characteristics comparison and prior knowledge.

After removing 3 participants with education information missing and 165 participants with PIR information missing, a total of 1150 participants were used to construct two logistic models to study the association between PFASs exposure and NAFLD. Model 1 was a univariate model, and model 2 was a multivariate model adjusted for covariates of gender, age, race, education and PIR. The results were shown in . Taking the first interval as the reference, all intervals of PFHxS, PFNA, PFOA, and PFOS had no significant association with NAFLD in models 1 and 2. And in PFDeA, the OR of quartile 4 was 0.47 (0.26-0.85) in model 1, but without a significant association in model 2. Besides, the trend tests of all the models were also performed. The trend tests for PFDeA were significant in both model 1 (p = 0.013) and model 2 (p = 0.038). The nonlinear relationship was explored between PFDeA and OR by RCS, as shown in . No statistically significant non-linear association was found in PFDeA with NAFLD in model 1 (p = 0.981 for the non-linear test) and model 2 (p = 0.597 for the non-linear test).
Figure 3. Effect of PFASs exposure on the risk of developing NAFLD. (A) Logistic regression result of NAFLD and single PFASs. Odds ratios (OR)1 and P1 for model 1 without adjusted; OR2 and P2 for model 2 with adjusted by gender, age, race and education and PIR. ‘#’ was the result of P for trend. (B) RCS results of NAFLD and ln (PFDeA). The median value of ln (PFDeA) was used as a reference point. Blue was the density of the ln (PFDeA) distribution.

3.2. Effects of PFAS exposure on NAFLD-related biomarkers
Liver ultrasound transient elastography is not the gold standard for NAFLD, so the association between PFASs with other NAFLD-related biomarkers such as body measure, biochemical indicators, and FLI, LFS, FSI, FIB-4, NFS, HFS were further analyzed. showed the biomarkers have significant differences among different PFASs exposure. The details were shown in Table S3. The concentration (ng/mL) for PFDeA was 0.20 [0.10, 0.30] (median [IQR]), for PFHxS was 1.20 [0.70, 1.90], for PFNA was 0.50 [0.30,0.70], PFOA was 1.47 [0.97, 2.17], for PFOS was 4.60 [2.70, 8.06]. The result suggests BMI changed significantly in all PFASs exposures while waist circumference significantly changed among PFDeA, PFHxS and PFNA exposure. AST was significantly related to PFHxS, PFOA and PFOS and seemed to be most closely related to PFASs exposure among the transaminases. ALT was related to the PFHxS and PFOS while the GGT was related to PFOS. Serum albumin was a critical plasma protein produced by the liver. Advanced cirrhosis was characterized by reduced albumin concentration as well as impaired albumin function [Citation36]. The result showed that differences in albumin might be related to PFHxS, PFOA and PFOS exposure. TG and HDL were related to PFOS and PFDeA respectively. Insulin resistance was the major hallmark of NAFLD, indirectly influencing hepatic glucose and lipid metabolism by increasing the flux of substrates that promoted lipogenesis and gluconeogenesis [Citation37]. PFOS was closely related to fasting plasma glucose (FPG), insulin and HOMA-IR. Liver damage was associated with widespread coagulation dysfunction. The latest research also showed that platelets accelerated liver regeneration and promoted liver fibrosis [Citation38]. In this result, platelet count was significantly related to PFHxS, PFNA, PFOA, and PFOS respectively.
Table 3. The P value of the Kruskal-Wallis tests which were conducted by PFASs quartile grouping.
FLI, LFS and FSI were to assess the relationship between PFASs and hepatic steatosis. FLI was significantly related to PFNA, while LFS and FSI were related to PFOS. PFASs exposure appeared to be more closely associated with liver fibrosis than with steatosis. NFS was significantly related to PFHxS, PFOA and PFOS while HFS was significantly related to PFNA, PFOA and PFOS separately. More importantly, FIB4 changes occurred in all the types of PFAS exposed.
As shown in , through Spearman’s correlation analysis, albumin, FPG, platelet count, FIB4 and HFS had significant correlations with all of PFASs. Platelet count had negative relations with PFASs, while albumin, FPG, FIB4 and HFS had positive relations. Interestingly, three hepatic steatosis indicators FLI, LFS and FSI were almost not significantly correlated with PFASs exposure. But fibrosis indicators FIB-4, NFS and HFS were positively correlated with almost all PFASs exposure. Therefore, PFASs exposure is not closely associated with hepatic steatosis but may aggravate liver fibrosis.
Figure 4. Correlation analysis (A) Spearman correlation coefficients between PFASs and NAFLD biomarkers. (B) Pearman correlation coefficients among PFASs logarithm. The correlation coefficient was shown in color. Blue was a positive correlation and red was a negative correlation. Color darker, circle bigger implied a stronger correlation. Demerit marks showed p > 0.05.

In addition, we also constructed a Pearson’s correlation analysis to explore the correlation between PFASs after logarithmic transformation, as shown in . All PFASs exposures were positively correlated. The correlation order was PFDeA vs PFNA (r = 0.74), PFNA vs PFOS (r = 0.72), PFHxS vs PFOS (r = 0.71), PFNA vs PFOA (r = 0.67), PFOA vs PFHxS (r = 0.63), PFDeA vs PFOS (r = 0.61), PFOA vs PFOS (r = 0.59), PFOA vs PFDeA (r = 0.51), PFHxS vs PFNA (r = 0.49), PFHxS vs PFDeA (r = 0.28). The P for all correlation tests of significance was less than 0.001.
3.3. Associations of hepatic fibrosis-related biomarkers with PFASs by GLM models
The GLM was used to further verify whether there were significant correlations between PFAS and liver fibrosis indicators. The result was shown in . In the univariate model, PFDeA (p = 0.005), PFHxS (p < 0.001), PFNA (p < 0.001), PFOA (p < 0.001) and PFOS (p < 0.001) were all positively related with FIB-4; PFHxS (p = 0.026), PFNA (p = 0.047), PFOA (p = 0.026) and PFOS (p = 0.002) were positively related with NFS; and PFNA (p = 0.009), PFOA (p = 0.007), PFOS (p = 0.010) were positively related to HFS. However, after the adjusted gender, age, race, education, and PIR, only the correlation between PFOS and FIB-4 was significant (p = 0.025).
Figure 5. The association between PFASs and hepatic fibrosis-related biomarkers was analyzed by GLM. Model 1 was unadjusted; model 2 was adjusted by gender, age, race and education and PIR.

The findings of FIB-4 remained robust in our sensitivity analysis using the study cohort without participant selection (N = 1929, PFASs test completed), as shown in Figure S2.
3.4. Multiple exposures of PFAS affected on FIB-4 by BKMR model
The BKMR model was used to explore the overall and individual of the PFASs by gender, age, race, education and PIR adjustment. The posterior inclusion probability (PIP) of each PFASs was calculated. The PIP values for PFASs from high to low were 1.000 for PFOS, 0.441 for PFHxS, 0.025 for PFOA, 0.005 for PFDeA, and 0.004 for PFNA. It suggested that PFOS was significantly correlated to FIB-4 in the BKMR model. Besides, the global effect of the mixed PFASs on FIB-4 was significant. As compared to all of the PFASs were fixed at their median values, when all of the PFASs were at their 75th percentile the FIB-4 increased by 0.143 units, while at their 25th percentile, the FIB-4 decreased by 0.102 units, as shown in . The higher exposure to the PFASs was accompanied by a greater risk of FIB-4. The interaction of the mixed PFASs was also assessed. For PFOS, it all showed significant and positive effects on the risk of FIB-4 increase whatever all of the other PFASs were fixed at their 25th, 50th, or 75th percentiles, as shown in . But other PFASs had no significant effect on FIB-4. Therefore, PFOS was the main reason that PFASs increased the risk of FIB-4. Models from sensitivity analyses were very similar to our preliminary results, which proved the robustness of our results, as shown in Figure S3.
Figure 6. The overall and individual exposure effect of PFASs on FIB-4. (A) The overall effect of the PFASs mixture being fixed to different percentiles as compared to their 50 percentiles. (B) The estimated values of the individual effect were calculated by comparing the FIB-4 increase risk when a single PFAS was at its 75th percentile as compared to when that was at its 25th percentile, where all of the remaining PFAS were fixed at their 25th (red line), 50th (green line), or 75th (blue line) percentile.

Discussion
In this study, the associations were investigated between several serum PFASs and NAFLD based on liver ultrasound transient elastography and other non-invasive biomarkers. The GLM and RCS were constructed to reveal there was no significant association between PFASs and CAP. The results of hepatic steatosis-related indicators FLI, LFS and FSI also demonstrated that PFASs had no significant correlation with hepatic steatosis based on ANOVA and Spearman’s tests. But PFASs exposure was indeed closely related to glucose and lipid metabolism. Serum TG has positive correlations with PFHxS, PFNA, PFOA and PFOS while FPG had positive correlations with all of the PFASs in the study. PFASs structurally resembled fatty acids and activated the peroxisome proliferators-activated receptors (PPARs) signaling pathway, responsible for perturbations of glucose and lipid homeostasis partially [Citation39]. A new study showed serum albumin mediated the effects of PFASs on serum lipid levels [Citation40]. PFASs had shown a strong binding affinity to serum proteins and were positively associated with serum albumin [Citation41], which was bound to PFASs and transported to target organs that regulate serum lipid levels [Citation40]. In addition, we also found that PFASs were negatively correlated with BMI and positively correlated with HDL which was also reported in a previous study among European teenagers [Citation42]. But a high level of PFOA (median level = 1635.96 ng/mL) was reported to negatively associate with HDL in workers of a fluorochemical plant [Citation43]. These evidences suggested that the effects of PFASs on hepatic steatosis appeared to be a double-edged sword and associated with the exposure concentration. The activation of PPARs induced genes in both lipogenesis/uptake and fatty acid oxidation pathways. But targeting PPARs was also an emerging strategy for the treatment of steatosis and inflammation. The agonists of PPARα (fenofibrate) and PPARγ (pioglitazone, lobeglitazone) had been shown to reduce hepatic steatosis in both animal studies and clinical trials. Additionally, the lipid metabolism pathways might be influenced by the feedback loop [Citation44]. As previously mentioned, PFOS and PFOA were found to relieve steatosis in mice with diet induce-fatty liver [Citation14, Citation15]. The molecular mechanisms of the switch between lipogenic and lipid oxidation effects induced by PFASs are not fully understood.
FIB-4, NFS and HFS were used to assess the association between PFASs exposure and hepatic fibrosis. The results of Spearman’s test showed FIB-4, NFS and HFS were positively correlated with PFASs. Elevated transaminases have been found under multiple PFASs exposures. It is consistent with previous studies [Citation45–48]. More importantly, platelet accounts significantly decreased under PFASs exposure. It was also found in the previous study among young and middle-aged Taiwanese populations that platelet count decreased significantly with increasing quartiles of PFOA and PFOS [Citation49]. Platelet was proven to decrease hepatic fibrosis. Platelets contacted hepatic stellate cells (HSCs) and released adenine nucleotides, which subsequently led to the production of adenosine through the degradation by HSCs. Activated HSCs were inactivated by adenosine and had a decreased ability to produce pro-fibrotic growth factor TGF-β and secrete extracellular matrix [Citation38]. The decrease in platelet count associated with PFASs exposure might be the main reason why PFASs showed an association with hepatic fibrosis but not steatosis. So, PFASs exposure appeared to be more closely associated with hepatic fibrosis than steatosis. The reduction of platelets caused by PFASs might be the key to the changes in liver fibrosis. Regular examination for platelet in people with high PFASs exposure might be effective in preventing the development of liver fibrosis.
The GLM was used to verify the association between PFASs and hepatic fibrosis-related indicators. PFOS exposure was linearly significant with FIB-4, both before and after adjustment. Due to the interaction between PFASs, we also adopted the BKMR model to verify the mixed effects and single effects of PFAS. The results showed that the higher the mixed PFASs concentration, the higher the FIB-4 of the participants. PFOS was the main cause of FIB-4 elevation. This result was also verified by the BKMR model under different priors.
Exposure to PFAS has generated publicity in some specific occupational populations. The median concentration of PFOA in the serum of professional ski waxers was 50 ng/ml [Citation50]. In the serum of fluorochemical plant workers, the median concentration of PFOA was 1635.96 ng/mL and PFOS was 33.46 ng/mL [Citation43]. And in the serum of firefighters, the median concentration of PFOA was 31.50 ng/mL and PFOS was 27.85 ng/mL [Citation51]. These were well above the normal levels in the general population in our study. These specific occupational populations are likely to be at high risk for liver fibrosis. Although US environmental protection agency has proposed maximum contaminant level goals in drinking water for PFASs [Citation52] and the US Conference for governmental industrial hygienists has established threshold limit values for three PFAS in the air: perfluoroisobutylene, perfluorobutyl ethylene, and ammonium perfluorooctanoate [Citation53]. The occupational protection administration for PFASs is far from adequate. Further studies of quantitative individual exposure and biomarker data in populations with occupational exposure to PFAS are urgently needed.
This study has several strengths. It is the first study using multiple NAFLD-related biomarkers to analyze the association between PFASs and NAFLD. Then, a variety of models including GLM, RCS, and BKMR were used to assess the single effect, nonlinear dose-response relationship, and overall effects of PFASs on NAFLD. Additionally, the present study used a nationally representative sample of the US population, with a relatively large population, and adjusted for potential confounders, which reduced the potential bias associated with the selection of study subjects and rendered the findings generalizable. Inevitably, the present study also has several deficiencies. The results were not based on the exact liver biopsy. Because of the limitations of a cross-sectional study itself, laboratory research and prospective cohort studies are needed to verify in the future.
Conclusion
Our study indicated that current exposure doses of PFAS did not significantly change the risk of developing NAFLD based on the liver ultrasound transient elastography. From the changes of NAFLD-related biomarkers, including FLI, LFS, FSI, FIB-4, NFS, HFS, PFASs exposure appeared to be more closely associated with hepatic fibrosis than steatosis. PFOS might be the main cause of PFASs associated with hepatic fibrosis.
Ethical approval and consent to participate
Not applicable.
Consent for publication
Not applicable.
Author contributions
Conception and writing original draft preparation: Wenli Cheng; Methodology and formal analysis: Min Li, Luyun Zhang and Cheng Zhou; Data acquisition: Xinyu Zhang, Chenyu Zhu and Luyi Tan; Interpretation of the data: Hui Lin; Design of the work and funding acquisition: Wenjuan Zhang and Wenji Zhang. All authors provided critical revisions and approved the final manuscript.
Supplemental Material
Download MS Word (7.1 MB)Acknowledgements
Not applicable.
Disclosure statement
The authors declare that they do not have any conflicts of interest related to this study. This manuscript has been read and approved by all the authors and has not been submitted to or is not under consideration for publication elsewhere.
Data availability statement
Data will be made available on request.
Additional information
Funding
References
- Cheng W, Li M, Zhang L, et al. New roles of N6-methyladenosine methylation system regulating the occurrence of non-alcoholic fatty liver disease with N6-methyladenosine-modified MYC. Front Pharmacol. 2022;13:1.
- Liu J, Tian Y, Fu X, et al. Estimating global prevalence, incidence, and outcomes of non-alcoholic fatty liver disease from 2000 to 2021: systematic review and meta-analysis. Chin Med J . 2022;135(14):1682–14.
- Teng ML, Ng CH, Huang DQ, et al. Global incidence and prevalence of non-alcoholic fatty liver disease. Clin Mol Hepatol. 2023;29(Suppl):S32–S42.
- Schaider LA, Balan SA, Blum A, et al. Fluorinated compounds in U.S. Fast food packaging. Environ Sci Technol Lett. 2017;4(3):105–111.
- Sinclair E, Kim SK, Akinleye HB, et al. Quantitation of gas-phase perfluoroalkyl surfactants and fluorotelomer alcohols released from nonstick cookware and microwave popcorn bags. Environ Sci Technol. 2007;41(4):1180–1185.
- Hill PJ, Taylor M, Goswami P, et al. Substitution of PFAS chemistry in outdoor apparel and the impact on repellency performance. Chemosphere. 2017;181:500–507.
- Podder A, Sadmani A, Reinhart D, et al. Per and poly-fluoroalkyl substances (PFAS) as a contaminant of emerging concern in surface water: a transboundary review of their occurrences and toxicity effects. J Hazard Mater. 2021;419:126361.
- Houde M, De Silva AO, Muir DC, et al. Monitoring of perfluorinated compounds in aquatic biota: an updated review. Environ Sci Technol. 2011;45(19):7962–7973.
- Calafat AM, Wong LY, Kuklenyik Z, et al. Polyfluoroalkyl chemicals in the U.S. population: data from the national health and nutrition examination survey (NHANES) 2003–2004 and comparisons with NHANES 1999–2000. Environ Health Perspect. 2007;115(11):1596–1602.
- Jain RB, Ducatman A. Selective associations of recent low concentrations of perfluoroalkyl substances with liver function biomarkers: NHANES 2011 to 2014 data on US adults aged ≥20 years. J Occup Environ Med. 2019;61(4):293–302.
- Bassler J, Ducatman A, Elliott M, et al. Environmental perfluoroalkyl acid exposures are associated with liver disease characterized by apoptosis and altered serum adipocytokines. Environ Pollut. 2019;247:1055–1063.
- Qazi MR, Abedi MR, Nelson BD, et al. Dietary exposure to perfluorooctanoate or perfluorooctane sulfonate induces hypertrophy in centrilobular hepatocytes and alters the hepatic immune status in mice. Int Immunopharmacol. 2010;10(11):1420–1427.
- Behr AC, Plinsch C, Braeuning A, et al. Activation of human nuclear receptors by perfluoroalkylated substances (PFAS). Toxicol in Vitro. 2020;62:104700.
- Li X, Wang Z, Klaunig JE. The effects of perfluorooctanoate on high fat diet induced non-alcoholic fatty liver disease in mice. Toxicology. 2019;416:1–14.
- Huck I, Beggs K, Apte U. Paradoxical protective effect of perfluorooctanesulfonic acid against high-fat diet-induced hepatic steatosis in mice. Int J Toxicol. Sep/Oct2018;37(5):383–392.
- Chalasani N, Younossi Z, Lavine JE, et al. The diagnosis and management of non-alcoholic fatty liver disease: practice guideline by the American gastroenterological association, American association for the study of liver diseases, and American college of gastroenterology. Gastroenterology. 2012;142(7):1592–1609.
- Younossi ZM, Paik JM, Al Shabeeb R, et al. Are there outcome differences between NAFLD and metabolic-associated fatty liver disease? Hepatology. 2022;76(5):1423–1437.
- Castera L, Friedrich-Rust M, Loomba R. Noninvasive assessment of liver disease in patients with nonalcoholic fatty liver disease. Gastroenterology. 2019;156(5):1264–1281.e4.
- Barr RG, Ferraioli G, Palmeri ML, et al. Elastography assessment of liver fibrosis: society of radiologists in ultrasound consensus conference statement. Radiology. 2015;276(3):845–861.
- Siddiqui MS, Vuppalanchi R, Van Natta ML, et al. Vibration-controlled transient elastography to assess fibrosis and steatosis in patients with nonalcoholic fatty liver disease. Clin Gastroenterol Hepatol. 2019;17(1):156–163.e2.
- Zhang X, Heredia NI, Balakrishnan M, et al. Prevalence and factors associated with NAFLD detected by vibration controlled transient elastography among US adults: results from NHANES 2017-2018. PLOS One. 2021;16(6):e0252164.
- Liu J, Tan L, Liu Z, et al. The association between non-alcoholic fatty liver disease (NAFLD) and advanced fibrosis with blood selenium level based on the NHANES 2017-2018. Ann Med. 2022;54(1):2259–2268.
- Heredia NI, Zhang X, Balakrishnan M, et al. Physical activity and diet quality in relation to non-alcoholic fatty liver disease: a cross-sectional study in a representative sample of U.S. adults using NHANES 2017–2018. Prev Med. 2022;154:106903.
- Brosset E, Ngueta G. Exposure to per- and polyfluoroalkyl substances and glycemic control in older US adults with type 2 diabetes mellitus. Environ Res. 2023;216(Pt 3):114697.
- Bedogni G, Bellentani S, Miglioli L, et al. The fatty liver index: a simple and accurate predictor of hepatic steatosis in the general population. BMC Gastroenterol. 2006;6(1):33.
- Kotronen A, Peltonen M, Hakkarainen A, et al. Prediction of non-alcoholic fatty liver disease and liver fat using metabolic and genetic factors. Gastroenterology. 2009;137(3):865–872.
- Long MT, Pedley A, Colantonio LD, et al. Development and validation of the framingham steatosis index to identify persons with hepatic steatosis. Clin Gastroenterol Hepatol. 2016;14(8):1172–1180.e2.
- Sterling RK, Lissen E, Clumeck N, et al. Development of a simple noninvasive index to predict significant fibrosis in patients with HIV/HCV coinfection. Hepatology. 2006;43(6):1317–1325.
- EASL clinical practice guidelines on non-invasive tests for evaluation of liver disease severity and prognosis – 2021 update. J Hepatol. 2021;75(3):659–689.
- Anstee QM, Castera L, Loomba R. Impact of non-invasive biomarkers on hepatology practice: past, present and future. J Hepatol. 2022;76(6):1362–1378.
- Angulo P, Hui JM, Marchesini G, et al. The NAFLD fibrosis score: a noninvasive system that identifies liver fibrosis in patients with NAFLD. Hepatology. 2007;45(4):846–854.
- Ampuero J, Pais R, Aller R, et al. Development and validation of hepamet fibrosis scoring system-A simple, noninvasive test to identify patients with nonalcoholic fatty liver disease with advanced fibrosis. Clin Gastroenterol Hepatol. 2020;18(1):216–225.e5.
- Paluch AE, Bajpai S, Ballin M, et al. Prospective association of daily steps with cardiovascular disease: a harmonized meta-analysis. Circulation. 2023;147(2):122–131.
- Bobb JF, Claus Henn B, Valeri L, et al. Statistical software for analyzing the health effects of multiple concurrent exposures via Bayesian kernel machine regression. Environ Health. 2018;17(1):67.
- Kim SS, Meeker JD, Keil AP, et al. Exposure to 17 trace metals in pregnancy and associations with urinary oxidative stress biomarkers. Environ Res. 2019;179(Pt B):108854.
- Spinella R, Sawhney R, Jalan R. Albumin in chronic liver disease: structure, functions and therapeutic implications. Hepatol Int. 2016;10(1):124–132.
- Samuel VT, Shulman GI. Nonalcoholic fatty liver disease as a nexus of metabolic and hepatic diseases. Cell Metab. 2018;27(1):22–41.
- Murata S, Maruyama T, Nowatari T, et al. Signal transduction of platelet-induced liver regeneration and decrease of liver fibrosis. Int J Mol Sci. 2014;15(4):5412–5425.
- Rosen MB, Das KP, Rooney J, et al. PPARα-independent transcriptional targets of perfluoroalkyl acids revealed by transcript profiling. Toxicology. 2017;387:95–107.
- Fan Y, Li X, Xu Q, et al. Serum albumin mediates the effect of multiple per- and polyfluoroalkyl substances on serum lipid levels. Environ Pollut. 2020;266(Pt 2):115138.
- Gao K, Zhuang T, Liu X, et al. Prenatal exposure to per- and polyfluoroalkyl substances (PFASs) and association between the placental transfer efficiencies and dissociation constant of serum proteins-PFAS complexes. Environ Sci Technol. 2019;53(11):6529–6538.
- Schillemans T, Iszatt N, Remy S, et al. Cross-sectional associations between exposure to per- and polyfluoroalkyl substances and body mass index among European teenagers in the HBM4EU aligned studies. Environ Pollut. 2023;316(Pt 1):120566.
- Wang J, Zhang Y, Zhang W, et al. Association of perfluorooctanoic acid with HDL cholesterol and circulating miR-26b and miR-199-3p in workers of a fluorochemical plant and nearby residents. Environ Sci Technol. 2012;46(17):9274–9281.
- Liu D, Xiao Y, Evans BS, et al. Negative feedback regulation of fatty acid production based on a malonyl-CoA sensor-actuator. ACS Synth Biol. 2015;4(2):132–140.
- Darrow LA, Groth AC, Winquist A, et al. Modeled perfluorooctanoic acid (PFOA) exposure and liver function in a mid-Ohio valley community. Environ Health Perspect. 2016;124(8):1227–1233.
- Gallo V, Leonardi G, Genser B, et al. Serum perfluorooctanoate (PFOA) and perfluorooctane sulfonate (PFOS) concentrations and liver function biomarkers in a population with elevated PFOA exposure. Environ Health Perspect.2012;120(5):655–660.
- Borghese MM, Liang CL, Owen J, et al. Individual and mixture associations of perfluoroalkyl substances on liver function biomarkers in the Canadian health measures survey. Environ Health. 2022;21(1):85.
- Zare Jeddi M, Dalla Zuanna T, Barbieri G, et al. Associations of perfluoroalkyl substances with prevalence of metabolic syndrome in highly exposed young adult community residents-A cross-sectional study in Veneto region, Italy. IJERPH. 2021;18(3):1194.
- Lin CY, Wang C, Sung FC, et al. Association between serum per- and polyfluoroalkyl substances and thrombograms in young and middle-aged Taiwanese populations. Ecotoxicol Environ Saf. 2022;236:113457.
- Freberg BI, Haug LS, Olsen R, et al. Occupational exposure to airborne perfluorinated compounds during professional ski waxing. Environ Sci Technol. 2010;44(19):7723–7728.
- Jin C, Sun Y, Islam A, et al. Perfluoroalkyl acids including perfluorooctane sulfonate and perfluorohexane sulfonate in firefighters. J Occup Environ Med. 2011;53(3):324–328.
- EPA. Proposed PFAS National Primary Drinking Water Regulation. United States Environmental Protection Agency. Accessed April 20, 2023. https://www.epa.gov/sdwa/and-polyfluoroalkyl-substances-pfas.
- NIOSH. Per- and polyfluoroalkyl substances (PFAS). The National Institute for Occupational Safety and Health. Accessed April 20, 2023. https://www.cdc.gov/niosh/topics/pfas/default.html.