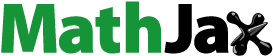
ABSTRACT
Objective
It was to assess the efficacy of preoperative guidance using cone beam computed tomography (CBCT) and the application of autologous concentrated growth factors (CGF) for implantation in maxillary teeth with inadequate bone mass.
Methods
An eigenvector-based multimodal elasticity algorithm was developed. Eighty patients with insufficient bone mass in the maxillary dental region were rolled into control group (n = 40, Bio-Oss bone powder treatment) and observation group (n = 40, autologous CGF and Bio-Oss bone powder treatment). The scale-invariant feature transform (SIFT) algorithm and the hierarchical attribute matching mechanism for elastic registration (HAMMER) were introduced, whose registration time and mutual information value were compared. Therapeutic outcomes of patients were also compared.
Results
The eigenvector-based multimodal elastic algorithm exhibited a significantly shorter registration time versus SIFT and HAMMER algorithms, and superior accuracy, sensitivity, and specificity (p < .05). Observation group demonstrated greater alveolar ridge elevation and bone augmentation versus control group, implying superior healing outcomes.
Conclusion
The feature point extraction algorithm is applicable for CBCT image processing in patients with insufficient maxillary bone mass. Autologous CGF exhibited superior therapeutic efficacy.
Introduction
Tooth loss adversely impacts masticatory function and facial aesthetics, often leading to psychological distress among patients (Gupta et al. Citation2019). The anatomical complexities associated with the maxillary sinus in the maxillary dental region further compound these challenges (Toy and Uslu Citation2020). Alveolar bone resorption and maxillary sinus pneumatization resulting from tooth loss contribute to insufficient alveolar bone in the maxillary dental zone (Lee, Jo, and Noh Citation2021). Additionally, osteoporosis may exacerbate difficulties in implant placement and restoration in this area (S. H. Wang et al. Citation2020). Consequently, implantation in cases of severe maxillary bone deficiency presents heightened complexities and risks. In recent years, advancements in implantation and restoration technologies have significantly improved. The widespread adoption of bioengineering materials, particularly the notable progress in osseointegration, has propelled the evolution of implantation techniques (Naujokat et al. Citation2020). With increasing modernization, the concept of dental implantation has gained widespread acceptance. Denture-based approaches for restoring dentition defects have been established as routine methods (Robau-Porrua et al. Citation2020).
Cone beam computed tomography (CBCT) stands out as one of the most commonly utilized methods for oral imaging, offering advantages such as real-time performance, high sensitivity, and ease of operation (Gaêta-Araujo et al. Citation2021; Woelber et al. Citation2018). Despite its merits, CBCT entails radiation exposure to the human body, necessitating careful consideration of its indications to mitigate potential radiation-related harm to patients. Presently, post-processing techniques are commonly employed to mitigate artifacts arising from repair materials in CBCT images, albeit at the cost of increased workload for medical personnel and diminished examination efficiency (McGuigan, Duncan, and Horner Citation2018). Effective image denoising and registration methods enable the accurate fusion of diverse information into a single image, thereby facilitating convenient and rapid observation of lesion structures from multiple perspectives by medical practitioners. The feature vector-based multimodal elastic algorithm, as an advanced technology, achieves efficient registration of multimodal data by extracting feature vectors of key features from images (Garcia Guevara et al. Citation2020). Its uniqueness lies in its ability to establish robust correspondences between different modalities, thereby enhancing the accuracy and reliability of image processing compared to traditional algorithms (Zhang et al. Citation2019). This algorithm not only possesses a shorter registration time but also demonstrates outstanding performance in medical imaging and other fields, providing a powerful tool for in-depth understanding and processing of multimodal images (Oyarzun Laura, Wesarg, and Sakas Citation2022).
Concentrated growth factors (CGF) are concentrates of growth factors derived from a patient’s own blood, including platelets, leukocytes, red blood cells, and abundant growth factors (Chen and Jiang Citation2020). During surgery, the patient’s blood can be transformed into CGF through simple centrifugation and gelation steps, forming an autologous blood clot. This clot is rich in growth factors such as platelet-derived growth factor, transforming growth factor-β, fibroblast growth factor, which play crucial regulatory roles in cell proliferation, differentiation, and healing processes (Lana et al. Citation2023). What distinguishes CGF is its rich content of fibrinogen, endowing it with remarkable biological activity and tissue compatibility. This autologous source of growth factor concentrate exhibits multifaceted potential applications in the field of oral implantation. Firstly, CGF can be utilized as a filler for bone defects, promoting bone tissue regeneration and growth (Huidrom et al. Citation2022). Secondly, owing to its potent healing-promoting effects, CGF demonstrates excellent performance in enhancing surgical success rates and shortening healing times, while its natural origin reduces the risk of immune rejection and lowers the incidence of surgical complications (Gaur et al. Citation2022). The utilization of autologous CGF has gained increasing popularity in current clinical practice (Hu et al. Citation2021; Y. H. Wang et al. Citation2021). Commonly employed materials for dental crown repair include metal alloys such as silver-palladium, cobalt-chromium, and nickel-chromium. However, these materials often lead to varying degrees of artifacts in tooth radiographic images, significantly compromising image quality and resolution. Consequently, diagnostic accuracy is adversely affected, hindering effective diagnosis and treatment (Yüce, Adalı, and Işık Citation2021). Despite these challenges, there remains a paucity of research on the combined application of CBCT and autologous CGF. Therefore, CBCT was introduced into dental implantation procedures to enhance image quality and augment its guidance capabilities.
In this study, patients with insufficient bone mass in the maxillary dental zone underwent dental implantation treatment utilizing autologous CGF in conjunction with Bio-Oss bone powder. The effectiveness of preoperative CBCT guidance and autologous CGF in implantation procedures for maxillary teeth with insufficient bone mass was evaluated. The research significance lies in the exploration of CBCT preoperative guidance facilitated by a feature point extraction algorithm in combination with autologous CGF for implantation in patients with insufficient bone mass in the maxillary dental zone. The primary objective of this study was to provide insights into implantation methods for patients with inadequate bone mass in the maxillary dental zone, while also offering guidance for the interpretation of CBCT images.
Materials and Methods
Research Objects
A total of 80 patients with insufficient bone mass in the maxillary dental area were selected as the research objects, as they received dental implantation treatment in the hospital from September 2020 to December 2020. Employing a randomized controlled trial methodology, the 80 patients were divided into two groups. 40 cases in the control group were treated with single Bio-Oss bone powder, and the other 40 cases in the observation group were given with autologous CGF as well as Bio-Oss bone powder. There were 22 males and 18 females in the observation group, aged 12–58 years old, with an average age of 32.09 ± 7.69 years old. They had the medical history of 3 months to 8 years, with an average medical history of 5.72 ± 1.89 years. The control group included 21 males and 19 females, and they were 13–59 years old with an average age of 31.78 ± 7.98 years old. The medical history of them lasted for 2 months to 9 years, with an average of 5.98 ± 1.78 years. The general data of patients were not statistically different between the two groups, thereby it was of comparability. This study was approved by the ethics board of XXXXX and was conducted in accordance with the Helsinki Declaration of 1975, as revised in 2013.
Patients meeting the following inclusion criteria were enrolled: individuals capable of providing comprehensive medical records and imaging data, exhibiting severe maxillary teeth atrophy, possessing normal occlusion and adequate repair space, and having not undergone head and neck radiotherapy within the past year. Informed consent was obtained from the patients and their families.
Exclusion criteria were also established, excluding individuals with complex vital organ diseases, infections or inflammation in the implantation area, or severely impaired occlusal function. Pregnant or postpartum women, as well as those unwilling to participate, were also excluded from the study.
Preoperative CBCT Imaging
CBCT imaging of maxillofacial area was performed on patients in both groups before surgery, A CBCT scanner was employed for maxillofacial imaging (National Medical Device Registration No.: 20163302530), in which all scans were acquired using New Tom CBCT (QRSY1 NEW7M 5 G, Cefla S.C., Italy). The QR-DVT 9000 NEWTOM software was used for analytical measurement and simulated implantation of implants. A simulated implant was constructed in the software, with the bone mass around the implant should be greater than 2 mm. The implant should be close to the anterior and posterior inferior walls of the maxillary sinus. The sagittal, axial, and coronal angles were measured. Scanning conditions included tube voltage 110 kV, tube current 3.77 mA, time of exposure 3.6 s, and layer thickness 0.3 mm. Two dental and maxillofacial radiologists with over five years of professional experience marked the software graphics to provide guidance on the implantation angle. In case of ambiguity, the final result was determined through mutual discussion between the two experts.
Lesion Localization Under Dual Attention-Based Cavitation Residual Classification Network
To reduce unnecessary data and enhance algorithm efficiency, a weighted averaging method was employed for grayscale processing of CBCT images. In a CBCT image, three channels were identified, wherein Channel A represented red, Channel B represented green, and Channel C represented blue. Given that CBCT image recognition is minimally reliant on color information, during the extraction of image features, the gradient information from the grayscale image was retained. Consequently, the image was converted into a single channel. It was assumed that R = G = B to obtain the original image after grayscale. The grayscale calculation method was shown in Equationequation (1)(1)
(1) .
The image denoising algorithm under coefficient classification was applied for image denoising. The flow was shown in .
The overcomplete binary wavelet was employed to adjust the filter length, facilitating multi-scale analysis and mitigating visual distortion resulting from nonlinear transformations. This two-dimensional overcomplete binary wavelet provided the CBCT image with three components: the overview coefficient, horizontal detail coefficient, and vertical detail coefficient. The overview coefficient was set to be Skf(a,b), the horizontal detail coefficient was W1kf(a,b), and the vertical detail coefficient was W2kf(a,b). The discrete binary wavelet transform of N-layer discrete image f(a,b) was shown as Equationequation (2)(2)
(2) .
X*[Y,Z] was defined to indicate that the two-dimensional sequence X was convolved with the one-dimensional filters Y and Z column-by-column and row-by-row, respectively. k represented the decomposition scale, M, N, and D stood for the low-pass filter, high-pass filter, and filter on the corresponding scale, respectively. Then three sub-bands could be expressed as Equationequation (3)(3)
(3) , Equationequation (4)
(4)
(4) , and Equationequation (5)
(5)
(5) , respectively.
The image reconstruction algorithm was described in Equationequation (6)(6)
(6) , where E and F were the reconstruction high-pass filters, and ^M was the reconstruction low-pass filter, which was the complex conjugate of the decomposition filter M.
As for the coefficient classification, each point (a0,b0) in the two-dimensional binary wavelet domain had a two-dimensional cone of influence on the scale, which was expressed as COI. The calculation method was shown in Equationequation (7)(7)
(7) , where O and P represented the half-support length of the wavelet mother function in the horizontal and vertical directions, respectively.
The two-dimensional COI-based operator Nk for any point (a0,b0) on the k-th scale was calculated according to Equationequation (8)(8)
(8) .
If a point exhibited a useful signal and possessed a Lipschitz exponent α ≥ 0, it satisfied Equationequation (9)(9)
(9) and was classified as a regular coefficient. Conversely, if α < 0, indicating an irregular coefficient, the point was deemed to represent noise.
Given the unknown noise distribution model of the CBCT image, an equation for estimating noise variance was necessary for layer-by-layer variance estimation. This noise variance estimation equation is denoted as Equationequation (10)(10)
(10) . D(a,b) meant a suitable small neighborhood centered on any point Wkf(a0,b0) on the sub-band.
Wiener filtering demonstrated superior efficacy in noise removal from images. Following filtering and denoising, the wavelet coefficients of the zero-mean normal distribution can be represented by Equationequation (11)(11)
(11) . c(a0,b0) and ^c(a0,b0) were the noise variances of the wavelet coefficient σn2 before and after denoising. The calculation method of the coefficient variance was shown in Equationequation (12)
(12)
(12) .
In image registration, a method was employed to adaptively select significant feature points for registration based on their importance. EquationEquation (13)(13)
(13) describes the calculation method for the importance level function. As the top-ranked points typically do not correspond one-to-one, the top N + 50 pixel points were chosen as the adaptive significant feature points of the floating image. Following the adaptive selection of significant feature points, the calculation of the importance of eigenvectors no longer necessitated consideration of interfering factors such as eigenvector combinations, eigenvector weight sets, and modal differences between different image modalities.
In the above equation, ei(x) was the i-th component of the eigenvector of the pixel x, n was the dimension of the eigenvector, and the weight wi stood for the importance of the i-th component of the eigenvector.
The flow of image registration was presented in .
Observation Indicators
The registration time and mutual information of the three algorithms were calculated. EquationEquation (14)
(14)
(14) showed the calculation method of mutual information, where H(J) and H(K) represented the entropy of images J and K, respectively. H(J,K) meant the joint entropy of the two images.
(14)
(14)
The mathematical expression of entropy was as Equationequation (15)
(15)
(15) . For an image, the expression of entropy was shown as Equationequation (16)
(16)
(16) . zi represented the total number of pixels with gray value i in the image R, J was the gray level of the image R, and pi was the probability of the occurrence of gray i.
(15)
(15)
(16)
(16)
(17)
(17)
Algorithm performance evaluation was as follows. The receiver operating characteristic curve (ROC) and the area under the ROC curve (AUC) were utilized to assess algorithm performance. The diagnostic performance of the model was evaluated by generating a confusion matrix and calculating accuracy, sensitivity, and specificity. The calculation of each metric is as follows:
(18)
(18)
(19)
(19)
(20)
(20)
Among these, TP represents true positive, FP stands for false positive, FN denotes false negative, and TN indicates true negative.
Surgical efficacy evaluation indicators mainly included the elevation of alveolar ridge, bone augmentation, bone density, and bone thickness of patients. The bone density and bone thickness just after surgery and one year after surgery were also counted.
Pink esthetic scores (PES) mainly consisted of seven indicators such as soft tissue level, color, shape, and texture. As a 3-stage scoring method was adopted, 0 point meant the worst and 2 points meant the best.
Additionally, the wound healing time and the number of patients experiencing primary and secondary healing were recorded.
Statistical Methods
SPSS 20.0 was applied for statistical analysis of the data with t test. The enumeration data were expressed as a rate (%), and p < .05 was considered to indicate the statistically significant differences.
Results
Type Distribution of Patients in Two Groups
A total of 80 patients were enrolled, comprising 28 with insufficient bone mass in the maxillary anterior zone and 52 with insufficient bone mass in the maxillary posterior zone. Within the observation group, there were 13 cases in the maxillary anterior zone and 27 in the maxillary posterior zone. Meanwhile, the control group consisted of 15 cases in the maxillary anterior zone and 25 in the maxillary posterior zone. The CBCT images of the maxillary dental zone are depicted in , where Figures illustrate the CBCT images of the maxillary posterior zone and the maxillary anterior zone, respectively.
Comparison of Registration Time and Mutual Information of Three Algorithms
presents a comparison of the registration time among the three algorithms for registering CBCT images of patients with insufficient bone mass in the maxillary dental zone. It is evident that the registration time of the eigenvector-based multimodal elasticity algorithm was significantly shorter than that of both the SIFT and HAMMER algorithms, with statistical significance (p < 0.05). illustrates a comparison of mutual information in CBCT image registration among the three algorithms. The mutual information value obtained with the eigenvector-based multimodal elasticity algorithm was notably higher than those achieved with the SIFT and HAMMER algorithms, with statistically significant differences (p < 0.05).
The Performance Comparison Among the Three Algorithms
The accuracy, sensitivity, and specificity assessed by the HAMMER algorithm were 66.28%, 67.15%, and 70.64%, respectively. For the SIFT algorithm, these metrics were 68.42%, 65.32%, and 69.54%, respectively. The Eigenvector-based Multimodal Elasticity algorithm achieved an accuracy, sensitivity, and specificity of 80.66%, 82.87%, and 97.62%, respectively. The performance of the Eigenvector-based Multimodal Elasticity algorithm significantly surpassed the other two methods (p < 0.05) ().
Comparison of Surgical Effects Between the Two Groups of Patients
presents a comparison of the surgical outcomes between the two groups of patients. Specifically, depicts alveolar ridge elevation, illustrates bone augmentation, while represent bone thickness and bone density, respectively, both immediately after surgery and one year post-surgery. From the figures, it is evident that the alveolar ridge elevation and bone augmentation observed in the observation group post-implantation were notably higher compared to those in the control group, with statistically significant differences (p < 0.05). There were no significant differences in bone thickness and bone density between patients immediately after surgical implantation. However, one year after implantation surgery, both bone thickness and bone density in the observation group were significantly greater than those in the control group, with statistically significant differences (p < 0.05).
Figure 7. Comparison of the surgical effect of the two groups on patients. (figures a, b, c, and d displayed the comparison of the alveolar ridge elevation, bone augmentation, bone thickness, and bone density, respectively. #P < 0.05 compared with those of the control group, *p < 0.05 compared with those at the end of implantation surgery.).

Comparison of PES Scores Between the Two Groups of Patients
In , the PES score of the patients in the observation group was 11.63, and the PES score of the control group was 10.73. The PES score of patients in the observation group was significantly higher than that of the control group, suggesting the difference was statistically significant (p < 0.05).
Comparison of the Healing Status of Patients
illustrates a comparison of the healing durations between the two patient groups, while presents a comparison of the distribution of healing types. The findings suggest that the mean healing duration in the observation group was 6.29 days, significantly shorter than that in the control group (9.13 days), with a statistically significant difference (p < .05). In terms of healing types, 45 patients in the observation group exhibited stage I healing, constituting 90% of the group, while 5 patients demonstrated stage II healing, accounting for 10%. In contrast, in the control group, 38 patients experienced stage I healing and 12 patients underwent stage II healing, representing 76% and 24% of the group, respectively. Notably, the difference in healing type distribution between the two groups was statistically significant (p < .05).
Discussion
Adequate bone mass in the implantation zone is a critical prerequisite for successful implantation repair. However, pathological factors and physiological stimulation can often lead to insufficient alveolar bone mass, thereby increasing the complexity of repair procedures (Rues et al. Citation2021). Bio-Oss bone powder, originating from animal bones, may potentially trigger immune reactions associated with contact with heterologous animal tissues. Additionally, due to its biological origin, some patients may encounter challenges related to the absorption of the bone powder, thus compromising the long-term stability postoperatively. CGF contain a significant concentration of fibrin and growth factors, predominantly comprising platelet-derived growth factors and vascular endothelial growth factors, which play crucial roles in promoting the healing and regeneration of bone tissues (Xu et al. Citation2019). Numerous studies have demonstrated that autologous CGF exerts a beneficial influence on wound healing, providing conducive conditions for accelerated wound recovery (Chen et al. Citation2018; W. Li et al. Citation2021). Specifically, CGF facilitates the activation of cytokine receptors within dental wounds, leading to extensive proliferation and differentiation of fibroblasts, thus enhancing the efficiency of healing (Özveri Koyuncu et al. Citation2020). Additionally, CGF has been shown to possess anti-inflammatory properties and to inhibit scar formation within wounds, thereby reducing postoperative pain, enhancing therapeutic outcomes, and improving patient satisfaction and comfort (X. Wang et al. Citation2021).
CBCT images offer several advantages, including excellent uniformity, high precision, low radiation dose, stability, and accuracy (Abdalla-Aslan et al. Citation2021). They enable fluoroscopic monitoring, volume imaging, and other procedures at the treatment site, and feature a robust online reset function (Jiang et al. Citation2019). Despite their high resolution for bone tissues, CBCT images often exhibit poor resolution for soft tissues. In instances where noise significantly impacts the clarity of weak edges, identifying and delineating target areas becomes challenging, hindering disease identification. Therefore, denoising and enhancing image quality are pressing concerns (Li et al. Citation2021). To address this, some researchers have proposed adaptive filtering denoising algorithms capable of removing Gaussian noise and impulse noise from medical images. However, implementing this algorithm into the core modules of imaging equipment presents challenges, making widespread adoption difficult (Hesar and Mohebbi Citation2021). Conversely, the image denoising algorithm based on coefficient classification demonstrated excellent denoising capabilities and rapid implementation, holding significant clinical promise. In recent years, advancements have greatly enhanced the quality and spatial resolution of CBCT images. Nevertheless, relying solely on a single medical image often yields suboptimal results, while incorporating multimodal images complicates the work of medical professionals. To address this, medical image registration emerges as a crucial solution (Rues et al. Citation2021). Image registration enables the integration of different images, allowing for the representation of information from multiple perspectives within a single image. This facilitates the provision of more intuitive and comprehensive structural and anatomical information (Abdel-Basset et al. Citation2017; Yang et al. Citation2019).
The study investigated the impact of combining autologous CGF with Bio-Oss bone powder versus using solely Bio-Oss bone powder on dental implantation outcomes in patients with insufficient bone mass in the maxillary teeth. Preoperative CBCT images were acquired, and a coefficient classification-based image denoising method was applied. Subsequently, image registration was performed using the eigenvector-based multimodal elasticity algorithm, with the comparative efficacy of different algorithms analyzed. The registration time of the multimodal elasticity algorithm was notably shorter compared to both the SIFT and HAMMER algorithms. Additionally, the mutual information obtained with the multimodal elasticity algorithm surpassed that of both the SIFT and HAMMER algorithms. These differences were statistically significant. Furthermore, the performance of the multimodal elasticity algorithm is significantly superior to the other two algorithms (p < 0.05). The utilization of the multimodal elasticity algorithm offered enhanced image recognition and facilitated improved image registration. Following treatment, the observation group exhibited significantly greater alveolar ridge elevation and bone augmentation compared to the control group. Moreover, one year post-implantation surgery, the observation group demonstrated significantly higher bone thickness and bone density than the control group. In addition, the observation group showed significantly higher PES, shorter healing duration, and a notably higher rate of stage I healing compared to the control group, with statistically significant differences (p < 0.05). These findings underscored the superior application efficacy and healing outcomes associated with autologous CGF in conjunction with Bio-Oss bone powder, thereby supporting its widespread utilization in the treatment of patients with insufficient maxillary bone mass.
In summary, this study introduced a multi-modal elastic algorithm based on feature vectors, which, compared to traditional SIFT and HAMMER algorithms, achieves superior results in CBCT image processing. Furthermore, compared to treatment with Bio-Oss bone powder alone, combining Bio-Oss bone powder with autologous CGF treatment can further improve efficacy, demonstrating practical significance. However, this study is limited to a sample size of 80 patients, potentially restricting the broad generalization of the research findings. Additionally, while the one-year post-implantation observations are promising, inadequate long-term follow-up data hinder a comprehensive assessment of treatment durability and stability. Future endeavors should involve enlarging the sample size, conducting more extensive experimental validation to ensure the reliability and stability of the multi-modal elastic algorithm based on feature vectors across diverse patient populations. Moreover, multi-center, multi-institutional studies could further validate the universality and applicability of the research findings.
Conclusion
The eigenvector-based multimodal elasticity algorithm demonstrated significant utility in CBCT image registration, characterized by reduced registration time and enhanced mutual information value. Its superior performance underscores its potential for clinical application. Furthermore, implantation using autologous CGF combined with Bio-Oss bone powder exhibited favorable therapeutic outcomes in patients with insufficient bone mass in the dental area, facilitating wound healing and providing an effective implantation approach for those with insufficient bone mass in the maxillary dental region. However, a limitation of this study was its small sample size, highlighting the need for future research to expand the sample size for validation purposes.
Disclosure statement
No potential conflict of interest was reported by the author(s).
References
- Abdalla-Aslan, R., N. Keshet, Y. Zadik, D. J. Aframian, and C. Nadler. 2021. Standardization of terminology, imaging features, and interpretation of CBCT sialography of major salivary glands: A clinical review. Quintessence Int. July 20. 52(8):728–19. PMID: 34076380. doi: 10.3290/j.qi.b1492217
- Abdel-Basset, M., A. E. Fakhry, I. El-Henawy, T. Qiu, and A. K. Sangaiah. 2017. Feature and intensity based medical image registration using particle swarm optimization. Journal of Medical Systems. November 3. 41(12):197. PMID: 29098445. doi: 10.1007/s10916-017-0846-9
- Chen, J., and H. Jiang. 2020, Jun. A comprehensive review of concentrated growth factors and their novel applications in facial reconstructive and regenerative medicine. ( Epub 2020. PMID: 31970453) Aesthetic Plastic Surgery. January 22. 44 (3):1047–57. doi: 10.1007/s00266-020-01620-6
- Chen, X., J. Wang, L. Yu, J. Zhou, D. Zheng, and B. Zhang. 2018. Effect of concentrated growth factor (CGF) on the promotion of osteogenesis in bone marrow stromal cells (BMSC) in vivo. Scientific Reports. April 12. 8(1):5876. PMID: 29651154; PMCID: PMC5897572. doi: 10.1038/s41598-018-24364-5
- Gaêta-Araujo, H., A. F. Leite, K. F. Vasconcelos, and R. Jacobs. 2021. Two decades of research on CBCT imaging in DMFR - an appraisal of scientific evidence. Dento maxillo facial radiology. May 1. 50(4):20200367. Epub 2021 Feb 15. PMID: 33555198; PMCID: PMC8077993. doi: 10.1259/dmfr.20200367
- Garcia Guevara, J., I. Peterlik, M. O. Berger, and S. Cotin. 2020. Elastic registration based on compliance analysis and biomechanical graph matching. ( Epub 2019 Sep 23. PMID: 31549328) Annals of Biomedical Engineering. Jan. 48 (1):447–62. doi: 10.1007/s10439-019-02364-4
- Gaur, S., A. Chugh, K. Chaudhry, A. Bajpayee, G. Jain, V. K. Chugh, P. Kumar, and S. Singh. 2022. Efficacy and safety of concentrated growth factors and platelet- rich fibrin on stability and bone regeneration in patients with immediate dental implants: A randomized controlled trial. ( PMID: 35904836) The International Journal of Oral & Maxillofacial Implants. July-August. 37 (4):784–92. doi: 10.11607/jomi.8924
- Gupta, S., R. Sabharwal, J. Nazeer, L. Taneja, B. K. Choudhury, and S. Sahu. 2019. Platform switching technique and crestal bone loss around the dental implants: A systematic review. ( PMID: 30729925; PMCID: PMC6380118) Annals of African Medicine. January-March. 18 (1):1–6. doi: 10.4103/aam.aam_15_18
- Hesar, H. D., and M. Mohebbi. 2021. An adaptive Kalman filter bank for ECG denoising. ( Epub 2021 Jan 5. PMID: 32224468) IEEE Journal of Biomedical and Health Informatics. January. 25 (1):13–21. doi: 10.1109/JBHI.2020.2982935
- Huidrom, E., V. Srivastava, A. Meenawat, A. Srivastava, Y. S. Khan, and R. Shahni. 2022. Evaluation of the efficacy of concentrated growth factor along with bovine-derived xenograft and collagen membrane in the treatment of degree II mandibular molar furcation defect - a clinicoradiographic study. ( Epub 2022 Mar 1. PMID: 35321290; PMCID: PMC8936011) Journal of Indian Society of Periodontology. March-April. 26 (2):130–36. doi: 10.4103/jisp.jisp_44_21
- Hu, M., Y. Zhong, S. Xie, H. Lv, and Z. Lv. Fuzzy system based medical image processing for brain disease prediction. Frontiers in Neuroscience 15:714318. doi: 10.3389/fnins.2021.714318 PMID: 34393718; PMCID: PMC8361453. 2021 Jul 30
- Jiang, Z., Y. Chen, Y. Zhang, Y. Ge, F. F. Yin, and L. Ren. 2019. Augmentation of CBCT reconstructed from under-sampled projections using deep learning. ( Epub 2019 Apr 23. PMID: 31021791; PMCID: PMC6812588) IEEE Transactions on Medical Imaging. November. 38 (11):2705–15. doi: 10.1109/TMI.2019.2912791
- Lana, J. F., J. Purita, P. A. Everts, P. A. T. de Mendonça Neto, D. de Moraes Ferreira Jorge, T. Mosaner, S. C. Huber, G. O. M. Azzini, L. F. da Fonseca, M. Jeyaraman, et al. 2023. Platelet-rich plasma power-mix gel (ppm)-an orthobiologic optimization protocol rich in growth factors and fibrin. Gels. July 7. 9(7):553. doi: 10.3390/gels9070553 PMID: 37504432; PMCID: PMC10379106.
- Lee, H., M. Jo, and G. Noh. 2021. Biomechanical effects of dental implant diameter, connection type, and bone density on microgap formation and fatigue failure: A finite element analysis. ( Epub 2020 Nov 22. PMID: 33308905) Computer Methods and Programs in Biomedicine. Mar. 200:105863. doi: 10.1016/j.cmpb.2020.105863
- Li, W., F. Wang, F. Dong, Z. Zhang, P. Song, H. Chen, J. Wang. 2021. CGF membrane promotes periodontal tissue regeneration mediated by hUcmscs through upregulating TAZ and osteogenic differentiation genes. Stem Cells Int. August 4; 2021:6644366. doi: 10.1155/2021/6644366 PMID: 34394357; PMCID: PMC8360720.
- Li, Y., J. Zhao, Z. Lv, and Z. Pan. 2021. Multimodal medical supervised image fusion method by CNN. Frontiers in neuroscience 15:638976. doi: 10.3389/fnins.2021.638976 PMID: 34149344; PMCID: PMC8206541. June 2
- McGuigan, M. B., H. F. Duncan, and K. Horner. 2018. An analysis of effective dose optimization and its impact on image quality and diagnostic efficacy relating to dental cone beam computed tomography (CBCT). Swiss Dent J. April 16. 128(4):297–316. PMID: 29589667. doi: 10.61872/sdj-2018-04-395
- Naujokat, H., Y. Açil, S. Harder, M. Lipp, F. Böhrnsen, and J. Wiltfang. 2020. Osseointegration of dental implants in ectopic engineered bone in three different scaffold materials. ( Epub 2019 May 1. PMID: 31053519) Int J Oral Maxillofac Surg. January. 49 (1):135–42. doi: 10.1016/j.ijom.2019.04.005
- Oyarzun Laura, C., S. Wesarg, and G. Sakas. 2022. Graph matching survey for medical imaging: On the way to deep learning. ( Epub 2021 Jul 1. PMID: 34216788) Methods. June. 202:3–13. doi: 10.1016/j.ymeth.2021.06.008
- Özveri, Koyuncu B., Işık G, Özden Yüce M, Günbay S, and Günbay T. 2020. Effect of concentrated growth factor (CGF) on short-term clinical outcomes after partially impacted mandibular third molar surgery: A split-mouth randomized clinical study. ( Epub 2019 Jul 9. PMID: 31299343) J Stomatol Oral Maxillofac Surg. April. 121 (2):118–23. doi: 10.1016/j.jormas.2019.07.002
- Robau-Porrua, A., Y. Pérez-Rodríguez, L. M. Soris-Rodríguez, O. Pérez-Acosta, and J. E. González. 2020. The effect of diameter, length and elastic modulus of a dental implant on stress and strain levels in peri-implant bone: A 3D finite element analysis. Bio-Medical Materials and Engineering 30 (5–6):541–58. doi: 10.3233/BME-191073 PMID: 31903978
- Rues, S., M. Schmitter, S. Kappel, R. Sonntag, J. P. Kretzer, and J. Nadorf. 2021. Effect of bone quality and quantity on the primary stability of dental implants in a simulated bicortical placement. ( Epub 2020 Jul 10. PMID: 32651646; PMCID: PMC7878229) Clinical Oral Investigations. March. 25 (3):1265–72. doi: 10.1007/s00784-020-03432-z
- Toy, V. E., and M. O. Uslu. 2020. Evaluation of long-term dental implant success and marginal bone loss in postmenopausal women. ( PMID: 32031087) Nigerian Journal of Clinical Practice. February. 23 (2):147–53. doi: 10.4103/njcp.njcp_295_19
- Wang, Y. H., G. Li, R. H. Ma, Y. P. Zhao, H. Zhang, J. H. Meng, C. C. Mu, C. K. Sun, and X. C. Ma. 2021. Diagnostic efficacy of CBCT, MRI, and CBCT-MRI fused images in distinguishing articular disc calcification from loose body of temporomandibular joint. ( Epub 2020 Aug 12. PMID: 32785850) Clinical Oral Investigations. April. 25 (4):1907–14. doi: 10.1007/s00784-020-03497-w
- Wang, S. H., Y. W. Shen, L. J. Fuh, S. L. Peng, M. T. Tsai, H. L. Huang, and J. T. Hsu. 2020. Relationship between cortical bone thickness and cancellous bone density at dental implant sites in the jawbone. Diagnostics (Basel). September 17. 10(9):710. doi: 10.3390/diagnostics10090710 PMID: 32957724; PMCID: PMC7555203.
- Wang, X., G. Wang, X. Zhao, Y. Feng, H. Liu, and F. Li. 2021. Short-term evaluation of guided bone reconstruction with titanium mesh membranes and CGF membranes in immediate implantation of anterior maxillary tooth. BioMed Research International 2021:4754078. doi: 10.1155/2021/4754078 PMID: 34869763; PMCID: PMC8635880. November 24
- Woelber, J. P., J. Fleiner, J. Rau, P. Ratka-Kruger, and C. Hannig. 2018. Accuracy and usefulness of CBCT in periodontology: A systematic review of the literature. ( PMID: 29447324) The International Journal of Periodontics & Restorative Dentistry. March/April. 38 (2):289–97. doi: 10.11607/prd.2751
- Xu, F., L. Qiao, Y. Zhao, W. Chen, S. Hong, J. Pan, and B. Jiang. 2019. The potential application of concentrated growth factor in pulp regeneration: An in vitro and in vivo study. Stem Cell Research & Therapy. May 20. 10(1):134. PMID: 31109358; PMCID: PMC6528367. doi: 10.1186/s13287-019-1247-4
- Yang, T., Q. Tang, L. Li, J. Song, C. Zhu, and L. Tang. 2019. Nonrigid registration of medical image based on adaptive local structure tensor and normalized mutual information. ( Epub 2019 May 23. PMID: 31124248; PMCID: PMC6560247) Journal of Applied Clinical Medical Physics. June. 20 (6):99–110. doi: 10.1002/acm2.12612
- Yüce, MO., Adalı E, and Işık G. 2021. The effect of concentrated growth factor (CGF) in the surgical treatment of medication-related osteonecrosis of the jaw (MRONJ) in osteoporosis patients: A randomized controlled study. ( Epub 2021 Jan 3. PMID: 33392802) Clinical Oral Investigations. July. 25 (7):4529–41. doi: 10.1007/s00784-020-03766-8
- Zhang, X., J. Wang, T. Wang, X. Ji, Y. Shen, Z. Sun, and X. Zhang. 2019. A markerless automatic deformable registration framework for augmented reality navigation of laparoscopy partial nephrectomy. ( Epub 2019 Apr 23. PMID: 31016562) International Journal of Computer Assisted Radiology and Surgery. August. 14 (8):1285–94. doi: 10.1007/s11548-019-01974-6