Abstract
Objective
To describe the mental models people hold about the COVID-19 pandemic, with a focus on how they understand the factors that drive the spread of COVID-19 and what kind of beliefs are associated with these models.
Design
In a series of three studies (total N = 461), we asked participants to identify factors that are relevant for COVID-19 proliferation (Study 1a), rate the importance of factors (Study 1 b), and create a mental model of how these factors relate to virus spread by employing a validated tool for mental model elicitation (Study 2). Main outcome measures: inclusion and centrality of factors in mental models of COVID-19 infection spread.
Results
Mitigation measures issued by government, adherence to measures, and virus characteristics were most strongly represented in participants’ mental models. Participants who perceived measures as appropriate or who experienced more control and more worry over the spread of the virus created more complex models compared to participants who were less satisfied with measures or who felt lower control and less worry.
Conclusion
These findings suggest that people are able to create sensible mental models of virus transmission but may appreciate transparent communication to comprehend the bigger picture behind the governmental mitigation strategy.
The outbreak of COVID-19 is unprecedented in terms of the global spread of infection and the resultant morbidity and burden on health care systems as well as the economic and social implications thereof (WHO, Citation2021). The scale of the pandemic has called for the implementation of severe mitigation measures aimed at preventing the spread of COVID-19. COVID-19 is mainly transmitted when people breathe in air contaminated by droplets and small airborne particles containing the virus. Infected people exhale those particles as they talk, cough, or sneeze. The risk of transmission is highest when people are in close proximity, but particles can also be inhaled over longer distances, particularly indoors (Wang et al., Citation2021). Accordingly, to reduce the chances of infection, governments globally were quick to encourage or mandate a range of preventive measures, including hygiene behaviors (e.g. handwashing, covering coughs and sneezes, ventilating indoor spaces), wearing face masks in public, and limiting social interactions (e.g. keeping distance from others, avoiding crowded places, staying at home, quarantining/self-isolating), and getting vaccinated (Lee et al., Citation2021). Most of these measures relate to people changing their own behavior or involve regulations that may deeply affect their personal lives (Margraf et al., Citation2020).
In view of the exceptional impact on the daily lives of everyone, including those who have not been directly affected by the virus, it is important to get a better understanding of how people perceive the pandemic with a particular emphasis on how they think about the factors that drive the spread of COVID-19 (Bogg & Milad, Citation2020). Whilst such insights are valuable on their own as they may clarify how people look at a complex phenomenon, this information is also important because it may eventually contribute to more targeted communication about strategies to curb the pandemic (Morgan et al., Citation2002). Indeed, public support for drastic public health measures depends on their alignment with people’s mental model of the situation (Leventhal et al., Citation1984). The World Bank has identified mental models as a strong leverage point authorities should consider when dealing with complex problems, such as a response to the COVID-19 pandemic (World Bank, Citation2014; cf. Greenhalgh, Citation2021; Hovmand et al., Citation2021; Michie et al., Citation2020).
Here, we set out to document people’s mental models of the pandemic, in particular their ideas on what causes the spread of COVID-19. We report on a series of studies that were conducted halfway through 2021, when the pandemic was underway for more than a year and people had been exposed to ample information about the COVID-19 virus through a variety of communication channels, including releases from governmental and public health authorities, opinions voiced by experts and laypeople on television shows and in newspapers, and social media posts by different groups and individuals.
Mental models are internal representations of external reality that people use to make sense of the world around them (Johnson-Laird, Citation1983; Wood et al., Citation2017). Because of cognitive limitations, people are unable to represent every detail that may be found in reality. Therefore, a mental model is by definition a simplified representation that allows people to explain ‘how things work’ (Johnson-Laird, Citation1983; Morgan et al., Citation2002). Mental models are construed based on experience, values, and beliefs (Biggs et al., Citation2011), influence how people process and store information (Johnson-Laird, Citation2010), and determine their attitudes, decisions, and behavior (Biggs et al., Citation2011; Goldberg et al., Citation2020; Güss & Robinson, Citation2014).
Many studies have examined what kind of beliefs people have about the COVID-19 pandemic and how it influences their behavior (e.g. Attema et al., Citation2021; Bostrom, Böhm, Hayes, & O’Connor, Citation2020; Pennycook et al., Citation2022; Van Stekelenburg et al., Citation2021; Vitriol & Marsh, Citation2021). However, beyond investigating these kinds of isolated beliefs there is no research examining people’s full mental models about COVID-19 proliferation. A better understanding of how people perceive the pandemic as a more or less complex system has the advantage of gaining more insight into their look at how the interaction of factors drive the spreading of COVID-19. Mapping the bigger picture of drivers of COVID-19 can eventually contribute to better communication about preventive measures in a way that aligns with people’s understanding of the pandemic.
Mental models exist within the mind and are therefore not available for direct measurement. A variety of elicitation techniques have been developed in different fields of research, including risk communication (Lowe & Lorenzoni, Citation2007) and climate change (Bostrom et al., Citation2012). Elicitation may either be indirect, with mental models being inferred from interviews with participants or direct, with participants actively creating a visual representation of their model themselves (Jones et al., Citation2011). Direct elicitation has the advantage of participants being able to verify their understanding of a given issue (Jones et al., Citation2011). This method presents people with a number of factors (presented in words or symbols), inviting them to arrange these factors and their connections into a cognitive map (Jones et al., Citation2011; Van den Broek, Klein et al., Citation2021). Individual mental models can be easily aggregated when participants are provided with the same set of factors that is typically derived from interviews, surveys or literature review (Aminpour et al., Citation2020).
Current studies. The main objective of our research is to describe what kind of mental models participants hold about the COVID-19 pandemic, with a particular focus on how they understand the factors that drive the spread of the virus. We designed three consecutive studies to gain insight into the mental models that people hold. First, we listed the factors that people name in response to an open undirected question about factors that contribute to the spread of COVID-19 and validated the list of factors in an online survey with a larger sample. Next, we documented mental models of COVID-19 proliferation by presenting these factors in M-tool, a validated instrument for direct elicitation of mental models (Van den Broek, Klein et al., Citation2021). Next to mapping mental models, we also examined how mental models relate to specific COVID-19 beliefs, including concern about getting infected, worrying about COVID-19, trust in government and appropriate mitigation measures, and personal or general control over the spread of COVID-19. These beliefs have been shown to influence adherence to mitigation measures in previous studies (Attema et al., Citation2021; Van Stekelenburg et al., Citation2021; Vitriol & Marsh, Citation2021). All studies involved samples from the Netherlands and were conducted halfway through 2021. As in many countries, measures were rapidly changing over the course of the pandemic. Nevertheless, basic measures remained similar over time as demonstrated in te main message communicated to the public stating: ‘Stop the spread of coronavirus. We’ll only get coronavirus under control if everyone does the following: stay at home as much as possible, keep your distance, wash your hands frequently, cough and sneeze into the crook of your elbow, use paper tissues and discard them immediately, and avoid shaking hands’ (government.nl/coronavirus). At the time of study, a partial lockdown with curfew was in place, testing and self-isolation when experiencing symptoms and quarantine after visiting a high-risk country or having been in contact with an infected person were required as were wearing face masks in public places and public transport. Next to the aforementioned basic measures, restricting social contacts by working from home and avoiding crowded places were strongly advised while vaccines became available for large parts of the population (www.rivm.nl/gedragsonderzoek/tijdlijn-maatregelen-covid).
Ethics Statement. Ethical approval for the studies was obtained from Ethics Committee of the Faculty of Social and Behavioral Sciences of Utrecht University, filed under number 21-0201. Data are available from the authors upon request.
Study 1
In two pilot studies, we first determined what kind of factors people associate with COVID-19 proliferation. Study 1a reports on the free elicitation of factors to generate the terms people use to describe these factors; Study 1 b assesses the importance of a selection of factors identified in Study 1a. The objective of both studies is to document factors that people can use when making their mental models of COVID-19 in Study 2, a procedure that is generally recommended when using direct elicitation of mental models (Aminpour et al., Citation2020).
Study 1a. Elicitation of factors associated with spread of COVID-19
Participants and procedure
This study aims to map factors that people spontaneously list in response to the question ‘Which factors contribute to the spread of COVID-19?’. To do so, we presented this question in person (either orally or in writing) to a convenience sample of 91 participants (46% female, 46% male, 8% missing information on gender), aged 18–81 years (M = 39.63, SD = 7.05) who were recruited on social media or via flyers; participants were not reimbursed. The sample was diverse in terms of education (25% lower education level, 23% medium level and 52% higher level; according to the categorization by Statistics Netherlands); 17% had a non-western background. Data were collected in March 2021, when the Netherlands upheld relatively stringent measures to prevent spread of COVID-19 (stringency level of 75 on a 0–100 scale according to the Oxford Stringency Index; https://ourworldindata.org/grapher/covid-stringency-index?tab=chart&country=∼NLD). These measures comprised, for example, the advice to wear mouth masks and to keep a distance in public places. More stringent measures included a curfew from 9 pm onwards and the partial lockdown of schools and outdoor sports (for a complete overview, see www.rivm.nl/gedragsonderzoek/tijdlijn-maatregelen-covid).
Results and discussion
On average, participants mentioned 4.8 factors (range 2–8 factors) with most participants (82%) mentioning a maximum of five factors. The majority of participants indicated that they had a good understanding of what the question was about, with the exception of a few participants who considered the question difficult to answer. Responses varied in detail: most of them consisted of one or two words (e.g. ‘vaccination’ or ‘hand washing’), whereas a minority contained more detailed information (e.g. ‘don’t sneeze right in front of you but into your elbow when you have a cold or sneeze into your hands and then wash your hands’). Responses were coded according to their main content, with all authors making individual categorizations that were subsequently compared; the few discrepancies that arose were resolved by discussion. The final categorization is shown in .
Table 1. Categories of factors, ordered by frequency (N = 91 participants).
shows that there is a large variety of factors that people consider to be important contributors to the spread of COVID-19. Willingness or motivation to comply with the rules in general is by far the largest category and was often accompanied by critical comments about other people who seem not to care about the pandemic. Together with a few explicit responses about lack of solidarity, these concerns about other people not following the rules are thus considered the main driver of spreading the virus. Next to low adherence, a substantial part of the responses echoes public health communication about preventive measures, including reducing social contact, keeping a distance, wearing face masks, and vaccination. Responses relating to social contact comprise a compilation of factors that either emphasize the gist of the rules (avoid social contact), specific elements of social contact (limit the number of home visits), the need for social contact, or the difficulties associated with avoiding crowds. Compared to social contact, other important ingredients of COVID-19 prevention policies - hand hygiene, testing, and isolation - were mentioned to a lesser extent. A minority of factors related to virological and epidemiological aspects of the pandemic, including prevalence and virulence of the virus and ventilation issues. Next to factors relating to key prevention policies, a substantial factor (named 12 times) relates to boosting your immunity by adopting a healthy lifestyle (e.g. ‘eating lots of vegetables’), which was not part of public health communication as a strategy to mitigate COVID-19 proliferation.
Factors that emerged in this first study provide insight into how people understand the spread of COVID-19. From these factors, we selected 22 that were mentioned most frequently (>3 times). Within eight factors that comprised heterogenous answers, we further distinguished between what people can do themselves (or are supposed to do themselves) and being provided with the opportunity to do so. For example, we differentiated between willingness to test or to get vaccinated and the availability of tests and vaccines; and between working from home and the employer taking care of safe working arrangements. Moreover, we differentiated between different types of social contact (group gatherings, parties, home visits, meeting many different people). As a result, we composed a set of 30 factors for further investigation in Study 1 b.
Study 1b. Assessing the importance of factors
Participants and procedure
Based upon the results from Study 1a, we employed a list of 30 factors that were presented in an online survey with the question of how much these factors contributed to the spread of COVID-19 on a 1 (‘not at all’) to 5 (‘very much’) scale (‘In the following, we present you with a list of factors that may influence COVID-19 spread. Please, indicate for each factor how much it contributes to COVID-19 spread’.). The survey was filled out by 223 participants (66% female) with a mean age of 21.91 years (SD = 1.02; range 19–73 years); the majority of participants had a high education level (72% university degree) and 8% had a non-Western background. Participants were recruited through convenience sampling on social media or via flyers; they were not reimbursed for participation. Data were collected in May 2021, when the Netherlands upheld relatively stringent measures to prevent the spread of COVID-19 (stringency level of 67.59 on a 0–100 scale according to the Oxford Stringency Index).
Results and discussion
shows the results and reveals that no factors were considered unimportant with a minimum mean of 2.96. Considering the factors that were on average rated as very important (means between 4 and 5), four central themes emerged: avoiding or minimizing social contact (stay at home in case of symptoms, group gatherings, parties, and meeting many different people), notions about the virus (virulence and virus mutations), public health policies (availability of vaccines and mitigation measures), and willingness to adhere to public health policies (willingness to get vaccinated and to get tested). Given the importance gradient, with no clear cut-off point, we decided to use the 15 factors that were rated as most important (> 3.8) to elicit mental models in Study 2, so as to balance the need for a complete overview of factors with a reasonable number of factors that people can handle in defining their mental model (Van den Broek, Klein et al., Citation2021). It should be noted that the factors we decided to retain implicate a strong focus on social contact. This may seem a bit unbalanced as other factors that are considered important to mitigate the pandemic are not represented in our final set. However, this selection is justifiable in case of mental model research since the factors are supposed to represent salient concepts of the target group. Whereas, for instance, wearing face masks or hand hygiene (that are absent from our list) are important elements in COVID-19 proliferation from a professional point of view, apparently these factors are less prominent in people’s minds when asked about the spread of COVID-19 – which may suggest that these factors are considered to have a milder impact on their lives.
Table 2. Mean (SD) ratings of importance of factors contributing to the spread of COVID-19, ordered by importance (N = 223).
Study 2
The objective of this study was to map the mental models people have about factors that drive COVID-19 proliferation and how these factors relate to each other. We also examined how these mental models are associated with specific COVID-19 beliefs.
Method
Participants and procedure
Participants were recruited through convenience sampling, advertising the study online (social media and university website) and offline (posters and flyers) to complete their mental model online. Additionally, 40 participants were recruited to complete their mental model on a tablet in the presence of a research assistant for validation purposes. Participants were not reimbursed. A total of 538 participants started the study, with 157 (29%) completing it. From this sample, the data of ten participants were excluded from analysis because of missing survey data (N = 6) or incomplete mental model data (N = 4). The final sample consisted of 147 participants (62% women, 37% men, 1% non-binary) between 17 and 80 years old (M = 36.51, SD = 13.51). The sample included primarily highly educated participants (3% lower education level, 7% medium education level, 90% higher education level). All data were collected in July and August 2021, when the Netherlands upheld relatively mild measures to prevent the spread of COVID-19 with stringency levels varying from 35.11 (July) to 41.67 (August) on a 0–100 scale according to the Oxford Stringency Index. This change in strategy comprised that the partial lockdown and the curfew were lifted.
Mapping of mental models
To map mental models, we used M-Tool that has been designed to assess mental models by direct elicitation with a fixed set of factors (Van den Broek, Klein et al., Citation2021; Van den Broek, Luomba, et al., Citation2021). Participants were asked to map the ‘factors affecting the spread of COVID-19’ by using the 15 factors with the highest importance ratings that were derived from Study 1 b. Factors were presented as icons with accompanying verbal description (see Appendix). Before completing the mental model, they were introduced to the procedure with a sample mental model of an issue unrelated to COVID-19 they had to replicate themselves (i.e. a model of factors related to happiness). Next, the 15 factors were explained by audio descriptions of the icons (, Panel A). Subsequently, the mapping screen was presented with audio instructions informing participants to select icons they considered relevant, move them to the canvas and connect them with arrows to map their mental model (, Panel B). The icons (representing the factors) were presented on the left panel, the mapping canvas in the middle, and the target variable of COVID-19 proliferation on the right side, to focus the mental model on the key issues and connections (Jetter & Kok, Citation2014). The right panel displayed three types of arrows that participants could use to connect the icons, varying in width to display their relative influence. It was emphasized that participants should only select icons they considered relevant, but there was no limit on the number of icons they could use. After completing the model ( Panel C shows an example), participants submitted their final model.
Validation
The 40 participants who completed their model in the presence of a research assistant were asked about their experiences when using M-Tool to map their model. Comments (N = 8) included missing the option to indicate the valence of the connections (positive or negative influences; N = 7) or moderation effects (N = 1). In addition, all participants (both online and offline) were asked whether the list of 15 factors was complete according to their personal view. A quarter of the participants mentioned missing factors, which included slight variations of factors already available in M-Tool or factors mentioned in Study 1 that were not considered the most important by participants, such as closing schools or shops and wearing face masks. Participants completed their model in six minutes on average (M = 6.15, SD = 4.38).
Other measures
After completing the cognitive map of their mental model, participants filled out questions about demographics (age, gender, education) and six questions about COVID-19 beliefs that have been shown to influence compliance with mitigation measures (Attema et al., Citation2021; Van Stekelenburg et al., Citation2021; Vitriol & Marsh, Citation2021), including concern about getting infected, worrying about COVID-19, trust in governmental approach of the pandemic, their judgment of appropriate mitigation measures, and personal and general control over the spread of COVID-19 (see for specific questions). All questions were answered on 1 to 5 Likert scales. Participants also indicated their infection status.
Table 3. Means (SD) of COVID-19 beliefs on a 1–5 scale (N = 147).
Analyses
First, we examined which factors and connections were included in the model and how their importance was rated. To this end, a weight (from 1 = weak influence to 3 = strong influence) was assigned to reflect the strength of influence of each connection (arrow width) as indicated by participants. The importance of factors was derived from their centrality in the model, and indicated by their strength comprising the sum of the weights of the incoming and outgoing connections of each factor. Next, we examined whether any of the six COVID-19 beliefs affected the composition of the model. Responses to the COVID-19 beliefs questions were recoded into low (1 and 2), medium (3), and high (4 and 5) to facilitate interpretation of findings. Factor selection (which factors were included in the model) and strength (the sum of weights of the arrows connecting the factors) served as dependent variables for the analyses and were computed using the M-Tool data analysis script in R (Van Boxtel & Van den Broek, Citation2021). Each dependent variable reflected a repeated measures structure, because factor selection and factor strength were computed for each factor for each participant. Hence, for both dependent variables we ran a multilevel model with a random subject intercept to account for the interdependence of data, and factor ID as a predictor to distinguish between each of the 15 factors in the model. Each regression analysis included the six beliefs and their two-way interactions with factor ID as predictors. Correlations were calculated between demographic variables age, gender and education and the two dependent variables (number of factors selected and mean strength of factors) to examine whether these should be included in the model as control variables.
For the regression on the factor selection, we conducted a logistic regression due to the binary nature of this variable for each factor. For the regression on the strength of the factors, we employed a negative binominal distribution to reflect values with discrete data with much variation (McCullagh & Nelder, Citation2019). A main effect for factor ID would indicate that the likelihood of selection or the strength differs across factors. A main effect for a particular belief variable would indicate that participants who differ in their beliefs select a different number of factors for their models. An interaction effect between factor ID and a belief variable would indicate that the differences of the selection or strength of the factor in the mental model differed across participants with low, medium or high levels of the particular belief. For example, an interaction between factor ID and individual control on the strength dependent variable would indicate that the relationship between individual control and the strength values of the factors varied across factors, suggesting that participants with different levels of control may focus on different factors in their mental models. Akaike’s information criterion (AIC) was used for model selection, with AIC estimating the amount of information that is lost by one model compared to another. Different models were compared and the model with the lowest AIC was deemed to have the least estimated information loss (Burnham & Anderson, Citation2002). Hence, a backward stepwise selection procedure was applied using the AIC criterion. Akaike’s Information Criterion minimises the out-of-sample error and makes a stepwise regression a suitable method to obtain the most accurate model (Hastie et al., Citation2017). We started with the full model, including the six COVID beliefs, factor, and their interactions, and computed the AIC for each term. The term with the lowest AIC was removed from the model, and the model was run again, to remove the next term with the lowest AIC until the AIC value for the entire model was lower than the AIC values for each term in the model. For the final model, we checked the model fit by inspecting deviance residual plots, and 95% profile (log-) likelihood confidence intervals were calculated.
Results
Descriptives
gives an overview of COVID-19 beliefs and shows that participants on average were moderately concerned about getting infected with the virus and worried quite a lot about the pandemic. They reported moderate trust in the government’s approach to curbing the pandemic but they were critical about the appropriateness of the mitigation measures. They also indicated to experience fairly strong personal control over the spread of the virus and had similar thoughts about other people being in control. Regarding infection status, the majority of participants (70%) reported not to have been infected with COVID-19, 22% indicated to have experienced an infection and 8% were uncertain about whether or not having been infected.
Mental models
Participants included on average ten factors in their mental model (M = 9.50, SD = 3.69). shows that the most frequently included factors were parties (80%), group gatherings (78%) and willingness to get vaccinated (76%); the least frequently included factors were willingness to test in case of symptoms (50%), working from home (47%) and ventilation (41%).
Table 4. Factors included in the mental model and their centrality scores degree (sum of incoming and outgoing connections of each factor) and strength (sum of the weights of the incoming and outgoing connections of each factor) (N = 147).
On average, participants identified about twelve connections between the factors in their model (M = 11.51, SD = 6.42), which equals about two per factor (M = 1.71, SD = .69). The factors most central in the mental models were mitigation measures by government, adherence to measures, and virulence: these factors received or exerted the strongest influence (labeled as strength, respectively M = 5.38, M = 5.71, and M = 5.65). Other factors (e.g. parties and traveling abroad) were named frequently but were quite isolated as they did not have many connections with other factors. The average proportion of direct connections with the target variable of virus spread was 58% and the remaining 42% pertained to connections between factors. The most frequently named direct connections with the target variable COVID-19 spread had heigh mean weights indicating a strong influence and included willingness to get vaccinated, virulence, and meeting many different people (see ). Next to direct connections between factors and the target variable, some between-factor connections were also named relatively often (see ). In particular, the perceived associations between availability of vaccines and willingness to get vaccinated, and between mitigation measures by government and adherence to measures suggest that participants discerned a dependence of their own behavior relating to arrangements made by government. The patterns described above are visualized in the aggregated mental model of the entire sample displayed in .
Figure 2. Aggregated mental model (N = 147). The arrows represent connections between factors with thickness of the arrows representing the weight assigned to the connections.
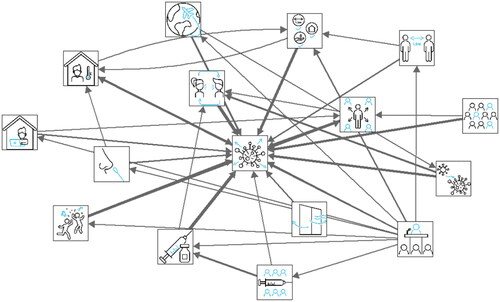
Table 5. Most frequently included connections in the mental models and their mean weight (average weight of connections between both factors) (N = 147).
Mental models relating to COVID-19 beliefs
Next, we examined to what extent mental models were driven by beliefs about COVID-19. We explored the influence of COVID-19 beliefs on two aspects of the mental model: the selection of factors included in the mental model (factor selection) and the sum of the weights of the incoming and outgoing arrows for the factors (strength). Both factor selection and strength represent elements of the model’s complexity.
Beliefs and factor selection
A regression analysis was conducted with factor selection as the dependent variable and factor ID, the six COVID-19 beliefs and their two-way interaction as predictors and including random subject intercepts. The full model (AIC = 2539.8) was reduced based on a series of stepwise backward regression analyses (see ), resulting in the final model including factor ID, individual control, concern, and worry (AIC = 2379.60; see Supplementary Materials).
Table 6. Changes in AIC with each step in the backward stepwise selection procedure. In each step the term with the smallest term in the model was omitted.
Participants with higher levels of personal control selected 2.31 times (profile log-likelihood CIOR= 0.87, 6.14) more factors in their model compared to participants who reported lower levels of control. Similarly, participants with higher levels of worry included 5.04 times more factors in their mental models (profile log-likelihood CIOR= 1.2, 21.24) compared to those with low level of worry. No difference across factors in factor selection was found depending on how much control participants experienced (as witnessed by the absence of interactions in the model). No contrasts were performed between specific factors; we refer to for an overview of selection of factors.
Beliefs and strength
A regression analysis was conducted with the strength values for each factor (i.e. the sum of the weights of the incoming and outgoing arrows for the factors) as the dependent variable and factor ID and the six COVID-19 beliefs and their two-way interactions as predictors and including random subject intercepts. Because a strong correlation was observed between age and the mean strength of the factors (r = .82, p <.001), participant age was added to the model. The full model (AIC = 6222.1) was reduced based on a series of stepwise backward regression analyses (see ), resulting in the final model including factor ID, the belief about appropriateness of governmental measures and general control (AIC = 6050.0; see Supplementary Materials). The mean strength of the connections of the factors in the models of participants who believed that proper measures were taken was 1.16 times higher compared to participants who believed insufficient measures were taken (95% profile (log-)likelihood CIOR = 1.03, 1.30). The strength values of participants who believed that the mitigation measures were too stringent were 1.10 higher compared to participants who believed insufficient measures were taken (95% profile (log-) likelihood CIOR = 0.91, 1.33). This is likely due to the small sample of participants (N = 16; compared to 68 participants who believed insufficient measures were taken and 63 participants who believed measures were fitting). The mean strength of the factors of participants who believed that people in general have high levels of control over the spreading of the virus was 1.30 times higher compared to participants who believed people have little control over the spreading of the virus (95% profile (log-)likelihood CIOR = 1.00, 1.68). These results show that participants with higher levels of general control and more satisfaction with the measures created more connected mental models. No contrasts were performed between specific factors; for an overview of strength values across factors, we refer to .
Discussion
Our findings reveal that participants construed a model comprising on average ten factors (out of 15 provided to them) that had either direct connections with the target variable of COVID-19 proliferation or connections with other factors with different degrees of influence. This shows that their understanding of factors driving the pandemic goes beyond a simple scheme of all factors equally contributing to the spread of the virus in a direct way and attests to the added value of mapping mental models. Social factors – varying from parties to social gatherings and meeting many people – play a prominent role in the spread of the virus in participants’ perception, although some of these factors (e.g. parties) were relatively isolated. Importantly, whereas the factors that we gathered from Studies 1a-b represented primarily specific measures and services (e.g. keeping a distance, availability of vaccines), participants’ average mental model highlighted the role of actors in dealing with the pandemic, in particular how mitigation measures were introduced by government, whether citizens adhered to measures, and their interconnection. This focus suggests that, according to participants, the interplay between governmental strategy and people’s response are at least as important as a whole range of specific measures in the pandemic strategy. Our findings further show that mental models were influenced by participants’ beliefs about personal or general control, worry about the pandemic, and appropriateness of governmental measures: more control, more worry, and thinking that measures were appropriate resulted in more complex models with more factors included. This again shows how governmental strategy and citizen response are both crucial ingredients of people’s understanding of the pandemic.
General discussion
In a series of three studies, we set out to document the mental models of the COVID-19 pandemic with a focus on how they understand factors contributing to the spread of the virus. Study 1a provided an overview of the factors that people associate with COVID-19 proliferation, including a wide variety of topics but with a prominent role for their own and others’ behavior in drivers of the pandemic. In Study 1 b we determined the importance of a selection of the factors that were mentioned most frequently. The fifteen most important factors were subsequently used to elicit mental models and examine how people make sense of factors that drive the spread of COVID-19 (Study 2). We found that people created relatively complex models consisting of about ten factors with a substantial number of interconnections next to their direct influence on the target variable of COVID-19 spread. In view of the overload of information about the pandemic that frequently changed over time (e.g. as witnessed by the Oxford Stringency Index) and intense debate on social media and in newspapers and television shows, it is noteworthy that participants were able to provide such a fairly comprehensive model. Overall, the average mental model covered a broad range of factors including virus characteristics, governmental mitigation measures, and their own behavior.
Three findings stand out. First, the factors named by participants reflect the main messages that have been communicated repeatedly by public health authorities (e.g. https://www.who.int/emergencies/diseases/novel-coronavirus-2019/advice-for-public), suggesting that communication about COVID-19 has come across. The significant role of public health communication also shows in the apparently remarkable absence of face masks as an important factor in COVID-19 proliferation. This may be due to the Dutch government not actively promoting face masks at the time of the study. It should be emphasized that it was not our objective to verify that participants have a correct understanding of the pandemic that resonates major virological and epidemiological views. Mental models provide insight into the lay understanding of the pandemic rather than a check of their factual understanding. From that point of view, it is noteworthy that participants tended to highlight social factors in their models, which may relate to reduced social contact having a significant impact on people’s lives (De Wit et al., Citation2022). It is also noteworthy that some high ranking factors reflect public debate next to public health communication. This especially applies to the factors of parties and willingness to get vaccinated, which were prominent topics in the media because they raised controversy: whereas some people considered partying and not getting vaccinated to be offensive and selfish, others deemed these behaviors to be an expression of the right to make an autonomous decision.
Second, a prominent feature of the mental models generated by participants is the distinction between measures and actors as crucial elements in drivers of the pandemic: participants clearly differentiated between how government communicates measures and people willing to adhere to measures. Apparently, many participants did envisage a clear role for preventive behaviors as an important factor that influences the spread of the virus. Whilst the way they tend to interpret this factor suggests that they refer to other people’s behavior rather than their own (as became apparent from responses in Study 1a), many participants seem to have taken good notice of the main message communicated by public health authorities that individual behavior plays a critical role in curbing the pandemic. Notwithstanding the appreciation of the prominent role of their own behavior, participants also discern their own behavior from opportunities to enact this behavior depending on arrangements made by public health authorities such as, for example, securing the availability of tests and vaccines.
Third, our results show that participants who were satisfied with mitigation measures or who experienced high levels of personal or general control or worry about the spread of COVID-19 created more extensive mental models with greater connectedness between factors or a greater number of factors compared to people who were not satisfied, felt less control or worry. This may suggest that people with doubts about the appropriateness of measures or who feel helpless have more trouble in understanding how the pandemic works, which may affect their motivation and ability to adjust their behavior.
Our findings bear implications for the improvement of communication about mitigation measures. Specifically, our data suggest that people may appreciate transparent communication to comprehend how distinct measures relate to each other and to appreciate the bigger picture behind the governmental strategy to curb the pandemic (Siegrist & Bearth, Citation2021). For example, it may be helpful to explicitly explain the main lines of action (reducing the number of contacts and reducing the risk per moment of contact) and how each measure falls under one or both of these lines. For a measure such as testing in case of symptoms, which was only weakly connected to staying at home and to spread of the virus, it may be helpful to explain that it is not only beneficial for individuals themselves but also for the entire community because testing (when followed by appropriate quarantine and/or isolation behavior) contributes to reducing the spreading of COVID-19.
Limitations
Our research has a number of limitations. Whereas Study 1a comprised a heterogeneous sample with significant contributions from people with migrant descent and lower education, the samples in Study 1 b and Study 2 included primarily young and higher educated participants. This affects the generalizability of our findings, as (older) people with lower education levels and/or from migrant descent have been shown harder to reach by public health communication about the pandemic and, as a result, may have a different understanding of the pandemic. Future research should address mental models in more heterogeneous samples including people who may be less knowledgeable and/or more critical about the pandemic. Notwithstanding this limitation, it should be emphasized that a large majority of people support public health measures (De Wit et al., Citation2022) and that our sample is not exceptional in their overall positive evaluation of the pandemic strategy. Also the high drop-out rate in Study 2 affects the generalizability of our findings. It may be that the online completion of the mental model was too complex for some participants. Previous research with M-Tool has shown that elicitation of mental models works well in samples with low education when assisted by a research assistant (Van den Broek, Luomba, et al., Citation2021), suggesting that drop-out is associated with using the online tool rather than dealing with the task of creating a map of their mental model. Notwithstanding this limitation, the majority of participants who completed the mental model online were positive about using the M-Tool, took sufficient time to create a map, and indicated that they were serious about completing the task.
Concluding remarks
In view of the promise that mental models hold to understand and explain how people perceive a complex phenomenon such as the COVID-19 pandemic (Michie et al., Citation2020; World Bank, Citation2014), future research should document these models in larger and more heterogeneous samples, examine how they unfold over time and to what extent they are influenced by communication by public health authorities.
Supplemental Material
Download MS Word (20.1 KB)Acknowledgements
We thank Maartje Aalders, Jetske Mulder and Heleen van der Zee for their kind help with data collection. We also thank Liselotte van de Sande for designing the icons en Jan van den Broek PhD for advice on statistical analyses.
Disclosure statement
No potential conflict of interest was reported by the authors.
Funding
The author(s) reported there is no funding associated with the work featured in this article.
References
- Aminpour, P., Gray, S. A., Jetter, A. J., Introne, J. E., Singer, A., & Arlinghaus, R. (2020). Wisdom of stakeholder crowds in complex social–ecological systems. Nature Sustainability, 3(3), 191–199. https://doi.org/10.1038/s41893-019-0467-z
- Attema, A. E., Haridon, O. L., Raude, J., & Seror, V. (2021). Beliefs and risk perceptions about COVID-19: Evidence from two successive French representative surveys during lockdown. Frontiers in Psychology, 12, 1–16. https://doi.org/10.3389/fpsyg.2021.619145
- Biggs, D., Abel, N., Knight, A. T., Leitch, A., Langston, A., & Ban, N. C. (2011). The implementation crisis in conservation planning: Could ‘mental models’ help? Conservation Letters, 4(3), 169–183. https://doi.org/10.1111/j.1755-263X.2011.00170.x
- Bogg, T., & Milad, E. (2020). Demographic, personality, and social cognition correlates of coronavirus guideline adherence in a U.S. sample. Health Psychology, 39(12), 1026–1036. https://doi.org/10.1037/hea0000891
- Bostrom, A., Böhm, G., Hayes, A. L., & O'Connor, R. E. (2020). Credible threat: Perceptions of pandemic coronavirus, climate change and the morality and management of global risks. Frontiers in Psychology, 11, 578562. https://doi.org/10.3389/fpsyg.2020.578562
- Bostrom, A., O’Connor, R. E., Böhm, G., Hanss, D., Bodi, O., Ekström, F., Halder, P., Jeschke, S., Mack, B., Qu, M., Rosentrater, L., Sandve, A., & Saelensminde, I. (2012). Causal thinking and support for climate change policies: International survey findings. Global Environmental Change, 22(1), 210–222. https://doi.org/10.1016/j.gloenvcha.2011.09.012
- Broek, K. L., Klein, S. A., Luomba, J., & Fischer, H. (2021). Introducing M-Tool: A standardised and inclusive mental model mapping tool. System Dynamics Review, 37(4), 353–362. https://doi.org/10.1002/sdr.1698
- Burnham, K., & Anderson, D. (2002). Model selection and multimodel inference. Springer-Verlag.
- De Wit, J. B. F., De Ridder, D. T. D., Van den Boom, W., Kroese, F. M., Van den Putte, B., Stok, F. M., Leurs, M., & De Bruin, M. (2022). Understanding public support for COVID-19 pandemic mitigation measures over time: Does it wear out? [Manuscript under review]
- Goldberg, M. H., Gustafson, A., & Van der Linden, S. (2020). Leveraging social science to generate lasting engagement with climate change solutions. One Earth, 3(3), 314–324. https://doi.org/10.1016/j.oneear.2020.08.011
- Greenhalgh, T. (2021). Miasmas, mental models and preventive public health: Some philosophical reflections on science in the COVID-19 pandemic. Interface Focus, 11(6), 20210017. https://doi.org/10.1098/rsfs.2021.0017
- Güss, C. D., & Robinson, B. (2014). Predicted causality in decision making: The role of culture. Frontiers in Psychology, 5, 479. https://doi.org/10.3389/fpsyg.2014.00479
- Hastie, T., Tibshirani, R., & Friedman, J. (2017). Model assessment and selection. In T. Hastie, R. Tibshirani, & J. Friedman (Eds.), The elements of statistical learning: Data mining, inference, and prediction. (pp. 219–259). Springer.
- Hovmand, P. S., Pronk, N. P., Kyle, T. K., Nadglowski, J., Nece, P. M., & Lynx, C. T. (2021). Obesity, biased mental models, and stigma in the context of the obesity COVID-19 syndemic. National Academy of Medicine Perspectives, National Academy of Medicine. https://doi.org/10.31478/202104a
- Jetter, A. J., & Kok, K. (2014). Fuzzy cognitive maps for futures studies. A methodological assessment of concepts and methods. Futures, 61, 45–57. https://doi.org/10.1016/j.futures.2014.05.002
- Johnson-Laird, P. N. (1983). Mental models: Towards a cognitive science of language, inference, and consciousness. Cambridge University Press.
- Johnson-Laird, P. N. (2010). Mental models and human reasoning. Proceedings of the National Academy of Sciences of the United States of America, 107(43), 18243–18250. https://doi.org/10.1073/pnas.1012933107
- Jones, N. A., Ross, H., Lynam, T., Perez, P., & Leitch, A. (2011). Mental models: An interdisciplinary synthesis of theory and methods. Ecology and Society, 16(1), 46. doi: www.ecologyandsociety.org/vol16/iss1/art46/ https://doi.org/10.5751/ES-03802-160146
- Lee, J.-K., Bullen, C., Ben Amor, Y., Bush, S. R., Colombo, F., Gaviria, A., Karim, S. S. A., Kim, B., Lavis, J. N., Lazarus, J. V., Lo, Y.-C., Michie, S. F., Norheim, O. F., Oh, J., Reddy, K. S., Rostila, M., Sáenz, R., Smith, L. D. G., Thwaites, J. W., Were, M. K., & Xue, L. (2021). Institutional and behaviour-change interventions to support COVID-19 public health measures: A review by the Lancet Commission Task Force on public health measures to suppress the pandemic. International Health, 13(5), 399–409. https://doi.org/10.1093/inthealth/ihab022
- Leventhal, H., Nerenz, D. R., & Steele, D. J. (1984). Illness representations and coping with health threats. In A. Baum, S. E. Taylor, & J. E. Singer (Eds.), Handbook of psychology and health (Vol. 4, pp. 219–252). Erlbaum.
- Lowe, T. D., & Lorenzoni, I. (2007). Danger is all around: Eliciting expert perceptions for managing climate change through a mental models approach. Global Environmental Change, 17(1), 131–146. https://doi.org/10.1016/j.gloenvcha.2006.05.001
- Margraf, J., Brailovskaia, J., & Schneider, S. (2020). Behavioral measures to fight COVID-19: An 8-country study of perceived usefulness, adherence and their predictors. PloS One, 15(12), e0243523. http://doi.org/10.1371/journal.pone.0243523
- McCullagh, P., & Nelder, J. A. (2019). Generalized linear models. Routledge.
- Michie, S., West, R., Amlôt, R., & Rubin, J. (2020). Slowing down the covid-19 outbreak: Changing behavior by understanding it. BMJ Opinion. www.comminit.com/content/slowing-down-covid-19-outbreak-changing-behaviour-understanding-it
- Morgan, M. G., Fischhoff, B., Bostrom, A., & Atman, J. (2002). Risk communication: A mental models approach. Cambridge University Press.
- Pennycook, G., McPhetres, J., Bago, B., & Rand, D. G. (2022). Beliefs about COVID-19 in Canada, the United Kingdom, and the United States: A novel test of political polarization and motivated reasoning. Personality and Social Psychology Bulletin, 48(5), c https://doi.org/10.1177/01461672211023652
- Siegrist, M., & Bearth, A. (2021). Worldviews, trust, and risk perceptions shape public acceptance of COVID-19 public health measures. Proceedings of the National Academy of Sciences, 118, e2100411118. https://doi.org/10.1073/pnas.2100411118
- Van Boxtel, W., & Van den Broek, K. L. (2021). M-Tool data analysis script. https://public.yoda.uu.nl/geo/UU01/Y8GP93.html
- Van den Broek, K. L., Luomba, J., Van den Broek, J., & Fischer, H. (2021). Evaluating the application of the Mental Model Mapping Tool (M-Tool). Frontiers in Psychology, 12, 761882. https://doi.org/10.3389/fpsyg.2021.761882
- Van Stekelenburg, A., Schaap, G., Veling, H., & Buijzen, M. (2021). Investigating and improving the accuracy of US citizens’ beliefs about the COVID-19 pandemic: Longitudinal survey study. Journal of Medical Internet Research, 23(1), e24069. https://doi.org/10.2196/24069
- Vitriol, J. A., & Marsh, J. K. (2021). A pandemic of misbelief: How beliefs promote or undermine COVID-19 mitigation. Frontiers in Political Science, 3, 1–14. https://doi.org/10.3389/fpos.2021.648082
- Wang, C. C., Prather, K. A., Sznitman, J., Jimenez, J. L., Lakdawala, S. S., Tufekci, Z., & Marr, L. C. (2021). Airborne transmission of respiratory viruses. Science, 373(6558), 6558. https://doi.org/10.1126/science.abd9149
- Wood, M. D., Thorne, S., Kovacs, D., Butte, G., & Linkov, I. (2017). Mental modeling approach. Springer.
- World Bank. (2014). Thinking with mental models. Mind, society and behavior. https://doi.org/10.1596/978-1-4648-0342-0_ch3
- World Health Organization. (2021). WHO coronavirus (COVID-19) dashboard. Retrieved December 18, 2021 from https://covid19.who.int.