Abstract
Objective
To develop a theory-informed behaviour change intervention to promote appropriate hospital antibiotic use, guided by the Medical Research Council’s complex interventions framework.
Methods
A phased approach was used, including triangulation of data from meta-ethnography and two qualitative studies. Central to intervention design was the generation of a robust theoretical basis using the Behaviour Change Wheel to identify relevant determinants of behaviour change and intervention components. Intervention content was guided by APEASE (Acceptability, Practicability, Effectiveness, Affordability, Side-effects, and Equity) criteria and coded using a Behaviour Change Technique Taxonomy. Stakeholders were involved throughout.
Results
From numerous modifiable prescribing behaviours identified, active ‘antibiotic time-out’ was selected as the target behaviour to help clinicians safely initiate antibiotic reassessment. Prescribers’ capability, opportunity, and motivation were potential drivers for changing this behaviour. The design process resulted in the selection of 25 behaviour change techniques subsequently translated into intervention content. Integral to this work was the development and refinement of a Digital Antibiotic Review Tracking Toolkit.
Conclusion
This novel work demonstrates how the Behaviour Change Wheel can be used with the Medical Research Council framework to develop a theory-based behaviour change intervention targeting barriers to timely hospital antibiotic reassessment. Future research will evaluate the Antibiotic Toolkit’s feasibility and effectiveness.
Background
Antibiotics have revolutionised medical practice and significantly reduced illness and death rates from infectious diseases since their introduction in the 1940s (Gaynes, Citation2017), but decades of antibiotic overuse have accelerated the evolution of bacteria that are resistant to antibiotics (Barber & Swaden-Lewis, Citation2017). The decline in new drug development and the sustained misuse of existing antibiotics have led to fears of a ‘post-antibiotic era’, where common infections, once considered minor, could kill again, claiming thousands of lives (World Health Organization, Citation2016). Antimicrobial resistance (AMR) is currently one of the top ten global public health threats, with an increasing number of countries reporting a high prevalence of infections resistant to critical antibiotics, causing at least 70,000 deaths each year globally (Barber & Swaden-Lewis, Citation2017; National Institute for Health & Care Excellence, Citation2018). In the European Union alone, AMR is responsible for an estimated 33,000 deaths and €1.5billion in extra healthcare costs per year (Cassini et al., Citation2019).
In the United Kingdom (UK), rising resistance levels and unavailability of newer antimicrobials have led to coordinated efforts to optimise the use of currently available antibiotics, but rates of antibiotic prescribing remain high compared to some other European countries (National Institute for Health & Care Excellence, Citation2018). Despite some progress in primary care, a sustained reduction in total antibiotic prescribing in secondary care has not been observed. Hospital usage has increased by 6.3% over the last five years, highlighting the need for renewed efforts to improve the quality of antibiotic prescribing (Public Health England, Citation2020). The recent COVID-19 pandemic has accelerated high levels of broad-spectrum antibiotic prescribing despite low rates of bacterial co-infection, giving weight to the concerns about the increased long-term threat of AMR (Russell et al., Citation2021). Although a wide range of interventions have been found effective in improving antibiotic use across hospital settings (Hulscher & Prins, Citation2017), their effect sizes vary considerably, with similar interventions reported to produce different results (Davey et al., Citation2017).
Antibiotic prescribing has only recently been recognised as a complex behaviour. This recognition has led to more attention being paid to changing individual prescriber behaviour (directly or indirectly), thereby guiding the more effective use of antibiotics as key to tackling AMR (Krockow et al., Citation2019; Teixeira Rodrigues et al., Citation2013). Yet, reviews to date show that the contribution of behavioural science remains underutilised in the design of antimicrobial stewardship (AMS) initiatives (Charani et al., Citation2011; Hulscher & Prins, Citation2017). For example, although behavioural change interventions—whether restrictive, persuasive, or structural—can improve patient outcomes, microbial outcomes, costs or some combination of these, most studies lack details of the underlying theory, limiting future replication and study comparisons (Davey et al., Citation2017). Consequently, there have been calls for the application of appropriate and more robust designs to developing behavioural change AMS interventions (Hulscher & Prins, Citation2017).
Theory-based intervention design
The UK Medical Research Council (MRC) has long advocated the importance of employing a theory-based approach for understanding the likely causal processes of change before undertaking the intervention effectiveness stage (Craig et al., Citation2008; Skivington et al., Citation2021). Understanding the underpinning factors, including the influence of social norms, attitudes and beliefs that drive behaviour, can facilitate the development of more effective interventions that target specific behaviour deficits (Lorencatto et al., Citation2018). Many frameworks exist upon which behaviour change interventions can be based; however, it remains unclear which is the most comprehensive and conceptually coherent (Michie et al., Citation2011). The Behaviour Change Wheel (BCW) aims to overcome this problem and synthesises 19 frameworks for classifying behaviour change interventions and allows direct matching of intervention targets (behaviour, population, and context) to specific mechanisms of action (the processes through which behaviour change occurs) (Connell et al., Citation2019). This step-by-step method for the systematic designing of behaviour change interventions has been successfully applied in a number of different contexts (Barker et al., Citation2018; Johnson et al., Citation2018).
The BCW consists of three components (). At its core is the COM-B model (Capability, Opportunity, and Motivation) representing the major influences on behaviour and requirements for it to occur. The second layer of the BCW comprises nine intervention functions that characterise the type of intervention that is required, such as education, training and incentivisation. The third and outer layer contains seven policy categories—high-level strategies to support the implementation of intervention types. An intervention achieves its function(s) through the use of behaviour change techniques (BCTs), which are the ‘active ingredients’ of an intervention, as specified in the Behaviour Change Techniques Taxonomy v1 (BCTTv1) (Michie et al., Citation2013). The BCTTv1 classification system contains agreed definitions, which allows the intervention content to be clearly described using standard terminology. A published guide to using the BCW in intervention design provides theory-based linkages between COM-B influences on behaviour, intervention types and BCTs, which aids selection of intervention content (Michie et al., Citation2014).
Figure 1. The Behaviour Change Wheel, highlighting the COM-B model, intervention functions and policy categories (Michie et al., Citation2014), licensed under the Creative Commons Attribution License 2.0.
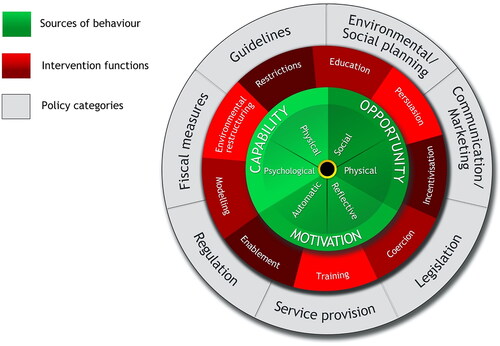
The BCW can also be mapped against other frameworks for understanding and changing behaviour, including the Theoretical Domains Framework (TDF). This framework builds on the systems identified in the COM-B to further uncover the underlying barriers and facilitators of evidence-based change (French et al., Citation2012). Comprising 14 domains, the TDF provides a comprehensive grouping of the overlapping constructs within behavioural theories. Such mapping can suggest possible intervention strategies likely to be effective in addressing different behavioural drivers in the target group (i.e. individual, socio-cultural, and environmental enablers and barriers) (Cane et al., Citation2015; Michie et al., Citation2014). These methods can effectively identify why an intervention that works in a particular setting might fail in another or show different effects (Shallcross et al., Citation2020).
This research aimed to develop, ahead of a future trial, a theory-based behaviour change intervention to improve hospital antibiotic use—the Digital Antibiotic Review Tracking Toolkit (DARTT). This paper describes how each phase of the BCW was operationalised and integrated within the 2008 MRC framework to guide the optimal development of the DARTT intervention. By reflecting on our use of the BCW in the development of a complex healthcare intervention, we hope to provide a detailed outline for future intervention designers in the use of a similar approach.
Methods
The DARTT intervention was developed in two phases: (1) initial intervention planning and development guided by the three-stage, eight-step approach of the BCW (Michie et al., Citation2014); and (2) refinement and optimisation of the intervention prototype. The process of intervention development involved three key stages (). The first stage (Steps 1–4) is concerned with ‘understanding the behaviour’ and includes the COM-B model at Step 4. The second stage (Steps 5 and 6) ‘identifies the intervention options’ and the third stage (Steps 7 and 8) ‘identifies the content and implementation options’. All eight steps are outlined in detail in the Results section below, including further details of the method. Ethical and management approvals were obtained from Edinburgh Napier University Research Integrity (ref: SHSC/0003) and the NHS Lothian R&D Committees (ref: 2018/0007). Two major acute NHS hospitals in Scotland, including clinicians and management, participated in the intervention development. Patient and professional involvement was at the heart of this research, with service providers and users being involved from project conception and throughout the study.
Results
Stage 1: understand the target behaviour
Step 1—define the problem in behavioural terms
The definition of the problem in behavioural terms was based on the clinical and research experience of the research team, findings from previous relevant literature and regular consultations with practice experts, including microbiology. The multidisciplinary research team (social scientist, psychologist, nurses) had considerable expertise in developing behaviour-change interventions, including previous involvement in projects exploring hospital antibiotic stewardship. After reviewing existing interventions that improve antibiotic prescribing in acute hospitals (Davey et al., Citation2017; Ramsay et al., Citation2003), major barriers and facilitators to improving antibiotic use (Krockow et al., Citation2019; Teixeira Rodrigues et al., Citation2013), and the utility of behavioural science to support the design of AMS interventions (Charani et al., Citation2011; Hulscher et al., Citation2010; Hulscher & Prins, Citation2017), we identified the behavioural problem as reducing inappropriate antibiotic use in acute hospitals.
Step 2—select target behaviour
To select the target behaviour, the research team generated a list of behaviours potentially relevant to the problem behaviour identified in Step 1, including improving the practice of initiation, monitoring, review and discontinuation of antibiotic treatment that is not concordant with guidelines (Monnier et al., Citation2018). However, as individual behaviours do not occur in isolation and are influenced by other behaviours (West et al., Citation2020), it was essential we understood the context relevant to the target behaviour first. To do this, we carried out a systematic review and qualitative evidence synthesis using Noblit and Hare’s meta-ethnography (1982)—a unique, rigorous and systematic method of synthesising multiple primary qualitative studies, which is best suited for developing conceptual models and theories (Noblit & Hare, Citation1988). This research has been published elsewhere (Wojcik et al., Citation2021).
To refine the theory derived from the meta-ethnography, identify which problem behaviours are amenable to change in this context and ensure that the future intervention is participant-centred, we carried out three focus groups with seventeen stakeholders—14 various healthcare professionals (HCPs) and 3 health-service users. Amongst the total group of participants, there were 10 females and 7 males, age range 29–68 years. Healthcare professionals had between 2.5–32 years of clinical experience (mean 11 years, SD 8.5), and the extent of their involvement in prescribing of antibiotics ranged from full clinical responsibility for prescribing decisions (2 consultant physicians) to aiding result interpretation and selection of targeted antibiotics (2 microbiology consultants), daily review and monitoring of prescriptions (2 clinical pharmacists), autonomous prescribing within the remit of professional competence (3 advanced nurse practitioners), regular audits and administration of antibiotics (5 infection surveillance and staff nurses). Participant characteristics are presented in Box 1. Focus group data were organised according to emerging categories and analysed using Framework Analysis (Ritchie & Lewis, Citation2013).
Box 1. Focus groups sample characteristics.
Using the results of our meta-ethnography supplemented with focus group data, we developed a conceptual model depicting the multiple determinants (at the macro and micro-level) that influence appropriate antibiotic use in hospital settings (Wojcik et al., Citation2021). Based on the identified factors that could be targeted for the intervention and in line with the UK Start Smart –Then Focus initiative advocating an active review of antibiotic prescriptions within 48–72 hours (Ashiru-Oredope et al., Citation2012), initiation of ‘antibiotic time-out’ (timely and systemic evaluation of the continuing need and choice of antibiotics), were deemed appropriate to address the behavioural problem. Data analysis generated a list of candidate target sub-behaviours to improve active antibiotic time-out (e.g. increasing the uptake of clinical reassessment, data review and modification of therapy based on the results, and improving documentation and communication about antibiotic decisions). Recommendations for improving practice were provided at this stage. From the subsequent data analysis, it became apparent that the intervention needed to fit the local context and target all HCPs involved in antibiotic decision-making but must also include patients and/or their families, in other words, to make active antibiotic time-out ‘everyone’s business’.
Step 3—specify the target behaviour
By answering the questions provided in BCW guidance (Michie et al., Citation2014), the target behaviour was specified within the context of a hospital environment and summarised in . Being clear about this detail was crucial for fully drawing out the range of barriers and facilitators of behaviour change in the next step.
Table 1. Specifying the target behaviour (Step 3).
Step 4—identify what needs to change
This step involved identifying the potential drivers of the target behaviour. This required moving beyond the MRC sub-phase of identifying the evidence base and developing theory to making a behavioural diagnosis (West et al., Citation2020). Although critical, this step is often overlooked in intervention design (Michie et al., Citation2014). As the meta-ethnography (Wojcik et al., Citation2021) and focus group findings overlapped, we were able to triangulate the data by cross-checking the themes for any refutations and new emergent knowledge and then mapping them onto the COM-B components and the corresponding TDF domains. We then determined what needed to change in the person and/or environment to achieve the desired target behaviour (initiation of antibiotic time-out) by selecting key items from the behavioural diagnosis considered most relevant for the DARTT intervention. To achieve this, we sought input from a behaviour change expert. For each TDF domain, we collated all the barriers and facilitators and prioritised those considered modifiable; exerting a strong influence on the target behaviour; likely to be feasible and relevant at the site; and acceptable to the prescribers (see Appendix 1).
In sum, the behavioural diagnosis revealed that changes were needed within all the COM-B components (across 12 TDF domains) for the problem behaviour (). We determined that the intervention needed to target prescribers’ Capability, both physical (e.g. all prescribers needed to acquire competence and further skills in reassessing antibiotic therapy) and psychological (e.g. all prescribers needed to possess relevant knowledge of how to initiate and perform antibiotic time-out); Opportunity, both social (a supportive organisational culture, including increased social support from peers and other specialities in the decision-making process during antibiotic time-out) and physical (e.g. increasing the use of resources to effectively document and communicate prescribing decisions); and Motivation, both reflective (e.g. promoting the expectation that all HCPs are encouraged to ask questions related to antibiotics and initiate antibiotic time-out) and automatic (e.g. prescribers needed to feel more comfortable in switching/stopping antibiotics). These drivers have been further considered in this intervention development described in Stage 2.
Table 2. Behavioural analysis of determinants influencing active ‘antibiotic time-out’ within the context of hospital prescribing (Step 4).
Stage 2: identify intervention options
Steps 5–6—identify intervention functions and policy categories
Once the relevant COM-B components that can be targeted as potential levers for change were identified, Phase 2 involved mapping them to the nine intervention functions (broad categories that enable a change in behaviour) using the grids published in the BCW guide and the recommended APEASE (affordability, practicability, effectiveness and cost-effectiveness, acceptability, side effects/safety, equity) criteria for making context-related decisions (Michie et al., Citation2014). Seven out of nine intervention functions were considered potentially relevant for the intervention within a hospital context. We selected the functions of education, training, persuasion, modelling, restriction, environmental restructuring and enablement as relevant. These seven intervention functions were subsequently mapped to the policy categories within the BCW, with three identified as likely to support the intervention delivery. These included guidelines (e.g. informing clinicians of antimicrobial guidelines); communication to influence organisational practice by communicating a vision for change (e.g. using verbal and electronic communication to generate awareness of AMR/consequences of overprescribing antibiotics or benefits of an active antibiotic time-out), and environmental/social planning (e.g. designing and utilising an automated system for reminding hospital prescribers to initiate antibiotic time-out). Details of the evaluation of each intervention option against the APEASE criteria are provided in Appendix 2.
Phase 3: identify content and implementation options
Step 7—identify behaviour change techniques
To identify suitable BCTs for each prioritised TDF domain, we used the labels and detailed definitions included in the BCT Taxonomy v1 (BCTTv1), which lists 93 BCTs with descriptions and examples of their application (Michie et al., Citation2013). The coding and identification of all possible BCTs aligned with the selected intervention functions were informed by published guidance (Cane et al., Citation2015). The list of all possible BCTs was then reduced to the ones most likely to alter prescribers’ behaviour and those that could most feasibly be applied within the hospital context. This process was deductive and cyclical. We decided on the final BCT inclusion through team discussions. As a result, we selected 25 BCTs as the most appropriate for effecting behaviour change, taking barriers and facilitators identified in Step 4 (see Appendix 1) into account. For example, one of the identified facilitators to the target behaviour was a robust audit trail of decisions (e.g. BCTs 12.1 restructure the physical environment and 12.5 add objects to the environment) linked to the COM-B Physical Opportunity) or the highlighted barrier was a lack of motivation to initiate antibiotic time-out (e.g. linked to the COM-B Psychological Capability, Social Opportunity, Reflective and Automatic Motivation, which may require BCT 1.1. goal setting (behaviour), 1.2 problem solving, more social support—BCT 3.1, 6.1 demonstration of the behaviour, 7.1 prompts and cues or restructuring of the social environment—BCT 12.2). These are not all the BCTs available, but the ones deemed most suitable for the proposed intervention based on the mapping exercises of the triangulated data. We then operationalised the active components of the intervention, which involved providing examples of how each selected BCT could be employed in practice, alongside the intervention functions that they serve and the COM-B elements that they address. See Appendix 3 for a summary of all phases of the BCW.
Step 8—identify mode of delivery
Once BCTs were identified, the selection of the mode of delivery and content development required creativity and pragmatism. To translate selected BCTs into intervention components and ensure the completeness of the description of this intervention, we used the Form of Delivery (FoD) framework, an essential ‘active ingredient’ that can drive intervention effectiveness, which provides a checklist of the information to include when describing an intervention (Dombrowski et al., Citation2016). For example, the selected mode of delivery had to accommodate high prescriber work demands. A digital intervention was chosen as the primary mode of delivery as suggested by the stakeholders in Step 2 of the project. This decision was further informed by a research team meeting focused on deliberating the intervention content using the APEASE criteria and published evidence of effectiveness to ensure it reflects the organisation’s overall commitment to streamlining clinical workflows (e.g. by utilising electronic clinical support tools). Then, the most appropriate mode of delivery of each technique was discussed and selected by the research team. Examples of modes of delivery include face-to-face or distance delivery at the individual or group level via phone (voice or text), print or digital media, broadcast media, outdoor media, or individually accessed computer programmes (Michie et al., Citation2014). The consensus team feedback was then collated into a table of positive and negative comments for each proposed intervention component and the subsequent modifications were made based on the data generated in the optimisation phase (described below). The next step involved consulting with the technology design agency to facilitate the process of translating identified BCTs into the DARTT intervention using the Design Sprint methodology, a unique process to solve critical issues through prototyping and brainstorming (Banfield et al., Citation2015). For example, to address the BCTs prompts/cues and habit formation, an integrated ‘traffic-light’ function was built into the dashboard of the antibiotic tracker, the core component of DARTT. This alert would prompt the responsible clinician to review clinical information pertinent to antimicrobial decision-making on the third calendar day of treatment and to document their decision regarding antibiotic continuation, discontinuation or optimisation when the relevant pathogen is identified. Additional elements of gamification techniques, such as logging activity minutes, were also included to enhance engagement and motivation.
Optimisation of the intervention
The MRC guidance emphasises the importance of early assessment of the intervention acceptability using prototypes (Skivington et al., Citation2021). This approach can highlight aspects that can be adapted based on end-user feedback without a full pilot trial. The intervention was optimised through in-depth qualitative research using online semi-structured interviews. The purposive sample comprised 15 HCPs and three lay participants. HCPs (various disciplines and varying levels of experience) were recruited from four Scottish Health Boards and two English Trusts. See Box 2 for participant characteristics. Interviews lasted 45–60 min, were audio-recorded and transcribed verbatim. Data analysis was guided by a priori issues identified using the RE-AIM (reach, efficacy/effectiveness, adoption, implementation and maintenance) Framework (Glasgow et al., Citation1999).
Box 2. Interviewee characteristics.
This study’s purpose was to assess the intervention’s initial acceptability and practicability using prototypes (screenshots of the antibiotic tracker’s graphical user interfaces) and to subsequently refine it based on service provider/user feedback. The user feedback helped to determine the intervention’s strengths and areas for improvement and ensure that it is appropriate for the context in which it is planned to be delivered (Levati et al., Citation2016). Overall, the findings provided clear recommendations on the intervention’s content, design and functionality. HCPs and service users found the intervention to have a clear clinical value, discussed adoption, and identified ways in which it could be modified to better reflect the hospital context. Particularly well received was the proposed functionality of the antibiotic tracker and the potential benefits of improving the quality of antibiotic reassessment decisions and patient safety. The optimisation phase also provided significant insights into the anticipated benefits of the intervention, including the reduction of inappropriate antibiotic prescribing and improvement of patient outcomes, which are part of the UK’s national action plan to tackle AMR (Department of Health, Citation2019). Nonetheless, further research is needed to assess how well the intervention works in practice. DARTT’s feasibility and effectiveness also need to be established.
The DARTT intervention
After completing each step of BCW, a DARTT intervention was developed to improve active initiation of antibiotic time-out, including clear documentation of decisions. DARTT is a complex multifaceted behavioural intervention targeting healthcare professionals involved in antibiotic prescribing or administration in hospital inpatients. It can be used flexibly, either as standalone components or as part of an integrated toolkit, with recommendations for using all materials, if this is appropriate. DARTT comprises four components, including:
Antibiotic review tracker—a digital system for tracking and monitoring antibiotic decisions designed to encourage a structured discussion about the continued need and choice of therapy.
Webinar—to introduce the tracker to clinical areas and raise awareness of AMR.
E-training—to provide web-based learning on using the tracker.
Patient information materials—to increase patient/family knowledge and awareness of AMR and promote shared decision-making.
Briefly, the antibiotic review tracker includes an electronic antimicrobial dashboard and a basic decision-support tool designed to flag up prescriptions needing a structured reassessment using the intervention functions of environmental restructuring, enablement and restriction. An integrated ‘traffic-light’ function prompts clinicians to initiate a formal antibiotic time-out on the third calendar day of treatment when the relevant pathogen is identified and make appropriate de-escalation decisions while fitting into hospital workflow. Hyperlinks to online resources, such as antibiotic guidelines, are embedded within the dashboard. The aim is to allow for increased autonomy without increasing the workload for local antimicrobial stewardship teams (Graber et al., Citation2015). The graphical user interface can run on different internet browsers and allows users to generate reports for patients who are eligible for a time-out. Each report contains basic patient information and relevant laboratory results to provide clinicians with objective criteria when assessing the patient’s response to antibiotics, allowing more time to focus on the evaluation of therapy rather than information collection or interpretation. It also includes relevant antimicrobial information and microbiology results, if available. The tracker has an inbuilt basic logic tree for correct dosing regimens (i.e. frequency and duration of antibiotic), free text fields for explaining the rationale for deviating from antibiotic guidelines and prompts for clear documentation of treatment plan and a discussion with the patient or their family. The tracker also allows overall performance reports on individual clinicians’ decisions (but not containing any patient data) to be generated and emailed to prescribers. There is evidence that integrated decision-support tools can improve specific aspects of patient care, including antibiotic decisions, by promoting behaviour change among clinicians (Rawson et al., Citation2017a). A summary of the intervention content, delivery and mechanism of action, is provided in Appendix 4. Full details of DARTT will be published separately.
Discussion
This paper describes the application of the Behaviour Change Wheel (BCW) to develop and refine a novel theory-driven DARTT intervention to improve the antibiotic reassessment. To our knowledge, this is the first study to operationalise the development phase of the MRC framework for complex interventions using the BCW in this context. This systematic approach provided insights into prescribers’ behaviours, barriers and facilitators to an active ‘antibiotic time-out’ and potential suggestions for addressing them. This study also provides a worked example of how primary and secondary data can be retrospectively applied to the BCW for intervention development and refinement, which other researchers may find useful. This work is novel as it extends the use of prospective qualitative approaches in assessing theoretical acceptability issues during the optimisation phase of intervention development.
Findings suggest that capability, opportunity, and motivation play an important role in antibiotic prescribing behaviours and that these factors are not independent, but all contribute to improving decisions surrounding de-escalation and (dis)continuation of prescriptions. Findings were incorporated into a behaviour intervention to optimise antibiotic time-out and increase the transparency of prescribing decisions. Seven intervention functions (education, training, persuasion, modelling, restriction, environmental restructuring, and enablement) and three policy categories (guidelines, communication and environmental/social planning) were identified to address a range of modifiable barriers and facilitators in a practical and acceptable way. Drawing on the behaviour change taxonomy, 25 BCTs aligned to these functions were then selected to specify the intervention content, addressing barriers and facilitators as potential targets to encourage active antibiotic time-out and improve documentation of decisions. Triangulated primary qualitative data with the secondary data derived from a systematic review and meta-ethnography enabled a rich exploration of identified barriers and facilitators, thus strengthening the design and creating more comprehensive research.
Comparison with existing literature
Previous literature has indicated that behavioural and social influences are often not considered in the design and evaluations of interventions aiming to improve antimicrobial prescribing (Pinder et al., Citation2015). This study extends the current evidence base by providing an in-depth understanding of the context-specific determinants of antibiotic prescribing behaviour in hospital settings, which is essential for the successful design, adoption, and implementation of quality stewardship interventions to improve practice. The behavioural theory developed as part of this work presents several promising targets for intervention development in this context. Mapping the salient TDF domains onto the COM-B model to explore factors influencing antibiotic prescribing has identified at least one barrier or enabler across 12 TDF domains. This means that addressing a single COM-B component will not bring about the desired behaviour change. Prior research has shown similar results (Chaves et al., Citation2014). However, evidence on the effectiveness of specific intervention options applied in the design of AMS initiatives remains scarce. Although the majority of BCTs identified in this study are congruent with a recent ‘review and revise’ behavioural intervention, which primarily focuses on communicating the degree of certainty surrounding the initial decision to prescribe antibiotics (Walker et al., Citation2019), several techniques selected for inclusion in DARTT were not identified in other published works (Courtenay et al., Citation2019; Rawson et al., Citation2017b). The findings provide new insights into the use of BCTs to target key influences on antibiotic prescribing and the application of these techniques. Policymakers could use the suggested software features to enhance the existing e-prescribing systems and as guidance for healthcare software developers. The findings could also be applied by clinicians to assist them in evaluating the suitability and functionality of new e-prescribing systems and anticipating potential challenges.
Similar to other qualitative studies (Broom et al., Citation2016; Charani et al., Citation2013), we found that key barriers to appropriate prescribing in hospitals were clinical uncertainty, poor communication of decisions and social pressures of the hospital, including the influence of senior clinicians on junior prescribers’ decisions and the culture of ‘non-interference’. However, our findings related to the loss of ownership for antibiotic decisions due to uncertainty about the original prescription, and the fear of consequences provided new insights into individual behaviours and highlighted the need for organisational support to address the individual-level requirements (e.g. support from senior prescribers, enhancing ‘visibility’ of antibiotic decisions by harnessing the IT infrastructure). Despite international efforts suggesting that clear documentation of decisions is a key principle in advancing patient safety and improving outcomes (Centers for Disease Control & Prevention, 2019), the current study highlights that poor documentation of prescriptions remains the key driver behind the unnecessary continuation of antibiotics. Prior research has also identified inconsistencies in the provision of information between various specialities, leading to the lack of adequate information to make an appropriate decision on whether to stop, continue or alter the treatment (Charani et al., Citation2019). This was also found here and resulted in the development of one of the core components of the DARTT intervention: the antibiotic tracker—a digital system for tracking and monitoring decisions.
Evidence shows that many complex intervention trials fail to show effectiveness, which can be due to a genuine lack of intervention effect, or sub-optimal design (Levati et al., Citation2016). To reduce research waste, the MRC framework recommends addressing any uncertainties and issues concerning the acceptability, optimisation, and delivery of the intervention before the effectiveness study stage (Skivington et al., Citation2021). The involvement of key stakeholders aided the identification of any anticipated challenges to the adoption and implementation of the intervention and facilitated its refinement. Such an approach ensured that the content and delivery of DARTT were adapted during the development stage to accommodate a range of users’ needs, reinforcing the importance of the person-centred approach in the development and refinement of complex interventions (Yardley et al., Citation2015).
Digitalising the entire prescription service remains one of the key areas for improving medication management in the UK (Tolley, Citation2012). However, implementation of digital interventions in healthcare settings is challenging (Michie et al., Citation2017) and little guidance on the best method to incorporate ‘antibiotic time-out’ practice into clinical workflow is available (Thom et al., Citation2019). Our optimisation study showed that the adoption and implementation of DARTT will be dependent on an organisation-wide approach, securing buy-in from key decision-makers (e.g. senior opinion leaders, management, senior clinicians) but also strong engagement with front-line staff as well as the involvement of the patient and/or the family. The stakeholders considered the webinar and e-training essential to the promotion of the adoption of the intervention. The optimisation study further identified prescribing habits, resistance to change, social roles, norms and rules, as well as time constraints as potential barriers to the effective uptake of DARTT. Evidence shows that all of these interrelated factors, including the relationship with the environment, directly or indirectly influence individual behaviours (Thom et al., Citation2019). Therefore, when developing and evaluating digital interventions, consideration needs to be given to the relationship between the ‘micro’ level of immediate engagement with the intervention and the ‘macro’ level of engagement with long-term behaviour change (Bronfenbrenner, Citation1979). These influences could be a target for a separate intervention.
In the next phases of our research, the proposed features of the antibiotic tracker will be assessed for transferability and feasibility based on the local needs of one hospital to examine whether it results in the desired behaviour change. In addition to aiding intervention design, the BCW can be used in the intervention evaluation as it enables intervention outcomes to be linked to specific mechanisms of action and thus help diagnose where an intervention has succeeded/failed to achieve its desired goal (Michie et al., Citation2014). With the current roll-out of the hospital e-prescribing system across Scotland, DARTT is in an ideal position to help improve antibiotic reassessment behaviours at a larger scale by reaching a large number of individual prescribers. To achieve sustained behavioural change within the digital intervention context, an implementation strategy, project management team, education and promotion, effective engagement and an iterative cycle of usability testing (e.g. using in-depth iterative qualitative development with users) are recommended (Mohr et al., Citation2014).
Implications for future research
Incorporating an understanding of the socio-cultural influences on prescribing derived from this research into local policy and practice has the potential to support interventions which target individual practice, such as an active antibiotic time-out. The implications are that antibiotic prescribing is a complex behavioural problem, likely to be influenced by a range of factors at the individual (personal to prescribers), social (or cultural, determined by colleagues) and organisational level (determined by the environment). To trigger a change, a complex behavioural intervention that considers the COM-B elements, fosters collaborative culture and breaks up pre-existing prescribing habits using focused behavioural planning, is required.
By integrating published and empirical evidence, this work has demonstrated a rigorous method of developing a solid theoretical foundation for health interventions. The exploratory sequential method applied, which incorporated the views of key stakeholders, allowed effective intervention operationalisation and development. Guided by the 2008 MRC framework, the systematic approach taken—starting from problem identification to designing the intervention content—presents an appealing case for applying to research funding bodies. Drawing on the findings, future research can use the described modelling process and the generated insights to assess the effectiveness of a behaviour change intervention.
Strengths and limitations
This study used a systematic and structured approach to integrate a body of evidence from a systematic review and meta-ethnography, two qualitative studies, and end-users’ expert knowledge to co-design and develop a novel theory-informed complex health intervention. The uniqueness of this work lies in adhering to the MRC guidance by using a theoretical approach, which lent direction, structure, and transparency to this process. Hospital-based AMS interventions and digital interventions supporting AMS have been the focus of previous research (Van Dort et al., Citation2022). However, no digital health intervention to date has specifically focused on initiating timely antibiotic time-out and improving documentation of decisions using behavioural science. Another strength of this study was the inductive qualitative research conducted with the key stakeholders (HCPs and patient and public representatives) throughout the planning, development and optimisation of DARTT, which previously has been lacking in this area of research (Rawson et al., Citation2017a). The use of the Design Sprint methodology (Banfield et al., Citation2015) during the optimisation phase enabled the research team to understand and address users’ concerns about the intervention, ensuring that it is useful and relevant for clinical practice. Implementing the advice can also help to earn users’ trust by showing them that they are understood and listened to (Yardley et al., Citation2015). This worked example of how to apply pragmatic research methods to integrate the evidence with stakeholders’ engagement can be modified and adapted to suit different contexts and populations.
Following the BCW steps allowed us to identify the behaviour that needed changing and select preferred intervention solutions. However, despite the highly structured and logical approach of the BCW, there are challenges associated with its use. For example, understanding the complex terminology was challenging, and the research team had to make a series of subjective and pragmatic decisions throughout the process of applying the labels to component techniques. While the use of the APEASE criteria was helpful in reducing some subjectivity, the process involved forecasting by the research team and stakeholders and this forecasting may not always be accurate (Ekberg et al., Citation2021). Similar to others, we found that the use of TDF and BCTTv1 requires skill and training and the process of translating BCTs into intervention content can be open to interpretation (Ojo et al., Citation2019). Due to a degree of overlap across some BCT labels and ambiguity of definitions, the process of coding and achieving good levels of reliability in BCT selection raised some issues. For example, finding a ‘perfect fit’ for some BCTs with varying complexity (e.g. instruction on how to perform behaviour and behavioural practice/rehearsal) and operationalising the less frequently used techniques within a digital behaviour change intervention was not straightforward. The absence of formulated techniques to guide the development of digital health interventions highlights current gaps in the field of behaviour change.
Furthermore, although the BCW describes very carefully the environmental issues in the middle and outside circles, the BCTs are all focused on the individual. However, according to Bandura’s social learning theory (Bandura & Schrenk, Citation1981), individual behaviour is dependent on interaction with the surroundings. To overcome this challenge, we drew on the social determinants and dynamic relationships of the hospital environment identified in our meta-ethnography (Wojcik et al., Citation2021). To modify the environment, we thus included BCTs, such as restructure the physical environment and add objects to the environment. The BCT instruction on how to perform behaviour was specifically selected to influence cognitive factors to predispose prescribers to be able to perform the desired behaviour, whilst the techniques demonstration of the behaviour, habit reversal, and behavioural practice/rehearsal were chosen to help prescribers weaken the negative knowledge acquired previously (i.e. prescribing habits) and increase their motivation and self-efficacy to perform the desired behaviour.
In addition, the multiple steps of our intervention development was a lengthy process—from initial systematic review and meta-ethnography to the final refinements of the intervention took over three years. Although the iterative process increased research rigour, it slowed down the intervention development at points. The COVID-19 pandemic also impacted progress when research activities were temporarily suspended. Although the steps taken ensured that the intervention is theoretically based, such a lengthy process must be taken into account by those considering undertaking and funding such evidence-based intervention development in future.
Conclusions
This paper describes how the MRC guidance and the BCW were systematically applied to develop a theory-informed, complex behaviour change intervention that is innovative and practical. The COM-B and TDF were used to map important barriers and facilitators relating to timely antibiotic review in acute hospitals and generated the theoretical basis for refining the intervention. By working closely with a range of HCPs and health service users, an inductive sequential research approach was used to gather and validate evidence, generate new ideas, and develop the DARTT intervention to improve antibiotic review practice. Initial exploration of DARTT using cost-effective and efficient methods has indicated that the proposed intervention is acceptable to the target audience, suitable for clinical practice, and potentially effective in improving the quality of the antibiotic review. Applying transparent and systematic methods in the development process ensured that the findings are replicable and could be modified in the implementation stages without losing methodological rigour.
Supplemental Material
Download MS Word (62.2 KB)Acknowledgments
The authors would like to acknowledge and thank all patients and healthcare professionals who participated in this study. Special thanks to Dr Donald Inverarity and Dr Graeme Grandison for additional insight and expertise.
Disclosure statement
No potential conflict of interest was reported by the authors.
Data availability statement
The data supporting the findings of this study are available from the corresponding author [GW] on request.
Additional information
Funding
References
- Ashiru-Oredope, D., Sharland, M., & Charani, E. (2012). Improving the quality of antibiotic prescribing in the NHS by developing a new antimicrobial stewardship programme: Start Smart-Then Focus. Journal of Antimicrobial Chemotherapy, 67, 51–63.
- Bandura, A., & Schrenk, D. (1981). Cultivating competence, self-efficacy, and intrinsic interest through proximal self-motivation. Journal of Personality and Social Psychology, 41, 586–597.
- Banfield, R., Lombardo, C. T., & Wax, T. (2015). Design sprint: A practical guidebook for building great digital products. O’Reilly Media.
- Barber, S., & Swaden-Lewis, K. (2017). Briefing paper, antimicrobial resistance - House of commons library. https://commonslibrary.parliament.uk/research-briefings/cbp-8141/
- Barker, F., De Lusignan, S., & Cooke, D. (2018). Improving collaborative behaviour planning in adult auditory rehabilitation: Development of the i-plan intervention using the behaviour change wheel. Annals of Behavioral Medicine, 52(6), 489–500. https://doi.org/10.1007/s12160-016-9843-3
- Bronfenbrenner, U. (1979). The ecology of human development: Experiments by nature and design. Harvard University Press.
- Broom, A., Broom, J., Kirby, E., & Adams, J. (2016). The social dynamics of antibiotic use in an Australian hospital. Journal of Sociology, 52, 824–839. https://doi.org/10.1177/1440783315594486
- Cane, J., Richardson, M., Johnston, M., Ladha, R., & Michie, S. (2015). From lists of behaviour change techniques (BCTs) to structured hierarchies: Comparison of two methods of developing a hierarchy of BCTs. British Journal of Health Psychology, 20(1), 130–150. https://doi.org/10.1111/bjhp.12102
- Cassini, A., Högberg, L. D., Plachouras, D., Quattrocchi, A., Hoxha, A., Simonsen, G. S., Colomb-Cotinat, M., Kretzschmar, M. E., Devleesschauwer, B., Cecchini, M., Ouakrim, D. A., Oliveira, T. C., Struelens, M. J., Suetens, C., & Monnet, D. L., Burden of AMR Collaborative Group. (2019). Attributable deaths and disability-adjusted life-years caused by infections with antibiotic-resistant bacteria in the EU and the European Economic Area in 2015: A population-level modelling analysis. The Lancet. Infectious Diseases, 19(1), 56–66. https://doi.org/10.1016/S1473-3099(18)30605-4
- Centers for Disease Control and Prevention. (2019). Core elements of hospital antibiotic stewardship programs. https://www.cdc.gov/antibiotic-use/healthcare/pdfs/hospital-core-elements-H.pdf
- Charani, E., Castro-Sanchez, E., Sevdalis, N., Kyratsis, Y., Drumright, L., Shah, N., & Holmes, A. (2013). Understanding the determinants of antimicrobial prescribing within hospitals: The role of “prescribing etiquette”. Clinical Infectious Diseases, 57(2), 188–196. https://doi.org/10.1093/cid/cit212
- Charani, E., de Barra, E., Rawson, T. M., et al. (2019). Antibiotic prescribing in general medical and surgical specialties: A prospective cohort study. Antimicrobial Resistance & Infection Control, 8, 1–10. https://doi.org/10.1186/s13756-019-0603-6
- Charani, E., Edwards, R., Sevdalis, N., Alexandrou, B., Sibley, E., Mullett, D., Franklin, B. D., & Holmes, A. (2011). Behaviour change strategies to influence antimicrobial prescribing in acute care: A systematic review. Clinical Infectious Diseases, 53(7), 651–662. https://doi.org/10.1093/cid/cir445
- Chaves, N. J., Cheng, A. C., Runnegar, N., Kirschner, J., Lee, T., & Buising, K. (2014). Analysis of knowledge and attitude surveys to identify barriers and enablers of appropriate antimicrobial prescribing in three Australian tertiary hospitals. Internal Medicine Journal, 44(6), 568–574. https://doi.org/10.1111/imj.12373
- Connell, L. E., Carey, R. N., de Bruin, M., Rothman, A. J., Johnston, M., Kelly, M. P., & Michie, S. (2019). Links between behaviour change techniques and mechanisms of action: An expert consensus study. Annals of Behavioral Medicine, 53(8), 708–720. https://doi.org/10.1093/abm/kay082
- Courtenay, M., Lim, R., Deslandes, R., Ferriday, R., Gillespie, D., Hodson, K., Reid, N., Thomas, N., & Chater, A. (2019). Theory-based electronic learning intervention to support appropriate antibiotic prescribing by nurses and pharmacists: Intervention development and feasibility study protocol. BMJ Open, 9(8), e028326. https://doi.org/10.1136/BMJOPEN-2018-028326
- Craig, P., Dieppe, P., Macintyre, S., Michie, S., Nazareth, I., & Petticrew, M., Medical Research Council Guidance. (2008). Developing and evaluating complex interventions: New guidance. BMJ (Clinical Research ed.), 337, a1655–6. https://doi.org/10.1136/bmj.a1655
- Davey, P., Marwick, C. A., Scott, C. L., Charani, E., McNeil, K., Brown, E., Gould, I. M., Ramsay, C. R., & Michie, S. (2017). Interventions to improve antibiotic prescribing practices for hospital inpatients. The Cochrane Database of Systematic Reviews, 2(2), CD003543. https://doi.org/10.1002/14651858.CD003543.pub4
- Department of Health. (2019). UK 5-year action plan for antimicrobial resistance, 2019 to 2024. https://www.gov.uk/government/publications/uk-5-year-action-plan-for-antimicrobial-resistance-2019-to-2024
- Dombrowski, S. U., O’Carroll, R. E., & Williams, B. (2016). Form of delivery as a key ‘active ingredient’ in behaviour change interventions. British Journal of Health Psychology, 21(4), 733–740. https://doi.org/10.1111/bjhp.12203
- Ekberg, K., Timmer, B., Schuetz, S., & Hickson, L. (2021). Use of the behaviour change wheel to design an intervention to improve the implementation of family-centred care in adult audiology services. International Journal of Audiology, 60(sup2), 20–29. https://doi.org/10.1080/14992027.2020.1844321
- French, S. D., Green, S. E., O’Connor, D. A., McKenzie, J. E., Francis, J. J., Michie, S., Buchbinder, R., Schattner, P., Spike, N., & Grimshaw, J. M. (2012). Developing theory-informed behaviour change interventions to implement evidence into practice: A systematic approach using the Theoretical Domains Framework. Implementation Science: IS, 7, 38–38. https://doi.org/10.1186/1748-5908-7-38
- Gaynes, R. (2017). The discovery of Penicillin - New insights after more than 75 years of clinical use. Emerging Infectious Diseases, 23, 849–853. https://doi.org/10.3201/eid2305.161556
- Glasgow, R. E., Vogt, T. M., & Boles, S. M. (1999). Evaluating the public health impact of health promotion interventions: The RE-AIM framework. American Journal of Public Health, 89(9), 1322–1327. https://doi.org/10.2105/ajph.89.9.1322
- Graber, C. J., Jones, M. M., Glassman, P. A., Weir, C., Butler, J., Nechodom, K., Kay, C. L., Furman, A. E., Tran, T. T., Foltz, C., Pollack, L. A., Samore, M. H., & Goetz, M. B. (2015). Taking an antibiotic time-out: Utilisation and usability of a self-stewardship time-out program for renewal of vancomycin and piperacillin-tazobactam. Hospital Pharmacy, 50(11), 1011–1024. https://doi.org/10.1310/hpj5011-1011
- Hulscher, M. E., & Prins, J. M. (2017). Antibiotic stewardship: Does it work in hospital practice? A review of the evidence base. Clinical Microbiology and Infection, 23(11), 799–805. https://doi.org/10.1016/j.cmi.2017.07.017
- Hulscher, M. E., Grol, R. P., & van der Meer, J. W. (2010). Antibiotic prescribing in hospitals: A social and behavioural scientific approach. The Lancet. Infectious Diseases, 10(3), 167–175. https://doi.org/10.1016/S14733099(10)70027-X
- Johnson, B. J., Zarnowiecki, D., Hendrie, G. A., Mauch, C. E., & Golley, R. K. (2018). How to reduce parental provision of unhealthy foods to 3- to 8-year-old children in the home environment? A systematic review utilizing the Behaviour Change Wheel framework. Obesity Reviews, 19(10), 1359–1370. https://doi.org/10.1111/obr.12702
- Krockow, E. M., Colman, A. M., Chattoe-Brown, E., Jenkins, D. R., Perera, N., Mehtar, S., & Tarrant, C. (2019). Balancing the risks to individual and society: A systematic review and synthesis of qualitative research on antibiotic prescribing behaviour in hospitals. The Journal of Hospital Infection, 101(4), 428–439. https://doi.org/10.1016/j.jhin.2018.08.007
- Levati, S., Campbell, P., & Frost, R. (2016). Optimisation of complex health interventions prior to a randomised controlled trial: A scoping review of strategies used. Pilot and Feasibility Studies, 2, 1–17. https://doi.org/10.1186/s40814-016-0058-y
- Lorencatto, F., Charani, E., Sevdalis, N., Tarrant, C., & Davey, P. (2018). Driving sustainable change in antimicrobial prescribing practice: How can social and behavioural sciences help? The Journal of Antimicrobial Chemotherapy, 73(10), 2613–2624. https://doi.org/10.1093/jac/dky222
- Michie, S., Atkins, L., & West, R. (2014). The behaviour change wheel: A guide to designing interventions. Silverback Publishing.
- Michie, S., Richardson, M., Johnston, M., Abraham, C., Francis, J., Hardeman, W., Eccles, M. P., Cane, J., & Wood, C. E. (2013). The behaviour change technique taxonomy (v1) of 93 hierarchically clustered techniques: Building an international consensus for the reporting of behaviour change interventions. Annals of Behavioral Medicine, 46(1), 81–95. https://doi.org/10.1007/s12160-013-9486-6
- Michie, S., van Stralen, M. M., & West, R. (2011). The behaviour change wheel: A new method for characterising and designing behaviour change interventions. Implementation Science: IS, 6, 42–12. https://doi.org/10.1186/1748-5908-6-42
- Michie, S., Yardley, L., West, R., et al. (2017). Developing and evaluating digital interventions to promote behaviour change in health and health care: Recommendations resulting from an international workshop. Journal of Medical Internet Research, 19, e232. https://doi.org/10.2196/jmir.7126
- Mohr, D. C., Schueller, S. M., Montague, E., et al. (2014). The behavioural intervention technology model: An integrated conceptual and technological framework for eHealth and mHealth interventions. Journal of Medical Internet Research, 16, e146. https://doi.org/10.2196/jmir.3620
- Monnier, A. A., Eisenstein, B. I., Hulscher, M. E., & Gyssens, I. C. (2018). Towards a global definition of responsible antibiotic use: Results of an international multidisciplinary consensus procedure. Journal of Antimicrobial Chemotherapy, 73, 3–16. https://doi.org/10.1093/jac/dky114
- National Institute for Health and Care Excellence. NICE impact antimicrobial resistance. (2018). https://www.nice.org.uk/media/default/about/what-we-do/into-practice/measuring-uptake/niceimpact-antimicrobial-resistance.pdf
- Noblit, G. W., & Hare, R. D. (1988). Meta-ethnography: Synthesizing qualitative studies. SAGE Publications Ltd.
- Ojo, S. O., Bailey, D. P., Brierley, M. L., Hewson, D. J., & Chater, A. M. (2019). Breaking barriers: Using the behaviour change wheel to develop a tailored intervention to overcome workplace inhibitors to breaking up sitting time. BMC Public Health, 19, 1–17. https://doi.org/10.1186/s12889-019-7468-8
- Pinder, R., Sallis, A., Berry, D., & Chadborn, T. (2015). Behaviour change and antibiotic prescribing in healthcare settings Literature review and behavioural analysis. Public Health England. https://www.gov.uk/government/publications/antibiotic-prescribing-and-behaviour-change-in-healthcare-settings
- Public Health England. (2020). English Surveillance Programme for Antimicrobial Utilisation and Resistance (ESPAUR). Report 2019 to 2020. https://assets.publishing.service.gov.uk/government/uploads/system/uploads/attachment_data/file/936199/ESPAUR_Report_2019-20.pdf
- Ramsay, C., Brown, E., Hartman, G., & Davey, P. (2003). Room for improvement: A systematic review of the quality of evaluations of interventions to improve hospital antibiotic prescribing. The Journal of Antimicrobial Chemotherapy, 52(5), 764–771. https://doi.org/10.1093/JAC/DKG460
- Rawson, T. M., Moore, L. S. P., Hernandez, B., Charani, E., Castro-Sanchez, E., Herrero, P., Hayhoe, B., Hope, W., Georgiou, P., & Holmes, A. H. (2017a). A systematic review of clinical decision support systems for antimicrobial management: Are we failing to investigate these interventions appropriately? Clinical Microbiology and Infection, 23(8), 524–532. https://doi.org/10.1016/j.cmi.2017.02.028
- Rawson, T. M., Moore, L. S. P., Tivey, A. M., Tsao, A., Gilchrist, M., Charani, E., & Holmes, A. H. (2017b). Behaviour change interventions to influence antimicrobial prescribing: A cross-sectional analysis of reports from UK state-of-the-art scientific conferences. Antimicrobial Resistance and Infection Control, 6, 11–18. https://doi.org/10.1186/s13756-017-0170-7
- Ritchie, J., & Lewis, J. (2013). Qualitative research practice: A guide for social science students and researchers (2nd ed.). SAGE Publications Ltd.
- Russell, C. D., Fairfield, C. J., Drake, T. M., et al. (2021). Co-infections, secondary infections, and antimicrobial use in patients hospitalised with COVID-19 during the first pandemic wave from the ISARIC WHO CCP-UK study: A multicentre, prospective cohort study. Lancet Microbe, 2, 1–12. https://doi.org/10.1016/S2666-5247(21)00090-2
- Shallcross, L., Lorencatto, F., Fuller, C., Tarrant, C., West, J., Traina, R., Smith, C., Forbes, G., Crayton, E., Rockenschaub, P., Dutey-Magni, P., Richardson, E., Fragaszy, E., Michie, S., & Hayward, A., PASS Research Group. (2020). An interdisciplinary mixed-methods approach to developing antimicrobial stewardship interventions: Protocol for the preserving antibiotics through safe stewardship (PASS) research programme. Wellcome Open Research, 5, 8–14. https://doi.org/10.12688/wellcomeopenres.15554.1
- Skivington, K., Matthews, L., Simpson, S. A., Craig, P., Baird, J., Blazeby, J. M., Boyd, K. A., Craig, N., French, D. P., McIntosh, E., Petticrew, M., Rycroft-Malone, J., White, M., & Moore, L. (2021). A new framework for developing and evaluating complex interventions: Update of Medical Research Council guidance. BMJ (Clinical Research ed.), 374, n2061–11. https://doi.org/10.1136/bmj.n2061
- Teixeira Rodrigues, A., Roque, F., Falcão, A., Figueiras, A., & Herdeiro, M. T. (2013). Understanding physician antibiotic prescribing behaviour: A systematic review of qualitative studies. International Journal of Antimicrobial Agents, 41(3), 203–212. https://doi.org/10.1016/j.ijantimicag.2012.09.003
- Thom, K. A., Tamma, P. D., Harris, A. D., et al. (2019). Impact of a prescriber-driven antibiotic time-out on antibiotic use in hospitalized patients. Clinical Infectious Diseases, 68, 1581–1584. https://doi.org/10.1093/cid/ciy85
- Tolley, R. (2012). An overview of e-prescribing in secondary care. Nursing Standard (Royal College of Nursing (Great Britain): 1987), 26(22), 35–38. https://doi.org/10.7748/ns2012.02.26.22.35.c8920
- Van Dort, B. A., Penm, J., Ritchie, A., & Baysari, M. T. (2022). The impact of digital interventions on antimicrobial stewardship in hospitals: A qualitative synthesis of systematic reviews. Journal of Antimicrobial Chemotherapy, 7, 1828–1837. https://doi.org/10.1093/jac/dkac112
- Walker, A. S., Budgell, E., & Laskawiec-Szkonter, M. (2019). Antibiotic Review Kit for Hospitals (ARK-Hospital): Study protocol for a stepped-wedge cluster-randomised controlled trial. Trials, 20, 1–16. https://doi.org/10.1186/s13063-019-3497-y
- West, R., Michie, S., Atkins, L., Chadwick, P., & Lorencatto, F. (2020). Achieving behaviour change: A guide for local government and partners. Public Health England. https://www.gov.uk/government/publications/behaviour-change-guide-for-local-government-and-partners
- Wojcik, G., Ring, N., McCulloch, C., Willis, D. S., Williams, B., & Kydonaki, K. (2021). Understanding the complexities of antibiotic prescribing behaviour in acute hospitals: A systematic review and meta-ethnography. Arch Public Health, 79, 1–19. https://doi.org/10.1186/S13690-021-00624-1
- World Health Organization. (2016). Health in the post-2015 development agenda: Need for a social determinants health approach. https://www.who.int/health-topics/social-determinants-of-health#tab=tab_1
- Yardley, L., Ainsworth, B., Arden-Close, E., & Muller, I. (2015). The person-based approach to enhancing the acceptability and feasibility of interventions. Pilot and Feasibility Studies, 1, 1–7. https://doi.org/10.1186/s40814-015-0033-z