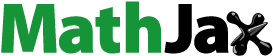
ABSTRACT
This research proposes a multi-period multiple parts mixed-integer linear programming optimization model for the trade-off analysis of spare parts supply through computer numerical control (CNC) manufacturing and additive manufacturing (AM). The multiple spare parts have different characteristics such as volume, shape size, and geometry complexity. The model focuses on minimizing lead times and thus reducing downtime costs. Scenario analyses are developed for some parameters to assess the robustness of the model. The analysis shows that the mix between AM-based spare parts and CNC-based spare parts is sensitive to changes in demand. For the given data, the findings demonstrate that AM is cost-effective with spare parts having high geometry complexity while CNC-based manufacturing is economically feasible for spare parts with low geometry complexity and large sizes. The proposed model can support decision-makers in selecting the optimal manufacturing method for multiple spare parts having different characteristics and attributes. The paper concludes with limitations and future directions.
1. Introduction
In large-scale applications, the unavailability of critical spare parts can have a significant impact on operations continuity and increase downtime costs, affecting the company’s profit. For example, in the oil and gas industry, downtime can cost up to 1 million USD per hour. To mitigate such a downtime situation, companies store large amounts of safety stock, particularly for spare parts that have long replenishment lead times and require specific raw materials for production (Chaudhuri et al. Citation2020). One way to overcome such a problem is to adopt additive manufacturing (AM) technology along with conventional manufacturing (CM).
AM has emerged as an industrial manufacturing technology for producing functional parts. The application of AM in the spare parts businesses contributes to cutting lead times and boosting supply chain efficiency (Boer, Lambrechts, and Krikke Citation2020). AM technology allows for customization in designs and the production of products with high geometric complexity. It is currently being adopted in several industries including aerospace, defense, military, automotive, and healthcare (Mecheter Citation2023). However, the adoption of AM in the oil and gas industry is still being explored (Vendra and Achanta Citation2020). In the aviation sector, AM has contributed to weight reduction of some parts. There are also examples of AM being used in medical applications, such as hearing aids, orthopedic implants, and prosthetics (Knofius, van der Heijden, and Zijm Citation2019). Metal AM has made it possible to produce metallic functional parts and components through 3D printing, enabling direct digital manufacturing (Kerbrat, Mognol, and Hascoët Citation2011). The use of metal AM is on the rise, with an increasing number of companies selling metal-based spare parts through this technology (Wohlers Citation2017). Metal AM is being adopted in various industries, including aerospace, energy, defense, and biomedical applications.
AM generates less waste and material scraping compared to other manufacturing methods (Liu et al. Citation2014). It also allows for customization and freedom in part design, making it possible to 3D print parts with internal cavities and complex geometries that are difficult to produce via traditional methods (Manogharan, Wysk, and Harrysson Citation2016). Furthermore, the carbon footprint of AM processes is lower compared to other manufacturing methods, as AM can have a near-optimal buy-to-fly ratio (Deppe and Koch Citation2014; Portolés et al. Citation2016). AM can also reduce the inventory of raw materials and its associated costs, including acquisition, and inventory handling costs. Rather than holding a large amount of physical stock of spare parts, the raw material for AM requires less space and allows for the production of a wide range of products. However, AM raw materials are usually costly, and AM suppliers are not very widespread (Kunovjanek, Knofius, and Reiner Citation2020).
AM has been adopted in parallel with CM methods. The integration of AM and CM methods can increase the robustness and flexibility of supply chains and avoid supply disruptions (Jin, He, and Du Citation2017). However, the choice would depend on the economic benefits of the whole supply chain. Therefore, this paper contributes by developing a generic framework for AM and CNC-based spare parts acquisition and development. A deterministic discrete multi-period mixed integer linear programming (MILP) for multiple spare parts supply chains is proposed to analyze the problem and solutions are developed through an IBM CPLEX OPL platform. This work aims to address the following research questions:
RQ1:
What framework is available for considering and analyzing spare parts supply chains for AM?
RQ2:
What modeling process is suitable to assess the trade-offs in the manufacturing processes?
The remainder of this paper is organized as follows. Section 2 reviews the related work and presents the research gaps. Section 3 illustrates the adopted methodology of the proposed work along with the mathematical formulation and data collection. Section 4 includes numerical experiments of the model, scenario analysis, discussion, and managerial insights into the model. In Section 5, a summary of the whole work, the limitations of the study, and future work are provided.
2. Literature review
Recent studies are investigating the adoption of AM technology in the spare parts supply chain by incorporating quantitative modeling and optimization methods. Marinho De Brito et al. (Citation2021) have studied the optimal deployment of 3D printers in different facilities for spare parts production through the utilization of classical p‐median, location-allocation modeling, and MILP. The model was tested for the optimal scenario through an elevator maintenance case study with nine production centers, each having a 3D printer. The authors have mentioned that this type of optimal analysis would help companies manage challenges at different locations where AM is implemented.
Knofius, van der Heijden, and Zijm (Citation2019) have developed a stochastic dynamic programming model to investigate the profitability of AM. The authors mentioned that sourcing with AM is better when the purchase price, demand, and backorder costs of a spare are high. The authors also mentioned that dual sourcing using AM and CM methods remains profitable. They state that AM is preferably used for urgent supply cases.
In another study, Knofius et al. (Citation2020) studied the value of sourcing spare parts using a mix of AM and CM methods in the aerospace industry. The authors have developed a Markov decision process-based optimization model to determine the optimal inventory policy, optimal sourcing, and maintenance strategy. The authors have assumed that the AM part reliability is inferior to that of CM. The analysis shows that dual sourcing outperforms single sourcing, and it is more beneficial when AM has short resupply lead times.
Cestana et al. (Citation2019) developed a cost minimization problem to investigate the effect of resupply time of different technologies, namely AM and CM. The authors have mentioned that AM is better than CM in inventory performance because of the lower stock level with AM and the longer setup times of CM.
Westerweel et al. (Citation2020) studied optimal inventory policy with an infinite time horizon to investigate on‐site AM production in remote locations. The model helps determine when to print a part and when to wait for its scheduled replenishment. The analysis showed that AM spare parts were the best solution to avoid stock‐out between the two replenishment periods. However, whenever the CM parts arrived, the AM parts were replaced as the reliability of AM parts was assumed to be inferior to CM ones.
Cardeal et al. (Citation2021) investigated the application of AM in maintenance applications in the process industry. As the process industry is known for high volumes of production with large stocks of spare parts, costly machines, and downtime, the authors have found that integrating AM in maintenance activities is very appealing. Their analysis has focused on the viability of AM to change maintenance activities and supply chains in the process industry. The authors conclude that AM technology is only cost-competitive for spare parts with low annual demand patterns and low production volumes.
Emelogu et al. (Citation2016) proposed a deterministic programming model which was later extended to stochastic programming to investigate the AM adoption in manufacturing biomedical implants for hospitals. The study suggested a harmonious implementation of AM and CM production where demands of biomedical implants can be fulfilled using both AM and CM depending on the product’s lead time, patient location, and AM facility capacity.
Cantini et al. (Citation2022) proposed a decision support system to decide on the optimal spare parts supply chain configuration. This decision support system assists also in the selection of the manufacturing technology of spare parts where they can be manufactured either through AM or CM. The authors mention that there is a possibility of producing spare parts through AM rather than CM as AM technology is more suitable under specific conditions. lists the existing optimization models in the literature along with their structure including approach, stage, objective, parameters, and period. It can be observed that most studies focus on cost minimization and are developed for a single period.
Table 1. Optimization models.
The proposed model offers two improvements over existing models. First, it considers a multi-period planning horizon, enabling decision-making over a longer timeframe. Second, it takes into account a wider range of cost items, reflecting the entire supply chain and allowing for a more comprehensive cost analysis. Together, these features make the model a more robust and effective tool for adopting AM in supply chain operations.
Quantitative models are increasingly being used to analyze the role of AM in supply chains. However, existing quantitative models lack complexity and do not consider the impact of lead time (Cardeal et al. Citation2021). The current literature lacks quantitative methods that capture the differences between AM and CM supply chains, making it difficult for practitioners and decision-makers to determine the optimal manufacturing technology (Cantini et al. Citation2022).
Therefore, this paper contributes by the following:
A comprehensive and generic framework that considers various aspects of AM adoption in the spare parts supply chain.
A MILP optimization model based on the proposed framework to analyze the trade-offs between CNC and AM manufacturing while considering multiple spare parts with various characteristics, such as shape, size, geometry complexity, volume, and cost.
Extension of the proposed MILP model to a multi-period planning horizon and considering various cost items such as purchase, production, inventory holding, transportation, lead time penalty, backorder, maintenance, and depreciation costs providing decision makers with a holistic and accurate view of their supply chain operations.
3. Materials and methods
This section will present the adopted methodology to develop the framework and model in this study.
3.1. Framework development
The proposed framework of this study handles various aspects of AM adoption in the spare parts supply chain. The BLOC-ICE concept as proposed by Pokharel (Citation2023) and illustrated in , is used to describe the proposed AM-based supply chain. A detailed review of all aspects, including inputs, processes, constraints, and outputs, is provided by Mecheter, Pokharel, and Tarlochan (Citation2022).
The proposed optimization model is based on the manufacturing strategy mentioned in , taking into account the inputs (demand, material, and supply potential), constraints (rules and regulations, sourcing, and AM technology), processes (manufacturing, logistics, and inventory control), and the output (AM spare parts).
3.2. Optimization methodology
The proposed model represents the situation of combining two manufacturing methods to meet the demand for spare parts. It provides a solution for the optimal manufacturing method of each spare part type in each period of the planning horizon. For the implementation, the model considers nine different types of spare parts, each with a different purchase cost, different lead time delivery from the CNC supplier, and AM printing time based on their characteristics related to geometry complexity level and spare part size. The trade-off between AM and CNC methods is formulated as a MILP problem. The main objective of the model is to minimize the operational costs of both manufacturing methods, including purchase, ordering, and transportation costs of CNC-manufactured spare parts, purchase and transportation of AM raw material, production costs of AM-produced parts, and penalty costs of lead time and back ordered demand. shows the specific inputs and outputs of the analysis process.
The objective of the optimization model is to minimize the sum of several cost items related to purchase, ordering, transportation, inventory holding, lead-time, and backorder penalty costs. The outcome of the model will be the decision on the optimal quantity of spare parts to be sourced from the CNC supplier and the number of spare parts to be on-site produced through AM. shows a graphical representation of the supply chain flow of the proposed optimization problem. CNC-based spare parts are ordered from the CNC supplier and transported to the main facility. Similarly, the raw material for AM, which is metal powder, is ordered from the supplier and delivered to the facility. The warehouse is common for both CNC parts and AM metal powder. In the AM facility, the AM parts are fabricated through a metal 3D printer where skilled operators are available for the setup of the printer and post-processing operations.
3.3. Modeling and data collection assumptions
A few key assumptions are made for the development of the model and data collection, and they are described below.
3.3.1. Modeling assumptions
The following basic assumptions are made for the development of the MILP model.
The planning horizon of the study is set as one year in accordance with the procurement cycle usually adopted in the companies.
At the start of each period, the demand for each of the nine spare parts is generated, and each demand can be fulfilled using AM, CNC, or both manufacturing methods.
All spare parts are produced through AM (after post-processing) and CNCmethods and they are equivalent in quality and reliability.
CNC-based spare parts are delivered by an external supplier, while AM-based spare parts are fabricated on demand in the facility.
Both the CNC spare parts and AM raw materials are delivered at the beginning of each period.
All spare parts are single components made of the same material (thus requiring no assembly in the case of AM).
Warehouse capacity is combined for both CNC-based parts and AM raw materials, and can be allocated by spare parts, raw materials, or both.
3.3.2. Data related assumptions
The following assumptions are made regarding the data for the application of the model.
For the application of the model, nine spare parts are defined and they are categorized based on three levels of geometry complexity and three sizes, following the suggestion by Shehadeh (Citation2019). lists the spare parts-related characteristics and data which are obtained from Shehadeh (Citation2019). As shown in , three levels of complexity are defined, and they are based on the geometry of the part.
Spare parts demand is considered deterministic and follows a Poisson distribution over the given planning horizon. It is to note that Cantini et al. (Citation2022), Sgarbossa et al. (Citation2021), and Knofius, Heijden, & Zijm (Citation2019) have also assumed a Poisson distribution for spare parts demand arrival. Lambda (λ), the mean number of events that occur in a given interval of time, is set to 40 units based on the randomization of demand.
Demand values are assumed to fluctuate within a ±10% range.
Transportation costs for AM raw materials are based on consignment volume shipment, while transportation costs for CNC-based spare parts are based on quantity.
The lead time for CNC-based spare parts is shipment-based and not quantity-dependent. The lead time is assumed to be constant for each particular spare part, as the contract between the supplier and customer is valid for a given lead time, so operations can continue.
The lead time to print AM-based spare parts is deterministic and is adopted from Shehadeh (Citation2019). The lead time for AM-based spare parts includes the setup time of production, printing time, and post-processing time of the spare parts.
The production cost of CNC-based spare parts is adopted from Shehadeh (Citation2019). For model application, an additional 10% is added to get the purchase price.
The production costs of AM-based spare parts are adopted from Shehadeh (Citation2019) and include energy costs, inert assistant gas costs (argon), and post-processing costs.
The lead time penalty is assumed to be a percentage fraction of the spare part’s purchase/production cost. Emelogu et al. (Citation2016) mentioned a range of this percentage to be between 10% and 270% of the monetary value per unit. Due to the immediate need for the spare parts, a monetary value of 250% of the spare part cost is assumed for calculating the lead time penalty cost per unit of time.
The supply capacity of AM metal powder is assumed to be constant over the planning horizon. The supply capacity of CNC-based spare parts is different for each spare part type and is assumed to be in the range of ±10% of the lambda demand value (λ = 40).
The warehouse capacity for storage is assumed to be 2×2x2 cubic meters. According to Cantini et al. (Citation2022), warehouses in spare parts supply chains are assumed to have unlimited capacities.
The depreciation cost of the AM machine is 20% of the capital machine investment in the first year of usage. Given the capital investment of AM machine, 1,369,863 USD, the depreciation expense in the first year is about 273,973 USD. Since the planning horizon of the study is one year, monthly depreciation expenses are assumed to decrease gradually throughout the year.
Table 2. Spare parts data.
3.4. Modelling formulation
The notations for sets, parameters, and decision variables to be used in the model, the formulation of the model objectives, and constraints are discussed in this section.
3.4.1. Notations
All the notations which are used in the formulas are summarized in .
Table 3. The notations used in the model.
3.4.2. Objective function
The objective function for cost minimization is given in EquationEquation (1)(1)
(1) with the purchase price of CNC-based spare parts (
, production cost of AM-based spare parts (
, transportation and ordering cost of CNC-based spare parts (
, operational costs of AM-based spare parts (
), purchase cost of AM raw materials (
), ordering, and transportation cost of AM raw materials (
), inventory holding cost for AM raw materials and CNC-based spare parts (
), penalty cost of lead time of CNC-based spare parts (
), penalty cost of lead time of AM-based spare parts (
), penalty of backordered demand (
), monthly depreciation and maintenance expenses of AM machine (
), and selling cost of remaining raw material and CNC-based parts at the end of the planning horizon (
).
3.4.3. Constraints
Constraint (14) converts the raw material volume of the particular spare part to spare parts quantity in units. Constraint (15) converts the inventory of CNC-based spare parts from cubic meter volume to quantity in units. Constraint (16) ensures that no inventory of AM raw material or CNC-based spare parts will be left at the end of the planning horizon. Constraint (17) sets the initial inventory of both AM raw material and CNC-based spare parts at the warehouse equal to zero. Constraint (18) ensures that no back-ordered demand for spare parts is left at the end of the planning horizon. Constraint (19) sets the initial back-ordered demand level equal to zero. Constraint (20) ensures that the inventory level has to be less than or equal to the storage capacity of the warehouse. Constraints (21) and (22) represent the supplier capacity constraints. Constraint (21) ensures that the ordered quantity of CNC-based spare parts does not exceed the supplier capacity of CNC-based spare parts. Constraint (22) ensures that the volume of ordered raw material of AM does not exceed the supplier capacity of AM metal powder. Constraint (23) represents the inventory balance constraint where the inventory level equals the summation of the produced quantity of AM-based spare parts, the sourced quantity of CNC-based spare parts, the back-ordered demand level, and the previous period’s inventory which are subtracted from the demand level and the previous period’s back-ordered demand. Constraint (24) is the non-negativity constraint.
4. Data analysis and results
4.1. Numerical experiments
The data used for the numerical experiments are listed in . The problem was coded and solved using IBM ILOG CPLEX Optimization Studio version 22.1.0.0 (ILOG CPLEX Optimization Studio | IBM Citation2022). The coding was done on a Windows 11 operating system with 16 GB of RAM and an Intel Core i7 CPU.
Table 4. Data used in parameter values.
4.2. Model solution
The proposed model considers nine spare parts, twelve periods, and two manufacturing methods. The solver platform took 0.03 seconds to solve the MILP problem with 24 iterations, 1026 variables, and 1476 constraints. The global optimal solution was found to be USD. It is to note that the lead times and demand values of all spare parts are known in advance, therefore, the orders are assumed to be made at the scheduled time.
4.2.1. Model performance by period
illustrates the total periodic cost breakdown per cost component. It is observed that the average cost fluctuates around 2,000,000 USD in each period. The second period stands out with the highest cost among all periods. This is mainly due to the significant cost associated with the purchase of AM raw material powder and the production of AM-based spare parts. It is worth noting that the second period also had the highest cumulative demand for all nine spare parts compared to other periods, which resulted in an increased production of AM-based spare parts to meet the demand. This is reflected in the higher cost of AM raw material purchase.
Figure 4. Cost breakdown for the total cost per period excluding penalty costs of CNC and AM lead times.

According to , the most significant cost across all periods is the purchase price of CNC-based spare parts, which can be explained by the fact that most of the spare parts are sourced through a CNC supplier. Meanwhile, AM is only used when the demand cannot be met with CNC-based spare parts. The second most dominant cost is the depreciation cost of the AM machine, which amounts to about 20% of the capital investment in the first year. The cost of purchasing AM raw materials, the transportation cost of CNC-based spare parts, and the production cost of AM-based parts are considered significant compared to other costs, which are relatively low. shows the quantity, inventory, demand, and back-ordered demand of each spare part taking the example of period 6.
shows that spare part 1 is primarily sourced from the CNC supplier; however, one demand unit is back-ordered, and one unit is produced with AM. The supplier capacity for sourcing spare part 1 is 40 units, and the demand for spare part 1 in period 6 is 42 units, which is why only one spare part is produced via AM, and the demand for the second part was back-ordered. Spare parts 6 and 9 have lower production costs with AM compared to their purchase price with CNC, and their demand are 36 and 39 units, respectively. Printing these parts with AM results in a shorter lead time rather than sourcing them with CNC, which explains their production via AM. Spare part 1 is mainly sourced with CNC because receiving it from the supplier takes a shorter lead time compared to printing the demanded quantity with AM. However, for spare parts of types 2, 3, 4, 5, 7, and 8, although sourcing them with CNC takes longer than AM, the associated cost of purchasing raw material and printing AM-based spare parts is extremely high so it is more cost-effective to order these as CNC based parts.
4.2.2. Model performance by spare parts
This section will present and discuss the outcomes of the decision variables for each spare part type throughout the 12 periods. shows the quantity, inventory, and demand for the nine types of spare parts in all periods. It is observed that CNC-based manufacturing is the most used method to source the spare parts and to satisfy the demand for seven types of spares, while AM is mainly used for spare parts 6 and 9 only. However, the model shows that AM-based spare parts are to be produced in cases where the demand could not be fulfilled through CNC due to the supplier capacity limit.
Factors such as part size, geometry complexity, lead time, and costs with different manufacturing methods all play a role in the decision-making process for each spare part. Due to variations in supplier capacity from one spare part type to another, and in some cases where demand for the spare part exceeds the supplier capacity, the model yields extra spare part units to store and use in the next period (such as spare parts 4 and 5).
Overall, AM is more feasible for producing spare parts with high geometry complexity and small to medium spare part sizes, while CNC-based manufacturing is cost-effective for large-sized spare parts with low to medium geometry complexity. The decision-making process for choosing the optimal manufacturing technology does not depend solely on cost, but also on other factors such as part size, geometry complexity, supply capacity, and lead time.
4.3. Model performance evaluation
To evaluate the performance of the model, a similar model formulation was implemented using the same dataset as the base case model for two case scenarios: satisfying all demand using only one manufacturing method, AM only, and CNC only.
shows the number of variables, constraints, iterations, and solving time of the MILP for each scenario. The results show that the CNC-only scenario has the lowest cost, as CNC-based spare parts are cheaper than AM-based ones and have moderately long lead times. On the other hand, the AM-only scenario has the highest total cost, mainly due to the high costs associated with raw material purchasing and the production cost of AM-based spare parts.
Table 5. Characteristics of different case scenarios.
) show the cost breakdown for the three case scenarios including and excluding the lead time penalty, respectively. In the CNC-based scenario, a penalty cost is incurred when demand cannot be satisfied with the available supplier capacity, resulting in back-ordered demand. In contrast, the AM-based scenario is associated with significant costs related to the purchase of AM raw materials and the production of spare parts using AM technology. Additionally, there are costs associated with the depreciation of AM machines.
Figure 7. Total cost comparison a) including costs of lead time, and b) excluding the cost of lead time.

However, in the proposed optimization case, the high-cost items that are present in both the CNC and AM scenarios are minimized and compromised. This suggests that the proposed model can find a balance between the long lead time and less expensive CNC method and the shorter lead time and more expensive AM technology. By finding this trade-off, the proposed optimization case can help to reduce overall costs while still meeting customer demand.
4.4. Scenario analysis
Scenario analysis is conducted on a set of model parameters to evaluate the performance and robustness of the proposed model. These parameters include the demand for spare parts, the lead time for CNC-based spare parts delivery from the supplier, and the lead time for AM raw material delivery. These parameters are varied within a specified range from their nominal value.
The scenario analysis aims to illustrate how changes in these parameters affect the model’s decision variables and how the quantities of AM and CNC spare parts react to these variations.
4.4.1. Demand
Scenario analysis is performed for the demand parameter where it is changed by ±10% while keeping the rest of the parameters at their original values. In a factory working environment, demand usually fluctuates within the proposed range of ±10%.
4.4.1.1. Decision variable analysis
shows the quantities of AM and CNC spare parts used to meet the demand for spare part 1 when the demand is varied by ±10%. As previously observed, when the demand is at its nominal value, the model uses AM-printed spare parts to meet the demand that cannot be satisfied by CNC-based spare parts. When the demand for spare part 1 is decreased, the model recommends sourcing all spare parts from the CNC supplier. However, when the demand is increased, the model produces more AM-based spare parts in addition to the ordered CNC-based spare parts to meet the demand. The maximum supplier capacity for spare part 1 is 40 units, so the model orders 40 units from the CNC supplier and produces the remaining spare parts with AM. Therefore, spare part 1 is sensitive to changes in demand.
On the other hand, the behavior of spare part 6 remains unchanged in all cases, whether the demand is increased or decreased. All the demand for spare part 6 is met through AM over the 12 periods. Thus, spare part 6 is insensitive to any changes in demand. Spare part 9 exhibits similar behavior to spare part 6, while spare parts 2, 3, 4, 5, 7, and 8 have similar behavior to spare part 1.
4.4.1.2. Cost analysis
illustrates the impact of changes in demand on the total cost of the base case, CNC, and AM scenarios. A decrease in demand results in a 3% decrease in the total cost, while an increase in demand leads to a 10% increase in the total cost. This increase can be attributed to the higher production cost of AM-based spare parts and the expensive purchase cost of raw materials, as the demand for these parts increases and the system relies more on AM technology to meet the demand.
Table 6. Change scenarios subject to demand changes.
When the demand decreases by 10%, the total cost of the AM-based scenario decreases by 9%, while in the proposed optimization scenario, the total cost only decreases by 3%. This indicates that AM is more cost-effective for low-demand volumes. The change in the total cost in the AM scenario is directly proportional to the demand change. In the CNC scenario, the decrease in demand is directly proportional to the change in cost, but when demand increases, the cost of the system increases exponentially. This drastic increase is due to the higher penalty for back-ordered demand, as the system has already ordered the maximum limit of spare parts from the CNC supplier. When demand exceeds this limit, the system has to back-order the demand that it cannot satisfy, which incurs additional costs.
illustrates the change in total costs for the base case model, CNC, and AM scenarios subject to demand change, with the grey area representing the total system cost. Although a demand variation range of ±10% was assumed in this study, a wider range of demand change (−60% to +60%) was assumed to highlight the difference between CNC and AM costs. Similar demand change ranges of 50% to 150% have been presented in the literature (Emelogu et al. Citation2016). As shown in , when demand increases, the costs of CNC spare parts increase until they become constant, while the costs of AM-related spare parts continue to increase with the increase in demand. This is because when demand is high, the system sources the maximum capacity of CNC-based spare parts and relies on AM to satisfy the demand. The break-even point, where the costs of AM and CNC are equivalent, is when the demand change is approximately 1.4 (an increase of +40%). At this point, the costs of CNC reach a steady state, while the costs of AM continue to increase.
Figure 9. CNC costs and AM costs subject to demand change of the base case scenario including all parts.

shows the behavior of the three scenarios as demand changes. The CNC-based supply increases drastically when demand rises because the CNC supplier’s spare parts capacity is reached, causing the system to start back-ordering unsatisfied demand. In contrast, for the proposed optimization, the total cost did not change significantly when demand increased. However, even when demand is high, the total cost of the proposed optimization is still lower than that of the AM-based scenario.
4.4.2. Lead time
Scenario analysis was conducted on the lead time parameter for a range of −20% to +20% based on a factory working environment. presents the changes in the total costs of the CNC-based scenario and the base case scenario. The change in the total cost of the base scenario and CNC-based scenario are very similar. This is because the penalty cost of lead time increases while the other cost components remain unchanged. This is expected since no change has occurred in the outcome of the decision variables of spare parts. Thus, the increase in total costs is reflected only in the cost of the lead time of the CNC supplier. We can conclude that the decision variables in terms of AM and CNC quantities are insensitive to any change in the CNC lead time for a range of ±20%.
Table 7. Change scenarios subject to CNC lead time change.
The behavior of spare part 1 remains unchanged when the lead time for AM raw material delivery changes, which is expected because spare part 1 is primarily sourced from the CNC supplier and only produced with AM when demand cannot be met through CNC. Similarly, the behavior of spare part 6 remains unchanged when the lead time is altered, indicating that both spare parts 1 and 6 are insensitive to changes in AM raw material lead time within the [−20%, +20%] range.
shows that the change in total cost behavior of the base case scenario and AM scenario is similar. All cost items remain constant except for the lead time cost, which increases in both cases. The increase in AM costs is due to the rise in the cost of AM raw material lead time. Therefore, the model is insensitive to any change in AM raw material lead time within the range of ±20%.
Table 8. Change scenarios subject to AM lead time change.
4.5. Discussion
The analysis of the given data with the proposed optimization model shows some important keypoints for AM and CNC-based spare parts supply. The different spare parts have different demand fluctuations, lead time changes, and characteristics. Overall, the model outcome shows that most of the spare parts are to be sourced via the CNC supplier and AM technology is to be utilized as an integrative method that complements the CNC method. However, certain spare parts, such as types 6 and 9, are better produced on-site through AM throughout the planning horizon. This is due to the high complexity of their geometry design, which makes the additive production of these parts less expensive than sourcing them from the CNC supplier. Additionally, the lead time to print spare parts 6 and 9 is shorter than that required to source them from the CNC supplier.
The total system cost fluctuates slightly, staying below and above 2,000,000 USD in every period. The highest accumulated demand for all spare parts is in the second period, which is why the cost in period 2 is the largest. As previously mentioned, most spare parts are sourced via a CNC supplier throughout the planning horizon, hence the largest periodic cost component is the purchase cost of CNC-based spare parts, followed by the depreciation cost of the AM machine, and the purchase cost of AM raw material.
The proposed optimization was compared with CNC-based and AM-based scenarios, where the highest cost was reported for the AM scenario and the lowest cost was reported for the CNC option. This is because, in the AM option, the lead times are relatively short, but the costs associated with AM raw material and spare parts production are high. However, for the CNC scenario, lead times tend to be longer, but the costs to purchase the CNC-based spare parts and the penalty for back-ordered demand are high. In the proposed optimization, the highest cost components of CNC and AM scenarios are compromised by solving the trade-off between the lead time and cost.
presents the accumulated total cost of each spare part over the planning horizon, with spare part 3 having the highest cost. This is because it provides the highest purchase price from CNC supplier and the highest production cost through AM.
As mentioned in Section 3 (), spare part 3 is large-sized and has a higher geometry complexity, which explains the higher cost associated with it. AM has shown to be feasible in producing complex stainless parts in less time and cost compared to CNC machining. As the geometry complexity of a part increases, its manufacturing cost with CNC increases. However, the cost to produce it with AM may not change because the printing cost and energy of a complex part do not depend on its shape complexity (Quinlan et al. Citation2017).
Although it may be more cost-effective to print a complex geometry spare part rather than producing it with CNC, the proposed model recommends sourcing spare part type 3 from CNC. This is because spare part 3 is large, and all large-sized spare parts (types 1, 2, and 3) are recommended to be sourced through CNC. This finding is aligned with the literature where Zhang et al. (Citation2019) stated that the size of a spare part impacts the AM operation, and in some cases, AM may not be able to compete financially with CM methods. Manufacturing large spare parts with CNC is more economical than using AM since printing them with AM requires more material, printing time, and energy, which increases the costs.
The outcome of the model shows that, for cost-effectiveness, spare parts with high geometric complexity are to be manufactured using AM, while spare parts with large sizes should be manufactured using CNC. However, it should be noted that this is not always the case, as other factors, such as lead time, spare part characteristics, and supply potential, are involved in the manufacturing process decision-making. Although the AM method offers shorter lead times and enables design customization, it can also be costly due to the high purchase price of AM raw materials, printing energy costs, and AM machine depreciation costs.
Based on the scenario analysis performed, it can be observed that the total cost is always sensitive to changes in demand and lead time parameters. When the demand changes from −60% to 60%, a shift in the mix of AM and CNC is observed, highlighting that AM can complement the CNC method and be used for urgent or offshore spare parts applications.
The results of the scenario analysis demonstrate that the mix of AM and CNC manufacturing methods is sensitive to changes in demand and lead time parameters, with the former having a more significant impact. For most spare parts, changes in demand lead to changes in the mix of AM and CNC, indicating that AM can complement the CNC method for urgent or offshore spare parts applications. However, spare parts 6 and 9 are found to be insensitive to demand changes. In contrast, all spare parts are found to be insensitive to changes in lead time for both methods. presents the adopted parameters for scenario analysis, along with the ranges of changes and their impact on the total cost of the base case scenario. lists the sensitivity of the decision variables for each spare part type to changes in each parameter. Notably, all spare part types show insensitivity to changes in lead time, but sensitivity to changes in demand, except for spare parts 6 and 9.
Table 9. Effect of parameter variation on the total cost.
Table 10. Effect of parameter variation on decision variable outcome by spare parts type.
4.5.1. Insights and managerial implications of the model
The optimization model analysis was used to assess a few of the questions as mentioned below.
How much to order from CNC spare parts supplier?
How much to order raw materials from AM supplier?
When to produce on-demand AM spare parts?
How much to store and for how long?
The answers to the above questions are addressed in the outcome of the decision variables of the optimization model. The quantity of CNC-based spare parts to be ordered answers the question of ‘how much to order from the CNC spare parts supplier’. The raw material volume to be ordered and used for producing AM-based spare parts answers the question of ‘how much to order raw material from the AM supplier’. The outcome of the inventory units stored in the warehouse in each period addresses the question of ‘how much to store and for how long’. The MILP optimization model guides the decision of the optimal quantities and when to implement them to minimize total costs.
The model offers an economic inventory production schedule that considers the trade-off between CNC manufacturing and AM for decision-makers. In real-life decision-making, this model implementation offers insights for decision-makers, providing them with the opportunity to make the right decisions and negotiate with supply chain partners based on information that cannot be previously quantified. The proposed model is generic and can be implemented in any business for spare parts that can be manufactured by AM and CNC machining. The model allows dual sourcing in the manufacturing method for each spare part and considers the lead time factor, which impacts the downtime cost, especially in heavy industries such as oil and gas where the cost of downtime is very high. Another distinctive feature of the model is the consideration of multiple spare parts with different characteristics, such as spare part size, geometry complexity, and lead time, which makes the model a reliable decision-making tool. The decision-making process on the manufacturing method of each spare part varies according to the spare part’s characteristics, such as geometry complexity, size, volume, production cost, and lead time.
The scenario analysis of various model parameters allows the decision-maker to explore and investigate which influential parameters significantly affect the system and how they affect it. These types of scenarios provide insight to stakeholders involved in planning, to evaluate which parameters to roughly estimate and which to precisely estimate. Adequate and proper planning of logistics, optimal and economic order quantities, inventory control, and resource allocation contribute to increased process efficiency, lower costs, and improved supplier experience and customer satisfaction.
5. Conclusion and future work
In this paper, an integrated framework is proposed by highlighting the inputs, processes, constraints, and outputs of AM technology in the spare parts supply chain. The trade-off between CNC-based spare parts and AM-based spare parts is analyzed through a multi-period, multiple spare parts MILP that optimizes the quantity of CNC-based spare parts to be ordered and AM-based spare parts to be produced at the minimal total cost. The formulated problem includes nine types of spare parts with varying geometry complexities, sizes, lead times, and costs for each manufacturing alternative. This generic problem can be applied to any spare parts system that contains parts manufacturable with AM and CNC methods.
This study highlights the effects of lead time, spare part geometry complexity, and size factors on the economic feasibility of CNC-based and AM-based spare parts supply chains. Results have shown that AM is economically feasible for small and medium-sized spare parts with complex geometries. The advantage of AM technology lies in its ability to produce complex geometry spare parts that may not be possible to produce through CM methods or that take long production times (Emelogu et al. Citation2016). The fabrication time of AM-based spare parts is insensitive to the complexity of the part’s geometry. Spare parts that are larger and have low to medium geometry complexity are CNC-based, and AM is used as a complementary method to satisfy demand. This result supports the literature finding of Thomas and Gilbert (Citation2014), who mentioned that two manufacturing technologies can be complementary and adopted alongside to gain greater benefits rather than adopting each technology separately.
The continuous increase of AM adoption may lead to a reduction in AM raw material costs through economies of scale, resulting in more integration of AM into the supply chains of systems and organizations. The cost of metal-based AM machines is likely to reduce significantly in the future, making it cheaper to produce AM parts. Additionally, AM technology may offer many advantages over CM methods, including lightweight parts, extended useful life, and more sustainable and recyclable products.
5.1. Limitations
Limitations of this work are the following:
The proposed model focuses only on the considered spare part types with the three proposed levels of geometry and sizes. The model did not consider other spare part types with different characteristics and geometries.
The data used for model implementation is based on the data given in the literature. The availability of real data and practical examples from real industry cases would reflect the entire situation in a more holistic manner.
5.2. Future work
The study has considered a deterministic model that captured rational values such as spare parts demand change, lead times, supply situation, and cost changes. However, in real-case scenarios, these parameters are not always deterministic, and they fluctuate from one period to another. Therefore, to capture the uncertainty of these parameters, extending the model to a stochastic-based optimization model would better represent real-life decision-making and enhance the model’s performance.
The proposed cost optimization model is a single-objective problem. Extending this work to multi-objective optimization problem with an environmental objective could be used to investigate emissions and carbon cap conditions, which may highlight the advantages of AM technology.
Another future direction of this analysis could involve further supply chain entities, such as the consideration of multiple suppliers, multiple facilities and warehouses, and multiple AM hubs, which would enable the analysis of different supply chain configurations to comprehensively examine the trade-off between AM and CNC manufacturing methods.
Author contribution
Conceptualization – Shaligram Pokharel, Faris Tarlochan, and Fujio Tsumori; Methodology – Asma Mecheter, Shaligram Pokharel, and Faris Tarlochan; Writing- original draft preparation – Asma Mecheter; Writing- review and editing – all authors; Formal analysis – Asma Mecheter; Validation – Asma Mecheter; Data curation – Asma Mecheter; Investigation – Asma Mecheter; Supervision, Shaligram Pokharel, Faris Tarlochan, Fujio Tsumori; Funding acquisition – Faris Tarlochan, Shaligram Pokharel, Fujio Tsumori. All authors have read and agreed to the published version of the manuscript.
Acknowledgements
This study was made possible by the Qatar University grant# M‐QJRC‐2020‐6. The findings of this study are solely the responsibility of the authors. Open Access funding provided by the Qatar National Library.
Disclosure statement
No potential conflict of interest was reported by the author(s).
Additional information
Funding
References
- Ashour Pour, M., S. Zanoni, A. Bacchetti, M. Zanardini, and M. Perona. 2019. “Additive Manufacturing Impacts on a Two-Level Supply Chain.” International Journal of Systems Science: Operations & Logistics 6 (1): 1–14. https://doi.org/10.1080/23302674.2017.1340985.
- Atzeni, E., and A. Salmi. 2012. “Economics of Additive Manufacturing for End-Usable Metal Parts.” The International Journal of Advanced Manufacturing Technology 62 (9–12): 1147–1155. https://doi.org/10.1007/s00170-011-3878-1.
- Baumers, M., C. John Tuck, R. Wildman, I. Ashcroft, E. Rosamond, and R. J. M. Hague. 2012. “Combined Build-Time, Energy Consumption and Cost Estimation for Direct Metal Laser Sintering.“ 2012 International Solid Freeform Fabrication Symposium, University of Texas at Austin.
- Boer, J. D., W. Lambrechts, and H. Krikke. 2020. “Additive Manufacturing in Military and Humanitarian Missions: Advantages and Challenges in the Spare Parts Supply Chain.” Journal of Cleaner Production 257:257. https://doi.org/10.1016/j.jclepro.2020.120301.
- Cantini, A., M. Peron, F. De Carlo, and F. Sgarbossa. 2022. “A Decision Support System for Configuring Spare Parts Supply Chains Considering Different Manufacturing Technologies.” International Journal of Production Research 1–21. https://doi.org/10.1080/00207543.2022.2041757/FORMAT/EPUB.
- Cardeal, G., D. Sequeira, J. Mendonça, M. Leite, and I. Ribeiro. 2021. “Additive Manufacturing in the Process Industry: A Process-Based Cost Model to Study Life Cycle Cost and the Viability of Additive Manufacturing Spare Parts.” Procedia CIRP 98:211–216. https://doi.org/10.1016/j.procir.2021.01.032.
- Cestana, A., E. Pastore, A. Alfieri, and A. Matta. 2019. “Reducing Resupply Time with Additive Manufacturing in Spare Part Supply Chain.” IFAC-Papersonline 52 (13): 577–582. https://doi.org/10.1016/j.ifacol.2019.11.220.
- Chaudhuri, A., H. A. Gerlich, J. Jayaram, A. Ghadge, J. Shack, B. H. Brix, L. H. Hoffbeck, and N. Ulriksen. 2020. “Selecting Spare Parts Suitable for Additive Manufacturing: A Design Science Approach.” Production Planning and Control 32 (8): 670–687. https://doi.org/10.1080/09537287.2020.1751890.
- Deppe, G., and R. Koch. 2014. “Exploring the Influence of an Additive Manufacturing Integration on Future MRO Processes in Aeronautics.” RTejournal-Forum Für Rapid Technologie 2014.
- Emelogu, A. A. 2016. “Optimal Supply Chain Configuration for the Additive Manufacturing of Biomedical Implants.” ProQuest diss. and Theses. https://search.proquest.com/docview/1858815032?accountid=188395.
- Emelogu, A., M. Marufuzzaman, S. M. Thompson, N. Shamsaei, and L. Bian. 2016. “Additive Manufacturing of Biomedical Implants: A Feasibility Assessment via Supply-Chain Cost Analysis.” Additive Manufacturing 11 (July): 97–113. https://doi.org/10.1016/J.ADDMA.2016.04.006.
- “ILOG CPLEX Optimization Studio | IBM.” 2022. https://www.ibm.com/products/ilog-cplex-optimization-studio.
- Jin, Y., Y. He, and J. Du. 2017. “A Novel Path Planning Methodology for Extrusion-Based Additive Manufacturing of Thin-Walled Parts.” International Journal of Computer Integrated Manufacturing 30 (12): 1301–1315. https://doi.org/10.1080/0951192X.2017.1307526.
- Kerbrat, O., P. Mognol, and J. Y. Hascoët. 2011. “A New DFM Approach to Combine Machining and Additive Manufacturing.” Computers in Industry 62 (7): 684–692. https://doi.org/10.1016/J.COMPIND.2011.04.003.
- Knofius, N., M. C. van der Heijden, A. Sleptchenko, and W. H. M. Zijm. 2020. Improving Effectiveness of Spare Parts Supply by Additive Manufacturing as Dual Sourcing Option. OR Spectrum. Berlin Heidelberg: Springer. https://doi.org/10.1007/s00291-020-00608-7.
- Knofius, N., M. C. van der Heijden, and W. H. M. Zijm. 2019. “Moving to Additive Manufacturing for Spare Parts Supply.” Computers in Industry 113:103134. https://doi.org/10.1016/j.compind.2019.103134.
- Kunovjanek, M., N. Knofius, and G. Reiner. 2020. “Additive Manufacturing and Supply Chains–A Systematic Review.” Production Planning and Control. 1–21. https://doi.org/10.1080/09537287.2020.1857874.
- Liu, P., S. H. Huang, A. Mokasdar, H. Zhou, and L. Hou. 2014. “The Impact of Additive Manufacturing in the Aircraft Spare Parts Supply Chain: Supply Chain Operation Reference (Scor) Model Based Analysis.” Production Planning & Control 25 (13–14): 1169–1181. https://doi.org/10.1080/09537287.2013.808835.
- Manogharan, G., R. A. Wysk, and O. L. A. Harrysson. 2016. “Additive Manufacturing–Integrated Hybrid Manufacturing and Subtractive Processes: Economic Model and Analysis.” International Journal of Computer Integrated Manufacturing 29 (5): 473–488. https://doi.org/10.1080/0951192X.2015.1067920.
- Marinho De Brito, F., G. Da Cruz Junior, E. M. Frazzon, J. P. T. V. Basto, and S. G. S. Alcala. 2021. “Design Approach for Additive Manufacturing in Spare Part Supply Chains.” IEEE Transactions on Industrial Informatics 17 (2): 757–765. https://doi.org/10.1109/TII.2020.3029541.
- Mecheter, A. 2023. “Supply Chain Modeling of Additively Manufactured versus CNC-Produced Spare Parts.” Master’s thesis, Qatar University.
- Mecheter, A., S. Pokharel, and F. Tarlochan. 2022. “Additive Manufacturing Technology for Spare Parts Application: A Systematic Review on Supply Chain Management.” Applied Sciences (Switzerland) 12 (9): 4160. https://doi.org/10.3390/app12094160.
- Pokharel, S. 2023. “Introducing BLOC-ICE for Exploring System Concept.” International Journal of Business and Systems Research 17 (4): 442–461. https://doi.org/10.1504/ijbsr.2022.10039739.
- Portolés, L., O. Jordá, L. Jordá, A. Uriondo, M. Esperon-Miguez, and S. Perinpanayagam. 2016. “A Qualification Procedure to Manufacture and Repair Aerospace Parts with Electron Beam Melting.” Journal of Manufacturing Systems 41:65–75. https://doi.org/10.1016/j.jmsy.2016.07.002.
- Quinlan, H. E., T. Hasan, J. Jaddou, and A. John Hart. 2017. “Industrial and Consumer Uses of Additive Manufacturing a Discussion of Capabilities, Trajectories, and Challenges.” Journal of Industrial Ecology 21 (S1): S15–S20. https://doi.org/10.1111/jiec.12609.
- Sgarbossa, F., M. Peron, F. Lolli, and E. Balugani. 2021. “Conventional or Additive Manufacturing for Spare Parts Management: An Extensive Comparison for Poisson Demand.” International Journal of Production Economics 233 (November 2020): 107993. https://doi.org/10.1016/j.ijpe.2020.107993.
- Shehadeh, A.-S.-S. 2019. “Evaluation of 316L Stainless Steel Part Fabrication Using Additive and Subtractive Manufacturing: A Guideline for Process Selection.” Master’s thesis, Qatar University.
- Thomas, D. S., and S. W. Gilbert. 2014. “Costs and Cost Effectiveness of Additive Manufacturing: A Literature Review and Discussion.” NIST Special Publication 1176 (12): 1–96.
- Vendra, L., and A. Achanta. 2020. “Metal Additive Manufacturing in the Oil and Gas Industry.” Solid Freeform Fabrication 2018: Proceedings of the 29th Annual International Solid Freeform Fabrication Symposium - An Additive Manufacturing Conference, SFF 2018, University of Texas at Austin: Austin, TX, USA, 454–460.
- Westerweel, B., R. Basten, J. den Boer, and G.-J. van Houtum. 2020. “Printing Spare Parts at Remote Locations: Fulfilling the Promise of Additive Manufacturing.” Production and Operations Management 30 (6): 1615–1632. https://doi.org/10.1111/poms.13298.
- Wohlers, T. 2017. “3D Printing and Additive Manufacturing State of the Industry, Annual Worldwide Progress Report Wohlers Report: Chapters Titles: The Middle East, and Other Countries, Wohlers Associates.” 344. Fort Collins, CO.
- Yilmaz, Ö. F. 2020. “Examining Additive Manufacturing in Supply Chain Context Through an Optimization Model.” Computers & Industrial Engineering 142 (November 2018): 106335. https://doi.org/10.1016/j.cie.2020.106335.
- Zhang, Y., S. Jedeck, L. Yang, and L. Bai. 2019. “Modeling and Analysis of the On-Demand Spare Parts Supply Using Additive Manufacturing.” Rapid Prototyping Journal 25 (3): 473–487. https://doi.org/10.1108/RPJ-01-2018-0027.