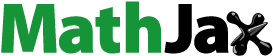
ABSTRACT
Studies of the determinants of the spread and mortality of COVID-19 indicate that the quality of health care systems and government type and capacity have a negligent role in explaining the variation of infection and death rates between countries. Research therefore suggests a role for societal factors, in particular social capital. But its measures vary widely, sometimes including indicators that refer to politics or governance. We clearly distinguish social cohesion from social capital and argue that the first better captures the societal influence on the pandemic, by its concern with the common good and relationships between (rather than within) social groups. We analyze the role of social cohesion in the spread and mortality of COVID-19 in a large cross-country analysis with a comprehensive index and two sub-indices of social cohesion. Moreover, the underlying indicators enable us to study the pathways through which social cohesion is likely to affect COVID-19 outcomes. Contrary to the recent empirical literature we find robust relationships, in particular for intergroup cohesion. Our findings suggest that more cohesive societies, in particular those with less divisiveness between social groups, may be better equipped to reduce the impact of a pandemic.
Introduction
The variation in the spread and fatality of COVID-19 outcomes between countries is related to population factors such as Body Mass Index (BMI) and age (Mallapaty, Citation2020; Popkin et al., Citation2020). However, recent studies show that the relationship with health care variables, such as the number of hospital beds, universal health coverage, or the Global Health Security Index are not meaningfully related to the impact of the virus (COVID-19 National Preparedness Collaborators, Citation2022; Deaton, Citation2021). Moreover, the virus seems to affect populations in middle- and high-income countries much more than those in low-income countries (Mukherjee, Citation2021). A detailed epidemiological cross-country study has indicated that the level of trust between individuals as well as in government institutions has a strong negative effect on COVID-19 infection rates (COVID-19 National Preparedness Collaborators, Citation2022). Various studies, often at the local level, have included measures of social capital. Many studies, but certainly not all, report negative effects of social capital on COVID-19 infection rates and/or death rates. But the measures used vary widely and are often not clearly related to theory while a justification of using social capital variables instead of social cohesion variables is lacking.
Many studies use a single indicator for social capital. Others use indicators that are not part of social capital at all, such as political participation (through voter turnout) or government institutions. This wide variation in measures and inconsistent use of the term social capital may be a reason for the mixed effects found in empirical results. This is not surprising, because earlier studies have also shown mixed effects of social capital on health outcomes (Bruhn, Citation2009; Kawachi & Berkman, Citation2014). Inconsistent findings may at least partially be due to the instrumental character of the concept of social capital. We think that the sociological concept of social cohesion is a more appropriate candidate for analyzing the impact of social relations on COVID-19 outcomes, because it refers to connectedness and belonging as well as to mutual respect between social groups, which involves interpersonal trust, prosocial norms, tolerance and willingness to cooperate with other groups (Durkheim, Citation1997; Manca, Citation2014).
In our cross-country analysis, we use a comprehensive multidimensional Social Cohesion Index. We have further detailed our analysis by distinguishing between the two dimensions of social cohesion that are generally recognized in the sociological literature (see, e.g. Manca, Citation2014): the lack of tolerance and conflict in the relationships between groups or factions in society, and the extent of community and engagement between individuals, including between strangers. The findings of our multivariate regression analysis with relevant confounding variables show that social cohesion is negatively correlated with COVID-19 infection rates and mortality rates, a result which supports recent findings of the collaborative SolPan project studying solidarity during COVID-19 (Hangel et al., Citation2022; Spahl et al., Citation2022; Zimmermann et al., Citation2022). The results for the Intergroup Cohesion Index are particularly strong, suggesting that polarization of societies, through divisiveness between social groups, allows a pandemic to be more devastating.
Social capital, social cohesion and COVID-19
Social capital, social cohesion, and its measures in studies on COVID-19
The empirical literature on societal determinants of COVID-19 refers more often to social capital than to social cohesion. Shachat et al. (Citation2021) find that a cooperative attitude supports the adherence to policy measures in China, while Müller and Rau (Citation2021) show that social responsibility attitudes support compliance with policy measures in Germany. Zhu et al. (Citation2021) find that in China, Japan and the US, pro-social norms are positively correlated to compliance with COVID-19 measures. Brodeur et al. (Citation2021) report that in high-trust counties in the United States (US) there is more compliance with the policy measures than in low-trust counties. Barrios et al. (Citation2021) report a positive relationship between ‘civic capital’ (trust in government institutions and in others) and social distancing and mask wearing, in a sample of US counties and several European countries.
Other studies find mixed results or no effect at all of social capital. For example, Romano et al. (Citation2021) find no effect of trust and cooperation in a survey including 34,000 individuals in 41 countries on the support for policy measures. Borgonovi et al. (Citation2021) report a negative correlation between social capital and the Covid death rate in US counties and mixed effects of political trust. Several studies report nonlinear effects, which are not explained by the social capital measures used. Shachat et al. (Citation2021) show in an experimental study in China that cooperative behavior was high in the first stages of the pandemic but reduced over time. Bartscher et al. (Citation2021) found that at the beginning of the pandemic, social capital measured as voter turnout in elections in seven European countries correlated with more infections, whereas later on the correlation turned negative. Min and Capraro (Citation2020) finds a U-shaped relationship in a study of 68 countries between trust radius and time till the virus infections peaked. In a study across 84 countries, Elgar et al. (Citation2020) report that civic engagement is related to lower mortality rates of COVID-19, whereas group affiliation and social trust are related to higher mortality rates. The earlier mentioned study on US counties using voter turnout as a measure of social capital reports a clear nonlinear effect over a year (Borgonovi et al., Citation2021). A study on 106 Italian provinces by Alfano and Ercolano (Citation2021) finds no effect of social capital (measured as the share of nonprofit organizations per province).
The empirical literature using COVID-19 spread- and mortality rates as the dependent variable is more recent and therefore more limited than the literature on behavioral outcomes. In general, this literature tends to find negative correlations with social capital and social cohesion measures, but again, not always. Gelfand et al. (Citation2021) use an index of loose versus tight cultures and find in a study of 57 countries that loose cultures have five times more infections and almost nine times more deaths than tight cultures, but their cultural index includes variables for government control. Kumar (Citation2021) finds a negative correlation between community orientation and COVID-19 outcomes in 62 countries. A recent large cross-country study finds that both trust between people and trust in government institutions are negatively related to COVID-19 infection rates but not to death rates (COVID-19 National Preparedness Collaborators, Citation2022).
What emerges from our review above, apart from the mixed findings, is that a wide variety of measures is used, of which it is not always clear if and how they measure social capital or social cohesion. The next sub-section will critically discuss the distinction between the two as the basis for our own analysis.
From social capital to social cohesion
Just like social capital, social cohesion has been analyzed in relation to health. John Bruhn (Citation2009) observes that “there is considerable evidence that suggests that community characteristics and community processes affect both health behaviors and health outcomes” (p.10). But how is social cohesion distinguished from social capital in health research? And does it matter?
The concept of social cohesion is much older than that of social capital, and dates back to Émile E. Durkheim (Citation1951, Citation1997) who considered it as the social quality of communities that provides belonging and mutual support. Many decades later, Mark Granovetter (Citation1973) has presented the idea of the strength of weak ties. Another decade later, sociologists have focused on the instrumental aspect of community ties, using the term social capital, for example for acquiring human capital (Coleman, Citation1988). This, in turn, has sparked the interest of economists, further narrowing down social capital to a resource in which one’s investments would generate pay-offs (see, for example, Dasgupta & Serageldin, Citation1999). But other economists have criticized the instrumental use of an inherently social concept (Christoforou & Davis, Citation2014; Van Staveren et al., Citation2008). Today, the two concepts are used next to each other but often with overlapping understandings. This has led to the current conceptual confusion and inconsistencies in measurement, which has also affected public health research, as has been argued by Carrasco and Bilal (Citation2016).
Some health researchers use the concept of social cohesion deliberately in contrast to social capital, in order to emphasize its societal-level character, meso-level dynamics or orientation towards the common good (Bruhn, Citation2009; Kawachi & Berkman, Citation2014). Measures of social cohesion are concerned with the extent, strength, duration, and underlying values of social relations (Christoforou & Davis, Citation2014). In elaborating the distinction, Carrasco and Bilal (Citation2016) have referred to Erich Fromm’s use of the notions of having and being. They link the first to social capital and the second to social cohesion. The authors advise that health researchers “should be aware of the difference between social capital and social cohesion when designing public health interventions, to ensure posing appropriate research questions and using the most conducive frameworks and approaches” (Carrasco & Bilal, Citation2016, p. 130). This is precisely what we have tried to do in our research.
In , we clarify the distinction between the two concepts with the help of two axes. The horizontal axis is concerned with social groups. To the left, social relations are inward-oriented (for example in religious or political groups), which sometimes is referred to as bonding social capital. Such in-group orientation may result, however, in us-them attitudes towards other groups. To the right, relations between social groups are characterized by tolerance and respect, rather than by divisiveness. The vertical axis refers to individual attitudes toward society. At the bottom, social relations are considered as connections for individual benefit, sometimes referred to as bridging social capital. At the top, social relations are considered as part of, as well as contributing to, the common good.
With these two axes, the figure boxes indicate that social capital is concerned with within-group orientation and individual benefit, whereas social cohesion refers to respectful between-group relations and a concern with the common good. But the distinction does certainly not imply a clear separation or opposition between social capital and social cohesion, which is reflected in the two hybrid boxes.
Our research analyzes whether countries with higher levels of social cohesion show lower COVID-19 infection and mortality rates. We expect a beneficial effect of social cohesion precisely because of the protective features of social cohesion, irrespective of government policies and institutions, the level of democracy, and the quality of the health care system. These protective features are inherent in the social-level of social cohesion, in contrast to the individual-level characteristics of social capital. Social cohesion is concerned with the common good, and fighting the pandemic requires people to show solidarity and concern with vulnerable groups, even if it involves personal costs such as mask wearing and self-isolation. This role of social cohesion is highlighted in studies emerging from the SolPan project on people’s values and attitudes of solidarity during the pandemic. For example, Zimmermann et al. (Citation2022) argue that “a sense of togetherness motivates people to comply with restrictive measures and can be fostered through campaigns and shared individual narratives that focus on cohesion rather than division” (p. 1342). Moreover, Hangel et al. (Citation2022) emphasize that government policy measures are insufficient. “In addition, social cohesion and individual willingness to act in solidarity towards a common goal are equally valid” (p.2).
Earlier research on social cohesion and health also provides evidence for the social-level effect of social cohesion, in contrast to social capital concerns with individual returns and in-group benefits (Bruhn, Citation2009; Kawachi & Berkman, Citation2014). For example, a study on the AIDS-epidemic by Lieberman (Citation2009) found that policies in countries with strong ethnic group boundaries tend to reinforce distrust and blaming between ethnic groups, a divisiveness which is not helpful in fighting the AIDS-epidemic.
Method and data
Method
We will test our hypothesis with a large sample of countries. We use multiple regression analysis (OLS) with several explanatory variables and confounders. Of course, it should be kept in mind that cross-section regression analysis does not establish causality and may suffer from endogeneity. However, reverse causality is not very likely in our study given the difference in the moment of measurement of the dependent variables and the key explanatory variables. The dependent variables refer to accumulated COVID-19 data until 1 November 2020, whereas the three social cohesion indices, use data from the years 2018, 2019 and, to a limited extent, 2020. Data for all other independent variables are from 2019.
The following equation describes the relationship between the COVID-19 outcomes and social cohesion.
Y refers to accumulated COVID-19 infections in the first series of models and accumulated COVID-19 deaths in the second series of models. SC is the key explanatory variable, social cohesion, for which we use three different composite measures: Social Cohesion Index, Intergroup Cohesion Index and Interpersonal Safety & Trust Index. They are used in three separate models. The other variables are confounders: Gross Domestic Product (GDP) per capita; government capacity (G) consisting of three separate variables: the quality of public services, governance performance and a corruption index; health care capacity (, which consists of the following four separate variables: government health expenditures as a percentage of GDP, the number of hospital beds per 1,000 inhabitants, universal health coverage, and a general health vulnerability indicator expressed by the percentage of the population over 65 years old. Finally, in order to account for differences in political systems across countries we control for the liberal democracy index (
.
Variables and data
The two dependent variables are measured as the accumulated number of cases and deaths per ten thousand inhabitants. The data are obtained from the COVID-19 Data Repository of the Center for Systems Science and Engineering (CSSE) at Johns Hopkins University. Due to the differences in the peak of the first wave of the pandemic we decided to use the total number of cases accumulated until November 2020, which is the last month before vaccination started worldwide. In a robustness test, we use the accumulated number of cases up to a full year later, November 2021.
There is a widely acknowledged measurement problem in the global COVID-19 infections and mortality data.Footnote1 Some studies therefore use excess mortality data. For example, an estimation using excess mortality data suggests that the actual number of COVID-19 deaths is three times higher than reported COVID-19 mortality (COVID-19 Excess Mortality Collaborators, Citation2022). Others have pointed at a possible overestimation when using excess mortality data. In a detailed estimation for England and Wales, Williams et al. (Citation2021) show that a lockdown had an unintended behavioral effect on other patients through evading hospital care. Their estimation suggests that only 63% of excess mortality can be attributed to COVID-19 death causes. We include a robustness test in the supplementary material (table A2) with excess mortality data from the WHO.Footnote2 The results are similar to our estimations with COVID-19 death registration data. In addition, we did pair-wise correlations between, on the one hand, the gap between excess deaths and COVID-19 deaths, and on the other hand social cohesion. The results in the supplementary material (table A3) show very small and negative correlations (<0.2) with each of the three social cohesion indices, indicating that it is not likely that countries with higher social cohesion also had systematically more (or less) underreporting of COVID-19 deaths.
The social cohesion data are from the Indices of Social Development database of the Institute of Social Studies of Erasmus University Rotterdam.Footnote3 The Intergroup Cohesion Index refers to relations of tolerance and respect between social groups in society. The index consists of 10 indicators, including societal polarization, confidence in law-and-order authorities, and the extent of grievances between groups. The Interpersonal Safety & Trust Index refers to interpersonal norms and behaviors of trust. The index has 22 indicators, including measures of contextualized trust, community norms, and crime rates. The Social Cohesion Index is constructed as the combination of all indicators.Footnote4 See supplementary material table A1 for the full list of indicators included in the three social cohesion indices. The 32 indicators come from reputable secondary data sources, such as the World Values Surveys, International Country Risk Guide and the United Nations. They include observable data (for example crime rates), attitudinal data (for example the percentage of respondents in a representative sample of a country who state that they generally trust other people) and expert opinions (for example the risk of internal conflict in a country assessed by various experts living in that country). The indices were constructed with the ranking method of matching percentiles (Foa & Tanner, Citation2010).Footnote5
As an economic variable, we include GDP per capita at constant 2010 prices from the World Bank. The data for health care expenditures as percentage of GDP, the number of hospital beds per 1000 inhabitants and the proportion of the population over 65 years old were all taken from the World Bank as well. The universal health coverage data are from the World Health Organization. The data on governance are from the Worldwide Governance Indicators (also World Bank).Footnote6 Finally, the data for the level of democracy are taken from the Varieties of Democracy database of the University of Gothenburg.Footnote7 This index consists of 69 observable indicators ranging from freedom of elections to freedom of religion.
shows the descriptive statistics. Our full sample consists of 219 countries: 184 developing countries and 35 developed countries. The models contain different numbers of countries (varying between 116 and 138) because we eliminated all cases with missing data on any variable. The countries that are dropped tend to be almost all small (island) states. Moreover, there is no bias against any region or income group. For example, when we look at the first estimation (n = 125), we see that there is a reasonable representation of low-income countries (18%) and medium-low income countries (35%) as well as African (37%) and Asian (31%) countries. Finally, we have used World Bank country classifications for regions for additional estimations presented in the supplementary material.Footnote8 All result tables report the estimated values, confidence intervals and p-values.
Table 1. Descriptive statistics.
Results
Results for COVID-19 infection rates
The results presented in indicate that there are 33 less cases per ten thousand inhabitants when countries have a one-point increase on the Social Cohesion Index (which equals a percentage point, since the scale is converted to 0–100, although the range is narrower). This means that, for example, if the UK had the same level of social cohesion as Estonia, it would have had 33 less cases per ten thousand inhabitants than it actually had by November 2020. This is a difference of 218,000 infections, given its population size of 68.2 million inhabitants. The table also points out that the regression result for the Social Cohesion Index seems to be driven largely by the Intergroup Cohesion Index.
Table 2. Social cohesion and COVID-19 infections.
The inclusion of confounding variables indicates that the relationship of social cohesion persists. The literature on social cohesion does not suggest multicollinearity between these confounders. The bi-variate regression results between social cohesion on the one hand and COVID-19 infections and deaths on the other hand shows that the effect of social cohesion is of the same size and level of significance as in the multivariate regressions.Footnote9 This indicates that social cohesion has a direct effect on COVID-19 outcomes, which is not, or at least not importantly, mediated through the other variables in the regression, such as GDP per capita for example.
We note that GDP per capita has a positive but small effect, which confirms that richer countries are more affected by the virus (see also, for example, Deaton, Citation2021). For government capacity, the quality of public services shows positive relationship, which may be a sign of higher levels of diagnosis of the virus in countries with better public services. We see a similarly positive relationship with public health care expenditures. Probably, countries that spend more on health care have a higher capacity to test and identify COVID-19 cases.
Results for COVID-19 death rates
The results presented in indicate that there were 1.8 less deaths per ten thousand inhabitants when countries score one point higher on the Social Cohesion Index. This means that, for example, if Brazil had the same level of social cohesion as Malaysia, it would have had 49,000 less deaths, given its population size of 213 million inhabitants. Again, the regression result for social cohesion is driven more by intergroup cohesion than by interpersonal safety & trust.
Table 3. Social cohesion and COVID-19 deaths.
When we look at the confounding variables, we note that GDP per capita has zero effect. For the level of democracy, there is a weak positive association which may signal that autocracies might systematically underreport COVID-19 mortality. The government capacity and health care variables do not seem to be much related. Finally, as expected, there is a positive correlation with the proportion of the population over 65 years old.
Pathways
Our results indicate that the level of inter-group relations is driving the correlations. This can be analyzed further: for the 10 indicators in the Intergroup Cohesion Index, we ran the same regressions as before (see table A6 in the supplementary material). Three out of the ten indicators show a clear negative relationship with the number of COVID-19 cases and/or deaths with confidence intervals indicating that the null hypothesis should be rejected: low grievances between groups, low societal polarization, and confidence in authorities being able to keep law and order. This suggests that divisiveness may be worse for the spread and mortality of the virus than distrust or a lack of safety and cooperation between individuals.
Other studies have found correlations between political polarization and populism on the one hand, and COVID-19 behavior and deaths on the other hand (Bayerlein et al., Citation2021; Charron et al., Citation2022; Morris, Citation2021). Of course, there is a difference between political polarization and populist governments on the one hand, and societal polarization and divisiveness on the other hand, but the two might go hand-in-hand as the first relies on and magnifies ideological differences between groups in society. Our finding suggests a pathway through attitudes of respect, community-feeling or even solidarity across society, despite ideological, religious, ethnic or social-economic differences between groups. It may also imply a shared attitude across groups that the virus is a common enemy, which can only be confronted jointly, aligning members of different groups to cooperate and to follow restrictive policy measures. The pathway implies that increasing polarization is not only detrimental for democracy but also for public health.
Robustness tests
Our first robustness test concerns income inequality, following the assumption that more unequal countries are less effective in dealing with the pandemic. shows that the negative effect of social cohesion on the number of COVID-19 deaths persists, while the Gini-coefficient has a small positive effect. This confirms our hypothesis that more unequal countries have higher COVID-19 infections and death rates.
Table 4. Social cohesion and Covid-19 infections and deaths controlling for inequality (Gini).
The second robustness test concerns the dependent variable. In order to check whether our results for social cohesion would change with a longer time period for COVID-19 cases, we ran the same regression with data beyond November 2020.Footnote10 We checked the change in the value of the coefficient for Social Cohesion for every single day for a whole year up to November 1st, 2021. shows the results of the trend in the coefficient (blue line and left axis) and in the value of the t-statistic (red line and right axis). The coefficient for social cohesion around November 1st, 2020 is −33, as we have shown in . Since that date, the size of the coefficient has gradually increased to −115, with a small decrease after September 2021 to −110. The average value of the coefficient over the additional years was −71, which is twice as much as the value that we obtained for November 1st, 2020.
Figure 2. Trend of the coefficient of social cohesion for Covid-19 infections for the period 1 November 2020–1 November 2021.

Finally, we also ran a robustness test with excess mortality replacing registered COVID-19 deaths, which resulted in similar results (see supplementary material tables A2 and A3). In addition, supplementary material tables A4 and A5 show the results where we include country regional groups, also showing very similar findings.
Discussion and conclusion
The results show consistent negative relationships between social cohesion on the one hand and the spread and mortality of COVID-19 on the other hand. Our results are in line with, but generally more robust than those obtained in other cross-country studies using less variables and/or smaller sample sizes, such as Barrios et al. (Citation2021), Gelfand et al. (Citation2021), Kumar (Citation2021) and Covid-19 National Preparedness Collaborators (Citation2022).
The relationship tends to be larger with narrower confidence intervals for the Intergroup Cohesion Index. This finding is an original contribution to the literature because empirical studies up to now only use variables that reflect social capital (within-group or interpersonal relations) or very general measures of social cohesion, but do not include the intergroup dimension of social cohesion. Our finding suggests that tolerance and respect, or even solidarity and cooperation, between different groups in a society may be more important for the adherence to policy measures and behavioral adaptations than only trust and cooperation between individuals. Qualitative research in countries with high distrust between groups supports this finding, for example in India. As the WHO cautioned, racial, religious or ethnic profiling of any kind is not useful as it leads to concealment of cases and delays in action (Down to Earth, Citation2020).
The results of our large cross-country sample provide four insights. First, a broad-based measure of social cohesion, that is clearly distinct from measures of social capital, shows strong negative correlations with COVID-19 infections and death rates. Second, intergroup cohesion shows larger and stronger results than interpersonal relationships, suggesting that divisiveness in societies can have a devastating public health effect. Third, our findings indicate that the effect of social cohesion is larger than the effects of the health care system, government capacity, a country’s level of economic development and democracy. Fourth, our findings also suggest that the effect of social cohesion remains when inequality is added, and that the effect of social cohesion seems to increase when the pandemic progresses over time.
We recognize that our analysis may have some weaknesses. The measures of social cohesion that we have used try to capture its complexity and layers, but they are not perfect. Also, our results do not necessarily extend to other epidemics.
In conclusion, we suggest that our study provides a novel perspective on the societal factors that have influenced the spread and mortality of COVID-19 across the world by pointing at the role of social cohesion, and in particular the cohesion between different social groups.
Supplemental Material
Download PDF (89.3 KB)Acknowledgements
This paper has benefitted from useful edits by Christina Sathyamala, helpful feedback at a seminar of the Rotterdam Global Health Initiative and suggestions at the ISD launch event of the 2020 data at the Institute of Social Studies in The Hague. We also acknowledge the important work on the database carried out by Christopher Knowles and Sergio Silvio Gutierrez. Finally, we gratefully acknowledge the feedback and comments we received from the two anonymous reviewers and the editor, which has clearly improved the text.
Disclosure statement
No potential conflict of interest was reported by the author(s).
Supplementary material
Supplemental data for this article can be accessed online at https://doi.org/10.1080/09581596.2023.2232526.
Additional information
Funding
Notes
1. For example, in the case of India, research indicates that it has been undercounting COVID-19 deaths (Srinivas, Citation2021).
2. For the data, see: https://www.who.int/data/stories/the-true-death-toll-of-covid-19-estimating-global-excess-mortality
4. For the combined index, please contact the authors.
5. This is a bootstrapping method to generate a stable ranking of countries on a scale between 0 and 1, as long as a country has data for at least three indicators. The key advantage is that it generates data for a large number of countries, as compared to an index consisting of less indicators but where all countries need to have data for every indicator in order to calculate a statistical average.
8. The regional grouping regressions use fixed effects estimations.
9. This result can be obtained from the authors and was not included due to lack of space.
10. We show only the results for COVID-19 infections and not death for reasons of space. Results for the trend in the coefficient of social cohesion for COVID-19 deaths can be obtained from the authors upon request.
References
- Alfano, V., & Ercolano, S. (2021). Social capital, quality of institutions and lockdown. Evidence from Italian provinces. Structural Change and Economic Dynamics, 59, 31–41. https://doi.org/10.1016/j.strueco.2021.08.001
- Barrios, J. M., Benmelech, E., Hochberg, Y. V., Sapienza, P., & Zingales, L. (2021). Civic capital and social distancing during the Covid-19 pandemic(☆). Journal of Public Economics, 193, 104310. https://doi.org/10.1016/j.jpubeco.2020.104310
- Bartscher, A. K., Seitz, S., Siegloch, S., Slotwinski, M., & Wehrhöfer, N. (2021). Social capital and the spread of covid-19: Insights from European countries. Journal of Health Economics, 80, 102531. https://doi.org/10.1016/j.jhealeco.2021.102531
- Bayerlein, M., Boese, V. A., Gates, S., Kamin, K., & Murshed, S. M. (2021). Populism and COVID-19: How populist governments (Mis)handle the pandemic. Journal of Political Institutions and Political Economy, 2(3), 389–428. https://doi.org/10.1561/113.00000043
- Borgonovi, F., Andrieu, E., & Subramanian, S. V. (2021). The evolution of the association between community level social capital and COVID-19 deaths and hospitalizations in the United States. Social Science & Medicine (1982), 278, 113948. https://doi.org/10.1016/j.socscimed.2021.113948
- Brodeur, A., Grigoryeva, I., & Kattan, L. (2021). Stay-at-home orders, social distancing, and trust. Journal of Population Economics, 34(4), 1321–1354. https://doi.org/10.1007/s00148-021-00848-z
- Bruhn, J. (2009). The group effect. Social Cohesion and Health Outcomes. Springer.
- Carrasco, M. A., & Bilal, U. (2016). A sign of the times: To have or to be? Social capital or social cohesion? Social Science & Medicine (1982), 159, 127–131. https://doi.org/10.1016/j.socscimed.2016.05.012
- Charron, N., Lapuente, V., & Rodríguez-Pose, A. (2022). Uncooperative society, uncooperative politics or both? Trust, polarization, populism and COVID-19 deaths across European regions. European Journal of Political Research. https://doi.org/10.1111/1475-6765.12529
- Christoforou, A., & Davis, J. B. (2014). Social capital and economics: Social values, power, and social identity. Taylor & Francis. https://doi.org/10.4324/9780203505014
- Coleman, J. S. (1988). Social capital in the creation of human capital. American Journal of Sociology, 94, S95–S120. https://doi.org/10.1086/228943
- COVID-19 Excess Mortality Collaborators. (2022). Estimating excess mortality due to the COVID-19 pandemic: A systematic analysis of COVID-19-related mortality, 2020–21. Lancet (London, England), 399(10334), 1513–1536. https://doi.org/10.1016/S0140-6736(21)02796-3
- COVID-19 National Preparedness Collaborators. (2022). Pandemic preparedness and COVID-19: An exploratory analysis of infection and fatality rates, and contextual factors associated with preparedness in 177 countries, from Jan 1, 2020, to Sept 30, 2021. Lancet (London, England), 399(10334), 1489–1512. https://doi.org/10.1016/S0140-6736(22)00172-6
- Dasgupta, P., & Serageldin, I. (1999). Social capital (Print ed.). World Bank. https://doi.org/10.1596/0-8213-4562-1
- Deaton, A. (2021). COVID-19 and global income inequality. LSE Public Policy Review https://ppr.lse.ac.uk/articles/10.31389/lseppr.26.
- Down To Earth. (2020). Refrain from religious profiling of COVID-19 cases: WHO in context of Tabligh. Down to Earth (New Delhi, India).
- Durkheim, É. (1951). Suicide (2nd ed.). Routledge.
- Durkheim, É. (1997). The division of labor in society (Paperback ed.). Free Press.
- Elgar, F. J., Stefaniak, A., & Wohl, M. J. A. (2020). The trouble with trust: Time-series analysis of social capital, income inequality, and COVID-19 deaths in 84 countries. Social Science & Medicine (1982), 263, 113365. https://doi.org/10.1016/j.socscimed.2020.113365
- Foa, R., & Tanner, J. (2010). Methodology of the indices of social development. Indices of Social Development. https://isd.iss.nl/wp-content/uploads/resources/Methodology%20of%20the%20Social%20Development%20Indices.pdf
- Gelfand, M. J., Jackson, J. C., Pan, X., Nau, D., Pieper, D., Denison, E., Dagher, M., Van Lange, P. A. M., Chiu, C., & Wang, M. (2021). The relationship between cultural tightness-looseness and COVID-19 cases and deaths: A global analysis. The Lancet Planetary Health, 5(3), e135–e144. https://doi.org/10.1016/S2542-5196(20)30301-6
- Granovetter, M. S. (1973). The strength of weak ties. American Journal of Sociology, 78(6), 1360–1380. https://doi.org/10.1086/225469
- Hangel, N., Schönweitz, F., McLennan, S., Fiske, A., Zimmermann, B. M., & Buyx, A. (2022). Solidaristic behavior and its limits: A qualitative study about German and Swiss residents’ behaviors towards public health measures during COVID-19 lockdown in April 2020. SSM Qualitative Research in Health, 2, 100051. https://doi.org/10.1016/j.ssmqr.2022.100051
- Kawachi, I., & Berkman, L. F. (2014). Social capital, social cohesion, and health. In L. F. Berkman, I. Kawachi, & M. M. Glymour (Eds.), Social epidemiology (p. 290). Oxford University Press. https://doi.org/10.1093/med/9780195377903.001.0001
- Kumar, R. (2021). Impact of societal culture on Covid-19 morbidity and mortality across countries. Journal of Cross-Cultural Psychology, 52(7), 643–662. https://doi.org/10.1177/00220221211025100
- Lieberman, E. S. (2009). Boundaries of contagion; How ethnic politics have shaped government responses to AIDS. Princeton University Press.
- Mallapaty, S. (2020). The coronavirus is most deadly if you are older and male - new data reveal the risks. Nature, 585(7823), 16–17. https://doi.org/10.1038/d41586-020-02483-2
- Manca, A. R. (2014). Social cohesion. In A. C. Michalos (Ed.), Encyclopedia of quality of life and well-being research (pp. 6026–6028). Springer Netherlands. https://doi.org/10.1007/978-94-007-0753-5_2739
- Min, J., & Capraro, V. (2020). Does social trust slow down or speed up the transmission of COVID-19? PLoS One, 15(12), e0244273. https://doi.org/10.1371/journal.pone.0244273
- Morris, D. S. (2021). Polarization, partisanship, and pandemic: The relationship between county-level support for Donald Trump and the spread of Covid-19 during the spring and summer of 2020. Social Science Quarterly, 102(5), 2412–2431. https://doi.org/10.1111/ssqu.13053
- Mukherjee, S. (2021, March). Why does the pandemic seem to be hitting some countries harder than others? The New Yorker. https://www.newyorker.com/magazine/2021/03/01/why-does-the-pandemic-seem-to-be-hitting-some-countries-harder-than-others
- Müller, S., & Rau, H. A. (2021). Economic preferences and compliance in the social stress test of the COVID-19 crisis. Journal of Public Economics, 194, 104322. https://doi.org/10.1016/j.jpubeco.2020.104322
- Popkin, B. M., Du, S., Green, W. D., Beck, M. A., Algaith, T., Herbst, C. H., Alsukait, R. F., Alluhidan, M., Alazemi, N., & Shekar, M. (2020). Individuals with obesity and COVID-19: A global perspective on the epidemiology and biological relationships. Obesity Reviews: An Official Journal of the International Association for the Study of Obesity, 21(11), e13128. https://doi.org/10.1111/obr.13128
- Romano, A., Spadaro, G., Balliet, D., Joireman, J., Caspar, V. L., Jin, S., Agostini, M., Bélanger, J. J., Gützkow, B., Kreienkamp, J., & Pontus Leander, N. (2021). Cooperation and trust across societies during the COVID-19 pandemic. Journal of Cross-Cultural Psychology, 52(7), 622–642. https://doi.org/10.1177/0022022120988913
- Shachat, J., Walker, M. J., & Wei, L. (2021). How the onset of the Covid-19 pandemic impacted pro-social behaviour and individual preferences: Experimental evidence from China. Journal of Economic Behavior & Organization, 190, 480–494. https://doi.org/10.1016/j.jebo.2021.08.001
- Spahl, W., Pot, M., & Paul, K. T. (2022). Understanding compliance as multi-faceted: Values and practices during the COVID-19 pandemic in Austria. Critical Public Health, 32(5), 665–676. https://doi.org/10.1080/09581596.2022.2039379
- Srinivas, R. (2021). Whole numbers and half truths : What data can and cannot tell us about modern India. Context, an imprint of Westland Publications Private Limited.
- van Staveren, I., & Knorringa, P. (Eds.). (2008). Beyond social capital. Routledge. https://doi.org/10.4324/9780203827109
- Williams, S., Crookes, A., Glass, K., & Glass, A. J. (2021). COVID-19 mortalities in England and Wales and the Peltzman offsetting effect. Applied Economics, 53(60), 6982–6998. https://doi.org/10.1080/00036846.2021.1955089
- Zhu, N., Smetana, J. G., & Chang, L. (2021). Acceptance of society-level and individual-level preventive measures during the COVID-19 pandemic among college students in three societies. Journal of Cross-Cultural Psychology, 52(7), 606–621. https://doi.org/10.1177/0022022121995971
- Zimmermann, B. M., Fiske, A., McLennan, S., Sierawska, A., Hangel, N., & Buyx, A. (2022). Motivations and limits for COVID-19 policy compliance in Germany and Switzerland. International Journal of Health Policy and Management, 11(8), 1342–1353. https://doi.org/10.34172/ijhpm.2021.30