ABSTRACT
The purpose of this paper is to identify strategies for integrating data analytics into teaching management accounting. We conducted a literature review and evaluated students’ perceptions of the introduction of data analytics in teaching management accounting courses. This research is based on the application of the Extended Technology Acceptance Model (ETAM) in management accounting education. We link the findings to the existing theory and practice by providing a foundation for the implementation of data analytics in management accounting subjects and the development of educational resources at both, national and international levels. Thus, the results will be of interest to global readership revealing that data analytics is considered by students both useful and easy to use and concluding that students would benefit from integrating data analytics into management accounting courses across the areas identified in this study. From our analysis of the results, we conclude that a high level of appreciation of such inclusion across perceived usefulness and perceived ease of use of data analytics leads to a positive attitude towards using and potentially high actual use of data analytics.
Introduction
This study aims to look at the management accounting courses’ effectiveness by analysing students’ perceptions of introducing data analytics topics in their curriculum.
In response to the growing demand for data analytic skills in the accounting professional industry, this research has important implications for both national and international academia and practitioners, contributing to a better understanding of how management accounting curricula should be changed from an educational perspective.
The results showed that the inclusion of data analytics is significantly positively related to the perceived usefulness and ease of use of data analytics in management accounting tasks and is likely to promote a positive attitude towards using systems and encourage their actual use in professional settings.
To address the research question of how the incorporation of data analytics in management accounting impacts student perceptions, an ETAM model was used with an exploratory factor analysis through the lens of the technology acceptance model (TAM) as well as partial least squares structural equation modelling (PLS-SEM) was performed.
In particular, we aim to address two specific research questions:
Based on existing research, how should data analytics be introduced to management accounting courses? (RQ 1)
In the context of the Extended Technology Acceptance Model (ETAM), how do students perceive the addition of data analytics to management accounting courses? (RQ 2)
A comprehensive literature review supplements the results to identify the top topics of data analytics required in management accounting courses (Phase 1). Those topics were added to a management accounting curriculum, and students’ perceptions of the addition were evaluated using the ETAM (Phase 2).
Data analytic skills are in high demand in professional business environments; they can simplify or automate routine tasks that management accountants perform and therefore work for further development of information systems in accounting (Stoner et al., Citation2020). There is a growing need for specialized analytics training from accounting educators, but modern curricula have been slow to adapt to modern job expectations (AICPA and NASBA, Citation2023 CPA Australia, Citation2021; Dow et al., Citation2021).
It isn’t entirely clear how best to integrate data analytics skills into existing classes. The topic is considered in some recent academic and practitioner literature, but such studies are still quite selective and emerging (Losi et al., Citation2022). This paper addresses this gap, reviewing how accounting education can respond to industry demands by equipping graduates with data analytics knowledge and skills (Moll & Yigitbasioglu, Citation2019).
Data analytics has been rapidly changing all areas of accounting over the past decades, and lacking analytical skills affects accounting graduates’ employability. The job market requires data literacy, data visualisation, and data story-telling skills, as well as proficiency in data analytics software, including PowerBI, Tableau, SQL, and Python (AICPA and NASBA, Citation2021, Citation2023; CPA Australia, Citation2021). During an economic downturn, tightening competition, and thinning job opportunities, accounting careers are being filled by IT candidates who offer highly sought-after data analytics skills. This causes additional threats to the employability of accounting students and is a concern for accounting academics.
The accounting profession is taking active steps to upskill its members and equip accountants with the required data knowledge and skills (AACSB, Citation2018; CPA Australia, Citation2020). However, there are insufficient educational resources to address this gap (Dai & Vasarhelyi, Citation2023; Nielsen, Citation2022). Data analytics must be integrated into education at the tertiary level (Moll & Yigitbasioglu, Citation2019) to ensure job readiness and facilitate professional development and upskilling in data analytics.
There have been attempts to integrate data analytics in different areas of accounting education, including financial accounting and auditing (Earley, Citation2015; Prokofieva, Citation2023; Sangster, Citation1992, Citation1995; Van der Heijden, Citation2023), but such attempts are non-existent in the management accounting area (Dalwai et al., Citation2021; Rikhardsson & Yigitbasioglu, Citation2018). We attempt to address this void and focus on the area of management accounting which is strategically suited for data analytics: advising management and providing information for decision making, including budgeting/planning, risk management, and long-term strategizing.
The paper uses a two-phase design. RQ1 was addressed by conducting a comprehensive qualitative literature review in Phase 1. The results were used to identify five major themes that were later included as topics to embed into the management accounting curriculum. The developed resources were used further to address RQ2 and to evaluate students’ perceptions using ETAM.
The comprehensive literature review that addresses RQ1 used data mining techniques to source papers and then employed the thematic mapping approach (Smith, Citation2016) to identify major themes. The identified themes were re-aligned with the existing management accounting curriculum and incorporated guidelines from the main accounting professional bodies, e.g. AICPA’s 2024 exam exposure draft focusing on Data and Technology Concepts (AICPA, Citation2022). This alignment was done to ensure that the adjusted curriculum would still satisfy the course accreditation requirements for accounting degrees. The study used professional accreditation guidelines from CPA Australia (Citation2023) related to main competency areas as guidance. The suggested changes in the curriculum were then evaluated for RQ2 using a survey of students who participated in the management accounting course. Additional feedback was solicited from academics who teach data analytics and management accounting.
Contribution
Yigitbasioglu et al. (Citation2023) and Jackson et al. (Citation2023) review the need for broadening the research literature on technology and its impact on student employability skills. To address this issue, this study makes the following important contributions to the existing knowledge. Firstly, it explores what topics should be incorporated into the management accounting curricula based on the relevant literature review. While the existing literature addresses the practice of covering particular issues within accounting education, the present study suggests a novel examination of the need to incorporate a set of specific topics into the management accounting course. Secondly, it examines the impact of such inclusion from the students’ perspective.
The next section of this paper provides an overview of data analytics, both in the recent literature and within its connection to accounting, followed by a brief overview of the ETAM used in the study. Further section describes the research methods employed, and Section 4 presents the results. The final section concludes with a discussion of findings, followed by the limitations of the study and directions for future research.
Background and literature review
Data analytics and accounting
Data flow, acceleration in information creation, knowledge mining, and the need for agile decision-making are putting great pressure on the accounting profession. Information system developments and rapid growth in technology accessibility have forced the accounting profession to adapt and evolve. The importance of data and the demands to use it have increased dramatically over recent years, both within and without accounting (Richardson et al., Citation2019), and companies face pressure to implement new technologies (Jackson et al., Citation2023). Traditional methods of collecting, aggregating, and analysing data may not yield optimal results for business decision-making (Appelbaum et al., Citation2017). Recent literature recognizes the need for data-driven decision-making experience, data literacy, and data analytics skills in accounting (Appelbaum et al., Citation2017; Drew, Citation2018; Dzuranin et al., Citation2018).
Technological disruptions affect the way companies conduct business and leverage their IT investment by creating value by using analytics and automation (Dow et al., Citation2021). Accelerating routine tasks and daily processes significantly increased efficiency and improved controls (Appelbaum et al., Citation2021). Companies have bolstered their investment in standalone and cloud IT infrastructure, allowing them to integrate data analytics into their day-to-day operations, automate formerly manual processes, integrate accounting/reporting systems, and ensure the transparency, accountability, and audibility of financial data (Kogan et al., Citation2021). Data-driven companies are more able to create value from agile decision-making (Hoelscher & Mortimer, Citation2018).
A large part of the existing literature is focused on investigating the advisory board’s (i.e. AACSB) calls for changes in accounting training (Dow et al., Citation2021). There is a need for a consistent and efficient framework for incorporating data analytics into accounting education, one that meets the demands of professional bodies (e.g. the AASCB, Citation2018; the American Institute of CPAs, Citation2020, the UK Association of Chartered Accountants, 2020, the Institute of Management Accountants) and integrates educators’ perspectives (Ballou et al., Citation2018; Dzuranin et al., Citation2018). Another focus of the literature is on transforming individual accounting subjects (e.g. Richardson & Shan, Citation2019) and introducing data analytics elements and tools to specific topics (e.g. Andiola et al., Citation2020; Prokofieva, Citation2021). The current paper is an attempt to connect these aspects of the literature.
Data analytics
Data analytics commonly refers to working with big data to discover and present valuable insights and patterns (Moll & Yigitbasioglu, Citation2019). Large datasets have become widespread in businesses as they naturally have access to a wide variety of valuable data: transactional data related to business operations, customer management, product information, etc. (Prokofieva, Citation2021; Prokofieva, Citation2023). Analysing that wealth of data involves inspecting, cleaning, and transforming it to develop data models to describe past events, explain financial insights, predict future events, and prescribe actions that are likely to lead to desired outcomes. The primary aim of data analytics is to support decision making, and in many cases, specifically business-related decisions, supporting agile approach and working with big data.
The primary subject of data analytics is ‘big data’ which is usually characterised by five Vs: volume, velocity, variety, veracity, and value (McAfee & Brynjolfsson, Citation2012). Volume refers to a large number of entries in big datasets, velocity to the speed the data is generated, variety to the high number of data sources, veracity of data sources’ diverse origins, and value to the insights and material changes to operations that can be driven by big data analytics. Big data was made possible by the development of IT technology that allows for quick transformation, communication, and aggregation of data, but processing and analysing big data comes with unique challenges. These challenges affect the timing, cost, and accuracy of data-driven decision-making. Despite this, data analysts have developed tools to facilitate working with big data, generating otherwise obtuse insights, and making analytics critical for a successful business operation.
Data analytics approaches can be classified into 4 different aspects: descriptive, diagnostic, predictive, and prescriptive. These types have different goals and vary in both the complexity and value they add to a business.
Descriptive analytics is used most often by businesses to answer the question ‘What happened?’ (IBM, Citation2013). It is characterised by relatively low complexity and usually refers to the use of descriptive statistics (Shmueli & Koppius, Citation2011) supported by visualization approaches (Dilla et al., Citation2010). While it generates valuable insights, its value is rather limited as these analytics is mainly retrospective and aims to use past data to summarise what happened. It is employed in monitoring business performance, understanding patterns, establishing trends, and benchmarking against some indicators (e.g. historical ratios).
Diagnostic analytics is focused on further exploration of data to diagnose what happened and establish the causes of the trends or events. Building on descriptive analytics, diagnostic analytics moves further to understand ‘Why did it happen?’ (IBM, Citation2013). It aims at root cause analysis and employs data discovery, data mining, drill down, and drill through analyses. Knowledge discovery, or knowledge extraction, refers to the creation of knowledge from different sources. However, for diagnostic analytics, the prevailing data is still in a structured format. Diagnostic analytics also uses data mining approaches, which refer to the analytical process when large datasets are used to extract and discover patterns and useful insights in raw data. By searching for specific patterns or trends, analysts can address a business problem and generate knowledge and insights for a decision-maker. Originally, it was viewed as one of the steps in knowledge discovery in databases.
In most cases, descriptive and diagnostic analytics are merged in the data analysis flow; data is used to develop statistical models to test causal hypotheses that attempt to explain specific empirical events. The starting point is the exploration of the data to build a causal theoretical model, which is developed into a set of hypotheses tested later by a statistical model using statistical inference ().
Figure 1. Workflow for the descriptive and diagnostic analytics.Footnote3
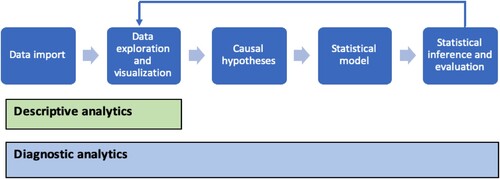
Predictive analytics offers the next step in complexity and adds further value to business decision-making by using past data to look into the future: ‘What will happen?’ (IBM, Citation2013). It uses predictive techniques, often relying on machine learning, to build predictive and probability models, forecasting approaches, etc. It usually requires advanced knowledge of statistics, Bayesian modelling, and neural networks.
Predictive analytics develops models aimed at empirical predictions, i.e. models that can predict new observations or scenarios (Shmueli & Koppius, Citation2011). While this type of analytics works with various data sources, it also offers tools to work with semi-structured and unstructured data, such as text and images. Additionally, unlike previous types of analytics, the focus of predictive analytics is on the accuracy of the prediction, often sacrificing the explanatory aspect of the model; a predictive analytics model may be a ‘black box,’ with data as input, a prediction as output, and no way of explaining what happens in between the two ().
Figure 2. Predictive analytics workflow.Footnote4
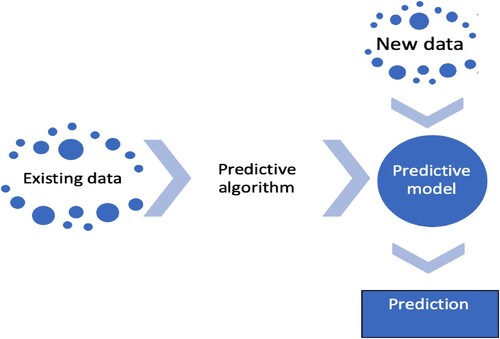
Prescriptive analytics maximises the value added to business decision-making but also demands a high level of complexity. It answers the question ‘What should be done?’ and employs an array of sophisticated statistical and machine-learning approaches (Lepenioti et al., Citation2020). The goal of prescriptive analytics is to provide solutions or scenarios to business problems or situations. Often this involves optimization approaches and heavily uses methods that are also employed in predictive analytics, but prescriptive analytics tends to take a closer look at business scenarios or events, including the availability of data, various data sources, and business inputs. As with predictive analytics, it also develops predictive models, but the aim is to suggest the best course of action to maximise business performance and improve business processes (), rather than increase the accuracy of a prediction (Bertsimas & Kallus, Citation2020).
Figure 3. Prescriptive analytics workflow.Footnote5

ETAM
While data analytics can offer significant benefits to management accountants, creating favorable conditions for its embedding in the curriculum, its adoption and implementation may present substantial difficulties and not particularly technology related (Möller et al., Citation2020). Acceptance of technology is a multifaceted phenomenon. Several competing theoretical frameworks were proposed over the years to explain the use and adoption of new information systems, including two main approaches – ETAM (Davis, Citation1989) and the Theory of Planned Behaviour (TPB) (Ajzen, Citation1991). While ETAM centres around personal adoption and use of technology, TPB emphasizes social influences on technology adoption and use (Chu & Chen, Citation2016).
ETAM aims to predict users’ acceptance and highlights potential issues with users’ interactions with technology (Dillon & Morris, Citation1996; Mohd et al., Citation2011). ETAM is one of the most popular models for understanding user attitudes and evaluating students’ perceptions of using new technologies in various applications and disciplines.
ETAM consists of several components ():
Figure 4. TAM components.Footnote6
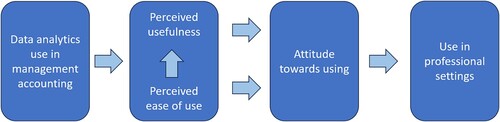
The figure above represents the workflow of data analytics use (DAU) in management accounting, users’ Perceived Usefulness (PU), Perceived Ease of Use (PEOU), and their effect on users’ perceptions of the system (Davis, Citation1989; Dillon & Morris, Citation1996). PU represents the degree to which a person believes that using a particular system would enhance their task/job performance, and PEOU shows the degree to which a person believes that using a particular system would be free of effort. At the same time, PU has a direct significant influence on a student’s attitude toward using data analytics in management accounting scenarios and, according to theory, affects their intention to use data analytics. For example, if students perceive benefits in data analytics, they will have a positive attitude and therefore will be more willing to use it in accounting tasks (Al-Ammary et al., Citation2014; Ansong-Gyimah, Citation2020).
Among the discussed constructs, PU and PEOU form key students’ beliefs on using data analytics and therefore predict their attitude toward analytic technology, which in turn predicts whether they will accept it as a prospective tool (Ma & Liu, Citation2004). In the current study, the PU component represents the perceived usefulness of data analytics to complete management accounting tasks in the workplace, and PEOU represents the perceived ease of use of data analytics tools to address management accounting routine tasks. When students perceive that the application of data analytics, i.e. data analytics use (DAU), is uncomplicated and data analytics tools are easy to use, they are more likely to adopt a positive attitude. The interaction between PEOU and PU has mainly a positive effect on the continued acceptance of the technology. The more that students perceive data analytics tools as stress-free, the more they are likely to continue using them (Bhatiasevi & Yoopetch, Citation2015; Lakhal & Khechine, Citation2021).
These two factors are critical as they affect behavioral intention to use data analytics to complete learning activities within the management accounting course, while potentially predicting future use of analytics in the workplace (i.e. as a proxy for actual usage).
The extension of the model was suggested by Venkatesh and Davis (Citation2000) who outlined perceived usefulness and usage intentions as related to social influence and cognitive components. The existing literature suggests that while both ETAM and TPB can predict the intention to use data analytics, ETAM offers more empirical advantages, including easy application and broader and more generalizable guidance about system use. The current study employs ETAMFootnote1, where the cognitive element is reflected in the application of data analytics to solve management accounting problems presented as a real-world case.
Research methods
To address the outlined research questions the two-phase design was developed. During Phase 1, RQ1 was addressed by undertaking a comprehensive literature review. The results of the review were used to implement data analytics in a management accounting course for undergraduate-level students at a European university. The implementation process, guided by ETAM, was completed over two semesters in 2021-2022. The evaluation of the implementation was completed at the end of each semester and was the basis for addressing RQ2.
Phase 1 (RQ1)
To address RQ1, which focuses on how data analytics should be introduced when teaching a management accounting course, the study used a comprehensive qualitative literature review to identify the main themes in the existing management accounting and data analytics literature and incorporate these themes into teaching.
Both empirical and theoretical research papers were reviewed and integrated where appropriate. Information in each sampled paper was reviewed and aggregated to match the management accounting curriculum (Apostolou et al., Citation2018; Dixon-Woods, Citation2011; Gough et al., Citation2012). The inclusion of both quantitative and qualitative studies provided extended coverage of the problems raised and a more comprehensive overview compared to other types of literature reviews.
The literature review started with problem identification, which was to identify the main areas in management accounting that benefit from data analytics. The developed themes were framed to map such areas based on existing studies and reported industry practices.
Given the scope of the review, the study used academic databases that include theoretical and empirical papers, conference proceedings, and industry reports. To conduct the review, several databases were used, including Web of Science, Business Source Complete, and Google Scholar. The search query used ‘analytics AND (management accounting OR accounting education)’. The search was limited to studies published in English between 2017-2022 to focus on more recent developments in data analytics and its role in accounting education. An extended search confirmed only limited studies were available before 2017. Given changes in the technology, earlier publications were also dated and not applicable. Only scholarly papers (published papers and early access papers) were included in the analysis with a focus on educational research, business, and computer science research to provide a comprehensive coverage of the topic.
The initial search identified 19,351 publications in Web of Science, 2,304 in Business Source Complete and Google Scholar. During the evaluation stage, the papers were further screened to remove duplicates, irrelevant studies (as defined in the aims of the paper), and low-quality publications (as defined by the journal ranking in ScimagoFootnote2). A manual review of the papers by two independent researchers reduced the dataset to 531 papers initially. Further review of the papers relevant to teaching management accounting resulted in a further reduction to 34 papers that were analysed to identify five major themes:
Inventory management: identification of applicable data analytics approaches and tools
Cost analysis: working with different data formats and tools
Variance analysis using PowerBI
Performance management: using dashboards and visuals for communication with stakeholders with PowerBI/Tableau
Risk management in short-term and long-term decision making: using basic machine learning for forecasting.
Phase 2 (RQ2)
Phase 2 of the research targeted RQ2, which refers to students’ perceptions of data analytics in teaching management accounting. During this phase, the developed teaching materials were used in class and a survey was conducted to evaluate students’ perceptions. The developed teaching material underwent consultations with academics and data analytics experts before inclusion in the course. The received feedback was incorporated and implemented in the final version of the management accounting course content. The study included a questionnaire based on ETAM to evaluate students’ perceptions of the usefulness of data analytics within management accounting courses. The two variables represented above (), ‘Attitudes toward using data analytics in management accounting’ and ‘Use in the professional settings’ were not measured in this study.
Participants were third-year undergraduate students pursuing an accounting degree from a major European University. The developed curriculum was offered in two semesters from 2021–2022 and occupied five sessions in each semester. Each session was focused on one of the five topic areas specified in Section 1 Appendix 1. With five distinct topics related to management accounting areas (Section 1 Appendix 1), the evaluation of Perceived usefulness (Section 2) and perceived ease of use (Section 3) remained consistent.
Students were pretested to understand their background and level of IT skills. Pretesting suggested that, though students were familiar with general information systems and their use, their knowledge, and skills in data analytics for management accounting were limited: 70% of respondents felt confident using Excel and almost 90% of respondents identified themselves as regular users of information systems applications in their daily life, but only 10% of respondents identified themselves as advanced users of IT solutions. For each semester and each session, a two-hour workshop was organised, where students were invited to complete a scenario that required them to apply data analytics to management accounting tasks. At the end of the workshop, participants were asked to complete an anonymous questionnaire to evaluate their perception of the developed materials and to proxy their perception of data analytics in management accounting (Appendix 1).
An example of the multiple-choice questions used to measure Data Analytics Use (DAU) is provided below:
‘Visual explanations (such as diagrams, tables, graphs, images) lead to greater understanding of materials.’ The students were suggested to choose ‘True’ or ‘False’, and the obtained results were used to measure the variable.
The scenario was aligned with Appendix 2 and workshops were delivered by course instructors. Out of 120 students enrolled in the course, 90 valid responses were received.
Instrument
The employed questionnaire was adopted from prior studies that use ETAM for the E-Service model (Taherdoost, Citation2018), procedural learning (Lee & Lehto, Citation2013), and Online Consumer Behavior (Koufaris, Citation2002). Since ETAM has been implemented in various information system contexts, it is useful for exploring the adoption behavior of a particular technology (Fathema & Sutton, Citation2013).
Based on an analysis of existing research, we have developed ETAM factors that were included in the survey. In the model above (see ), the perceived usefulness and perceived ease of use are represented as the main determinants of user motivation. In the current research, we adopted the ETAM within the implementation of data analytics into management accounting by third-year undergraduate students.
The questionnaire structure is represented in Appendix 1 and includes three sections. The first section related to the use of management accounting area (‘attitude towards using’ and ‘use in professional settings’ components in ETAM) within the five previously identified topics. This part supported a management case presented in the workshop and targeted assessment of the student’s understanding of management accounting concepts and the application of data analytics tools to the problems raised in the case. Each of the five workshops in each semester related to only one specific management accounting topic. Sections 2 and 3 were aligned with the Perceived Usefulness and Perceived Ease of Use components of ETAM and were adapted from the ETAM literature (Koufaris, Citation2002; Lee & Lehto, Citation2013; Taherdoost, Citation2018).
Before the survey, the questionnaire was presented to a group of accounting and IT academics for their feedback, which was incorporated into the final version of the instrument. The breakdown of the instrument items is presented in Appendix 1. A five-point Likert scale was used for each item, with responses ranging from (1) Strongly Disagree; (2) Disagree; (3) Neither Agree nor Disagree; (4) Agree; (5) Strongly Agree. To encourage voluntary participation, we did not force responses. Therefore, the number of respondents for each task varied, with 90 responses for Section 1, 81 responses for Section 2, and 77 responses for Section 3. After completing the first task, the participants were instructed to proceed to the second part of the survey. The list of items in the instrument used to assess PEOU and PU is provided in Appendix 1.
Findings
Phase 1
Phase 1 results were analysed to understand trends in the current literature. and below show the distribution of results across subject areas, publication years, and countries to illustrate trends of accelerated growth in the literature over recent years and the geography of the existing studies.
The conducted analysis showed that most of the papers come from educational research and computer science areas, with a large proportion of publications released in recent years (2020-current), supporting the argument that there is increasing interest in using data analytics in management accounting education.
Further analysis includes the distribution of the relevant research papers across years. The results suggest that there was a significant increase in the number of relevant research papers in recent years ().
There is a strong, consistent rise in the amount of research papers devoted to the topics defined. This can be viewed as evidence of the existing demand for change in accounting education practice. We further analysed the distribution of the research papers across countries, and the results show that the main share of papers was written by authors from more such countries as USA and UK, with an increasing number of papers coming from China.
A sample of the collected papers is available in Appendix 3. The results were used to identify five major themes, including the following:
The skillset required to perform data analytics, with a focus on broader skill categories, such as critical thinking, analytical skills, and programming skills.
Data management in accounting, including working with different data formats and presentation formats.
Business intelligence solutions, including working with software applications like Microsoft Excel, Microsoft PowerBI, and Tableau.
The role of management accountants in business transformations, including digital innovation and the evolution of business models.
Emerging technologies, including machine learning and AI, blockchain, etc.
Phase 2
The main topics of the management accounting courses following the themes identified are introduced further in the research. We believe that the obtained results help improve student learning and student retention within the management accounting course experience. shows the alignment of management accounting topics with data analytics areas (i.e. identified themes) and provides sample papers for each entry.
Table 1. Example of introducing the data analytics within the Management Accounting course with domain knowledge alignment.
To examine student views about data analytics in management accounting tasks, the participants were asked to complete a management accounting scenario where they were required to use their accounting knowledge as well as data analytics skills (as discussed earlier). Demographic data was collected as part of the questionnaire as presented in . The sample characteristics are consistent with the overall population.
Table 2. Demographic information about participants.
The questionnaire structure is shown in Appendix 1 and includes three sections. The first section relates to management accounting (the Actual System Use component in ETAM), while sections 2 and 3 are aligned with the Perceived Usefulness and Perceived Ease of Use components of ETAM. Section 1 included questions specific to the case and targeted all five topic areas identified in Phase 1 .
Table 3. Survey results.
The survey results underwent preliminary screening and analysis to confirm the data quality and normality of the distribution. The study followed a procedure for ETAM studies and assessed the internal consistency of the results using Cronbach’s alpha and composite reliability. Factor loadings and average variance extracted (AVE) were used to estimate the convergent validity, which is measured using factor analysis. Assessment of the discriminant validity was completed using the HTMT criterion (Anderson & Gerbing, Citation1988; Henseler et al., Citation2015; Hair, 2018). Construct convergent validity was confirmed with AVE > 0.5 and factor loadings for all items were above 0.651. Cronbach’s alpha and composite reliability were estimated and matched the required threshold value of 0.7 to confirm their reliability (Hair, 2018).
To address RQ2, the results were evaluated across three dimensions: DAU, PU, and PEOU. Following prior literature, partial least squares structural equation modelling (PLS-SEM) was performed using SmartPLS 3 (Anderson & Gerbing, Citation1988; Hair et al., Citation2019; Henseler et al., Citation2015). The choice of procedure was guided by sample size limitations (Avkiran, Citation2018) and data selection recommendations (Hair Jr et al., Citation2017, Hair et al., Citation2018).
The results of the path analysis are shown in below. According to that, data analytics use has a positive impact on perceived usefulness (0.867, p < 0.001) and ease of use (0.732, p < 0.001) and suggests a positive perception of data analytics use in management accounting tasks. The coefficient of determination (R2) was calculated at 0.800 to confirm the predictive power of the model for the endogenous variables.
Table 4. Perceived usefulness and ease of use determination.
The results of the study indicate a positive attitude of students toward the use of data analytics in management accounting tasks, reflected in their perception of ease of use and perceived usefulness of data analytics applications. These findings confirm prior results where the adoption of IT solutions is reflected through their perceived usefulness and ease of use to promote positive attitudes towards using systems and actual use in professional settings (Mortenson & Vidgen, Citation2016). This is particularly important where IT solutions (i.e. data analytics) complement professional skill sets (i.e. accounting knowledge and skills) and extend the application of ETAM to data analytics (Verma et al., Citation2018).
Discussion and conclusion
This paper aimed to investigate the management accounting course curricula through the students’ perceptions of introducing data analytics topics in it. The study contributes to the limited literature that has examined the potential implications of data analytics on accounting education and profession. In addition, the paper emphasizes the need for a global change in management accounting education by providing evidence of the existing demand for data analytic skills in various countries. Furthermore, this study contributes to the limited research on data analytics in management accounting by testing the inclusion of data analytics in management accounting education and reviews the interconnections between data analytics, management accounting, and IT skills of accounting undergraduates. The paper extends ETAM and employs it to evaluate the development of a management accounting curriculum that embeds data analytics.
Through the two-phase design, including a comprehensive literature review (Phase 1 related to RQ1) and implementation of data analytics in the management accounting course (Phase 2 related to RQ2), the results of this study facilitated the identification of five themes to include when teaching data analytics to management accounting students. We argue that the current challenges of data analytics provide opportunities to develop accounting education by introducing different approaches to management accounting courses. The evaluation of student’s perceptions through ETAM reveals the potential for further changes in accounting education toward data analytics as a must-have skill in accounting graduates’ professional work.
The results are thus consistent with Ballantine et al., Citation2024 conclusion that ‘Without change, the discipline and the profession will become increasingly irrelevant to the needs of business, organisations, and government, as well as society and the broader public interest’. Thus, accounting education has yet to address this demand and incorporate the development of data analytics skills in the accounting curriculum.
This paper contributes to the theoretical understanding and practical application of data analytics in management accounting education and will be of interest to accounting academics and practitioners at both, national and international levels. Through the research conducted, we identified 6 main areas for potential inclusion of data analytics themes, as follows:
- New role and expectations for management accountants
- Cost management
- Capital budgeting
- Planning and control
- Variance analysis
- Managing risk and uncertainty
Current business practices focus on these topics and undergo constant changes with technological advancements and innovation. These developments put more emphasis on accountants’ capacity as business advisors over traditional roles relating to record-keeping and compliance (Howieson, Citation2003), and require changes in how accounting is taught globally.
The inclusion of the identified themes within two semesters of a management accounting course showed that students perceived data analytics as a useful and accessible tool, as reflected in survey results relating to perceived usefulness and ease of use. Students support implementing data analytics in accounting tasks, as reflected in their positive perceptions towards data analytics use in management accounting tasks and positive attitudes towards using data analytics in their future careers.
This study is subject to several limitations. The research area is narrowed to management accounting with a limited focus on accounting undergraduate students. Further research could be extended to the other educational levels (e.g. master’s degree, micro degree, MBA, Ph.D., etc.) to evaluate common trends and differences in students’ perceptions across groups with different professional experiences and existing skill sets. Further work may lead to developing self-paced learning resources that can be integrated at different levels of education and industry offerings (e.g. upskilling, and professional development opportunities). The extension of the research is suggested in understanding learners’ perceptions of data analytics use from the point of external factors that inhibit or promote actual use of the technological solutions and time required for the development of active use.
Acknowledgments
We thank the Editor-in Chief (Alan Sangster), the Associate Editor and the reviewers for their insight and helpful suggestions. We are also grateful to Miklos Vasarhelyi and Steven Katz for their helpful comments.
Disclosure statement
No potential conflict of interest was reported by the author(s).
Notes
1 An in-depth discussion of ETAM and its comparison with TPB is out of the scope of this paper, but can be found in a recent literature review in Granić and Marangunić (Citation2019).
3 Source: developed by the authors based on conducted research.
4 Source: developed by the authors based on conducted research.
5 Source: developed by the authors based on conducted research.
6 Source: adopted from Davis, Citation1989.
References
- AICPA. (2022). Maintaining the Relevance of the Uniform CPA Examination – Aligning the Exam with the CPA Evolution Licensure Model. https://www.aicpa.org/resources/download/exposure-draft-proposed-2024-cpa-exam-changes
- AICPA and NASBA. (2021). Accounting program curriculum gap analysis. https://www.evolutionofcpa.org/Documents/Accounting%20Program%20Curriculum%20Gap%20Analysis%20Report%203.15.2021.pdf
- AICPA and NASBA. (2023). Evolving initial CPA licensure requirements to build a future-ready profession for a rapidly changing marketplace. https://www.evolutionofcpa.org/
- Ajzen, I. (1991). The theory of planned behavior. Organizational Behavior and Human Decision Processes, 50(2), 179–211. https://doi.org/10.1016/0749-5978(91)90020-T
- Al-Ammary, J. H., Al-Sherooqi, A. K., & Al-Sherooqi, H. K. (2014). The acceptance of social networking as a learning tools at University of Bahrain. International Journal of Information and Education Technology, 4(2), 208–214. https://doi.org/10.7763/IJIET.2014.V4.400
- Anderson, J. C., & Gerbing, D. W. (1988). Structural equation modeling in practice: A review and recommended two-step approach. Psychological Bulletin, 103(3), 411–423. https://doi.org/10.1037/0033-2909.103.3.411
- Andiola, L. M., Masters, E., & Norman, C. S. (2020). Integrating technology and data analytic skills into the accounting curriculum: Accounting department leaders’ experiences and insights. Journal of Accounting Education, 50, 100655. https://doi.org/10.1016/j.jaccedu.2020.100655
- Ansong-Gyimah, K. (2020). Students’ perceptions and continuous intention to use e-learning systems: The case of google classroom. International Journal of Emerging Technologies in Learning (iJET), 15(11), 236–244. https://doi.org/10.3991/ijet.v15i11.12683
- Apostolou, B., Dorminey, J. W., Hassell, J. M., & Rebele, J. E. (2018). Accounting education literature review (2017). Journal of Accounting Education, 43, 1–23. https://doi.org/10.1016/j.jaccedu.2018.02.001
- Appelbaum, D., Kogan, A., & Vasarhelyi, M. A. (2017). Big data and analytics in the modern audit engagement: Research needs. Auditing: A Journal of Practice & Theory, 36(4), 1–27. https://doi.org/10.2308/ajpt-51684
- Appelbaum, D., Scott Showalter, D., Sun, T., & Vasarhelyi, M. A. (2021). A framework for auditor data literacy: A normative position. Accounting Horizons, 35(2), 5–25. https://doi.org/10.2308/HORIZONS-19-127
- Association of Chartered Certified Accountants. (2020). Syllabus. ACCA Global. Accessed April 7, 2020.
- Association to Advance Collegiate Schools of Business (AACSB). (2018). Eligibility Procedures and Standards for Accounting Accreditation. Tampa, FL: AACSB. Available at: https://www.aacsb.edu/-/media/documents/accreditation/accounting/standards-and-tables/2018-accounting-standards.pdf?rev=a2f3f6a396c24b0eb357b19a41c6cba8&hash=965FED94C79C756976B038B5F2151591
- Avkiran, N. K. (2018). An in-depth discussion and illustration of partial least squares structural equation modeling in health care. Health Care Management Science, 21(3), 401–408. https://doi.org/10.1007/s10729-017-9393-7
- Ballantine, J., Boyce, G., & Stoner, G. (2024). A critical review of AI in accounting education: Threat and opportunity. Critical Perspectives on Accounting, 99(2024), 102711. https://doi.org/10.1016/j.cpa.2024.102711
- Ballou, B., Heitger, D. L., & Stoel, D. (2018). Data-driven decision-making and its impact on accounting undergraduate curriculum. Journal of Accounting Education, 44, 14–24. https://doi.org/10.1016/j.jaccedu.2018.05.003
- Bertsimas, D., & Kallus, N. (2020). From predictive to prescriptive analytics. Management Science, 66(3), 1025–1044. https://doi.org/10.1287/mnsc.2018.3253
- Bhatiasevi, V., & Yoopetch, C. (2015). The determinants of intention to use electronic booking among young users in Thailand. Journal of Hospitality and Tourism Management, 23, 1–11. https://doi.org/10.1016/j.jhtm.2014.12.004
- Chu, T. H., & Chen, Y. Y. (2016). With good we become good: Understanding e-learning adoption by theory of planned behavior and group influences. Computers & Education, 92-93, 37–52. https://doi.org/10.1016/j.compedu.2015.09.013
- CPA Australia. (2020). Why employers want to see micro-credentials on your resume. https://www.cpaaustralia.com.au/tools-and-resources/podcasts/career-skills/micro-credentials-on-your-resume
- CPA Australia. (2021). Surprising tech skills in demand for accounting and finance. https://intheblack.cpaaustralia.com.au/careers-and-workplace/tech-skills-in-demand-for-accounting-and-finance#:~:text = Employers%20across%20all%20industries%20are,skills%20offer%20a%20speed%20advantage
- CPA Australia. (2023). Professional accreditation guidelines. https://www.cpaaustralia.com.au/get-involved/academic-institution-support/professional-accreditation-guidelines
- Dai, J., & Vasarhelyi, M. (2023). Management accounting 4.0: The future of management accounting. Journal of Emerging Technologies in Accounting, 20(1), 1–13. https://doi.org/10.2308/JETA-2023-009
- Dalwai, T., Mohammadi, S.S., Chugh, G., Somerville, A., (2021). Big data analytics and accounting education: A systematic literature review. In A. l. Mawali, N. R. Al Lawati, & A.M. S. Ananda (Eds), Fourth Industrial Revolution and Business Dynamics. Palgrave Macmillan. https://doi.org/10.1007/978-981-16-3250-1_8.
- Davis, F. D. (1989). Perceived usefulness, perceived ease of use, and user acceptance of information technology. MIS Quarterly, 13(3), 319–340. https://doi.org/10.2307/249008
- Dilla, W., Janvrin, D. J., & Raschke, R. (2010). Interactive data visualization: New directions for accounting information systems research. Journal of Information Systems: Fall, 24(2), 1-37.
- Dillon, A., & Morris, M. (1996). User acceptance of information technology: Theories and models. Annual Review of Information Science and Technology, 31, 3–32.
- Dixon-Woods, M. (2011). Using framework-based synthesis for conducting reviews of qualitative studies. BMC Medicine, 9(1), 39–40. https://doi.org/10.1186/1741-7015-9-39.
- Dow, K. E., Jacknis, N. J., & Watson, M. W. (2021). A framework and resources to create a data analytics-infused accounting curriculum. Issues in Accounting Education, 36(4), 183–205.
- Drew, J. (2018). Merging accounting with ‘big data’ science. Journal of Accountancy, 226(1), 48–52.
- Dzuranin, A. C., Jones, J. R., & Olvera, R. M. (2018). Infusing data analytics into the accounting curriculum: A framework and insights from faculty. Journal of Accounting Education, 43, 24–39. https://doi.org/10.1016/j.jaccedu.2018.03.004
- Earley, C. (2015). Data analytics in auditing: Opportunities and challenges. Business Horizons, 58(2015), 493–500. https://doi.org/10.1016/j.bushor.2015.05.002
- Fathema, N., & Sutton, K. L. (2013). Factors influencing faculty members’ learning management systems adoption behavior: An analysis using the technology acceptance model. International Journal of Trends in Economics Management & Technology, 2(6), 20–28.
- Gough, D., Thomas, J., & Oliver, S. (2012). Clarifying differences between review designs and methods. Systematic Reviews, 28(1), 1–9. https://doi.org/10.1186/2046-4053-1-28
- Granić, A., & Marangunić, N. (2019). Technology acceptance model in educational context: A systematic literature review. British Journal of Educational Technology, 50(5), 2572–2593. https://doi.org/10.1111/bjet.12864
- Hair Jr, J. F., Matthews, L. M., Matthews, R. L., & Sarstedt, M. (2017). PLS-SEM or CB-SEM: Updated guidelines on which method to use. International Journal of Multivariate Data Analysis, 1(2), 107–123. https://doi.org/10.1504/IJMDA.2017.087624
- Hair, J. F., Risher, J. J., Sarstedt, M., & Ringle, C. M. (2019). When to use and how to report the results of PLS-SEM. European Business Review, 31(1), 2–24. https://doi.org/10.1108/EBR-11-2018-0203
- Hair, J. F., Sarstedt, M., Ringle, C. M., & Gudergan, S. P. (2018). Advanced issues in partial least squares structural equation modeling (PLS-SEM). Sage. https://doi.org/10.3926/oss.37.
- Henseler, J., Ringle, C. M., & Sarstedt, M. (2015). A new criterion for assessing discriminant validity in variance-based structural equation modeling. Journal of the Academy of Marketing Science, 43(1), 115–135. https://doi.org/10.1007/s11747-014-0403-8
- Hoelscher, J., & Mortimer, A. R. (2018). Using Tableau to visualize data and drive decision-making. Journal of Accounting Education, 44, 49–59.
- Howieson, B. (2003). Accounting practice in the new millennium: Is accounting education ready to meet the challenge? The British Accounting Review, 35(2), 69–103. https://doi.org/10.1016/S0890-8389(03)00004-0
- IBM. (2013). Descriptive, predictive, prescriptive: Transforming asset and facilities management with analytics. In: Thought Leadership White Paper, (October 2013).
- Jackson, D., Michelson, G., & Munir, R. (2023). Developing accountants for the future: New technology, skills, and the role of stakeholders. Accounting Education, https://doi.org/10.1080/09639284.2022.2057195
- Kogan, G., Myers, N., Gaydon D. J., Douglas, M. B. (2021). Strategic Finance. https://sfmagazine.com/post-entry/december-2021-advancing-digital-transformation/
- Koufaris, M. (2002). Applying the technology acceptance model and flow theory to online consumer behavior. Information Systems Research, 13(2), 205–223. https://doi.org/10.1287/isre.13.2.205.83
- Lakhal, S., & Khechine, H. (2021). Technological factors of students’ persistence in online courses in higher education: The moderating role of gender, age and prior online course experience. Education and Information Technologies, 26(3), 3347–3373. https://doi.org/10.1007/s10639-020-10407-w
- Lee, D. Y., & Lehto, M. R. (2013). User acceptance of YouTube for procedural learning: An extension of the Technology Acceptance Model. Computers & Education, 61(2013), 193–208. https://doi.org/10.1016/j.compedu.2012.10.001
- Lepenioti, K., Bousdekis, A., Apostolou, D., & Mentzas, G. (2020). Prescriptive analytics: Literature review and research challenges. International Journal of Information Management, 50(2019), 57–70. https://doi.org/10.1016/j.ijinfomgt.2019.04.003
- Losi, H. J., Isaacson, E. V., & Boyle, D. M. (2022). Integrating data analytics into the accounting curriculum: Faculty perceptions and insights. Issues in Accounting Education, 37(4), 1–23. https://doi.org/10.2308/ISSUES-2021-086
- Ma, Q., & Liu, L. (2004). The technology acceptance model. Journal of Organizational and End User Computing, 16(1), 59–72. https://doi.org/10.4018/joeuc.2004010104
- McAfee, A., & Brynjolfsson, E. (2012). Big data: The management revolution. Harvard Business Review, 90(10), 60–6, 68, 128.
- Mohd, F., Ahmad, F., Samsudin, N., & Sudin, S. (2011). Extending the technology acceptance model to account for social influence, trust and integration for pervasive computing environment: A case study in university industry. American Journal of Economics and Business Administration, 3(3), 552–559. https://doi.org/10.3844/ajebasp.2011.552.559
- Moll, J., & Yigitbasioglu, O. (2019). The role of internet-related technologies in shaping the work of accountants: New directions for accounting research. The British Accounting Review, 51(6), https://doi.org/10.1016/j.bar.2019.04.002
- Möller, K., Schäffer, U., & Verbeeten, F. (2020). Digitalization in management accounting and control: An editorial. Journal of Management Control, 31(1-2), 1–8. https://doi.org/10.1007/s00187-020-00300-5
- Moore, C., Rowe, B. J., & Widener, S. K. (2001). HCS: Designing a balanced scorecard in a knowledge-based firm. Issues in Accounting Education, 16(4), 569–601. https://doi.org/10.2308/iace.2001.16.4.569
- Mortenson, M. J., & Vidgen, R. (2016). A computational literature review of the technology acceptance model. International Journal of Information Management, 36(6), 1248–1259. https://doi.org/10.1016/j.ijinfomgt.2016.07.007.
- Nielsen, S. (2022). Management accounting and the concepts of exploratory data analysis and unsupervised machine learning: A literature study and future directions. Journal of Accounting & Organizational Change, 18(5), 811–853.
- Presley, T. J. (2019). A risk based approach to large datasets: Analysis of time series data for a large merchandising firm. Journal of Accounting Education, 49, 100639. https://doi.org/10.1016/j.jaccedu.2019.100639
- Prokofieva, M. (2021). Using dashboards and data visualizations in teaching accounting. Education and Information Technologies, 26(5), 5667–5683. https://doi.org/10.1007/s10639-021-10525-z
- Prokofieva, M. (2023). Integrating data analytics in teaching audit with machine learning and artificial intelligence. Education and Information Technologies, 28(6), 7317–7353. https://doi.org/10.1007/s10639-022-11474-x
- Richardson, V., Teeter, R., & Terrell, K. (2019). Data analytics for accounting. McGraw Hill Education.
- Richardson, V. J., & Shan, Y. (2019). Data analytics in the accounting curriculum. In T. G. Calderon (Ed.) Advances in Accounting Education: Teaching and Curriculum Innovations (Advances in Accounting Education, Vol. 23) (pp. 67–79). Emerald Publishing Limited.
- Rikhardsson, P., & Yigitbasioglu, O. (2018). Business intelligence & analytics in management accounting research: Status and future focus. International Journal of Accounting Information Systems, 29, 37–58. https://doi.org/10.1016/j.accinf.2018.03.001
- Sangster, A. (1992). Computer-based instruction in accounting education. Accounting Education, 1(1), 13–32. https://doi.org/10.1080/09639289200000003
- Sangster, A. (1995). The integration of expert systems within the accounting curriculum. Accounting Education, 4(3), 211–216. https://doi.org/10.1080/09639289500000025
- Shmueli, G., & Koppius, O. R. (2011). Predictive analytics in information systems research. MIS Quarterly, 35(3), 553–572. https://doi.org/10.2307/23042796
- Smith D. A. (2016). Online interactive thematic mapping: Applications and techniques for socio-economic research. Computers, Environment and Urban Systems, 57(2016), 106–117. https://doi.org/10.1016/j.compenvurbsys.2016.01.002.
- Stoner, G., Wootton, C. W., & Kemmerer, B. E. (2020). Mechanisation, computerisation and information systems. In J. R. Edwards, & S. P. Walker (Eds.), Routledge companion to accounting history (pp. 136–155). Routledge.
- Tadesse, A. F., & Vincent, N. E. (2022). Combining data analytics with XBRL: The viewdrive case. Issues in Accounting Education, 37(1), 197–215. https://doi.org/10.2308/ISSUES-2020-048
- Taherdoost, H. (2018). Development of an adoption model to assess user acceptance of e-service technology: E-Service Technology Acceptance Model. Behaviour & Information Technology, 37(2), 173–197. https://doi.org/10.1080/0144929X.2018.1427793
- Van der Heijden, H. (2023). Predicting industry sectors from financial statements: An illustration of machine learning in accounting research. The British Accounting Review, 54(5), 101096. https://doi.org/10.1016/j.bar.2022.101096
- Vasarhelyi, M. A., Kogan, A., & Tuttle, B. M. (2015). Big data in accounting: An overview. Accounting Horizons, 29(2), 381–396. https://doi.org/10.2308/acch-51071
- Venkatesh, V., & Davis, F. D. (2000). A theoretical extension of the technology acceptance model: Four longitudinal field studies. Management Science, 46(2), 186–204. https://doi.org/10.1287/mnsc.46.2.186.11926
- Verma, S., Bhattacharyya, S. S., & Kumar, S. (2018). An extension of the technology acceptance model in the big data analytics system implementation environment. Information Processing & Management, 54(54), 791–806. https://doi.org/10.1016/j.ipm.2018.01.004
- Vesty, G., & Brooks, A. (2017). St George Hospital: Flexible budgeting, volume variance, and balanced scorecard performance measurement. Issues in Accounting Education, 32(3), 103–116. https://doi.org/10.2308/iace-51588
- Yigitbasioglu, O., Green, P., & Cheung, M. Y. D. (2023). Digital transformation and accountants as advisors. Accounting, Auditing & Accountability Journal, 36(1), 209–237. https://doi.org/10.1108/AAAJ-02-2019-3894
Appendices
Appendix 1. The Questionnaire structure
Appendix 2. Sampled studies across identified themes
Appendix 3. Sample list of papers in the literature review (Phase 1)
Prokofieva, M. Integrating data analytics in teaching audit with machine learning and artificial intelligence. Educ Inf Technol (2022). https://doi.org/10.1007/s10639-022-11474-x
Rezaee Z., Dorestani A., Aliabadi S. (2018). Application of Time Series Analyses in Big Data: Practical, Research, and Education Implications. Journal of Emerging Technologies in Accounting 1 July 2018; 15 (1): 183–197. https://doi.org/10.2308/jeta-51967
Nielsen, S. (2022), Management accounting and the concepts of exploratory data analysis and unsupervised machine learning: a literature study and future directions, Journal of Accounting and Organizational Change, vol. 8, no. 5, pp. 811-853. https://doi.org/10.1108/JAOC-08-2020-0107
Pervan, I., i Dropulić, I. (2019). ‘The impact of integrated information systems on management accounting: Case of Croatia’, Management, 24(1), str. 21-38. https://doi.org/10.30924/mjcmi.24.1.2
Uwizeyemungu S., Bertrand J., Poba-Nzaou P. (2020) Patterns underlying required competencies for CPA professionals: a content and cluster analysis of job ads, Accounting Education, 29:2, 109-136, DOI: 10.1080/09639284.2020.1737157
De Castro, V., Sridharana, B., Watty, K., & Safari, M. (2020). The impact of learner engagement on performance outcomes: a longitudinal study in accounting education. Accounting and Finance, 61(1), 1639–1664. https://doi.org/10.1111/acfi.12640
Raschke R.L., Charron K.F.; Review of Data Analytic Teaching Cases, Have We Covered Enough?. Journal of Emerging Technologies in Accounting 1 September 2021; 18 (2): 247–255. https://doi.org/10.2308/JETA-2020-036
Libby T., Schwebke J.M., Goldwater P.M.; Using Data Analytics to Evaluate the Drivers of Revenue: An Introductory Case Study Using Microsoft Power Pivot and Power BI. Issues in Accounting Education 1 November 2022; 37 (4): 97–105. https://doi.org/10.2308/ISSUES-2021-057
Losi H.J., Isaacson E.V., Boyle D.M.; Integrating Data Analytics into the Accounting Curriculum: Faculty Perceptions and Insights. Issues in Accounting Education 1 November 2022; 37 (4): 1–23. https://doi.org/10.2308/ISSUES-2021-086
Vasarhelyi, M. A., Kogan, A., & Tuttle, B. M. (2015) “Big data in accounting: Anoverview”, Accounting Horizons, vol. 29(2): 381–396