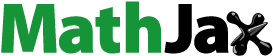
ABSTRACT
The tendency of falsely remembering events that did not happen in the past increases with age. This is particularly evident in cases in which features presented at study are re-presented at test in a recombined constellation (termed rearranged pairs). Interestingly, older adults also express high confidence in such false memories, a tendency that may indicate reduced metacognitive efficiency. Within an existing cohort study, we aimed at investigating age-related differences in memory metacognitive efficiency (as measured by meta d’ ratio) in a sample of 1522 older adults and 397 young adults. The analysis showed an age-related deficit in metacognition which was more pronounced for rearranged pairs than for new pairs. We then explored associations between cortical thickness and memory metacognitive efficiency for rearranged pairs in a subsample of 231 older adults. By using partial least square analysis, we found that a multivariate profile composed by ventromedial prefrontal cortex, insula, and parahippocampal cortex was uniquely associated with between-person differences in memory metacognitive efficiency. These results suggest that the impairment in memory metacognitive efficiency for false alarms is a distinct age-related deficit, above and beyond a general age-related decline in memory discrimination, and that it is associated with brain regions involved in metacognitive processes.
It is well known that episodic memory, the ability to remember past experiences, declines with age (Balota et al., Citation2000; Hoyer & Verhaeghen, Citation2006; Light, Citation1991; Rönnlund et al., Citation2005). Older adults have distinct problems when remembering the temporal, spatial, or contextual features related to experienced events (Spencer & Raz, Citation1995) and exhibit difficulties remembering associative information from different sources (Naveh-Benjamin, Citation2000), particularly when the tasks require explicit recognition (Dew & Giovanello, Citation2010).
Older adults are also more vulnerable to false memories, namely to incorrectly remember information that did not occur in the past (Devitt & Schacter, Citation2016; Fandakova et al., Citation2013, Citation2018; Jacoby & Rhodes, Citation2006; Kliegl & Lindenberger, Citation1993; Shing et al., Citation2009). In particular, older adults are more likely than young adults to incorrectly endorse as old pairs that consist of items they have seen before but had not been presented together (Fandakova et al., Citation2013, Citation2015; Shing et al., Citation2009). Although there is evidence that age effects on false memories are stronger than age effects on veridical memory (Bender et al., Citation2010; Old & Naveh-Benjamin, Citation2008), age-related differences on false memories have received comparably less attention. This is surprising, given that the ability to reject false memories is crucial in everyday life, for example to avoid becoming victims of fraudulent schemes which specifically target older adults (Jacoby & Rhodes, Citation2006).
Studies have also shown that older adults have a higher tendency to not only falsely remember events that did not occur, but also to express a higher subjective confidence that those events took place in the past and represent reality (Dodson & Krueger, Citation2006; Fandakova et al., Citation2013; Shing et al., Citation2009), a propensity which also increases for highly uncertain stimuli (Fandakova et al., Citation2018; Shing et al., Citation2009). These findings are indicative of a deficit in memory metacognitive sensitivity, the ability to distinguish one’s own correct vs incorrect judgments (Fleming & Lau, Citation2014), which is especially expressed when calibrating confidence in falsely remembered information.
As metacognitive sensitivity can be strongly affected by memory performance, it is still not clear whether the observed age-related decline in metacognitive sensitivity is due to a more general decline in memory. Previous studies have tried to decouple metacognitive accuracy from general memory performance using experimental manipulations to equate performance in young and older adults’ samples (e.g., Palmer et al., Citation2014). In the present study, we investigated whether an age-related deficit in memory metacognition is distinct from a general decline in memory by varying the mnemonic demands of task material and using a measure of metacognitive efficiency which is separable from basic memory performance (meta–d’/d’). In addition, we explored associations between preserved memory metacognitive efficiency and structural brain profiles.
Memory metacognition
Memory metacognition refers to the ability to introspectively monitor one’s memory accuracy (Chua et al., Citation2014) and it is important for guiding learning, decision-making, and social interactions (Flavell, Citation1979; Frith, Citation2012). To assess memory metacognition, researchers typically first ask subjects to make a decision on whether or not to-be-remembered material was encountered before, often called “type 1 task”, followed by confidence ratings about their memory accuracy on the current trial, which is called “type 2 task” (Galvin et al., Citation2003). The ability to distinguish one’s correct and incorrect performance at type 1 tasks, also referred to as metacognitive sensitivity (Fleming & Lau, Citation2014), requires tracking one’s objective behaviour on a trial-by-trial basis. A subject with high memory metacognitive sensitivity will show high confidence for correctly identified old objects (“hits”) or correctly rejected new objects (“correct rejections”) in a recognition test, and low confidence for incorrectly rejected old objects (“misses”) and incorrectly accepted new objects (“false alarms”). By contrast, a subject with poor memory metacognitive sensitivity will report confidence that is not in accordance with type 1 task performance. Although metacognitive sensitivity may be affected by type 1 task performance, it has been shown that it can vary between individuals when type 1 task performance has been kept constant, suggesting that it can be studied independently of basic cognitive capacity (see Fleming & Dolan, Citation2012, for a review). For this reason, it is crucial to study metacognitive sensitivity accounting for type 1 performance, a concept that is called metacognitive efficiency.
Memory metacognition in ageing
Studying memory metacognition independently from cognitive performance measures is especially important in ageing. Age-group differences in metacognitive abilities can be strongly affected by differences in cognitive performance (Daniels et al., Citation2009), and thus metacognitive accuracy needs to be disentangled from age-related declines in performance. A measure that estimates metacognitive efficiency without the confound of type 1 task performance is meta-d’/d’. This measure quantifies metacognitive ability under signal detection theory (Maniscalco & Lau, Citation2012), and estimates subjects’ awareness of their type 1 task performance (as reflected in confidence ratings) given a certain level of cognitive capacity d’.
Using meta-d’/d’, Palmer et al. (Citation2014) showed that perceptual metacognitive efficiency declines with age, while memory metacognitive efficiency remains stable. However, the task used by the authors was likely to place low demands on associative memory components, a condition in which metacognitive performance might be relatively preserved (Koen & Yonelinas, Citation2014). In addition, the authors adapted the level of difficulty of the task to participants by using a staircase procedure and varying the duration of the presentation of the to-be-remembered material. Although this manipulation may lead to a reduction in individual differences in type I performance, it is also likely to induce changes in the strategies that subjects use to encode the items. Therefore, it is currently unclear whether a measure of memory metacognitive efficiency controlling for individual differences in associative memory would reveal age-related differences in tasks in which older adults have been shown to produce higher false alarms (Naveh-Benjamin, Citation2000).
Memory metacognition may be more crucial in tasks that involve cognitive processes that are particularly sensitive to senescent decline. For example, Dodson and Krueger (Citation2006) showed that when memory was matched between young and older adults, older adults committed more high-confidence errors in a source-monitoring task, but not in a recognition memory task. Source memory tasks, and in general memory tasks that place substantial demands on strategic retrieval, might require additional monitoring processes to recognise whether the decision that has been made is correct or if an alternative course of action needs to be pursued. In fact, older adults show reduced metamemory monitoring particularly when the material presented at test is highly similar to the studied material (Fandakova et al., Citation2013). For example, in a task where at test the to-be-remembered materials are represented in a recombined order compared to the materials presented at study (rearranged pairs), older subjects might rely on the high familiarity elicited by the elements in the pairs, which might lead to false recognition of the pair and failure to recruit strategic monitoring processes, which in turn may result in deficits in metacognitive efficiency (Fandakova et al., Citation2013, Citation2018).
Brain structures supporting metamemory monitoring
There is convergent evidence showing that the neural basis of metacognitive efficiency can be traced back in prefrontal and parietal areas (McCurdy et al., Citation2013; Vaccaro & Fleming, Citation2018; White et al., Citation2014), suggesting the presence of a domain-general network supporting metacognitive abilities.
Among the frontal areas, the ventromedial prefrontal cortex (vmPFC) has been suggested to be involved in self-reflective processes, which also characterise theory of mind tasks (D’Argembeau et al., Citation2007; Jenkins & Mitchell, Citation2011). Therefore, it may be responsible for the production of a general evaluation of task performance, regardless of whether such performance originates from self or others (Nicolle et al., Citation2012). Other frontal areas that have been implicated in metacognitive efficiency are the dorsal anterior cingulated cortex (Baird et al., Citation2015) the inferior frontal gyrus (IFG), and posteromedial prefrontal cortex (pmPFC; Vaccaro & Fleming, Citation2018).
Frontal brain structures are also closely related to confidence judgements about memory (White et al., Citation2014). The propensity to commit more high-confidence false alarms in more demanding associative tasks observed in older adults has been related to dysfunctions of strategic retrieval mechanisms which rely on frontal brain structures (Gilboa et al., Citation2006). Individuals with orbitofrontal lobe lesions have been shown to perform well in memory tasks, but to be more likely than controls to falsely claim to have experienced events that did not happen in the past with high confidence (Schnider, Citation2003). Frontal lobe areas are also the brain areas that are subject to shrinkage in ageing (Raz et al., Citation2005, Citation2010), suggesting that age-related increases in high-confidence false alarms might be related to strategic and monitoring processes, which in turn rely on frontal regions.
Among the parietal areas, the lateral parietal cortex (LPC) has been generally linked to metacognition (Vaccaro & Fleming, Citation2018), while several findings show that the precuneus is specifically linked to memory metacognition (McCurdy et al., Citation2013; Ye et al., Citation2018). Moreover, lesion studies also showed that damage to the parietal cortex results in reduced feeling of confidence (Davidson et al., Citation2008) and diminished retrospective confidence ratings (Simons et al., Citation2010).
In addition to the fronto-parietal network, general metacognitive efficiency has also been found to rely on the insula, whereas the parahippocampal cortex is specifically implicated in retrospective metamemory judgments (Vaccaro & Fleming, Citation2018). The authors argued that the activation of the insula may reflect the contribution of error monitoring to the formation of confidence judgements, while the involvement of the parahippocampal gyrus may be linked to the importance of memory representations for metacognitive judgements (Vaccaro & Fleming, Citation2018).
Successful rejection of novel configurations, as for rearranged pairs, is also likely to involve structures of the medial temporal lobe, and in particular the hippocampus (HC), required to retrieve the previously encoded materials and detect mismatches between the currently presented material and the stored representation (Kumaran & Maguire, Citation2007). In particular, the CA1 region of the HC has been shown to track divergence between current and past sensory inputs (Chen et al., Citation2011) and has been related to associative memory performance (Carr et al., Citation2017). At the same time, the reduced recognition memory in individuals diagnosed with mild cognitive impairment and increased false alarm rates have been related to decreased volumes of the CA3-4/DG region (Bennett et al., Citation2019; Shing et al., Citation2011). These areas might contribute to memory metacognitive efficiency as they support the quality of the representation upon which metacognitive judgements are based.
The current study
In data from the Berlin Aging Study (BASE II, Bertram et al., Citation2014; Gerstorf et al., Citation2016), we examined memory metacognitive efficacy in an associative task. The study included a group of young and older adults and structural brain imaging. To assess memory metacognition, we used meta-d’/d’, a measure that controls for individual differences in memory performance, and, in this sense, is not biased against individuals with poor memory. Our first research aim was to investigate whether there was a specific impairment in memory metacognitive efficiency in older adults that was distinct from age-related impairments in memory.
We hypothesised that, if the presence of such distinct metacognitive deficit is revealed, then it should be higher for material that places more strategic demands (i.e., rearranged pairs), as monitoring mechanisms are more strongly recruited (Fandakova et al., Citation2013, Citation2018; Shing et al., Citation2009). Our second research aim was to identify distinct brain profiles of brain regions that are related to metamemory monitoring. We applied a multivariate statistical technique (partial least square correlation, PLSC) to link memory metacognition to a set of structural regions-of-interest (ROIs), including the ones indicated by previous studies and meta-analyses of metacognitive efficiency. In addition to areas specifically related to metacognitive functioning, we included hippocampal subfield regions, as they have been related to performance in associative memory tasks. If memory metacognitive deficits are more related to a decline in the quality of memory representations, we would expect to find associations between memory metacognitive efficiency and a brain profile where hippocampal subfields play a prominent role. In contrast, if ageing deficits in memory metacognition are related to distinct metacognitive processes, we would expect to reveal associations with a brain profile comprising cortical areas specifically related to metacognitive efficiency.
Materials and methods
Participants
Participants of this study were assessed within the baseline assessment of the BASE-II study cohort (Bertram et al., Citation2014; Gerstorf et al., Citation2016). The BASE-II study comprises 397 young adults (Mage = 28.32, SDage = 3.28, range = 21–46, 201 female) and 1522 older adults (Mage = 67.56, SDage = 3.58 range = 60–83, 772 female) who were volunteers from the Berlin metropolitan area (see for sample characteristics). The participants did not have any neurological or psychiatric disorder, or history of head injuries, and did not take medications that might affect memory functions. In addition, all participants reported normal or corrected-to-normal vision and scored over 27 on the Mini-Mental State Examination test (Folstein et al., Citation1975). The study was approved by the ethics section of the German Psychological Society (SK 012013_6). All participants provided informed consent in accordance with the Declaration of Helsinki.
Table 1. Sample characteristics by age group (young adults, older adults).
The behavioural analyses included in this study were carried out on the entire sample. Participants completed the behavioural assessment of the study on two separate initial appointments, and participants eligible for magnetic resonance imaging (MRI) were re-invited to participate in an MRI session within an average time interval of 3.2 months after completing the behavioural sessions. This MRI subsample consisted of 75 young adults (Mage = 27.50, SDage = 3.34, range = 21–36, 29 female) and 231 older adults (Mage = 67.31, SDage = 3.58, range = 60–80, 92 female). Overall, the behavioural and the MRI subsamples exhibited no significant differences in year of education (young adults: t(121) = 0.82, p = .414; older adults: t(80) = 0.24, p = .803) or digit symbol score (young adults: t(80) = 0.36, p = .718; older adults: t(80) = 0.36, p = .718). However, there was a significant gender distribution disparity within older adults’ subsamples, with males outnumbering females in the MRI subsample (χ2 = 12.80, p < .001), while there were no gender differences in the behavioural sample, χ2 = 2.95, p = .086. Furthermore, the MRI subsample of young adults was significantly younger than the behavioural subsample, t(82.6) = 2.19, p = .031, while there was no age difference between the behavioural and the MRI subsamples for older adults, t(256.2) = 1.05, p = .293. As we were interested in revealing a profile of brain regions related to metacognitive efficiency in late adulthood, we exclusively applied the PLSC to the older adult subsample.
Face-profession task
Materials
To test episodic memory, we used the face-profession task. For this task, 54 unique faces were selected from the CAL/PAL Face Database (Ebner et al., Citation2010; Minear & Park, Citation2004), and randomly associated with a profession ().
Figure 1. Schematic illustration of the task. The task was composed of two main phases separated by a short distractor task: the encoding phase and the recognition phase. In the encoding phase, participants were presented with images depicting real faces together with the names of professions. They had to decide whether or not the professions fitted the faces displayed. In the recognition face, participants were again presented with face-profession pairs. These pairs could be the same as the ones presented in the encoding face (target pairs) or could be presented in a recombined order (rearranged pairs). In addition, never-seen-before pairs were also presented (new pairs). Participants had to decide whether they had seen each pair before (“old”) or not (“new”). Additionally, for each pair they were asked to indicate their level of confidence on a scale from 1 (“not sure”) to 3 (“very sure”).
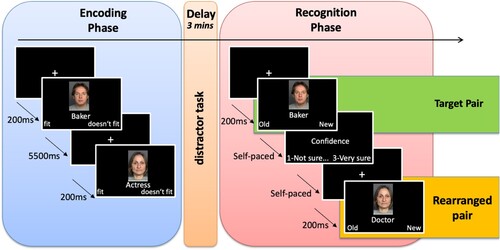
Each of the 45 face-profession pairs was shown once during the encoding task. Of the 45 face-profession pairs shown at encoding, 27 of them were randomly selected to be shown again during the recognition test (target pairs), while 18 of them were selected to reappear as recombined pairs (rearranged pairs). The rearranged pairs were constructed by pairing a face shown at encoding with a profession from a different pair. Both the target and rearranged pairs were unique, such that they were presented only once throughout the recognition test. Each face was also selected only once for recombination with a profession. Finally, nine face-profession pairs that were not shown at encoding and thus never seen by the participants before were used (new pairs). The order of the presentations of the target, rearranged, and new pairs was randomised within the recognition task.
Procedure
Participants were invited to two cognitive testing sessions scheduled 1 week apart and were tested in groups of 4–6 subjects on a comprehensive cognitive battery that covers key cognitive abilities measured by 21 tasks. Each session lasted about 3.5 h. The face-profession task was embedded in the first part of the first session. It was the third task of the session, following a visual memory task and a reading battery, both of which lasted approximately 55 min in total. During the encoding task, on each trial participants first saw a fixation cross for 200 ms, followed by a face-profession pair. In the face-procession pair, the face was displayed on top of the profession. The face-profession pairs were presented sequentially each for 3.5 s. The participants were asked to decide whether the profession fitted the face, by pressing a key for “fit” or “doesn’t fit” on a custom-made button box. Participants had up to 5.5 s from the pictures onset to make the decision. After going through the 45 face-profession pairs, participants were invited to perform a distractor task, at the end of which they were presented with a surprise recognition task.
In the recognition task, participants were presented with the target, rearranged, and new pairs. For each pair, they had to decide whether they had already seen it before, by selecting either “old” or “new”. After the old-new judgement, for each pair the participants indicated how confident they were about their decision, on a scale from 1, meaning “not sure”, to 3, meaning “very sure”. Both the old-new judgement and the confidence ratings were self-paced.
Measures
To analyse performance on the recognition task, we first calculated the amount of “hits”, “miss”, “correct rejections”, and “false alarms”. For each target pair, responding “old” was considered a “hit”, while responding “new” was considered a “miss”. For each rearranged and new material, responding “new” was categorised as a “correct rejection”, while responding “old” was considered a “false alarm”. Correct rejections and false alarms were considered separately for new and rearranged pairs. Hit rates were then calculated as the total number of hits divided by the sum of hits and misses; false alarm rates were calculated as the total number of false alarms divided by the sum of false alarms and correct rejections. False alarm rates were also considered separately as a function of the lure type (new vs rearranged pairs).
Memory metacognitive efficiency was measured using the ratio meta-d’/d’ (Fleming, Citation2017; Fleming & Dolan, Citation2012). It is well known that measures of metacognitive efficiency are biased by basic task performance. Accordingly, metacognitive ability in memory judgements, namely confidence in one’s own ability to correctly recognise or reject an object in a memory test, is dependent on basic memory abilities to distinguish old from new materials. For example, if a participant is performing at chance level on the recognition task, he/she would theoretically not be able to have distinguishable confidence levels for correct and incorrect trials, as the correct trials are correct only by chance. The meta-d’ measure allows to overcome these limitations.
Meta-d’ is the value of d’ performance (i.e., type 1 performance, memory sensitivity regardless of confidence ratings) that is predicted to give rise to the observed confidence ratings assuming an ideal observer with meta-d’ = d’. It can be compared to d’ performance to obtain a relative measure of metacognitive sensitivity, the ratio meta-d’/d’, which is a measure of metacognitive efficiency or capability, expressing the level of metacognition given a particular level of task performance.
We estimated the ratio meta-d’/d’ using hierarchical Bayesian estimation (Fleming, Citation2017). Hierarchical Bayesian estimation offers several advantages over single-subject estimations. Firstly, single-subject estimations of meta-d’ tend to be rather noisy; Bayesian estimation incorporates estimation uncertainty, ensuring that group-level parameters are not influenced by fits with a high degree of uncertainty. Secondly, Bayesian estimation allows to specify prior based on previous studies to weakly constrain parameter values and reduce the noise in the estimation Finally, in Bayesian estimation the group level parameter of interest is the ratio of meta-d’/d’ rather than meta-d’, allowing one to estimate metacognitive efficiency without being influenced by intergroup-level variability in d’.
We estimated the meta-d’/d’ separately for lure type (new vs rearranged pairs), and for young and older adults. As the estimation of meta-d’/d’ requires both new and old materials, we used the same set of individual-level confidence and accuracy data for old materials when estimating both new and rearranged pairs. The Hmeta-d package (Fleming, Citation2017) was used to estimate the parameters. To check convergence of the estimations, we inspected trace plots and examined scale-reduction statistic (Gelman & Rubin, Citation1992), where values smaller than 1.1 indicate convergence.
Data analysis
The preprocessing and data analysis of the behavioural data were performed in R (Version 4.2.1, R Core Team, 2022). For statistical analysis, we used linear mixed-effect models with the lmer function from the package lme4 (Bates et al., Citation2014). For the analysis of trial-level data, such as confidence ratings, participants were added as random intercepts, whereas the predictors of interest were added as both fixed effects and random slopes. Therefore, we used the maximal random structure justified by the design (Barr, Citation2013). For the analysis of aggregated, subject-level data (such as response rates and meta-d’/d’), only random effects of participants were modelled. Parameters were estimated through restricted maximum-likelihood estimation and the statistical significance of the parameters was tested through analysis of variance with Satterthwaite’s method. Effect sizes were calculated by taking the relative importance of specific predictors (semi-partial marginal R2) with the Nakagawa and Schielzeth approach (Jaeger et al., Citation2016).
Magnetic resonance imaging
Image acquisition
MRI data were acquired on a 3 T Siemens Magnetom Tim Trio scanner. For most participants, a 32-channel head coil was used; a 12-channel coil was used for two participants because the 32-channel coil was uncomfortable for them. MRI scanning data included at T1-weighted magnetization-prepared rapid gradient echo (MPRAGE) sequence, acquired in the sagittal plane with a single repetition time TR – 2500 ms, echo time TE = 4.77 ms, with an isotropic voxel size of 1.0 × 1.0 × 1.0, using a 3D distortion correction filter and pre-scan normalisation with FOV = 256, and generalised auto-calibrating partially parallel acquisition (GRAPPA) acceleration factor = 2. Acquisition also included a single T2-weighted, turbo spin echo high-resolution sequence in a coronal direction, which was oriented perpendicularly to the long axis of the left HC, with voxel size = 0.4 × 0.4 × 2.0 mm3, 30 slices, TR = 8150 ms, TE = 50 ms, and flip angle = 120°, positioned to cover the entire extent of the HC.
Cortical thickness measures
Cortical thickness measures were derived using FreeSurfer (FreeSurver version 7, Dale et al., Citation1999) and the Desikan-Killiany (Desikan et al., Citation2006) atlas. We identified ROIs depending on previous studies and reviews on memory metacognition (Fandakova et al., Citation2017; Fleming & Dolan, Citation2012; McCurdy et al., Citation2013; Vaccaro & Fleming, Citation2018). We thus selected the vmPFC, IFG, dorsolateral prefrontal cortex (dlPFC), vmPFC, and LPC. These ROI were obtained by aggregating the following areas from the Desikan-Killiany atlas: for the pmPFC, caudal-anterior cingulate and posterior-anterior cingulate; for the IFG, pars orbitalis, pars opercularis, and pars triangularis; for the dlPFC, rostral middle-frontal gyrus, causal middle-frontal gyrus, and superior-frontal gyrus; for the vmPFC, rostral-anterior cingulate, medial orbitofrontal gyrus; for the LP, supramarginal gyrus, superior-parietal and inferior-parietal gyri. In addition, the precuneus, the insula, and the parahippocampal cortex were added to the ROIs, as they have been indicated as crucial areas for memory metacognition and error processing (McCurdy et al., Citation2013; Vaccaro & Fleming, Citation2018). The ROIs from the left and right hemispheres were aggregated, as our hypotheses were not related to lateralisation.
HC subfields
To delineate the subregions of the HC, the Automated Segmentation of Hippocampal Subfields software (ASHS, Yushkevich et al., Citation2015) was used. ASHS uses a method based on multiple atlases to integrate segmentation data from several participants. The high-resolution turbo spin echo T2 scans of the HC were used. Three different HC subfields were identified: cornu ammoni regions 1 and 2 (CA1-2), CA3 and dentate gyrus (CA3-DG), and the entorhinal cortex (ERC). As brain volumes are influenced by head sizes, HC subfield volumes were adjusted for intracranial volumes using a covariance approach (Raz et al., Citation2005; Voevodskaya, Citation2014).
PLSC analysis
To investigate associations between our ROIs and metacognitive efficiency in memory, we used a multivariate PLSC approach (Keresztes et al., Citation2017; Krishnan et al., Citation2011). PLSC has several advantages over univariate methods. First, it is known that multivariate methods allow to find more robust associations between brain and behavioural methods (Marek et al., Citation2022). Second, PLSC overcomes the correlation inherent between brain anatomy measures, which leads to statistical multicollinearity and thus reduces statistical power to reveal brain-behaviour associations. Finally, PLSC is capable of handling large datasets, featuring many variables. Overall, PLSC is a powerful technique that we deemed ideal for our aim of linking metacognitive sensitivity in memory to multiple structural ROIs.
We used meta-d’/d’ as a measure of memory metacognitive efficiency and extracted a latent brain profile that maximised the common variance between meta-d’/d’ and our ROIs. This multivariate approach is considered to be better suited than an univariate assessment of relationships between single ROIs and behavioural measures because the ROIs are interconnected and interdependent (Genon et al., Citation2022).
PLSC looked for latent variables that maximised the amount of information that was common to the behavioural and brain measures, computing latent variables with maximal covariance. This approach is described in . First, the relationship between a matrix X containing participants’ measures of all ROIs and a matrix Y containing participants’ behavioural measures (meta-d’/d’ for new or for rearranged pairs) is stored in a cross-product matrix R. R is a matrix of correlations between the normalised values of X and Y, which is then decomposed through single values decomposition into a vector of saliences V. The saliences represent the brain measures that best characterise R. The original matrix X is finally projected onto the vector of saliences V to create the matrix of latent variables of X, Lx, which is called “brain scores”.
Figure 2. Schematic description of PLSC. looked for latent variables that maximised the amount of information that was common to the behavioural and brain measures, computing latent variables with maximal covariance. The relationship between a matrix X containing participants’ measures of all ROIs (e.g., pmFC and IFG) and a matrix Y containing participants’ behavioural measures (e.g., meta-d’/d’ for new or for rearranged pairs) is stored in a cross-product matrix R. R is a matrix of correlations between the normalised values of X and Y, which is then decomposed through single values decomposition (SVD) into a vector of saliences V, representing the brain measures that best characterise R. The original matrix X is finally projected onto the vector of saliences V to create the matrix of latent variables of X, Lx, which is called “brain scores”. Note: pmPFC – posteromedial prefrontal cortex; IFG – inferior frontal gyrus.
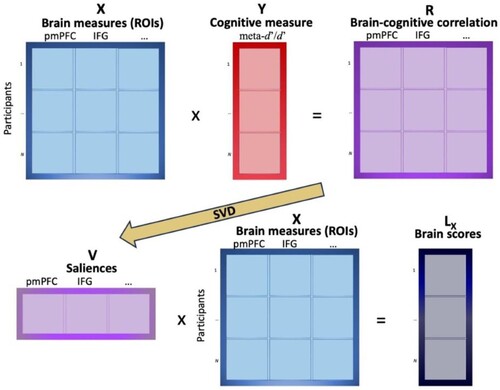
To obtain p-values and identify generalisable latent variables (brain scores), 5000 permutation tests were run (McIntosh & Lobaugh, Citation2004). After confirming that the latent variable was generalisable (p < .05), the next step was the identification of saliences that were stable throughout the permutations. This stability was obtained by dividing each element by its standard error (bootstrap ratio, BSR). The estimation of the standard error was then done by creating bootstrap samples through sampling with replacement of the observations in the two matrices. The BSRs are similar to Z-scores, and thus numbers larger than 1.96 (α < .05) correspond to significantly stable variables. These stable saliences represent the ROIs which show reliable associations to meta-d’/d’ measures.
The PLSC analyses were performed with the custom scripts using the statistics toolbox in Matlab (MATLAB Version: Citation9.Citation13.Citation0 (R2022b), Citation2022).
Results
Memory response rates and confidence
We first analysed response rates as a function of response type (Hits, FA/new, FA/rearranged). A plot of the observed values is shown in .
Figure 3. Response rates as a function of response type and age group. Note: YA – young adults; OA – older adults.

Analysis revealed a significant main effect of response type, F(2, 3834) = 5692.77, = 0.35, p < .001, with participants making higher hit rates for target pairs than both FA-rate for new (pcorr < .001) and rearranged lure pairs (pcorr < .001). Importantly, there was also an interaction between response type and age group, F(2, 3834) = 238.74,
= 0.04, showing that the difference between FA-rate/rearranged and FA-rate/new was significantly higher in older adults than the same difference in young adults, t(1, 1917) = 11.57, p < .001. This result showed that older adults commited higher false alarms in rearranged pairs in relation to new pairs, compared to their younger counterparts. Hit rate was also significantly higher in young adults, compared to older adults, t(1, 1917) = 5.07, p < .001.
We then analysed confidence ratings at recognition as a function of the stimulus type (new, rearranged, target), accuracy (correct vs incorrect), and age group (young adults vs older adults). A graph with the observed data is shown in . Results revealed a significant three-way interaction between age group, stimulus type, and accuracy, F(2, 1591.1) = 20.0, = .001, p < .001. Pairwise comparisons revealed that there was a significant difference in confidence ratings between correct and incorrect trials in both young and older adults for the target pairs (young adults: β = 0.54, SE = 0.02, z = 24.67, pcorr < .001; older adults: β = 0.32, SE = 0.01, z = 29.04, pcorr < .001) and for the new pairs (young adults: β = 1.11, SE = 0.07, z = 15.31, pcorr < .001; older adults: β = 0.49, SE = 0.02, z = 21.05, pcorr < .001). Importantly, for the rearranged pairs, the difference in confidence ratings was significant only for young adults, β = 0.50, SE = 0.02, z = 20.08, pcorr < .001, but not for older adults, β = 0.04, SE = 0.01, z = 3.36, pcorr = .050. These results suggest that older adults have considerably more difficulty to evaluate their memory judgement for rearranged pairs.
Meta-d’/d’
Visual inspection of MCMC trace plots for the estimated meta-d’/d’ indicated that convergence was achieved. In addition, the scale-reduction statistic was <1.1 for all participants. However, the inspection of the distribution of participant-level meta-d’/d’ values revealed the presence of outliers. Therefore, we removed participants with meta-d’/d’ below the 2.5th and above the 97.5th percentiles. This resulted in the exclusion of 194 participants (154 older adults) in the behavioural data sample, and 35 participants (24 older adults) in the brain data sample. The remaining behavioural analyses were thus conducted on a sample of 1377 older adults and 359 young adults.
shows a graph of meta-d’/d’ as a function of lure type and age group. Analysis revealed a main effect of age group, F(1, 1734) = 626.09, = .04, p < .001, with young adults showing higher meta-d’/d’ than older adults, (young adults: M = 0.945, SD = 0.13; older adults: M = 0.78, SD = 0.15), while there was no main effect of lure type, F(1, 1734) = 0.91,
= .001, p = .339. The interaction between lure type and age group was significant, F(1, 1734) = 156.26,
= .03, p < .001.
Figure 5. Meta-d’/d’ as a function of lure type and age group. Note: YA – young adults; OA – older adults.
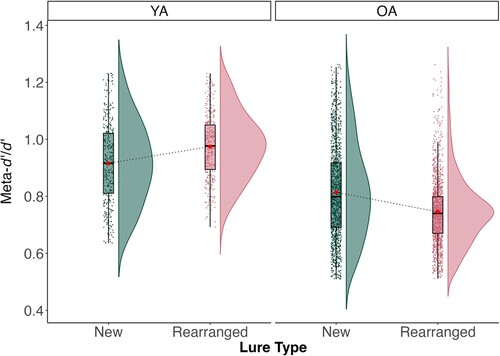
To break down the interaction, meta-d’/d’ values were analysed separately for young and older adults. In young adults, meta-d’/d’ were significantly higher for rearranged pairs (M = 0.97, SD = 0.11) than for new pairs (M = 0.92, SD = 0.11), F(1, 358) = 51.40, = .05, p < .001. Conversely, in older adults meta-d’/d’ were significantly higher for new pairs (M = 0.81, SD = 0.12) than for rearranged pairs (M = 0.74, SD = 0.12), F(1, 1376) = 208.97,
= .05, p < .001. These results show that the two age groups are characterised by opposite patterns: Whereas young adults showed higher metacognitive capabilities in evaluating their memory on rearranged pairs, compared to new ones, older adults present lower metacognitive capabilities for rearranged than for new pairs.
PLSC analysis
Before applying PLSC analysis to older adults’ data, one participant with cortical thickness measure at zero, indicating abnormal measurements, was excluded from further analyses. The final sample was then 207 older adults. We proceeded to analyse data using PLSC to extract brain profiles in structural brain measures that were related to Meta-d’/d’ (see ). The brain-behaviour pairings were estimated for older adults, for both rearranged and new pairs (see Supplementary Material for PLSC analysis in the young adults sample). For rearranged pairs, a stable latent variable reflecting the brain-behaviour association was identified, p = .011. This latent variable (“brain score”) represented the significant association between a unique profile of ROIs and the Meta-d’/d’ scores (r = 0.23, p = .001). Within the multivariate profile, we then identified the specific ROIs that expressed consistent salience using bootstrap ratio (BSR), and thus showed association with Meta-d’/d’. The ROIs identified were all cortical thickness measures: vmPFC (BSR = 2.88), precuneus (BSR = 3.08), insula (BSR = 3.00), and parahippocampal cortex (BSR = 2.16). These stable saliences of the latent variables represent the shared variance common to Meta-d’/d’ measures and the multivariate pattern of ROIs in cortical thickness structures.
Figure 6. Results of PLSC analyses for Meta-d’/d’. Multivariate brain profile associated with metacognitive efficiency for rearranged pairs (top-left) and new pairs (bottom-left). The bars represent the stability of the saliencies (z-score), and the dotted red lines represent the cut-off values used to evaluate the reliability of the saliencies (± 1.96). Top-left and bottom-left show the correlations between brain scores and age and brain score and meta-d’/d’ for rearranged and new pairs, respectively. Note: pmPFC – posteromedial prefrontal cortex; IFG – inferior frontal gyrus; dlPFC – dorsolateral prefrontal cortex; vmPFC-ventromedial prefrontal cortex; LPC – lateral parietal; parHCgyrus – parahippocampal gyrus; HC sub – subiculum; HC ERC – entorhinal cortex.
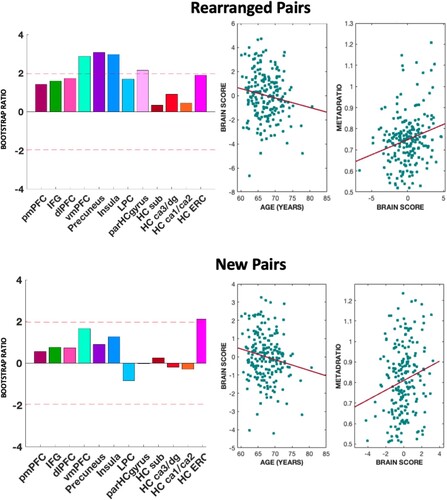
The composite “brain score” variable was positively associated with Meta-d’/d’, r = .22, p = .001, showing that the thicker the structures of the multivariate profile of brain structures identified, the better the memory metacognitive efficiency. The “brain score” measure was also negatively correlated with age, r = −0.17, p = .017, showing that the identified multivariate pattern of brain structures undergoes cortical thinning throughout late adulthood.
The multivariate profile for new pairs expressed consistent salience only for the ERC (BSR = 2.10) and was positively correlated with Meta-d’/d, r = −0.18 p = .009, and negatively correlated with age, r = −0.16 p = .017. However, the estimation of the cross-correlational matrix for new pairs did not express a stable latent variable, p = .439.
We additionally ran the PLSC analysis using d’ instead of meta-d’/d’ as behavioural measure, with our areas of interest as brain measures. Results are shown in . For rearranged pairs, the stability of the latent score was marginally significant (p = .077). The multivariate profile was positively correlated with d’, r = 0.22 p = .001, and negatively correlated with age, r = −0.21 p = .002. The ROIs identified were: IFG (BSR = 2.24), insula (BSR = 2.80), and ERC volume (BSR = 2.48). For new pairs, the stability of the latent score was also marginally significant (p = .059). The multivariate profile was positively correlated with d’, r = 0.19 p = .004, and negatively correlated with age, r = −0.18 p = .009. The ROIs that expressed consistent salience were LPC (BSR = 2.47) and the CA1/CA2 subregions of the HC (BSR = 2.64).
Figure 7. Results of PLSC analyses for d’. Multivariate brain profile associated with metacognitive efficiency for rearranged pairs (top-left) and new pairs (bottom-left). The bars represent the stability of the saliencies (z-score), and the dotted red lines represent the cut-off values used to evaluate the reliability of the saliencies (± 1.96). Top-left and bottom-left show the correlations between brain scores and age and brain score and meta-d’/d’ for rearranged and new pairs, respectively. Note: pmPFC – posteromedial prefrontal cortex; IFG – inferior frontal gyrus; dlPFC – dorsolateral prefrontal cortex; vmPFC-ventromedial prefrontal cortex; LPC – lateral parietal; parHCgyrus – parahippocampal gyrus; HC sub – subiculum; HC ERC – entorhinal cortex.

These results show that the PLSC run with d’ for rearranged and new pairs produced a multivariate profile which is distinct from the one found running the same analysis with Meta-d’/d’.
Additional analyses
We investigated whether the pattern of confidence ratings was due to a general tendency to respond with high confidence for the older adult sample. This overall level of metacognitive bias can be captured by a “meta-c” index (e.g., Legrand et al., Citation2021), which corresponds to the average level of confidence. We thus analysed confidence ratings as a function of age group (young vs older adults) and lure type (new vs rearranged pairs), regardless of accuracy. Results are shown in . There was a main effect of age group, F(1, 1916.9) = 74.2, = .001, p < .001, with older adults showing lower confidence overall, compared to their younger counterparts (β = −0.21, SE = 0.02, t = −8.61, pcorr < .001). Lure type was also significant, F(1, 1916.9) = 383.8,
= .001, p < .001, with lower confidence for rearranged vs new pairs (β = −0.20, SE = 0.01, t = −19.59, pcorr < .001). The interaction between age group and lure type also reached significance, F(1, 1916.9) = 1.4,
= .000, p = .047. This interaction indicated that the age group difference between confidence ratings for rearranged pairs was significantly smaller than the age group difference between confidence ratings for new pairs (β = 0.04, SE = 0.02, t = 1.99, pcorr < .001). These findings suggest that older adults might be biased towards responding with less confidence overall.
Discussion
The current study was motivated by two main research questions. First, we investigated whether the age-related memory metacognitive deficits reported by previous studies can be considered distinct from a more general age-related memory deficit. Second, we examined whether individual differences in memory metacognitive efficiency in the older adults’ sample were related to a certain brain structural profile which included cortical areas and hippocampal subfields. We used a task in which participants encoded trial-unique face-profession pairs. At a subsequent recognition task, participants were then presented again with the same pairs previously encoded (intact pairs), together with pairs composed of previously encoded items but in a recombined order (rearranged pairs), as well as completely new pairs. Using a measure that controls for the influence of task performance and response bias, we found that while young adults showed higher metacognitive efficiency for rearranged pairs, compared to new pairs, older adults had significantly lower metacognitive efficiency for rearranged pairs, compared to new pairs. In addition, we found that a multivariate brain profile composed of vmPFC, insula, precuneus, and parahippocampal cortex was uniquely associated with memory metacognitive efficiency for rearranged pairs in the group of older adults.
Our result revealing that older adults commit more false alarms, compared to their younger counterparts, is in line with previous studies suggesting that older adults are more prone to falsely remember events which did not occur in the past (Devitt & Schacter, Citation2016; Fandakova et al., Citation2013, Citation2018; Jacoby & Rhodes, Citation2006; Shing et al., Citation2009). In addition, our results also showed that older adults are particularly impaired in rejecting rearranged pairs, replicating previous similar findings (Fandakova et al., Citation2018) and consistent with a recall-to-reject deficit in adulthood (Gallo et al., Citation2006). In order to reject a rearranged pair, older adults need to retrieve the memory trace formed by the old item and its original associates. As older adults have specific deficits in binding together different sources of information (Naveh-Benjamin, Citation2000), the retrieval of the original pairs is more likely to fail. In the absence of the recollection of the exact pairs, elderly participants might rely on the familiarity signal elicited by the old items of the rearranged pairs and falsely consider the pairs as old.
Our results also showed that when older adults falsely remembered rearranged pairs, they reported similar levels of confidence as when they correctly rejected them. This finding is in line with previous studies showing that older adults are more likely to commit high-confidence false alarms (Dodson & Krueger, Citation2006; Fandakova et al., Citation2013; Shing et al., Citation2009). It suggests that older adults may rely on the familiarity signal that is elicited by items of the rearranged pairs when making confidence judgments, regardless of whether the original pairs of items were recollected or not. The reliance on familiarity signals to make a confidence decision may also result from the lower capacity to recur to recall-to-reject strategies.
Young adults intriguingly showed higher memory metacognitive efficiency for rearranged pairs, compared to new pairs, when type 1 memory performance is controlled for. This result is in line with the idea that recall-to-reject mechanisms are required to correctly reject rearranged pairs. In fact, while for rejecting a pair in which none of the two items was previously shown participants can rely on a familiarity signal, for rejecting a pair in which both the items were previously shown but in a different order participants have to recollect at least one of the item’s old pair-associate. Such more detailed retrieval supporting decisions on rearranged pairs might have resulted in the recruitment of additional monitoring processing (Cabeza et al., Citation2001; Fandakova et al., Citation2018), which underlies young adults’ abilities to calibrate their confidence ratings to performance.
Older adults showed worse memory metacognition efficiency for rearranged pairs, compared to new pairs, suggesting that they fail to recruit the additional monitoring processes needed to efficiently evaluate whether they have been successful in rejecting the recombined material. This result is thus consistent with previous studies showing reduced monitoring of memory outcomes in late adulthood (e.g., Fandakova et al., Citation2013).
The cortical regions identified within the brain profile related to memory metacognitive efficiency in rearranged pairs in older adults have already been indicated in the literature as part of a metacognitive network (Vaccaro & Fleming, Citation2018; White et al., Citation2014). The vmPFC, which included the rostral-anterior cingulate and the medial orbitofrontal gyrus, has also been implicated in self-reflective processes (D’Argembeau et al., Citation2007) and in retrospective judgements of performance. The cingulate cortex, together with the insula, has been also shown to be more active in the correct rejection of highly uncertain stimuli (Fandakova et al., Citation2018). The insula in particular has been related to error processing and error awareness (Bonini et al., Citation2014; Ullsperger et al., Citation2010). Taken together, our analysis showing the importance of cortical thickness in these areas for memory metacognitive efficiency in rearranged pairs suggests that these areas are recruited when participants subjectively evaluate their own errors.
The brain profile identified also included the precuneus, a finding that is consistent with previous studies providing evidence for the implication of the medial parietal cortex in memory metacognition, especially for retrospective memory judgements (McCurdy et al., Citation2013; Simons et al., Citation2010; Ye et al., Citation2018). The praecuneus might hold representations of highly vivid self-related material (Sreekumar et al., Citation2018), and thus it may be involved in the production of the subjective experience of recollection of highly detailed material. Parietal cortex damage has been related to reduced confidence and decreased richness of recollected experience (Davidson et al., Citation2008), suggesting that this area might be important for evaluating the detailedness of memories.
Finally, the brain profile identified included the thickness of the parahippocampal cortex, but not the volume of the hippocampal subfields. While the involvement of this area reflects the contribution of mnemonic representations to metacognitive efficiency, its preferential involvement can be explained by the specific task used. Correctly rejecting rearranged pairs requires the retrieval of context information associated with the to-be-remembered materials, a memory process for which the parahippocampal cortex might be more important than the HC proper (Aminoff et al., Citation2013; Kirwan & Stark, Citation2004; Ranganath & Ritchey, Citation2012). The preservation of cortical thickness in this area may be crucial for the retrieval of relevant mnemonic details, which is especially required when rejecting rearranged pairs. However, the parahippocampal gyrus has also been indicated as one of the areas which are specifically related to memory metacognition (Vaccaro & Fleming, Citation2018), and thus it may be implicated in general metamemory monitoring processes, regardless of the mnemonic task.
Our findings showing the identification of a multivariate brain profile through PLSC analysis suggest that the different areas that it comprises may be densely interconnected and work in concert to produce individual differences in memory metacognitive efficiency. The observed profile composed of parahippocampal gyrus, insula, precuneus, and vmPFC may support memory metacognitive efficiency by integrating the specific contribution of each area. The parahippocampal gyrus has previously been identified as part of a posterior-medial network (including precuneus) responsible for conscious recollection of contextual episodic memories (Ranganath & Ritchey, Citation2012; Ritchey & Cooper, Citation2020). The successful retrieval of a distinct pair may thus rely on this area, which provides the “memory evidence” upon which the metacognitive judgements are based. Conversely, the detection of high uncertainty, potentially through insula and vmPFC, which arises in trials characterised by high mnemonic demands may call for the recruitment of additional frontal and parietal areas for introspection and confidence calibration.
It is important to note that the areas identified were related to variability in cortical thickness which reflects individual differences in memory metacognitive performance in older adults. Accordingly, we cannot conclude that these areas are functionally involved when subjects are evaluating their memory judgments. Future studies using functional neuroimaging techniques are needed to test whether meta-d’/d’ reflecting memory metacognitive capacity is related to the activation of the brain profile that we identified.
The finding showing a more pronounced age-related deficit in metacognitive efficiency for rearranged pairs suggests a decline in strategic monitoring processes. Accordingly, executive functions impairments, which are also common in ageing and related to grey matter loss in prefrontal cortex (Boutzoukas et al., Citation2021) may also have driven the metacognitive deficits observed in memory in the current study. Future studies may use a battery of executive tasks to investigate which executive function may be specifically related to age-related declines in memory metacognitive efficiency.
Although the meta-d’/d’ accounts for differences in basic memory performance, certain cognitive factors may remain unaccounted for by this statistical measure. For instance, meta-d’/d’ may overlook the cognitive resources that participants employ to achieve their performance. This limitation hinders our ability to conclusively differentiate the age-related metacognitive deficit observed from a general memory deficit. An alternative approach to equate memory performance could involve adjusting the difficulty of the memory task based on participants’ memory abilities, for example, by varying the number of pairs presented depending on performance on a separate recognition task. However, this staircase approach might lead to variations in the strategies that individuals use to memorise the items.
Another limitation of the study emerges from the different number of new and rearranged pairs presented to participants at retrieval. Specifically, the number of new pairs included in the recognition pairs was smaller compared to the number of rearranged pairs (18 and nine, respectively). Consequently, rejecting new pairs might have been easier compared to rejecting rearranged pairs. Given that successful rejection of new pairs relies on familiarity, a cognitive process that is considered to be relatively preserved in older adults (Koen & Yonelinas, Citation2014), the disparity between new and rearranged pairs at recognition might have increased the difference in performance between these two conditions for older adults, compared to young adults.
The administration of the task after two cognitive tasks, specifically a visual memory task and a reading battery, might have influenced the age-related differences observed in this study. Participants engaged in these two cognitive tasks for approximately one hour before commencing the face profession task, potentially impacting the memory and metacognitive performances of older adults to a greater extent than those of younger adults.
The high confidence false alarm rates observed in older adults may have been driven by older adults’ general inclination to respond with higher confidence. This propensity might have also been influenced by the narrow range of the confidence scale (1–3), which could have reduced participants’ ability to differentiate between varying levels of confidence. However, our analysis of average confidence ratings across lure types, irrespective of accuracy, showed that this was not the case. If anything, older adults showed a tendency towards responding with lower confidence levels. This finding may explain the reduced overall confidence for correct rejections but fails to account for the high confidence ratings observed for rearranged pairs.
In conclusion, with the current study, we provided evidence for an age-related decline in memory metacognitive efficiency which is more substantial when the task places heavy demands on associative memory components. This deficit appears to be distinct from a general decline in memory. Additionally, we identified a brain profile that was associated with memory metacognitive efficiency in older adults, showing that the maintenance of memory metacognitive efficiency in late adulthood is related to the preservation of the structure of distinct brain areas.
Supplemental Material
Download MS Word (194.6 KB)Data availability statement
The data that support the findings of this study are available from the corresponding author, FP, upon reasonable request.
Disclosure statement
No potential conflict of interest was reported by the author(s).
Additional information
Funding
References
- Aminoff, E. M., Kveraga, K., & Bar, M. (2013). The role of the parahippocampal cortex in cognition. Trends in Cognitive Sciences, 17(8), 379–390. https://doi.org/10.1016/j.tics.2013.06.009
- Baird, B., Cieslak, M., Smallwood, J., Grafton, S. T., & Schooler, J. W. (2015). Regional white matter variation associated with domain-specific metacognitive accuracy. Journal of Cognitive Neuroscience, 27(3), 440–452. https://doi.org/10.1162/jocn_a_00741
- Balota, D. A., Dolan, P. O., & Duchek, J. M. (2000). Memory changes in healthy young and older adults. In The Oxford handbook of memory, The Oxford (pp. 395–409). http://www.psych.wustl.edu/coglab/publications/BalotaDolanDuchekMemchapter2000.pdf
- Barr, D. J. (2013). Random effects structure for testing interactions in linear mixed-effects models. Frontiers in Psychology, 4, 328.
- Bates, D., Mächler, M., Bolker, B., & Walker, S. (2014). Fitting linear mixed-effects models using lme4. ArXiv Preprint ArXiv:1406.5823.
- Bender, A. R., Naveh-Benjamin, M., & Raz, N. (2010). Associative deficit in recognition memory in a lifespan sample of healthy adults. Psychology and Aging, 25(4), 940–948. https://doi.org/10.1037/a0020595
- Bennett, I. J., Stark, S. M., & Stark, C. E. L. (2019). Recognition memory dysfunction relates to hippocampal subfield volume: A study of cognitively normal and mildly impaired older adults. The Journals of Gerontology: Series B, 74(7), 1132–1141. https://doi.org/10.1093/geronb/gbx181
- Bertram, L., Böckenhoff, A., Demuth, I., Düzel, S., Eckardt, R., Li, S. C., Lindenberger, U., Pawelec, G., Siedler, T., Wagner, G. G., & Steinhagen-Thiessen, E. (2014). Cohort profile: The Berlin Aging Study II (BASE-II). International Journal of Epidemiology, 43(3), 703–712. https://doi.org/10.1093/ije/dyt018
- Bonini, F., Burle, B., Lieǵeois-Chauvel, C., Reǵis, J., Chauvel, P., & Vidal, F. (2014). Action monitoring and medial frontal cortex: Leading role of supplementary motor area. Science (New York, N.Y.), 343(6173), 888–891. https://doi.org/10.1126/science.1247412
- Boutzoukas, E. M., O’Shea, A., Albizu, A., Evangelista, N. D., Hausman, H. K., Kraft, J. N., Van Etten, E. J., Bharadwaj, P. K., Smith, S. G., Song, H., Porges, E. C., Hishaw, A., DeKosky, S. T., Wu, S. S., Marsiske, M., Alexander, G. E., Cohen, R., & Woods, A. J. (2021). Frontal white matter hyperintensities and executive functioning performance in older adults. Frontiers in Aging Neuroscience, 13, 672535. https://doi.org/10.3389/fnagi.2021.672535
- Cabeza, R., Rao, S. M., Wagner, A. D., Mayer, A. R., & Schacter, D. L. (2001). Can medial temporal lobe regions distinguish true from false? An event-related functional MRI study of veridical and illusory recognition memory. Proceedings of the National Academy of Sciences, 98(8), 4805–4810. https://doi.org/10.1073/pnas.081082698
- Carr, V. A., Bernstein, J. D., Favila, S. E., Rutt, B. K., Kerchner, G. A., & Wagner, A. D. (2017). Individual differences in associative memory among older adults explained by hippocampal subfield structure and function. Proceedings of the National Academy of Sciences, 114(45), 12075–12080. https://doi.org/10.1073/pnas.1713308114
- Chen, J., Olsen, R. K., Preston, A. R., Glover, G. H., & Wagner, A. D. (2011). Associative retrieval processes in the human medial temporal lobe: Hippocampal retrieval success and CA1 mismatch detection. Learning & Memory, 18(8), 523–528. https://doi.org/10.1101/lm.2135211
- Chua, E. F., Pergolizzi, D., & Weintraub, R. R. (2014). The cognitive neuroscience of metamemory monitoring: Understanding metamemory processes, subjective levels expressed, and metacognitive accuracy. The Cognitive Neuroscience of Metacognition, 9783642451904, 267–291. https://doi.org/10.1007/978-3-642-45190-4_12
- Dale, A. M., Fischl, B., & Sereno, M. I. (1999). Cortical surface-based analysis: I. Segmentation and surface reconstruction. NeuroImage, 9(2), 179–194. https://doi.org/10.1006/nimg.1998.0395
- Daniels, K. A., Toth, J. P., & Hertzog, C. (2009). Aging and recollection in the accuracy of judgments of learning. Psychology and Aging, 24(2), 494–500. https://doi.org/10.1037/a0015269
- D’Argembeau, A., Ruby, P., Collette, F., Degueldre, C., Balteau, E., Luxen, A., Maquet, P., & Salmon, E. (2007). Distinct regions of the medial prefrontal cortex are associated with self-referential processing and perspective taking. Journal of Cognitive Neuroscience, 19(6), 935–944. https://doi.org/10.1162/jocn.2007.19.6.935
- Davidson, P. S. R., Anaki, D., Ciaramelli, E., Cohn, M., Kim, A. S. N., Murphy, K. J., Troyer, A. K., Moscovitch, M., & Levine, B. (2008). Does lateral parietal cortex support episodic memory? Evidence from focal lesion patients. Neuropsychologia, 46(7), 1743–1755. https://doi.org/10.1016/j.neuropsychologia.2008.01.011
- Desikan, R. S., Ségonne, F., Fischl, B., Quinn, B. T., Dickerson, B. C., Blacker, D., Buckner, R. L., Dale, A. M., Maguire, R. P., Hyman, B. T., Albert, M. S., & Killiany, R. J. (2006). An automated labeling system for subdividing the human cerebral cortex on MRI scans into gyral based regions of interest. NeuroImage, 31(3), 968–980. https://doi.org/10.1016/j.neuroimage.2006.01.021
- Devitt, A. L., & Schacter, D. L. (2016). False memories with age: Neural and cognitive underpinnings. Neuropsychologia, 91, 346–359. https://doi.org/10.1016/j.neuropsychologia.2016.08.030
- Dew, I. T. Z., & Giovanello, K. S. (2010). Differential age effects for implicit and explicit conceptual associative memory. Psychology and Aging, 25(4), 911–921. https://doi.org/10.1037/a0019940
- Dodson, C. S., & Krueger, L. E. (2006). I misremember it well: Why older adults are unreliable eyewitnesses. Psychonomic Bulletin & Review, 13(5), 770–775. https://doi.org/10.3758/BF03193995
- Ebner, N. C., Riediger, M., & Lindenberger, U. (2010). FACES—A database of facial expressions in young, middle-aged, and older women and men: Development and validation. Behavior Research Methods, 42(1), 351–362. https://doi.org/10.3758/BRM.42.1.351
- Fandakova, Y., Lindenberger, U., & Shing, Y. L. (2015). Maintenance of youth-like processing protects against false memory in later adulthood. Neurobiology of Aging, 36(2), 933–941. https://doi.org/10.1016/j.neurobiolaging.2014.10.022
- Fandakova, Y., Sander, M. C., Grandy, T. H., Cabeza, R., Werkle-Bergner, M., & Shing, Y. L. (2018). Age differences in false memory: The importance of retrieval monitoring processes and their modulation by memory quality. Psychology and Aging, 33(1), 119–133. https://doi.org/10.1037/pag0000212
- Fandakova, Y., Selmeczy, D., Leckey, S., Grimm, K. J., Wendelken, C., Bunge, S. A., & Ghetti, S. (2017). Changes in ventromedial prefrontal and insular cortex support the development of metamemory from childhood into adolescence. Proceedings of the National Academy of Sciences, 114(29), 7582–7587. https://doi.org/10.1073/pnas.1703079114
- Fandakova, Y., Shing, Y. L., & Lindenberger, U. (2013). High-confidence memory errors in old age: The roles of monitoring and binding processes. Memory (Hove, England), 21(6), 732–750. https://doi.org/10.1080/09658211.2012.756038
- Flavell, J. H. (1979). Metacognition and cognitive monitoring: A new area of cognitive-developmental inquiry. American Psychologist, 34(10), 906–911. https://doi.org/10.1037/0003-066X.34.10.906
- Fleming, S. M. (2017). HMeta-d: Hierarchical Bayesian estimation of metacognitive efficiency from confidence ratings. Neuroscience of Consciousness, 2017(1), https://doi.org/10.1093/nc/nix007
- Fleming, S. M., & Dolan, R. J. (2012). The neural basis of metacognitive ability. Philosophical Transactions of the Royal Society B: Biological Sciences, 367(1594), 1338–1349. https://doi.org/10.1098/rstb.2011.0417
- Fleming, S. M., & Lau, H. C. (2014). How to measure metacognition. Frontiers in Human Neuroscience, 8, 443. https://doi.org/10.3389/fnhum.2014.00443
- Folstein, M. F., Folstein, S. E., & McHugh, P. R. (1975). “Mini-mental state”: a practical method for grading the cognitive state of patients for the clinician. Journal of Psychiatric Research, 12(3), 189–198. https://doi.org/10.1016/0022-3956(75)90026-6
- Frith, C. D. (2012). The role of metacognition in human social interactions. Philosophical Transactions of the Royal Society B: Biological Sciences, 367(1599), 2213–2223. https://doi.org/10.1098/rstb.2012.0123
- Gallo, D., Bell, D., Beier, J., & Schacter, D. (2006). Two types of recollection-based monitoring in younger and older adults: Recall-to-reject and the distinctiveness heuristic. Memory (Hove, England), 14(6), 730–741. https://doi.org/10.1080/09658210600648506
- Galvin, S. J., Podd, J. V., Drga, V., & Whitmore, J. (2003). Type 2 tasks in the theory of signal detectability: Discrimination between correct and incorrect decisions. Psychonomic Bulletin & Review, 10(4), 843–876. https://doi.org/10.3758/BF03196546
- Gelman, A., & Rubin, D. B. (1992). Inference from iterative simulation using multiple sequences. Statistical Science, 7(4), 457–472. https://doi.org/10.1214/SS/1177011136
- Genon, S., Eickhoff, S. B., & Kharabian, S. (2022). Linking interindividual variability in brain structure to behaviour. Nature Reviews Neuroscience, 23(5), 307–318. https://doi.org/10.1038/s41583-022-00584-7
- Gerstorf, D., Bertram, L., Lindenberger, U., Pawelec, G., Demuth, I., Steinhagen-Thiessen, E., & Wagner, G. G.. (2016). Editorial. Gerontology, 62(3), 311–315. https://doi.org/10.1159/000441495Editorial.
- Gilboa, A., Alain, C., Stuss, D. T., Melo, B., Miller, S., & Moscovitch, M. (2006). Mechanisms of spontaneous confabulations: A strategic retrieval account. Brain, 129(6), 1399–1414. https://doi.org/10.1093/brain/awl093
- Hoyer, W. J., & Verhaeghen, P. (2006). Memory aging. In Handbook of the psychology of aging, 209–232. https://doi.org/10.1016/B978-012101264-9/50013-6
- Jacoby, L. L., & Rhodes, M. G. (2006). False remembering in the aged. Current Directions in Psychological Science, 15(2), 49–53. https://doi.org/10.1111/j.0963-7214.2006.00405.x
- Jaeger, B. C., Edwards, L. J., Das, K., & Sen, P. K. (2016). An R2 statistic for fixed effects in the generalized linear mixed model. Journal of Applied Statistics, 44(6), 1086–1105. https://doi.org/10.1080/02664763.2016.1193725
- Jenkins, A. C., & Mitchell, J. P. (2011). Medial prefrontal cortex subserves diverse forms of self-reflection. Social Neuroscience, 6(3), 211–218. https://doi.org/10.1080/17470919.2010.507948
- Keresztes, A., Bender, A. R., Bodammer, N. C., Lindenberger, U., Shing, Y. L., & Werkle-Bergner, M. (2017). Hippocampal maturity promotes memory distinctiveness in childhood and adolescence. Proceedings of the National Academy of Sciences, 114(34), 9212–9217. https://doi.org/10.1073/pnas.1710654114
- Kirwan, C. B., & Stark, C. E. L. (2004). Medial temporal lobe activation during encoding and retrieval of novel face-name pairs. Hippocampus, 14(7), 919–930. https://doi.org/10.1002/hipo.20014
- Kliegl, R., & Lindenberger, U. (1993). Modeling intrusions and correct recall in episodic memory: Adult age differences in encoding of list context. Journal of Experimental Psychology: Learning, Memory, and Cognition, 19(3), 617–637. https://doi.org/10.1037/0278-7393.19.3.617
- Koen, J. D., & Yonelinas, A. P. (2014). The effects of healthy aging, amnestic mild cognitive impairment, and Alzheimer’s disease on recollection and familiarity: A meta-analytic review. Neuropsychology Review, 24(3), 332–354. https://doi.org/10.1007/s11065-014-9266-5
- Krishnan, A., Williams, L. J., McIntosh, A. R., & Abdi, H. (2011). Partial Least Squares (PLS) methods for neuroimaging: A tutorial and review. NeuroImage, 56(2), 455–475. https://doi.org/10.1016/j.neuroimage.2010.07.034
- Kumaran, D., & Maguire, E. A. (2007). Match–mismatch processes underlie human hippocampal responses to associative novelty. The Journal of Neuroscience, 27(32), 8517–8524. https://doi.org/10.1523/JNEUROSCI.1677-07.2007
- Legrand, N., Engen, S. S., Correa, C. M. C., Mathiasen, N. K., Nikolova, N., Fardo, F., & Allen, M. (2021). Emotional metacognition: Stimulus valence modulates cardiac arousal and metamemory. Cognition and Emotion, 35(4), 705–721. https://doi.org/10.1080/02699931.2020.1859993
- Light, L. L. (1991). Memory and aging: Four hypotheses in search of data. Annual Review of Psychology, 42(1), 333–376. http://www.annualreviews.org/doi/pdf/10.1146annurev.ps.42.020191.002001
- Maniscalco, B., & Lau, H. (2012). A signal detection theoretic approach for estimating metacognitive sensitivity from confidence ratings. Consciousness and Cognition, 21(1), 422–430. https://doi.org/10.1016/j.concog.2011.09.021
- Marek, S., Tervo-Clemmens, B., Calabro, F. J., Montez, D. F., Kay, B. P., Hatoum, A. S., Donohue, M. R., Foran, W., Miller, R. L., Hendrickson, T. J., Malone, S. M., Kandala, S., Feczko, E., Miranda-Dominguez, O., Graham, A. M., Earl, E. A., Perrone, A. J., Cordova, M., Doyle, O., … Dosenbach, N. U. F. (2022). Reproducible brain-wide association studies require thousands of individuals. Nature, 603(7902), 654–660. https://doi.org/10.1038/s41586-022-04492-9
- MATLAB version: 9.13.0 (R2022b). (2022). The MathWorks Inc.
- McCurdy, L. Y., Maniscalco, B., Metcalfe, J., Liu, K. Y., de Lange, F. P., & Lau, H. (2013). Anatomical coupling between distinct metacognitive systems for memory and visual perception. The Journal of Neuroscience, 33(5), 1897–1906. https://doi.org/10.1523/JNEUROSCI.1890-12.2013
- McIntosh, A. R., & Lobaugh, N. J. (2004). Partial least squares analysis of neuroimaging data: Applications and advances. NeuroImage, 23(SUPPL. 1), S250–S263. https://doi.org/10.1016/j.neuroimage.2004.07.020
- Minear, M., & Park, D. C. (2004). A lifespan database of adult facial stimuli. Behavior Research Methods, Instruments, & Computers, 36(4), 630–633. https://doi.org/10.3758/BF03206543
- Naveh-Benjamin, M. (2000). Adult age differences in memory performance: Tests of an associative deficit hypothesis. Journal of Experimental Psychology: Learning, Memory, and Cognition, 26(5), 1170–1187. https://doi.org/10.1037/0278-7393.26.5.1170
- Nicolle, A., Klein-Flügge, M. C., Hunt, L. T., Vlaev, I., Dolan, R. J., & Behrens, T. E. J. (2012). An agent independent axis for executed and modeled choice in medial prefrontal cortex. Neuron, 75(6), 1114–1121. https://doi.org/10.1016/j.neuron.2012.07.023
- Old, S. R., & Naveh-Benjamin, M. (2008). Memory for people and their actions: Further evidence for an age-related associative deficit. Psychology and Aging, 23(2), 467–472. https://doi.org/10.1037/0882-7974.23.2.467
- Palmer, E. C., David, A. S., & Fleming, S. M. (2014). Effects of age on metacognitive efficiency. Consciousness and Cognition, 28(1), 151–160. https://doi.org/10.1016/j.concog.2014.06.007
- Ranganath, C., & Ritchey, M. (2012). Two cortical systems for memory-guided behaviour. Nature Reviews Neuroscience, 13(10), 713–726. https://doi.org/10.1038/nrn3338
- Raz, N., Ghisletta, P., Rodrigue, K. M., Kennedy, K. M., & Lindenberger, U. (2010). Trajectories of brain aging in middle-aged and older adults: Regional and individual differences. NeuroImage, 51(2), 501–511. https://doi.org/10.1016/j.neuroimage.2010.03.020
- Raz, N., Lindenberger, U., Rodrigue, K. M., Kennedy, K. M., Head, D., Williamson, A., Dahle, C., Gerstorf, D., & Acker, J. D. (2005). Regional brain changes in aging healthy adults: General trends, individual differences and modifiers. Cerebral Cortex, 15(11), 1676–1689. https://doi.org/10.1093/cercor/bhi044
- Ritchey, M., & Cooper, R. A. (2020). Deconstructing the posterior medial episodic network. Trends in Cognitive Sciences, 24(6), 451–465. https://doi.org/10.1016/j.tics.2020.03.006
- Rönnlund, M., Nyberg, L., Bäckman, L., & Nilsson, L. G. (2005). Stability, growth, and decline in adult life span development of declarative memory: Cross-sectional and longitudinal data from a population-based study. Psychology and Aging, 20(1), 3–18. https://doi.org/10.1037/0882-7974.20.1.3
- Schnider, A. (2003). Spontaneous confabulation and the adaptation of thought to ongoing reality. Nature Reviews Neuroscience, 4(8), 662–671. https://doi.org/10.1038/nrn1179
- Shing, Y. L., Rodrigue, K. M., Kennedy, K. M., Fandakova, Y., Bodammer, N., Werkle-Bergner, M., Lindenberger, U., & Raz, N. (2011). Hippocampal subfield volumes: Age, vascular risk, and correlation with associative memory. Frontiers in Aging Neuroscience, 3, 1–8. https://doi.org/10.3389/fnagi.2011.00002.
- Shing, Y. L., Werkle-Bergner, M., Li, S.-C., & Lindenberger, U.. (2009). Committing memory errors with high confidence: Older adults do but children don't. Memory, 17(2), 169–179https://doi.org/10.1080/09658210802190596.
- Simons, J. S., Peers, P. V., Mazuz, Y. S., Berryhill, M. E., & Olson, I. R. (2010). Dissociation between memory accuracy and memory confidence following bilateral parietal lesions. Cerebral Cortex, 20(2), 479–485. https://doi.org/10.1093/CERCOR/BHP116
- Spencer, W. D., & Raz, N. (1995). Differential effects of aging on memory for content and context: A meta-analysis. Psychology and Aging, 10(4), 527–539. https://doi.org/10.1037/0882-7974.10.4.527
- Sreekumar, V., Nielson, D. M., Smith, T. A., Dennis, S. J., & Sederberg, P. B. (2018). The experience of vivid autobiographical reminiscence is supported by subjective content representations in the precuneus. Scientific Reports, 8(1), 1–19. https://doi.org/10.1038/s41598-018-32879-0
- Ullsperger, M., Harsay, H. A., Wessel, J. R., & Ridderinkhof, K. R. (2010). Conscious perception of errors and its relation to the anterior insula. Brain Structure and Function, 214(5–6), 629–643. https://doi.org/10.1007/s00429-010-0261-1
- Vaccaro, A. G., & Fleming, S. M. (2018). Thinking about thinking: A coordinate-based meta-analysis of neuroimaging studies of metacognitive judgements. Brain and Neuroscience Advances, 2, 239821281881059. https://doi.org/10.1177/2398212818810591
- Voevodskaya, O. (2014). The effects of intracranial volume adjustment approaches on multiple regional MRI volumes in healthy aging and Alzheimer’s disease. Frontiers in Aging Neuroscience, 6, 264. https://doi.org/10.3389/FNAGI.2014.00264.
- White, T. P., Engen, N. H., Sørensen, S., Overgaard, M., & Shergill, S. S. (2014). Uncertainty and confidence from the triple-network perspective: Voxel-based meta-analyses. Brain and Cognition, 85(1), 191–200. https://doi.org/10.1016/j.bandc.2013.12.002
- Ye, Q., Zou, F., Lau, H., Hu, Y., & Kwok, S. C. (2018). Causal evidence for mnemonic metacognition in human precuneus. The Journal of Neuroscience, 38(28), 6379–6387. https://doi.org/10.1523/JNEUROSCI.0660-18.2018
- Yushkevich, P. A., Pluta, J. B., Wang, H., Xie, L., Ding, S. L., Gertje, E. C., Mancuso, L., Kliot, D., Das, S. R., & Wolk, D. A. (2015). Automated volumetry and regional thickness analysis of hippocampal subfields and medial temporal cortical structures in mild cognitive impairment. Human Brain Mapping, 36(1), 258–287. https://doi.org/10.1002/hbm.22627