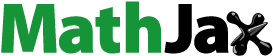
ABSTRACT
Urban Agglomeration (UA) is regarded as an emerging complex urban system in China. The development of UA demands a reasonable scale structure, which can be investigated by Zipf’s law. However, few studies have been conducted to quantify the optimal scale of UA and how its development deviates from the optimal scale. With the continuous urban expansion, the problem of UAs’ scale structure has received increasing attention. In this study, we propose a method based on Zipf’s law for estimating the theoretical optimal scale of UAs in China and assessing the deviation rate from their optimal scales. Twelve typical UAs in China are selected, and their development is assessed via urban impervious surface data from 2000 to 2018. The results show that the average deviation rate of the investigated UAs decreased from 3.40% in 2000 to 2.32% in 2018, demonstrating that these UAs are on a positive evolution trajectory. Furthermore, according to the development stage, we make recommendations on “large cities vs. medium/small-sized cities and promoting vs. restraining” to each UA based on its size. The conceptual and analytical knowledge, as well as the results from this study, are expected to offer valuable insights and new references for regulating and managing UAs’ development in China.
1. Introduction
Rapid urbanization is one of the most significant changes occurring in contemporary human society (Huang, Liu, and Li Citation2021). The process of urbanization has promoted linkages between cities as well as their surroundings, and the dependence between neighboring cities is increasing (Fang, Li, and Song Citation2017). Urban Agglomeration (UA) is regarded as an emerging complex urban system, which is the highest spatial organization form in the mature stage of urban development (Fang Citation2019; He et al. Citation2019). In China, the process of urbanization has been accelerated since the reform and opening up in 1978. The policies are a series of plans guided by government decision-making and focused on the sustainable use of land resources. They include policies concerning the economy, culture, public service layout and land regional division and can determine future regional plan activities by optimizing the land function structure and supporting the accomplishment of established development goals and sustainable development (Yang and Shi Citation2017; Li et al. Citation2022).
With the constant expansion of UAs in China, the Urban Impervious Surface (UIS), a foundational element of urban growth that characterizes human activities and socioeconomic development, has increased dramatically. UIS is a general term for built-up land, which includes land use types of buildings, roads, squares, parking lots, etc. (Shao et al. Citation2019; Tang et al. Citation2021). In 2015, the UIS area in China reached 48,800 km2 (Kuang Citation2020). However, there exists a notable contradiction between UAs’ development, land protection and environmental sustainability in China (Fang et al. Citation2019; Trinder and Liu Citation2020; Wang et al. Citation2021). In the early 1990s, China began to improve land use management by safeguarding arable land services and limiting the growth of built-up areas (Yu, Zhou, and Yang Citation2019). The Chinese government has implemented several policy measures, such as the policy of “Increasing vs. decreasing the balance of urban-rural built-up land” (Chen et al. Citation2016). Nonetheless, the conflict between regional land sources and economic growth is becoming increasingly serious. Therefore, determining effective land management strategies for UAs’ sustainable development is much needed.Rank-size distribution is one of the basic laws in interurban geography (Chen and Zhou Citation2006). Under the concept of Rank-size distribution, Zipf’s law, an efficient way to understand the static organization, represents a Self-Organized Critical (SOC) state of complex spatial systems (Zhang, Yu, and Fan Citation2012; Bee, Riccaboni, and Schiavo Citation2019). SOC provides inspiration to investigate the urbanization process and to discuss the coordinated evolution and development of reasonable city sizes. In recent years, Zipf’s law is often used to explore the land structure of the urban system, including evaluating the evolution of urban structure on the national scale (Gangopadhyay and Basu Citation2010) and quantifying the complexity and diversity of urban internal forms (Blumenfeld-Lieberthal and Portugali Citation2010; Rezayan et al. Citation2010). Ghosh and Basu (Citation2019) argued that a complete urban system should have a reasonable self-sustaining hierarchical structure. Although Zipf’s law is regarded as the universal law of the system, we have to acknowledge that policy and legal intervention in urban systems at all scales in China can affect the self-organized development of the system. There are numerous studies that focused on sustainable land use strategies and that proposed few frameworks to evaluate the implementation of land plans and policies in China, which are important for macro-regional socioeconomic development. Wan et al. (Citation2020) confirmed the opposing impacts of government interventions and market factors on the deviations of city sizes from Zipf’s law. Liu, Wang, and Guo (Citation2018) explored the adjustment and strategies of land use under the background of “new normal”. However, few studies paid attention to the issue of the effects of land policies and deviations from Zipf’s law in a quantitative manner (González-Val Citation2010; Wan et al. Citation2020). Finance and Swerts (Citation2020) raised the issue of self-organization degradation or alienation, implying that if urban management interventions are counterproductive, the fractal structure and self-organized distribution could be disturbed. It is unclear whether the land evolution of UAs is insufficient or surplus under the land policy. This paper attempts to quantify the deviations between the actual scale and optimum scale based on Zipf’s law and provide valuable references for UAs’ future adjustment and development.The main objective of this study is to explore the land structure evolution of UAs in China and assess the deviations from Zipf’s law. More specifically, using UIS remote sensing datasets of 12 typical UAs from 2000 to 2018 in China, we make the following contributions. First, most studies on Zipf’s law are based on data at the national scale, whereas we concentrate on the UAs, emerging complex urban systems in China. We systematically investigated the land expansion and structural evolution of the UAs. Second, we propose a model for quantifying the UAs’ optimal scale from Zipf’s law based on continuous expression instead of the discrete expression and estimate the deviation rates of UAs between the actual scale and optimal scale. Third, according to the scale structure and deviation rate from our findings, we give specific development suggestions for each UAs’ land regulation. The results of this study offer valuable insights and new references for the rational urban growth model and sustainable goals of UAs in regulation and management.
2. Study area and data
2.1. Study area
China’s New Urbanization strategy released in 2014 highlights the progressive development of UAs in China (NDRC Citation2014). Considering the data availability and the development status of UAs, a total of 12 typical UAs in China were chosen as study areas to be evaluated, as shown in .. Three UAs that include Beijing-Tianjin-Hebei (BTH-UA), Yangtze River Delta (YRD-UA), and Pearl River Delta (PRD-UA) are expected to grow into world-class UAs, representing the most developed areas of the country with a strong economic foundation, which have the most potential to attract growth (Cai et al. Citation2021; Guan et al. Citation2018; Liu et al. Citation2020). Middle Reaches of Yangtze River (MRYR-UA), Chongqing-Chengdu (CC-UA), Shandong Peninsula (SP-UA), and West Coast of Taiwan Straits (WCTS-UA) are planned to become large nation-class UAs. Nation-class UAs represent rapidly growing areas that can mimic those of world-class UAs. The developing process of region-class UAs that include Harbin-Changchun (HC-UA), Central Plain (CP-UA), Beibu Gulf (BG-UA), Central and Southern Liaoning (CSL-UA), Guanzhong Plain (GP-UA) is expected to be accelerated. These UAs generally lag behind world-class and national class UAs. More details regarding the definition of UAs can be found (Fang and Yu Citation2017; Guo et al. Citation2007). The selected UAs consist of a total of 158 cities, mainly in the east and middle of China ().
Table 1. Status among the selected 12 urban agglomerations in 2018.
2.2. Data acquisition and processing
The rapid development of earth observation technologies has supplied valuable scientific information for monitoring and investigating the urbanization process as well as dynamic patterns from a spatial perspective (Ding et al. Citation2021; Shao, Wu, and Li Citation2021). Two types of remote sensing datasets are used to describe urban boundaries and impervious surface expansion in this study. UIS data is derived to characterize urban built-up land by clipping impervious surface data with urban boundaries data, as shown in . The investigated temporal period covers the years 2000, 2005, 2010, 2015, and 2018.
Figure 2. An example of urban impervious surfaces expansion of BTH-UA during the investigated period.

Impervious surface data is extracted from the Global Artificial Impervious Area (GAIA) dataset, an annual product in raster format with a 30 × 30 m resolution between 1985 and 2018, with an average accuracy of more than 90% for multiple years (Gong et al. Citation2020). Furthermore, Urban Boundary (UB) data is retrieved from the Global Urban Boundaries (GUB) dataset by Li et al. (Citation2020), which is a multi-temporal vector dataset from 2000 to 2018 based on GAIA data. The GUB dataset has great consistency with other products and can better capture the irregular shapes of cities around urban fringes.
3. Methodology
The designed model contains three major steps: 1) determining the structure of UA; 2) diagnosing the scale deviations of UA; and 3) providing suggestions for the sustainable development of UA. The following sub-sessions detail these steps.
3.1. Determining the structure of UAs
Zipf’s law provides an opportunity to determine which scale class dominates the urban system and how this distribution changes over time (Farrell and Nijkamp Citation2019). The common form of Zipf’s law of rank-size distribution (Zipf Citation1949) is defined as:
where denotes the rank of the city in UA,
is the UIS scale of the cities,
is a constant and
can be termed Zipf’s scaling exponent that represents the scale structure of UAs. Chen and Zhou (Citation2006) have proved that the fractal dimension,
noise and Zipf’s dimension are mathematically expressed in relation to each other, which can illustrate three different self-organizing critical states of the system. According to the value of
, the scale structure of UA is categorized into three types (Gan, Li, and Song Citation2006). When
approaches 1, the UA scale distribution satisfies Zipf’s criterion, suggesting the optimal distribution in the natural state; when
, the scale distribution of cities is relatively scattered, as large cities in the UA are not prominent while small and medium-sized cities are developed; when
, the scale of cities is relatively concentrated, as the UA is dominated by large cities while small and medium-sized cities are insufficiently developed. When
, all cities are developed in an equal manner. A
that approaches infinite suggests that this UA has only one city (Zhang et al. Citation2019).
3.2. Deviation rate from Zipf’s law
Zipf’s law is used to reflect the scale of cities and their relationship with the entire system in terms of rank. It has been used as a quantitative criterion for optimizing the scale of the urban system (Fang and Wang Citation2015). The optimal scale of UA based on Zipf’s law by discrete expression is defined as:
where refers to the optimal area of UA,
is the optimal UIS scale of the cities in UAs,
and
are Zipf’s scaling exponents determined by EquationEquation (1)
(1)
(1) .
Zipf’s distribution can be abstracted as a simple problem of -series whose
is exactly the
of Zipf’s distribution. Zipf’s model of rank-size distributions is a well-known two-parameter model that is proved to be equivalent to Pareto’s cumulative distribution function. For pure Zipf’s distribution, the exponent
equals 1. In this case, the two-parameter model is reduced to a one-parameter model, i.e.
, which describes the inverse relationship between rank and scale. If
, the p-series changes to the harmonic sequence. The changing regularity of a sequence can be judged through generalized integral (Chen Citation2021). For a scaling function, both its differential and integral results obey the scaling law (Finance and Swerts Citation2020). EquationEquation (2)
(2)
(2) reaches theoretical optimal when the number of cities tends to infinity. However, in reality, the number of cities in UA is limited. In theoretical studies, the analytical process based on continuous expression is superior to that based on discrete sequences in the condition of a limited number of cities. Therefore, the optimal scale of UA based on Zipf’s law with continuous expression is proposed as:
where denotes deviations rate, and
is the current UIS scale of UA.
3.3. Model for the development suggestion of urban agglomeration
We propose a model for UA’s development suggestion based on the structure index () by EquationEquation (1)
(1)
(1) and the Deviation Rate (
) by EquationEquation (4)
(4)
(4) .
represents the process of decision-making, where a positive value of
means “promotion” and a negative value of
means “restraining”. It is assumed that the major domination in UA is “large cities” when
and “small-medium sized cities” when
. We argue that UA’s regulation needs to approach the origin of the coordinate system where
and
. Thus, the development suggestions of UA are categorized into four types: 1) restraining medium-small-sized cities (L-H), 2) promoting large cities (L-L), 3) promoting medium-small-sized cities (H-L), and 4) restraining large cities (H-H). shows the concept of the four-quadrant graph of our proposed suggestion model.
4. Results
4.1. The expansion of urban agglomerations
shows the magnitude of UA’s expansion. Among the selected 12 UAs, the YRD-UA, the UA with the most UIS growth, expanded by a total of 20,476 km2 from 2000 to 2018. YRD-UA and BTH-UA are UAs with the top two fastest rates of 1717 km2/yr and 1656 km2/yr during 2015–2018, respectively. While the expansion scales of different levels of UAs differed over the past two decades, most UAs had experienced substantial expansion during the investigated period. The UIS and UB scales of various UAs experienced rapid and synchronous development, particularly in the period from 2015 to 2018, when the accelerated trends occurred.
We also measured the proportion of UIS within the UB, denoted as PUIS, which was shown in . PUIS variations are mainly used to represent the changes in human activity intensities and local environmental conditions (Kuang Citation2020). The results showed that the average PUIS is 64.27%±13.86%. A notable exception is CY-UA, whose PUIS was less than 55%. We observed that PUIS had a marked decline from 2015 to 2018, owing to the fact that UB expanded faster than UIS. Our findings coincide with those of Kuang (Citation2020) and Sun and Zhao (Citation2018), who also documented similar trends.
4.2. Scale structure of urban agglomerations
presents Zipf’s scaling exponent, which aids in identifying the structural types of UAs intuitively. The coefficients of determination were greater than 0.95, and measurement results passed testing by 1%, indicating that the fitted values and real values of regression formulas corresponded well. This shows that the land scale structure of UAs accords with Zipf’s law. As of 2018, two UAs (i.e. YRD-UA and PRD-UA) were in the optimal distribution (), suggesting optimal rank-size structures that exhibit well-fitting distributions. Six UAs were in scattered distribution (
) whose medium/small-sized cities were relatively developed. These UAs include MRYR-UA, BTH-UA, HC-UA, CP-UA, SP-UA, BG- UA, and WCTS-UA, whose scale distributions only differed slightly, and the urban scale systems were more balanced. What’s more, the scale structure of four UAs (i.e. CC-UA, GP-UA, LZ-UA, and CSL-UA) was unbalanced (
). It also means that big cities were over-developed or medium/small-sized cities were under-developed. We observed that the scale difference between cities was considerable, as few hub cities had greater aggregating potential and magnetic forces to attract socioeconomic variables or flows, potentially resulting in the cumulative Matthew effect (Liu, Wang, and Guo Citation2018).Furthermore, we found that the scaling index of UAs fluctuated from 2000 to 2018. Theoretically, a reasonable self-organized system tends to gradually approach the direction of optimized scale structure (
). However, several UAs, in particular, SP-UA and MRYR-UA, gradually progressed in the direction of deviation. Such fluctuation of the scale structure of UA reflected the uncertainty introduced by the external impact of land policies.
Table 2. Zipf’s scaling exponent of the selected 12 urban agglomerations in China.
4.3. Scale deviations of urban agglomerations and suggestions
illustrates the deviation rates against the scales of the 12 UAs based on EquationEquation (5(5)
(5) ). The UAs can be classified into two different categories based on the deviation rate: exceeding scale (
) and inadequate scale (
). As of 2018, five UAs that include PRD-UA, SP-UA, CSL-UA, HC-UA, and BG-UA, were at exceeding scales. The remaining seven UAs were at inadequate scales. From 2000 to 2018, the majority of the deviation from Zipf’s law displayed a declining pattern, with the exception of HC-UA, whose deviation rates were increasing. The average value of the absolute deviation rate of 12 UAs decreased from 3.40% in 2000 to 2.32% in 2018, which demonstrates that the UAs are on a positive trajectory.
Furthermore, we generated four-quadrant graphs to intuitively reach the development suggestions, as shown in . Give the situation in the year 2018, H-L includes CC-UA and GP-UA; L-H includes CP-UA, MRYR-UA, BTH-UA, WCTS-UA, and YRD-UA; H-H includes only CSL-UA; L-L includes SP-UA, HC-UA, PRD-UA, BG-UA. Note that there existed quadrant changes among UAs. For example, YRD-UA was H-L during 2000–2015, but it changed to L-H in 2018. Such quadrant changes suggest that land policies should not be fixed but adjustable in a dynamic manner. In addition, BG-UA and CSL-UA with increased distances from the origin deviated from expectations during the investigated period. The above results are expected to provide quantitative and intuitive references for the systematic policy recommendations.
5. Discussion and conclusion
In this study, we investigate the evolution of UA under rapid land urbanization. Specifically, we focus on the scale, structure, and deviation of UAs with a novel framework. Our study areas are located in China, an ideal country to analyze urbanization due to its fast urbanization rate and policies implemented by governments at all levels in regulating land urbanization as well as the scale distributions of UAs. Various land policies and macro-control strategies are the propellers of urban system evolutions. On the basis of Zipf’s law, we target the structure of twelve selected UAs and propose a developed model to quantify the deviation rate of UAs’ scale. According to the four-quadrant graphs shown in , the land development suggestions of UAs are intuitively divided into four modes: “large cities vs. medium/small-sized cities and promoting vs. restraining”. The results from this study facilitate the identification of the structure and deviations of UAs and provide targeted development suggestions for various UAs, benefiting the realization of sustainable urban growth.
Zipf’s law is an observational regulation that has been a common product of spontaneous or natural phenomena. In China, government interventions are expected to increase while market forces are expected to decrease, resulting in deviations of UA development from Zipf’s law (Wan et al. Citation2020). Although the average deviation rate of the selected twelve UAs demonstrates that the UAs are on a positive trajectory, our findings suggest that the scale and deviation of UAs present great diversity. In 1988, the “Paid Transfer of Land Use Rights” scheme was implemented, aiming to promote the growth of the local land scale (Wu, Guo, and Hewings Citation2019). In the early 2000s, the land use program was implemented as a major part of macro-control policies of the national economy (Wu, Guo, and Hewings Citation2019). Since then, local governments started to assist the expansion of built-up areas by increasing land supply to facilitate investment and foster manufacturing as well as real estate. However, researchers and policymakers have been concerned over the land urbanization bubble, a rapid and catastrophic rise and fall in urban development fueled by a combination of misplaced exuberance and speculation (Jiao and Liu Citation2012; Zhang and Li Citation2020). Restriction on growth has been a major trend of local governance under the supervision of the central government. The evolution of Chinese land policies experienced a transformation from the stringent management of cultivated land quotas to the intensive use of existing land, with a shift from an emphasis on quantity to quality (Wang et al. Citation2018; Zhuang et al. Citation2021). However, indicated by the homogeneity of scale expansion and the chaotic volatility of the structure index, certain UAs gradually lost their self-organized characteristics, with policies, to a certain degree, failing to diminish entropy.
Fortunately, UA planning has recently been acknowledged as a critical component of China’s territorial spatial planning system. Cities are no longer regarded as isolated entities. Instead, they form complex urban systems connected by broad economic and social structures that evolve in a continuous manner. The scale structure management of UA has been highlighted in the planning material of “Regulations for Compilation of Urban Land Spatial Planning in 2021”. We compare the historical UAs’ development planning with the suggestions shown in . Taking YRD-UA and HC-UA as examples, the YRD-UA highlighted the development vitality of small and medium-sized cities, as well as the leading role of large cities such as Shanghai, which is consistent with our findings (Liu Citation2012; Xu et al. Citation2021). However, contradicting the “restraining medium/small-sized cities”, HC-UA has been encouraging the growth of large cities such as Harbin, leading to increased deviation from its optimal scale indicated by Zipf’s law. Furthermore, a rising number of UAs are following “promoting large cities” and “restraining medium/small-sized cities” policies, potentially resulting in large cities with an insufficient land scale and medium/small-sized cities with excessive expansion. By investigating the evolution of selected UAs in China, the results from this study provide valuable strategies for UAs’ optimization and development, preventing the entropy increase in the evolution process and allowing for healthy evolution in the context of sustainable self-organization.
We also need to acknowledge the limitation of this study. First, we assume that the number of cities in UAs is constant during the investigated period. In China, however, we have seen a changed number of cities in certain UAs in response to the policies regulation. This limitation, however, is expected to slightly alter the analytical results and does not harm the validity of our conclusions. For future studies, a more detailed survey of UAs is recommended to determine the rank-size rule or the primate-city rule in Zipf’s law. Furthermore, we quantitatively evaluated the deviation of the UAs’ development from Zipf’s law in this study, while the city-level deviation within UAs remains underexploited. Future studies are encouraged to shed light on the evolution of the UAs’ microstructures.
Acknowledgments
The authors are sincerely grateful to the editors as well as the anonymous reviewers for their valuable suggestions and comments that helped us improve this paper significantly.
Data availability statement
The data that support the findings of this study are available from the corresponding author upon reasonable request.
Disclosure statement
No potential conflict of interest was reported by the author(s).
Additional information
Funding
Notes on contributors
Bowen Cai
Bowen Cai received the BS degree in remote sensing science and technology from Wuhan University in 2018. He is currently pursuing his PhD degree at the School of Remote Sensing and Information Engineering, Wuhan University. His research interests include urban remote sensing, land use policy and remote sensing applications.
Zhenfeng Shao
Zhenfeng Shao received his PhD degree in photogrammetry and remote sensing from Wuhan University in 2004. Since 2009, he has been a Full Professor with the State Key Laboratory of Information Engineering in Surveying, Mapping and Remote Sensing (LIESMARS), Wuhan University. He has authored or coauthored over 70 peer-reviewed articles in international journals. His research interests include urban remote sensing, pattern recognition, and urban remote sensing applications.
Shenghui Fang
Shenghui Fang is an associate professor in the School of Remote Sensing and Information Engineering, Wuhan University. His research interests include quantitative remote sensing and remote sensing applications.
Xiao Huang
Xiao Huang received his PhD degree in Geography from the University of South Carolina in 2020. He is currently an Assistant Professor in the Department of Geosciences at the University of Arkansas. His research interests include remote sensing, big data and social sensing.
Yun Tang
Yun Tang received her MS degree in Cartography and Geographic Information System, Fuzhou University in 2018. She currently pursuing her PhD degree at the School of Remote Sensing and Information Engineering, Wuhan University. Her research interests include urban remote sensing, and geography.
Muchen Zheng
Muchen Zheng is currently pursuing her PhD degree at the School of Resource and Environmental Sciences, Wuhan University. Her research interests include map generalization, geography and remote sensing applications.
Hao Zhang
Hao Zhang is currently pursuing his PhD degree at the School of Remote Sensing and Information Engineering, Wuhan University. His research interests include digital photogrammetry and remote sensing.
References
- Alexanderson, G. 2005. “Gamma: Exploring Euler’s Constant.” The Mathematical Intelligencer 27 (1): 86–88. doi:10.1007/BF02984820.
- Bee, M., M. Riccaboni, and S. Schiavo. 2019. ”Distribution of City Size: Gibrat, Pareto, Zipf.” In Modeling and Simulation in Science, Engineering and Technology. Cham: Springer. doi:10.1007/978-3-030-12381-9_4.
- Blumenfeld-Lieberthal, E., and J. Portugali. 2010. ”Network Cities: A Complexity-Network Approach to Urban Dynamics and Development.” In Geospatial Analysis and Modelling of Urban Structure and Dynamics. Dordrecht: Springer. doi:10.1007/978-90-481-8572-6_5.
- Cai, B., Z. Shao, S. Fang, X. Huang, M. Huq, Y. Tang, Y. Li, and Q. Zhuang. 2021. “Finer-Scale Spatiotemporal Coupling Coordination Model Between Socioeconomic Activity and Eco-Environment: A Case Study of Beijing, China.” Ecological Indicators 131: 108165. doi:10.1016/j.ecolind.2021.108165.
- Chen, Y. 2021. “Exploring the Level of Urbanization Based on Zipf’s Scaling Exponent.” Physica A: Statistical Mechanics and Its Applications 566: 125620. doi:10.1016/j.physa.2020.125620.
- Chen, Y., Z. Chen, G. Xu, and Z. Tian. 2016. “Built-Up Land Efficiency in Urban China: Insights from the General Land Use Plan (2006–2020).” Habitat International 51: 31–38. doi:10.1016/j.habitatint.2015.10.014.
- Chen, Y., and Y. Zhou. 2006. “Reinterpreting Central Place Networks Using Ideas from Fractals and Self-Organized Criticality.” Environment and Planning B: Urban Analytics and City Science 33 (3): 345–364. doi:10.1068/b31131.
- Ding, Q., Z. Shao, H. Huang, and A. Orhan. 2021. “DSA-Net: A Novel Deeply Supervised Attention-Guided Network for Building Change Detection in High-Resolution Remote Sensing Images.” International Journal of Applied Earth Observation and Geoinformation 105: 102591. doi:10.1016/j.jag.2021.102591.
- Fang, C. 2019. “The Basic Law of the Formation and Expansion in Urban Agglomerations.” Journal of Geographical Sciences 29 (10): 1699–1712. doi:10.1007/s11442-019-1686-y.
- Fang, C., X. Cui, G. Li, C. Bao, Z. Wang, H. Ma, S. Sun, H. Liu, K. Luo, and Y. Ren. 2019. “Modeling Regional Sustainable Development Scenarios Using the Urbanization and Eco-Environment Coupler: Case Study of Beijing-Tianjin-Hebei Urban Agglomeration, China.” The Science of the Total Environment 689: 820–830. doi:10.1016/j.scitotenv.2019.06.430.
- Fang, L., P. Li, and S. Song. 2017. “China’s Urban Development Policies and City Growth Dynamics: An Analysis Based on the Gibrat’s Law.” International Review of Economics & Finance 52: 322–329. doi:10.1016/j.iref.2017.01.017.
- Fang, C., and Z. Wang. 2015. “Quantitative Diagnoses and Comprehensive Evaluations of the Rationality of Chinese Urban Development Patterns.” Sustainability 7 (4): 3859. doi:10.3390/su7043859.
- Fang, C., and D. Yu. 2017. “Urban Agglomeration: An Evolving Concept of an Emerging Phenomenon.” Landscape and Urban Planning 162: 126–136. doi:10.1016/j.landurbplan.2017.02.014.
- Farrell, K., and P. Nijkamp. 2019. “The Evolution of National Urban Systems in China, Nigeria and India.” Journal of Urban Management 8 (3): 408–419. doi:10.1016/j.jum.2019.03.003.
- Finance, O., and E. Swerts. 2020. ”Scaling Laws in Urban Geography. Linkages with Urban Theories, Challenges and Limitations.” In Theories and Models of Urbanization. Cham: Springer. doi:10.1007/978-3-030-36656-8_5.
- Gangopadhyay, K., and B. Basu. 2010. ”The Morphology of Urban Agglomerations for Developing Countries: A Case Study with China.” In Econophysics and Economics of Games, Social Choices and Quantitative Techniques. Milano: Springer. doi:10.1007/978-88-470-1501-2_11.
- Gan, L., D. Li, and S. Song. 2006. “Is the Zipf Law Spurious in Explaining City-Size Distributions?” Economics Letters 92 (2): 256–262. doi:10.1016/j.econlet.2006.03.004.
- Ghosh, A., and B. Basu. 2019. “Universal City-Size Distributions Through Rank Ordering.” Physica A: Statistical Mechanics and Its Applications 528: 121094. doi:10.1016/j.physa.2019.121094.
- Gong, P., X. Li, J. Wang, Y. Bai, B. Chen, T. Hu, X. Liu, et al. 2020. “Annual Maps of Global Artificial Impervious Area (GAIA) Between 1985 and 2018.” Remote Sensing of Environment 236: 111510. doi:10.1016/j.rse.2019.111510.
- González-Val, R. 2010. “Deviations from Zipf’s Law for American Cities: An Empirical Examination.” Urban Studies 48 (5): 1017–1035. doi:10.1177/0042098010371394.
- Guan, X., H. Wei, S. Lu, Q. Dai, and H. Su. 2018. “Assessment on the Urbanization Strategy in China: Achievements, Challenges and Reflections.” Habitat International 71: 97–109. doi:10.1016/j.habitatint.2017.11.009.
- Guo, R., C. Miao, X. Li, and D. Chen. 2007. “Eco-Spatial Structure of Urban Agglomeration.” Chinese Geographical Science 17 (1): 28–33. doi:10.1007/s11769-007-0028-7.
- He, Q., C. Zeng, P. Xie, S. Tan, and J. Wu. 2019. “Comparison of Urban Growth Patterns and Changes Between Three Urban Agglomerations in China and Three Metropolises in the USA from 1995 to 2015.” Sustainable Cities and Society 50: 101649. doi:10.1016/j.scs.2019.101649.
- Huang, X., A. Liu, and J. Li. 2021. “Mapping and Analyzing the Local Climate Zones in China’s 32 Major Cities Using Landsat Imagery Based on a Novel Convolutional Neural Network.” Geo-Spatial Information Science 24 (4): 528–557. doi:10.1080/10095020.2021.1892459.
- Jiao, L., and Y. Liu. 2012. “Analyzing the Spatial Autocorrelation of Regional Urban Datum Land Price.” Geo-Spatial Information Science 15 (4): 263–269. doi:10.1080/10095020.2012.714103.
- Kuang, W. 2020. “National Urban Land-Use/cover Change Since the Beginning of the 21st Century and Its Policy Implications in China.” Land Use Policy 97: 104747. doi:10.1016/j.landusepol.2020.104747.
- Li, X., P. Gong, Y. Zhou, J. Wang, Y. Bai, B. Chen, T. Hu, et al. 2020. “Mapping Global Urban Boundaries from the Global Artificial Impervious Area (GAIA) Data.” Environmental Research Letters 15 (9): 094044. doi:10.1088/1748-9326/ab9be3.
- Li, L., S. Ma, Y. Zheng, and X. Xiao. 2022. “Land Use Policy Integrated Regional Development : Comparison of Urban Agglomeration Policies in China.” Land Use Policy 114: 105939. doi:10.1016/j.landusepol.2021.105939.
- Liu, H. 2012. “Comprehensive Carrying Capacity of the Urban Agglomeration in the Yangtze River.” Habitat International 36 (4): 462–470. doi:10.1016/j.habitatint.2012.05.003.
- Liu, Y., J. Li, and Y. Yang. 2018. “Strategic Adjustment of Land Use Policy Under the Economic Transformation.” Land Use Policy 74: 5–14. doi:10.1016/j.landusepol.2017.07.005.
- Liu, C., T. Wang, and Q. Guo. 2018. “Factors Aggregating Ability and the Regional Differences Among China’s Urban Agglomerations.” Sustainability 10 (11): 4179. doi:10.3390/su10114179.
- Liu, X., B. Zou, H. Feng, N. Liu, and H. Zhang. 2020. “Anthropogenic Factors of PM 2.5 Distributions in China’ S Major Urban Agglomerations: A Spatial-Temporal Analysis.” Journal of Cleaner Production 264: 121709. doi:10.1016/j.jclepro.2020.121709.
- NDRC (National Development and Reform Commission). 2014. “The New Urbanization Strategy of China.” http://www.ndrc.gov.cn/fzgggz/fzgh/ghwb/gjjh/201404/t20140411_606659.html.
- Rezayan, H., M. Delavar, A. Frank, and A. Mansouri. 2010. “Spatial Rules That Generate Urban Patterns: Emergence of the Power Law in the Distribution of Axial Line Length.” International Journal of Applied Earth Observation and Geoinformation 12 (5): 317–330. doi:10.1016/j.jag.2010.04.003.
- Shao, Z., H. Fu, D. Li, O. Altan, and T. Cheng. 2019. “Remote Sensing Monitoring of Multi-Scale Watersheds Impermeability for Urban Hydrological Evaluation.” Remote Sensing of Environment 232: 111338. doi:10.1016/j.rse.2019.111338.
- Shao, Z., W. Wu, and D. Li. 2021. “Spatio-Temporal-Spectral Observation Model for Urban Remote Sensing.” Geo-Spatial Information Science 24 (3): 372–386. doi:10.1080/10095020.2020.1864232.
- Sun, Y., and S. Zhao. 2018. “Spatiotemporal Dynamics of Urban Expansion in 13 Cities Across the Jing-Jin-Ji Urban Agglomeration from 1978 to 2015.” Ecological Indicators 87: 302–313. doi:10.1016/j.ecolind.2017.12.038.
- Tang, Y., Z. Shao, X. Huang, and B. Cai. 2021. “Mapping Impervious Surface Areas Using Time-Series Nighttime Light and MODIS Imagery.” Remote Sensing 13 (10): 1900. doi:10.3390/rs13101900.
- Trinder, J., and Q. Liu. 2020. “Assessing Environmental Impacts of Urban Growth Using Remote Sensing Assessing Environmental Impacts of Urban Growth Using Remote Sensing.” Geo-Spatial Information Science 23 (1): 20–39. doi:10.1080/10095020.2019.1710438.
- Wang, J., Y. Lin, A. Glendinning, and Y. Xu. 2018. “Land-Use Changes and Land Policies Evolution in China’s Urbanization Processes.” Land Use Policy 75: 375–387. doi:10.1016/j.landusepol.2018.04.011.
- Wang, Z., Q. Meng, M. Allam, D. Hu, L. Zhang, and M. Menenti. 2021. “Environmental and Anthropogenic Drivers of Surface Urban Heat Island Intensity: A Case-Study in the Yangtze River Delta, China.” Ecological Indicators 128: 107845. doi:10.1016/j.ecolind.2021.107845.
- Wan, G., D. Zhu, C. Wang, and X. Zhang. 2020. “The Size Distribution of Cities in China: Evolution of Urban System and Deviations from Zipf’s Law.” Ecological Indicators 111: 106003. doi:10.1016/j.ecolind.2019.106003.
- Wu, J., Q. Guo, and G. Hewings. 2019. “Land Regulating Economy as a Policy Instrument in Urban China.” Cities 94: 225–234. doi:10.1016/j.cities.2019.06.009.
- Wu, W., H. Zhao, Q. Tan, and P. Gao. 2019. “An Urban Scaling Estimation Method in a Heterogeneity Variance Perspective.” Entropy 21 (4): 1–21. doi:10.3390/e21040337.
- Xu, F., Z. Wang, G. Chi, D. Wang, Z. Zhang, and D. Zuo. 2021. “Differentiation and Progress of Urban Regionalization in China : Perspectives of Land Use and Geography.” Applied Geography 137: 102600. doi:10.1016/j.apgeog.2021.102600.
- Yang, S., and L. Shi. 2017. “Prediction of Long-Term Energy Consumption Trends Under the New National Urbanization Plan in China.” Journal of Cleaner Production 166: 1144–1153. doi:10.1016/j.jclepro.2017.08.092.
- Yu, J., K. Zhou, and S. Yang. 2019. “Land Use Efficiency and Influencing Factors of Urban Agglomerations in China.” Land Use Policy 88: 104143. doi:10.1016/j.landusepol.2019.104143.
- Zhang, X., and H. Li. 2020. “The Evolving Process of the Land Urbanization Bubble: Evidence from Hangzhou, China.” Cities 102: 102724. doi:10.1016/j.cities.2020.102724.
- Zhang, H., X. Ning, Z. Shao, and H. Wang. 2019. “Spatiotemporal Pattern Analysis of China’s Cities Based on High-Resolution Imagery from 2000 to 2015.” ISPRS International Journal of Geo-Information 8 (5): 241. doi:10.3390/ijgi8050241.
- Zhang, Y., J. Yu, and W. Fan. 2012. “Fractal Features of Urban Morphology and Simulation of Urban Boundary.” Geo-Spatial Information Science 11 (2): 121–126. doi:10.1007/s11806-008-0032-9.
- Zhuang, Q., Z. Shao, X. Huang, Y. Zhang, W. Wu, X. Feng, X. Lv, Q. Ding, B. Cai, and O. Altan. 2021. “Evolution of Soil Salinization Under the Background of Landscape Patterns in the Irrigated Northern Slopes of Tianshan Mountains, Xinjiang, China.” Catena 206: 105561. doi:10.1016/j.catena.2021.105561.
- Zipf, G. 1949. Human Behavior and the Principle of Least Effort: An Introduction to Human Ecology. Cambridge, MA: Addison-Wesley.