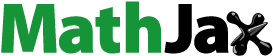
ABSTRACT
Due to the strong penetrability, long-wavelength synthetic aperture radar (SAR) can provide an opportunity to reconstruct the three-dimensional structure of the penetrable media. SAR tomography (TomoSAR) technology can resynthesize aperture perpendicular to the slant-range direction and then obtain the tomographic profile consisting of power distribution of different heights, providing a powerful technical tool for reconstructing the three-dimensional structure of the penetrable ground objects. As an emerging technology, it is different from the traditional interferometric SAR (InSAR) technology and has advantages in reconstructing the three-dimensional structure of the illuminated media. Over the past two decades, many TomoSAR methods have been proposed to improve the vertical resolution, aiming to distinguish the locations of different scatters in the unit pixel. In order to cope with the forest mission of European Space Agency (ESA) that is designed to provide P-band SAR measurements to determine the amount of biomass and carbon stored in forests, it is necessary to systematically evaluate the performance of forest height and underlying topography inversion using TomoSAR technology. In this paper, we adopt three typical algorithms, namely, Capon, Multiple Signal Classification (MUSIC), and Compressed Sensing (CS), to evaluate the performance in forest height and underlying topography inversion. The P-band airborne full-polarization (FP) SAR data of Lopè National Park in the AfriSAR campaign implemented by ESA in 2016 is adopted to verify the experiment. Furthermore, we explore the effects of different baseline designs and filter methods on the reconstruction of the tomographic profile. The results show that a better tomographic profile can be obtained by using Hamming window filter and Capon algorithm in uniform baseline distribution and a certain number of acquisitions. Compared with LiDAR results, the root-mean-square error (RMSE) of forest height and underlying topography obtained by Capon algorithm is 2.17 m and 1.58 m, which performs the best among the three algorithms.
1. Introduction
Forest is known as the “lungs of the earth”, playing an essential role in preventing wind and sand fixation, conserving water and soil, and maintaining the global carbon cycle in human life and production. According to statistics, forests cover about one-third of the land area globally, which is a crucial resource repository for human survival. Therefore, it is vital that keep an eye on forest resource changes to meet the global resource crisis (Bohn and Huth Citation2017; Mitchard Citation2018; Spies Citation1998).
The vertical structure directly reveals the growing trend of the forest and is an essential parameter for estimating the Above-Ground Biomass (AGB) and the carbon storage (Spies Citation1998; Zhang et al. Citation2014; Ramli and Tahar Citation2020). As the penetrability of long-wavelength radar, interferometric synthetic aperture radar (InSAR) has become the most potent tool for reconstructing the forest vertical structure (Pardini et al. Citation2018), especially in L-band and P-band. In terms of many InSAR technical methods, SAR Tomography (TomoSAR) technology can obtain the three-dimensional structure of the penetrable natural medium and has a unique advantage in acquiring forest height and underlying topography (Reigber and Moreira Citation2000; Yu et al. Citation2020; Aghababaei et al. Citation2020). Polarimetric InSAR (PolInSAR) technology can also obtain high-precision Canopy Height Model (CHM) and Digital Elevation Model (DEM) products, which has been proven in many works (Papathanassiou and Cloude Citation2001; Cloude and Papathanassiou Citation2003; Kugler et al. Citation2015; Neumann, Ferro-Famil and Reigber Citation2008; Cheng, Pinto, and Gong Citation2012; Wu et al. Citation2019). It follows a physical model, namely, random volume over ground (RVoG) model (Treuhaft, Moghaddam, and van Zyl Citation1996), which establishes a linear relationship with different complex coherence coefficients in the complex unit circle space. The geometrical relationship can solve forest height and underlying topography under the pure volume coherence assumption (Lee and Pottier Citation2017). However, considering the actual situation, PolInSAR technology can only obtain the phase center height of a single-pixel that is discrete and single through complex coherence coefficients. In addition, PolInSAR technology can only use multi-polarization (MP) or full-polarization (FP) datasets to invert high-precision forest height and underlying topography based on the RVoG model (Yardibi et al. Citation2010).
Reigber et al. firstly used L-band airborne FP SAR data in 2000 to obtain the three-dimensional structure of the forest using Fast Fourier Transformation (FFT) technology (Reigber and Moreira Citation2000). It is the first successful experiment of TomoSAR technology in forest applications, motivating many scientists to explore the new TomoSAR methods in forest applications. Inspired by the Direction-Of-Arrival (DOA) estimation technology, the forest structure is assumed to be divided into two layers: the ground and the canopy. The estimation of forest height and underlying topography becomes finding the phase center position of the ground and the canopy, which is similar to the estimation of source locations in the DOA estimation and appearing in the tomographic profile as a peak position (Yardibi et al. Citation2010; Del Campo, Nannini, and Reigber Citation2020). After more than 10 years of development, a variety of DOA estimation methods have been developed and introduced in TomoSAR technology. This paper mainly categorizes the TomoSAR methods into four categories: (1) Non-parametric estimation method similar to Capon algorithm. Non-parametric methods mainly depend on the estimation accuracy of the covariance matrix and can be solved without a priori knowledge of the number of scatterers. Based on the theory of statistical regularization, sparse iterative covariance-based estimation (SPICE) method (Stoica, Babu, and Li Citation2010), maximum likelihood estimation (Del Campo, Nannini, and Reigber Citation2018), iterative adaptive approach (IAA) method (Yardibi et al. Citation2010; Peng et al. Citation2018), regularized IAA (RIAA) method (Roberts et al. Citation2010), and other algorithms are introduced to improve the vertical resolution. (2) Parametric estimation method based on subspace fitting technique (Viberg Citation1990). Those methods need to know the number of scattering sources, divide the signal into noise subspace and signal subspace, and reconstruct the tomographic profile of the required signal by using the mathematical relationship of different subspaces, such as multiple signal classification (MUSIC) algorithm (Schmidt and Schmidt Citation1986), weighted subspace fitting (WSF) algorithm (Huang, Ferro-Famil, and Reigber Citation2011), etc. (3) Compressed Sensing (CS) (Budillon, Evangelista, and Schirinzi Citation2010; Li et al. Citation2015; Zhu and Bamler Citation2010; Aguilera, Nannini, and Reigber Citation2012, Citation2013). CS algorithm assumes that the forest signal obtained is sparse under the sparse basis. After selecting appropriate user parameters, convex optimization tools can be used to solve and reconstruct the tomographic profile. (4) Sum of Kronecker Product Decomposition (SKPD) (Tebaldini Citation2009). SKPD algorithm follows the assumption of two layers of the forest and applies the principle of algebraic geometry to separate the FP covariance matrix into the ground structure matrix and volume structure matrix. After that, any TomoSAR algorithm based on covariance matrix can be used to obtain the tomographic profile.
Whether using PolInSAR or TomoSAR technology to obtain the vertical structure of the forest, the forest height and the underlying topography have always been important parameters that are closely related to the AGB. As an emerging SAR technology, TomoSAR technology can offer resolution in the third dimension and detect ground objects, especially in forest areas. It is different from the traditional InSAR technology with two-dimensional focusing in the range-azimuth direction. In the case of multiple data stacks, it resynthesizes the aperture at the normal-to-slant-range direction, forming its unique perspective of observing ground objects. Furthermore, it can provide the scattering information of the penetrable natural medium in the form of continuous profiling along the height direction (see ). Therefore, TomoSAR technology has great potential in obtaining the three-dimensional structure of the forest and provides a powerful technical tool for the upcoming BIOMASS mission.
In this paper, we mainly discuss the performance of obtaining underlying topography and forest height by using the first three kinds of TomoSAR methods and analyze the influence of baseline design and filters on the reconstruction of the tomographic profile. SKPD algorithm must use FP SAR data, and it is difficult to determine the optimal parameters with different forests, which is very time-consuming. So, in this paper, we do not consider the SKPD algorithm. The article is arranged as follows: the second part mainly introduces the basic theory of the TomoSAR model and three kinds of methods; the third part mainly introduces the research area and the data processing; the fourth part mainly introduces the results of forest height and underlying topography obtained by the three methods. The final two parts show the discussion and the summary.
2. TomoSAR model and methods
2.1. TomoSAR model
The essence of SAR tomography is similar to single-snapshot DOA estimation in signal processing. The model estimated by TomoSAR can be expressed as follows (Fornaro, Lombardini, and Serafino Citation2005; Tebaldini and Guarnieri Citation2010):
where Y represents single look complex (SLC) data stack after registration and phase flattening, A represents the known steering matrix, X represents the unknown vertical reflectivity profile, and represents complex random Gaussian zero-mean additive noise vector. To clarify the meaning of the above parameters, we write them in matrix form as follows:
where subscript represents the numbers of height samples which is set to reconstruct the continuous tomographic profiles (i.e. a height range from −60 to 30 m with an interval of 1 m, N equals 91); superscript
represents the ith SLC image;
represents the potential source location associated with the position of the peaks of the tomographic profile;
is the vertical reflectivity profile response to the location
,
is the phase-to-height parameter associated with the pair formed by the ith acquisitions and the first acquisitions;
where , and R represent the vertical baseline, radar wavelength, incidence angle, and slant-range, respectively.
Since the continuous complex reflectivity profile cannot be accurately recovered, we can only use the discrete sampling method to approximate the true reflectivity. In fact, TomoSAR technology is interested in obtaining the backscattering power profile (i.e. the second-order statistics of the complex reflectivity, also named tomographic profile, for the consistent definition, hereinafter collectively referred to as tomographic profile) for a certain azimuth-range location,
where represents statistical expectation,
indicates temporal or spatial ensemble averaging,
represents the Hermitian operator. The tomographic profile consists of the diagonal elements of the P matrix. The TomoSAR methods in obtaining the forest tomography profile are mostly established based on the covariance matrix. Therefore, the accuracy of tomographic profile estimation by TomoSAR is closely related to the estimation accuracy of the covariance matrix. The following formula indicates the relationship between the tomographic profile and the covariance matrix:
where represents noise covariance matrix.
2.2. Capon algorithm
Capon algorithm is a typical non-parametric estimator based on the covariance matrix, expressed in the following manner,
where represents the obtained tomographic profile using Capon algorithm,
represents the inverse of the covariance matrix R. When the sampling height interval
is determined, the tomographic profile can be obtained by using the above formula.
2.3. MUSIC algorithm
MUSIC is a short name of the multiple signal classification algorithm. First, MUSIC algorithm needs to know the number of scattering sources , as the input parameter. Then, the covariance matrix R can be obtained from the observed SLC data stack and divided into noise subspace and signal subspace by the eigendecomposition tool.
where represents the lth eigenvalue corresponding to the eigenvector
;
represents the eigenvectors in the signal subspace;
represents the eigenvectors in the noise subspace;
represents the eigenvalues in the form of the diagonal matrix where
. Finally, using the orthogonal relationship between the steering matrix A and the eigenvector of the noise subspace and the equality transformation, the tomographic profile
expression is as follows.
2.4. CS algorithm
In recent years, the theory of CS has been widely applied in sparse signal recovery. SAR technology has also been successfully applied CS technology for building height extraction, four-dimensional urban deformation, and forest tomographic profile inversion. The forest tomographic profile can be reconstructed by CS algorithm with a limited amount of covariance samples under the following assumptions that (1) the signals must be sparse, indicating that there are a low number of non-zero coefficients in the reconstructed tomographic profile and (2) the steering matrix A must satisfy the restricted isometry property (RIP) criterion.
Based on the representation of CS theory, we rewrite the TomoSAR model into vector form
where represents the vec-operator which stacks the columns of a matrix;
,
represents Khatri – Rao product;
represents sparse basis matrix;
represents complex random Gaussian zero-mean additive noise;
represents the sparse forest signal associated with the tomographic profile.
, where
denotes getting the diagonal elements of the matrix.
According to the above equations,the core of tomographic profile estimation is solving the sparse signal . The solution of
can be simplified as the following constrained inequality minimization problem.
where is the hyperparameter determined by the user related to the noise level. In order to simplify the problem, this paper adopts the constraint of nonlinear inequality adopted in literature (Cazcarra-Bes et al. Citation2019) to solve the problem.
where and
represent mixed (2,1) and Frobenius norm, respectively.
and
are weighted parameters and can be set 2 and 0.5 in this article, respectively. This problem is a convex optimization problem and can be solved by the CVX toolkit (http://cvxr.com/cvx/download/).
3. Study area and data processing
3.1. Basic overview of SAR data
In this paper, airborne FP P-band SAR data of Lopè National Park, launched in Gabon, Africa, are used for validation. This test area is one of the four study areas in the biomass mission named “AfriSAR” implemented by European Space Agency (ESA) in 2016. The topography of this area varies greatly, with an average elevation of about 288 m and the mature stands of canopy height distributed between 30 and 50 m. Lopè park is characterized by a mosaic between grasslands and (dense) tropical forests, with a biomass ranging from about 50 to 600 t/ha. The dry season here is mainly concentrated from mid-June to mid-September, with an average annual rainfall of about 1440 mm/year (from 1984 to 2016) and a temperature range of 20–23°C. It has an average of 35 abundant tree species per ha, with a wide variation between savanna and forest. In the AfriSAR campaign, a total of 10 FP P-band acquisitions are obtained to study the TomoSAR technology in the rainforest. The interval of the spatial baseline is uniform. We can ignore the impact of temporal decoherence due to the short time interval between acquisitions. Refer for some specific SAR data parameters. Considering the purpose of the paper, we have selected a part of the area with a topography range between 200 and 300 m and a forest height between 0 and 50 m for the experiment. shows the RGB images based on Pauli basis in the UTM coordinate system, including the selected experimental area (azimuth:4500–6499 pixel; range:2200–3200 pixel).
Figure 2. The RGB composite image of Lopè park. The right is the selected experiment area in the red box on the left.

Table 1. The parameters of the FP P-band datasets.
3.2. LiDAR data
While acquiring airborne FP P-band SAR data, the LiDAR data are provided by the National Aeronautics and Space Administration (NASA), covering the same region. The lidar footprint on ground is around 20 m wide.The LiDAR data acquired by the LVIS system include level 1 products and level 2 products. Level 2 products include CHM and DEM derived from level 1 products. To be consistent with the selected experimental area, we crop the LiDAR data in the same range as shown in . While evaluating TomoSAR DEM and CHM, LiDAR data are geocoded to SAR coordinates for evaluation. For details of more parameters, please refer https://lvis.gsfc.nasa.gov/Data/Maps/Gabon2016Map.html
3.3. Data processing
After registered and phase flattening, the SLC images form the TomoSAR data stack, and the tomographic profile can be obtained using the above three methods. It should be noted that AfriSAR data use TanDEM-X data for phase flattening. Therefore, the topography containing canopy height is removed simultaneously in the phase flattening procedure.
Some literature supports that HH polarization is sensitive to double-bounce scattering, which often occurs between the branches and the ground, leading to the corresponding phase center being located on the ground level. The HV polarization is sensitive to volume scattering, which generally acts on the interior of the canopy (Arii, Van Zyl, and Kim Citation2010; Freeman Citation2007; Tebaldini and Rocca Citation2012). Due to the long-wavelength and strong penetrability of P-band SAR, the canopy phase center is usually lower than the real forest height. This phenomenon is represented in the tomographic profile, as shown in . Because of that, the HH data stack is used to obtain the underlying topography, while the HV data stack is used to get the forest height with the power loss method proposed in the literature (Tebaldini and Rocca Citation2012). The criterion of power loss is:
where represents the max peak position of the canopy phase center in tomographic profile; H represents the actual forest height;
represents the power loss value obtained by using a small amount of LiDAR sample data for data verification.
The primary data processing processes in this paper are summarized in . The processing chain of obtaining underlying topography and forest height is summarized as follows:
Select the SLC (after registration and phase flattening) images with certain baselines to form the TomoSAR data stack (i.e. EquationEquation (2)
(2)
(2) );
Select a suitable filter, such as hamming window filter, to estimate the covariance matrix R of the TomoSAR data stack (i.e. EquationEquation (5)
(5)
(5) );
Based on the known vertical wave number
, set the sampling height and calculate the steering matrix A (i.e. EquationEquation (2)
(2)
(2) );
Pixel-by-pixel calculation can be performed using the Capon (i.e. EquationEquation (6)
(6)
(6) ), MUSIC (i.e. EquationEquations (7)
(7)
(7) and (Equation8
(8)
(8) )), and CS (EquationEquations (9)
(9)
(9) –(Equation11
(11)
(11) )) algorithm mentioned in the above to obtain a tomographic profile;
Determine the underlying topography and the forest height based on the peak positions and power loss method with HH tomographic profile and HV tomographic profile.
When estimating the covariance matrix, a large but appropriate filtering window setting can effectively suppress the side lobes. In this paper, a 31 × 31 pixel window is used to evaluate the covariance matrix. In order to verify the results, this paper uses LiDAR DEM and LiDAR CHM as the comparative verification data. We evaluate TomoSAR DEM and TomoSAR CHM by 30 × 30 pixel window to avoid the deviation of the pixel-by-pixel assessment. When evaluating the TomoSAR CHM, we ignore the forest height less than 10 m, which is not statistically significant in the tropical rainforest.
The proposed evaluation index factors are root-mean-square error (RMSE) and relative error (△), and the calculation formulas are as follows:
where K denotes the number of the selected samples that are used to participate in the experimental verification.
4. Experimental result
As mentioned above, we can obtain the underlying topography from HH data and the forest height from HV data. For this purpose, we firstly focus on the ability of each algorithm to reconstruct the tomographic profile. We use three different algorithms for drawing the same profile corresponding to the selected white line (range index: 200) in . As a result, all of the following analyses correspond to the same profile with the top white dashed line is LiDAR CHM, and the bottom is LiDAR DEM. All of the following tomographic profiles are normalized 0 to 1.
4.1. The performance of tomographic profile reconstruction based on different algorithms
) is the tomographic profile of HH data and HV data based on Capon algorithm. The results show that the HV tomographic profile demonstrates the ability to obtain the canopy height, and its power peaks are located in the phase center of the canopy (slightly lower than the top white dashed line). HH data have also shown the potential to invert underlying topography. Although some regions have obvious two-layer power centers, the phase centers of the ground can be found out easily by some criteria (Pardini et al. Citation2018; D’Alessandro and Tebaldini Citation2019). Since the experimental area is a tropical rainforest with high forest and density, the double-bounce scattering occurs not only on the surface and branches but also between branches in the canopy. Therefore, there is also a power peak at the phase center of the canopy and the result shows that the HH data also contain a strong volume scattering contribution.
Figure 7. Normalized tomographic profile obtained by Capon algorithm (a)–(b) and CS algorithm (c)–(d).

The tomographic profile of CS algorithm is similar to Capon’s, which can obtain underlying topography by HH data and forest height by HV data, as shown in ). The difference is that CS algorithm needs to input hyper-parameter. With different forest types, changeable parameters may lead to different results.
The most interesting is the result of MUSIC algorithm. shows that the tomographic profile of HH and HV data obtained by MUSIC algorithm, with different numbers of scattering sources. By comparison, we find that the assumption that there are only two scattering sources is inappropriate in the rainforest. As shown in , the location of the maximum peak of the HH tomographic profile cannot be the ground phase center when the number of scattering sources equals two. From quantitative evaluation, we find that the best performance in inverting underlying topography by using MUSIC algorithm when the number of scattering sources equals four. When drawing the HV tomographic profile, the best parameter to obtain the phase center of the canopy equals two. When the number of input scattering sources is larger than two, the HV tomographic profile tends to have a peak at the ground, like the result of scattering sources equal four. Through quantitative analysis, we find that the highest accuracy of TomoSAR CHM is obtained only when the number of input scattering sources equals two.
Figure 8. Normalized HH and HV tomographic profile obtained by MUSIC algorithm with different numbers of scattering sources.

Tropical rainforest is complex, penetrable volume layer, and the acquired signal contains not only from the canopy and the ground but also from inside the forest structure. Radar wave penetration through the vegetation canopy to the ground is a power attenuation process, and the scattering process is complex and not easy to interpret. Therefore, the assumption of the forest as a two-layer structure consisting of two point-like scatterers is not universal in the MUSIC algorithm. Second, due to the specificity of the forest scene and the high density of tropical rainforest, even if HH is sensitive to the double-bounce scattering that occurs more often between the ground and the branches, such contributions still exist in the canopy. Therefore, the two scattering sources cannot get the optimal result, which also shows the uncertainty of MUSIC algorithm.
4.2. The results of underlying topography and forest height-based TomoSAR algorithms
The underlying topography and forest height obtained by Capon algorithm using HH data and HV data are shown in . Compared with the LiDAR DEM in , there is little difference between LiDAR DEM and TomoSAR DEM of Capon algorithm, with an RMSE of 1.58 m and a relative error of 1.1%. The reliability of the underlying topography obtained by using HH data sensitive to the double-bounce scattering is proved.
Figure 9. The estimated results of Capon algorithm: (a) underlying topography; (b) scatterplot of TomoSAR DEM validation; (c) forest height; (d) scatterplot of TomoSAR CHM validation.

In order to find the optimal power loss value in the tomographic profile by using a small amount of LiDAR CHM samples, we set the power loss as 0–4 dB with an interval is 0.5 dB and quantitatively evaluated the difference between the TomoSAR CHM and the LiDAR CHM. The results are shown in . The results show that the maximum peak location (the power loss is equal to 0) of the canopy phase center based on HV data is not the actual forest height, significantly lower than the LiDAR CHM, as shown in . That can be interpreted as the strong penetrability of the P-band leading to the canopy phase center being lower than the forest height. As power loss increases, we can see that the forest height is closer to the LiDAR CHM, and when power loss is more than the optimal value, there will be a significant overestimation error. We find that the power loss equals 2 dB, RMSE is the minimum, 2.17 m, and the relative error is 12.1%, as shown in ).
shows the estimated underlying topography and forest height by MUSIC algorithm. MUSIC algorithm is a parametric method that needs to input the number of scattering sources when estimating the tomographic profile. For forest scenarios, although the “two-layer” assumption of the RVoG model in PolInSAR technique is reasonable, the result is unsatisfactory when using two scattering sources in estimating the underlying topography by MUSIC algorithm. Compared with LiDAR DEM, we find that when the number of scattering sources equals four, the underlying topography is most close to LiDAR DEM, with 2.14 m of RMSE and 1.5% of the relative error. The obtained forest height by MUSIC algorithm is shown in ). The result shows that the RMSE is 2.79 m, and the relative error is 15.5%, which is very close to Capon’s products. It can be seen that both MUSIC algorithm and Capon algorithm can obtain approximately accurate DEM and CHM results compared with LiDAR data.
Figure 11. The estimated results of MUSIC algorithm: (a) underlying topography; (b) scatterplot of TomoSAR DEM validation; (c) forest height; (d) scatterplot of TomoSAR CHM validation.

The estimated underlying topography and forest height based on CS algorithm are shown in . It can be concluded from the results that, like Capon algorithm and MUSIC algorithm, the TomoSAR DEM is very close to LiDAR DEM based on HH data, with an RMSE is 1.86 m and a relative error is 1.3%. At the same time, the precision of the forest height is slightly lower than that of Capon algorithm, with an RMSE of 2.38 m and a relative error of 13.3%.
Figure 12. The results based on CS algorithm: (a) underlying topography; (b) scatterplot of TomoSAR DEM validation; (c) forest height; (d) scatterplot of TomoSAR CHM validation.

Although all algorithms can obtain high-precision underlying topography and forest height, the non-parametric Capon algorithm seems to perform the best among the three algorithms and has the advantage of no need for prior parameters. On the other hand, MUSIC algorithm needs to know the number of scattering sources, which may vary with different forest types. Moreover, CS algorithm is a convex optimization process, which is very time-consuming when using the CVX toolbox to solve the problem.
5. Analysis and discussion
In this paper, three typical TomoSAR methods are used to discuss and analyze forest height and underlying topography inversion in tropical forest area. It can be found from the results that all three algorithms can obtain precise underlying topography and forest height. Based on the algorithm principle, we know that the number of acquisitions and covariance matrix are crucial for reconstruction of tomographic profile. To further analyze the capability of tomographic profile reconstruction based on TomoSAR methods, we investigate the effects of different processing operations on tomographic inversion, such as baseline designs and filter methods. It can be seen from the above results that the correct canopy tomographic profile can be obtained using HV data among all three algorithms. Therefore, the results of HH data are mainly analyzed in the following. Due to Capon algorithm performs the best among three algorithms, only Capon algorithm is used for analysis and comparison to avoid redundancy and duplication of work in the experiment.
5.1. The influence of the baseline design
A total of ten acquisitions covering the Lopè national park in AfriSAR campaign are adopted in the experiment. Due to the uniform baseline interval, the influence of baseline interpolation on the inversion of forest height and underlying topography is not considered. The spatial baseline of ten acquisitions ranges from −80 to 80 m, supporting exploring the influence of different baseline designs on tomographic profile reconstruction, including the number of acquisitions, baseline order, and regularity of baseline distribution.
shows the results of the tomographic profile reconstruction with different number of available acquisitions. As can be seen from the results in the red dashed circle, the results of the tomographic profile reconstruction using the ten acquisitions () are significantly better than those using the six acquisitions (). The more acquisitions, the more redundant observations, which can provide a higher vertical resolution. To more obviously show the difference, we draw the evaluated DEM in the same plot, as shown in . The black line represents the LiDAR DEM, the green line represents the DEM obtained using the ten acquisitions, and the red line represents the DEM obtained using the six acquisitions. From the results, we can see many misjudgments in some areas due to the fewer acquisitions that cannot separate the ground contribution and the volume contribution.
Figure 13. Normalized tomographic profile obtained with different number of baseline: (a) ten acquisitions; (b) six acquisitions; (c) the estimated TomoSAR DEM profile compared with LiDAR DEM (N represents the number of baseline).

shows the obtained tomographic profile by using six acquisitions. shows the uniform distribution of the baseline (i.e. the selected images are No.02/03/04/05/06/07, corresponding to vertical baseline distribution:0/10/20/40/60/80), while shows the non-uniform distribution (i.e. the selected images are No.02/03/04/07/09/11, corresponding to vertical baseline distribution: 0/10/20/80/-40/-80). As shown from the part marked by red dotted circles, the tomographic profile with uniform baseline distribution is significantly better than those with the non-uniform. The non-uniform baseline cannot separate ground and volume contribution, revealing the strict requirements for baseline design in TomoSAR technology.
Figure 14. Normalized tomographic profile obtained by different baseline distribution: (a) uniform (the selected images areNo. 02/03/04/05/06/07); (b) non-uniform. (the selected images are No. 02/03/04/07/09/11).

illustrates the influence of baseline arrangement order on tomographic profile reconstruction with ten acquisitions. The results show that the disordered or ordered baselines arrangement does not affect reconstructing tomographic profile. The essence of tomographic profile reconstruction is to seek the eigenvalue of the covariance matrix (Stoica, Li, and Tan Citation2009). The elements in covariance are unchanged, and the baselines are not correlated with each other, so the eigenvalue of covariance is not changed eventually.
5.2. The effect of filters
Some literature has explored the application of different filters to accurately estimate the covariance matrix considering the heterogeneity of experimental scenarios and successfully applied them to detect the presence of weak scatterers in urban areas (D’Hondt et al. Citation2017; Aghababaei Citation2020). In this paper, we consider the heterogeneity of forest structure and analyze the effect of filter window size and different filters on tomographic profile reconstruction. shows the results of the tomographic profile with different Hamming window sizes. The results show that the filter window is small, the tomographic profile appears noisy with prominent sidelobe. The sidelobe decreases as the filter window size increases, but more details are lost. For the natural scene with heterogeneity, such as forest, a large filter window is often required to suppress the misjudgment of the sidelobe to obtain better statistical results of forest height and underlying topography.
At the same time, we also use different filters to evaluate the covariance matrix, including Boxcar filter, NL-SAR filter (Deledalle et al. Citation2014), and NDSAR-NLM filter (D’Hondt et al. Citation2017). The window size of the Boxcar filter is set to 31. The experimental results are shown in . From the results, the tomographic profile of the Boxcar filter is smooth with many details lost, and the contribution from the ground and canopy is not separated in many areas. The NL-SAR filter result fully considers the heterogeneity of the forest; however, that does not satisfy the assumption that the forest is a two-layer structure. We expect the peak locations to appear on the ground and the canopy. Obviously, the NL-SAR filter does not meet the requirement and is time-consuming. The results between the NDSAR-NLM filter and Hamming window filter are similar and perform the best among these filters. However, due to the need for continuous iterations and search processing, the NDSAR-NLM filter is very time-consuming. When evaluating the covariance matrix, we should consider the consistency of the pixels in the sliding window so that an accurate covariance matrix can be obtained. Whereas tropical forests are typically natural scenes with complex structures, unlike urban buildings, the signal from forests is random. So NDSAR-NLM filter has no outstanding advantages in the forest area of this paper.
6. Conclusion
TomoSAR technology is different from traditional two-dimensional SAR technology. By synthesizing aperture in the direction of cross-slant range, TomoSAR technology can obtain the tomographic profile that consists of power distribution with different heights and has real three-dimensional resolution ability. This paper assesses the performance of three typical TomoSAR algorithms for obtaining underlying topography and forest height in tropical forests. The results show that all algorithms can effectively retrieve these two products from the tomographic profile of HH and HV data. According to the scattering characteristics of the forest, we obtained the underlying topography by HH data and the forest height by HV data. We analyzed the performance of three typical super-resolution algorithms to reconstruct the tomographic profile. Furthermore, we discussed the effects of different baseline designs and filters on the tomographic profile reconstruction. The conclusions are summarized as follows:
All three algorithms can reconstruct the tomographic profile representing the ground or canopy. Capon algorithm performs well, and the RMSE of the forest height obtained from HV data and the underlying topography obtained from HH data is 2.17 m and 1.58 m, respectively.
Under the same conditions, the more acquisitions, the more uniform baselines distribution, and the better performance in reconstructing the tomographic profile.
Aim to obtain forest height and underlying topography, it is necessary to select the appropriate filter window size and filters. Smaller window size fails to suppress side lobes, and larger ones lose more details. In the experiment, Hamming window filter performs well and is recommended.
Disclosure statement
No potential conflict of interest was reported by the author(s).
Data availability statement
The data that support the findings of this study are available in the official website of European Space Agency [https://doi.org/10.1080/10095020.2022.2083985].These data were derived from the following resources available in the public domain: https://doi.org/10.1080/10095020.2022.2083985. A short proposal must be written and submitted to access this data.
Additional information
Funding
Notes on contributors
Chuanjun Wu
ChuanJun Wu is currently pursuing the PhD degree in Wuhan university. His research interests are SAR tomography for forest application, including inversion of forest height, underlying topography, and above ground biomass.
Xinwei Yang
XinWei Yang received his PhD degree from Wuhan university and currently works in State Key Laboratory of Remote-Sensing Science, Aerospace Information Research Institute, Chinese Academy of Sciences, Beijing. His research interests are SAR remote sensing in forest.
Yanghai Yu
YangHai Yu is currently pursuing the PhD degree in Wuhan university. His research interests are high-resolution SAR imaging.
Stefano Tebaldini
Stefano Tebaldini is an IEEE senior member and an associate Professor in Polytechnic University of Milan. His research is mostly focused on remote sensing of the Earth using Radar technology. His research activities include the development of new processing techniques for Radar imaging and calibration, as well as scientific investigations on the physical properties of natural media based on their interaction with EM waves.
Lu Zhang
Lu Zhang is a professor in Wuhan University. His research interests include synthetic aperture radar interferometry as well as remote-sensing classification and change detection.
Mingsheng Liao
Mingsheng Liao is a professor in Wuhan University. He has published more than 100 peer-reviewed journal papers and four books focused on synthetic aperture radar interferometry techniques and applications. His research interests include algorithms and application for interferometric synthetic aperture radar, remote-sensing image processing and analysis, and the integration and fusion of multisource spatial information.
References
- Aghababaei, H. 2020. “On the Assessment of Non-Local Multi-Looking in Detection of Persistent Scatterers Using SAR Tomography.” Remote Sensing 12 (19): 3195. doi:10.3390/rs12193195.
- Aghababaei, H., G. Ferraioli, L. Ferro-Famil, Y. Huang, M. Mariotti D’-Alessandro, V. Pascazio, G. Schirinzi, and Tebaldini, S. 2020. “Forest SAR Tomography: Principles and Applications.” IEEE Geoscience and Remote Sensing Magazine 8 (2): 30–45. doi:10.1109/MGRS.2019.2963093.
- Aguilera, E., M. Nannini, and A. Reigber. 2012. “A Data-Adaptive Compressed Sensing Approach to Polarimetric SAR Tomography of Forested Areas.” IEEE Geoscience and Remote Sensing Letters 10 (3): 543–547. doi:10.1109/LGRS.2012.2212693.
- Aguilera, E., M. Nannini, and A. Reigber. 2013. “Wavelet-Based Compressed Sensing for SAR Tomography of Forested Areas.” IEEE Transactions on Geoscience and Remote Sensing 51 (12): 5283–5295. doi:10.1109/TGRS.2012.2231081.
- Arii, M., J.J. Van Zyl, and Y.J. Kim. 2010. “Adaptive Model-Based Decomposition of Polarimetric SAR Covariance Matrices.” IEEE Transactions on Geoscience and Remote Sensing 49 (3): 1104–1113. doi:10.1109/TGRS.2010.2076285.
- Bohn, F.J., and A. Huth. 2017. “The Importance of Forest Structure to Biodiversity–productivity Relationships.” Royal Society Open Science 4 (1): 160521. doi:10.1098/rsos.160521.
- Budillon, A., A. Evangelista, and G. Schirinzi. 2010. “Three-Dimensional SAR Focusing from Multipass Signals Using Compressive Sampling.” IEEE Transactions on Geoscience and Remote Sensing 49 (1): 488–499. doi:10.1109/TGRS.2010.2054099.
- Cazcarra-Bes, V., M. Pardini, M. Tello, and K.P. Papathanassiou. 2019. “Comparison of Tomographic SAR Reflectivity Reconstruction Algorithms for Forest Applications at L-Band.” IEEE Transactions on Geoscience and Remote Sensing 58 (1): 147–164. doi:10.1109/TGRS.2019.2934347.
- Cheng, X.G., N. Pinto, and J.Y. Gong. 2012. “Terrain Radiometric Calibration of Airborne UAVSAR for Forested Area.” Geo-Spatial Information Science 15 (4): 229–240. doi:10.1080/10095020.2012.745050.
- Cloude, S.R., and K.P. Papathanassiou. 2003. “Three-Stage Inversion Process for Polarimetric SAR Interferometry.” IEE Proceedings-Radar, Sonar and Navigation 150 (3): 125–134. doi:10.1049/ip-rsn:20030449.
- D’-Alessandro, M.M., and S. Tebaldini. 2019. “Digital Terrain Model Retrieval in Tropical Forests Through P-Band SAR Tomography.” IEEE Transactions on Geoscience and Remote Sensing 57 (9): 6774–6781. doi:10.1109/TGRS.2019.2908517.
- D’-Hondt, O., C. Lopez-Martinez, S. Guillaso, and O. Hellwich. 2017. “Nonlocal Filtering Applied to 3-D Reconstruction of Tomographic SAR Data.” IEEE Transactions on Geoscience and Remote Sensing 56 (1): 272–285. doi:10.1109/TGRS.2017.2746420.
- Del Campo, G.M., M. Nannini, and A. Reigber. 2018. “Towards Feature Enhanced SAR Tomography: A Maximum-Likelihood Inspired Approach.” IEEE Geoscience and Remote Sensing Letters 15 (11): 1730–1734 doi:10.1109/LGRS.2018.2858571.
- Del Campo, G.M., M. Nannini, and A. Reigber. 2020. “Statistical Regularization for Enhanced TomoSar Imaging.” IEEE Journal of Selected Topics in Applied Earth Observations and Remote Sensing 13: 1567–1589. doi:10.1109/JSTARS.2020.2970595
- Deledalle, C.A., L. Denis, F. Tupin, A. Reigber, and M. Jäger. 2014. “NL-SAR: A Unified Nonlocal Framework for Resolution-Preserving (Pol)(in)sar Denoising.” IEEE Transactions on Geoscience and Remote Sensing 53 (4): 2021–2038. doi:10.1109/TGRS.2014.2352555.
- Fornaro, G., F. Lombardini, and F. Serafino. 2005. “Three-Dimensional Multipass SAR Focusing: Experiments with Long-Term Spaceborne Data.” IEEE Transactions on Geoscience and Remote Sensing 43 (4): 702–714. doi:10.1109/TGRS.2005.843567.
- Freeman, A. 2007. “Fitting a Two-Component Scattering Model to Polarimetric SAR Data from Forests.” IEEE Transactions on Geoscience and Remote Sensing 45 (8): 2583–2592. doi:10.1109/TGRS.2007.897929.
- Huang, Y., L. Ferro-Famil, and A. Reigber. 2011. “Under-Foliage Object Imaging Using SAR Tomography and Polarimetric Spectral Estimators.” IEEE Transactions on Geoscience and Remote Sensing 50 (6): 2213–2225. doi:10.1109/TGRS.2011.2171494.
- Kugler, F., S.K. Lee, I. Hajnsek, and K.P. Papathanassiou. 2015. “Forest Height Estimation by Means of Pol-InSar Data Inversion: The Role of the Vertical Wavenumber.” IEEE Transactions on Geoscience and Remote Sensing 53 (10): 5294–5311. doi:10.1109/TGRS.2015.2420996.
- Lee, J.S., and E. Pottier. 2017. Polarimetric Radar Imaging: From Basics to Applications. Boca Raton: CRC press.
- Li, X.W., L. Liang, H.D. Guo, and Y. Huang. 2015. “Compressive Sensing for Multibaseline Polarimetric SAR Tomography of Forested Areas.” IEEE Transactions on Geoscience and Remote Sensing 54 (1): 153–166. doi:10.1109/TGRS.2015.2451992.
- Mitchard, E.T.A. 2018. “The Tropical Forest Carbon Cycle and Climate Change.” Nature 559 (7715): 527–534. doi:10.1038/s41586-018-0300-2.
- Neumann, M., L. Ferro-Famil, and A. Reigber. 2008. “Multibaseline Polarimetric SAR Interferometry Coherence Optimization.” IEEE Geoscience and Remote Sensing Letters 5 (1): 93–97. doi:10.1109/LGRS.2007.908885.
- Papathanassiou, K.P., and S.R. Cloude. 2001. “Single-Baseline Polarimetric SAR Interferometry.” IEEE Transactions on Geoscience and Remote Sensing 39 (11): 2352–2363. doi:10.1109/36.964971.
- Pardini, M., M. Tello, V. Cazcarra-Bes, K.P. Papathanassiou, and I. Hajnsek. 2018. “L-And P-Band 3-D SAR Reflectivity Profiles versus Lidar Waveforms: The AfriSar Case.” IEEE Journal of Selected Topics in Applied Earth Observations and Remote Sensing 11 (10): 3386–3401. doi:10.1109/JSTARS.2018.2847033.
- Peng, X., X.W. Li, C.C. Wang, H.Q. Fu, and Y.N. Du. 2018. “A Maximum Likelihood Based Nonparametric Iterative Adaptive Method of Synthetic Aperture Radar Tomography and Its Application for Estimating Underlying Topography and Forest Height.” Sensors 18 (8): 2459. doi:10.3390/s18082459.
- Ramli, M.F., and K.N. Tahar. 2020. “Homogeneous Tree Height Derivation from Tree Crown Delineation Using Seeded Region Growing (SRG) Segmentation.” Geo-Spatial Information Science 23 (3): 195–208. doi:10.1080/10095020.2020.1805366.
- Reigber, A., and A. Moreira. 2000. “First Demonstration of Airborne SAR Tomography Using Multibaseline L-Band Data.” IEEE Transactions on Geoscience and Remote Sensing 38 (5): 2142–2152. doi:10.1109/36.868873.
- Roberts, W., P. Stoica, J. Li, T. Yardibi, and F.A. Sadjadi. 2010. “Iterative Adaptive Approaches to MIMO Radar Imaging.” IEEE Journal of Selected Topics in Signal Processing 4 (1): 5–20. doi:10.1109/JSTSP.2009.2038964.
- Schmidt, R., and R.O. Schmidt. 1986. “Multiple Emitter Location and Signal Parameter Estimation.” IEEE Transactions on Antennas and Propagation 34 (3): 276–280. doi:10.1109/TAP.1986.1143830.
- Spies, T.A. 1998. “Forest Structure: A Key to the Ecosystem.” Northwest Science 72: 34–39.
- Stoica, P., J. Li, and X. Tan. 2009. “Spatial Power Spectrum Estimation Using the Pisarenko Framework.” IEEE Transactions on Signal Processing 56 (10): 5109–5119. doi:10.1109/TSP.2008.928935.
- Stoica, P., P. Babu, and J. Li. 2010. “SPICE: A Sparse Covariance-Based Estimation Method for Array Processing.” IEEE Transactions on Signal Processing 59 (2): 629–638. doi:10.1109/TSP.2010.2090525.
- Tebaldini, S. 2009. “Algebraic Synthesis of Forest Scenarios from Multibaseline PolInsar Data.” IEEE Transactions on Geoscience and Remote Sensing 47 (12): 4132–4142. doi:10.1109/TGRS.2009.2023785.
- Tebaldini, S., and A.M. Guarnieri. 2010. “On the Role of Phase Stability in SAR Multibaseline Applications.” IEEE Transactions on Geoscience and Remote Sensing 48 (7): 2953–2966. doi:10.1109/TGRS.2010.2043738.
- Tebaldini, S., and F. Rocca. 2012. “Multibaseline Polarimetric SAR Tomography of a Boreal Forest at P- and L-Bands.” IEEE Transactions on Geoscience and Remote Sensing 50 (1): 232–246. doi:10.1109/TGRS.2011.2159614.
- Treuhaft, R.N., M. Moghaddam, and J.J. van Zyl. 1996. “Vegetation Characteristics and Underlying Topography from Interferometric Radar.” Radio Science 31 (6): 1449–1485. doi:10.1029/96RS01763.
- Viberg, M. 1990. “Subspace Fitting Concepts in Sensor Array Processing.” Signal Processing 19 (4): 345. doi:10.1016/0165-1684(90)90165-U.
- Wu, C.J., C.C. Wang, P. Shen, J.J. Zhu, H.Q. Fu, and H. Gao. 2019. “Forest Height Estimation Using PolInsar Optimal Normal Matrix Constraint and Cross-Iteration Method.” IEEE Geoscience and Remote Sensing Letters 16 (8): 1245–1249. doi:10.1109/LGRS.2019.2895869.
- Yardibi, T., L. Jian, P. Stoica, X. Ming, and A.B. Baggeroer. 2010. “Source Localization and Sensing: A Nonparametric Iterative Adaptive Approach Based on Weighted Least Squares.” IEEE Transactions on Aerospace and Electronic Systems 46 (1): 425–443. doi:10.1109/TAES.2010.5417172.
- Yu, Y.H., M.M. D’-Alessandro, S. Tebaldini, and M.S.L. And. 2020. “Signal Processing Options for High Resolution SAR Tomography of Natural Scenarios.” Remote Sensing 12 (10): 1638. doi:10.3390/rs12101638.
- Zhang, G., S. Ganguly, R.R. Nemani, M.A. White, C. Milesi, H. Hashimoto, W. Wang, Saatchi, S., Yu, Y., and Myneni, R.B. 2014. ”Estimation of Forest Aboveground Biomass in California Using Canopy Height and Leaf Area Index Estimated from Satellite Data”. Remote Sensing of Environment 151: 44–56. doi:10.1016/j.rse.2014.01.025.
- Zhu, X.X., and R. Bamler. 2010. “Tomographic SAR Inversion by L1 Norm Regularization – the Compressive Sensing Approach.” IEEE Transactions on Geoscience and Remote Sensing 48 (10): 3839–3846. doi:10.1109/TGRS.2010.2048117.