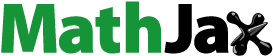
ABSTRACT
Poverty has always been a global concern that has restricted human development. The first goal (SDG 1) of the United Nations Sustainable Development Goals (SDGs) is to eliminate all forms of poverty all over the world. The establishment of a scientific and effective localized SDG 1 evaluation and monitoring method is the key to achieving SDG 1. This paper proposes SDG 1 China district and county-level localization evaluation method based on multi-source remote sensing data for the United Nations Sustainable Development Framework. The temporal and spatial distribution characteristics of China’s poverty areas and their SDG 1 evaluation values in 2012, 2014, 2016, and 2018 have been analyzed. Based on the SDGs global indicator framework, this paper first constructed SDG 1 China’s district and county localization indicator system and then extracted multidimensional feature factors from nighttime light images, land cover data, and digital elevation model data. Secondly, we establish SDG 1 China’s localized partial least squares estimation model and SDG 1 China’s localized machine learning estimation model. Finally, we analyze and verify the spatiotemporal distribution characteristics of China’s poverty areas and counties and their SDG 1 evaluation values. The results show that SDG 1 China’s district and county localization indicator system proposed in this study and SDG 1 China’s localized partial least squares estimation model can better reflect the poverty level of China’s districts and counties. The estimated model R2 is 0.65, which can identify 72.77% of China’s national poverty counties. From 2012 to 2018, the spatial distribution pattern of SDG evaluation values in China’s districts and counties is that the SDG evaluation values gradually increase from western China to eastern China. In addition, the average SDG 1 evaluation value of China’s districts and counties increased by 23% from 2012 to 2018. This paper is oriented to the United Nations SDGs framework, explores the SDG 1 localized evaluation method of China’s districts and counties based on multisource remote sensing data, and provides a scientific and rapid regional poverty monitoring and evaluation program for the implementation of the 2030 agenda poverty alleviation goals.
1. Introduction
For a long time, poverty has been a complex social problem that hinders the sustainable development of human society. Countries all over the world are working hard to eliminate poverty in order to achieve the sustainable development of human society (Guo, Zhou, and Liu Citation2019). To take into account the three aspects of sustainable development, society, economy, and environment, on 25 September 2015, the United Nations Sustainable Development Summit adopted “Change Our World: 2030 Agenda for Sustainable Development” and established the United Nations Sustainable Development Goals (SDGs), a total of 17 goals, of which the first goal (SDG 1) is to eliminate all forms of poverty in the world (Reid et al. Citation2017; Allen, Metternicht, and Wiedmann Citation2018; Janoušková, Hák, and Moldan Citation2018; Rosati and Faria Citation2019; Menton et al. Citation2020; Pizzi et al. Citation2020). The Agenda has poverty eradication as its first sustainable development goal and hopes that we can be the first generation to successfully eradicate poverty worldwide. The agenda points out that eliminating poverty in all forms and manifestations is the world’s greatest challenge and an indispensable requirement for achieving sustainable development.
To promote the implementation of the 2030 Agenda for Sustainable Development, the United Nations established an SDGs global indicator framework containing 232 indicators in 2017, of which SDG 1 has 13 indicators. The SDGs global indicator framework is a universally applicable and guiding SDGs indicator system proposed to the world. It provides countries with a unified SDGs evaluation method (Dizdaroglu Citation2017). However, in some regions, there are no established methods and monitorable indicators in the SDGs global indicator framework, and developing a country-specific SDGs indicator system based on a country’s national conditions is a key challenge in implementing SDGs (Moreno Pires, Fidélis, and Ramos Citation2014; Giles-Corti, Lowe, and Arundel Citation2020). The Agenda also proposes to adapt to national circumstances, capabilities, needs, and priorities of countries to achieve the SDGs. Designing a country-specific indicator system for SDG 1 in the United Nations Sustainable Development Framework is a key part of monitoring the progress toward eradicating poverty (Mori and Christodoulou Citation2012; Khalid, Sharma, and Dubey Citation2018; Dickens et al. Citation2019; Fisher and Fukuda-Parr Citation2019; Xu et al. Citation2019). At present, many studies have proposed a series of poverty indicator systems based on the concept of poverty (Caizhen Citation2010; Cohen Citation2010; Wang, Cheng, and Zhang Citation2012; Khan et al. Citation2014; Yuan et al. Citation2018; Ma et al. Citation2019; Niu, Chen, and Yuan Citation2020; Shi et al. Citation2020). In general, scholars believe that poverty is multidimensional, and it is necessary to measure poverty in terms of income, ability, rights, etc. Some scholars choose to construct a poverty evaluation indicator system based on a mixed urban-rural administrative division (Wang, Cheng, and Zhang Citation2012; Khan et al. Citation2014; Shi et al. Citation2020). Wang, Cheng, and Zhang (Citation2012) selected 17 socioeconomic aspects of health care, education, income, and living area to establish a comprehensive poverty index to evaluate the poverty level of China at the provincial level. Rural areas are areas where the poor are concentrated. Some scholars build a rural poverty evaluation indicator system based on the characteristics of rural social life. The indicators of the system, such as agricultural mechanization and agricultural technology penetration rate, can better reflect the current situation of rural poverty (Caizhen Citation2010; Cohen Citation2010; Ma et al. Citation2019). At the same time, some scholars constructed an urban poverty evaluation indicator system based on the characteristics of urban social life (Yuan et al. Citation2018; Niu, Chen, and Yuan Citation2020). Niu, Chen, and Yuan (Citation2020) analyzed the urban poverty distribution in Guangzhou, China, from the aspects of housing, infrastructure services, and building structure. However, how to construct a poverty indicator system at the district/county scale for SDGs in line with China’s national conditions still needs further research.
Existing studies rely more on statistical data for poverty monitoring (Liu, Yu, and Wang Citation2015; Hossain et al. Citation2016; Ren et al. Citation2018; Antoniades, Widiarto, and Antonarakis Citation2019; Biggeri et al. Citation2019; Heimann Citation2019; Vanham et al. Citation2019; Echendu Citation2020). Antoniades, Widiarto, and Antonarakis (Citation2019) analyzed the impact of the financial crisis on the process of poverty eradication using a multidimensional poverty indicator system and statistical data. Based on statistical data, it is possible to completely calculate and obtain the degree of regional poverty in accordance with the constructed poverty indicator system. However, it is not only difficult to ensure the completeness of statistical data at the district/county scale in China but also the statistical standards of different districts and counties are different, which makes China’s district and county SDG 1 evaluation and monitoring difficult to achieve. Geographic data can reflect the details of human social activities, thereby monitoring regional socio-economic development. Geographic data have the advantages of long time series, large scope, and unified standards and can quickly monitor the characteristics of human social activities at a low cost. Therefore, it is widely used in social development, economic level, and environmental monitoring (Lu and Liu Citation2014; Liang et al. Citation2020; Wang et al. Citation2021b). Some scholars suggest that we should pay attention to the use of geographic data in sustainable development (Molla et al. Citation2017; Geng et al. Citation2018; Holloway and Mengersen Citation2018; Liverman Citation2018; Solís et al. Citation2018; Avtar et al. Citation2019; Liu et al. Citation2019a; Avtar et al. Citation2020; Chen et al. Citation2020a; Giuliani et al. Citation2020; Im Citation2020; Wu et al. Citation2020). Liu et al. (Citation2019a) believe that geographic data will become an important data source for sustainable development monitoring in the future. Avtar et al. (Citation2019) pointed out that geospatial data are an effective tool for monitoring the implementation of SDGs and formulating sustainable development plans for the future.
There are also many current studies applying geographic data to sustainable development evaluation and monitoring practices, including poverty monitoring (Yu et al. Citation2015; Steele et al. Citation2017; Vaziri et al. Citation2018; Li et al. Citation2019b; Zhao et al. Citation2019; Niu, Chen, and Yuan Citation2020; Wang et al. Citation2021a; Xu, Mo, and Zhu Citation2021b), per capita building volume (Ghosh et al. Citation2020), sustainable protection and utilization of terrestrial ecosystems (Liu, Bai, and Chen Citation2019b), water migration (Nagabhatla and Brahmbhatt Citation2020), urbanization (Ghasemkhani et al. Citation2020), agricultural development (Niu et al. Citation2021), and land planning (Chen et al. Citation2020b). Poverty is a complex social phenomenon that can be reflected in many aspects. Many poverty studies based on geographic data have selected socioeconomic, accessibility, and surface coverage to study the distribution of regional poverty (Xu et al. Citation2021a). Geographic data mainly include nighttime light images, land cover, and road network data. Zhao et al. (Citation2019) extracted information from geographic data such as nighttime light images, road network data, land cover data, and Google images to estimate the poverty level in Bangladesh; Yu et al. (Citation2015) used NPP-VIIRS information contained in the nighttime light images effectively to analyze the current poverty situation in China’s counties; Steele et al. (Citation2017) used mobile phone data and satellite imagery to analyze the poverty distribution in Bangladesh; Xu, Mo, and Zhu (Citation2021b) used geographic data such as nighttime light images and land cover data to analyze the poverty distribution in some contiguous special slum areas in China.
Therefore, to address the challenges of rapid poverty monitoring and evaluation in large-scale Chinese districts and counties, such as difficulty or lack of traditional statistical data acquisition, scientific advancement of the SDG 1 process, and continuous national rural revitalization, this paper designs and establishes an SDG 1 China’s district and county localization indicator system for SDGs. Combined with multisource geographic data, the SDG 1 China localization estimation model is constructed to provide fast and accurate SDG 1 quantitative monitoring and evaluation methods for China’s districts and counties, and it can also provide a reference for China’s medium- and long-term sustainable development planning.
2. Materials and methods
2.1. Research area
The research area of this paper is 2,838 districts and county-level administrative regions in China. In 2014, the Chinese government announced the list of National Poverty Counties (NPCs, 832 districts and counties in total) (). Poverty has plagued China for a long time and has severely restricted the sustainable development of Chinese society. In 2012, based on the absolute poverty level below a per capita annual net income of 2,300 set by the Chinese government, there were 98.99 million people in absolute poverty in China, which is a poverty incidence rate of 10.20%. After the continuous implementation of China’s poverty alleviation policies in recent years, China’s poverty eradication has been a great success. On 3 December 2020, at the Standing Committee of the Political Bureau of the CPC Central Committee, the Chinese government announced the elimination of absolute poverty. After that, the Chinese government proposed shifting the focus of poverty alleviation work to the comprehensive implementation of the rural revitalization strategy to achieve balanced urban and rural development. SDG 1 proposes to eliminate absolute poverty by 2030. In “Poverty: A Study of Town Life”, S. Rowntree believes that income cannot meet the material conditions necessary for survival in absolute poverty. SDG 1 believes that the measure of absolute poverty is that the daily living cost of each person is less than US$1.25, and the Chinese government stipulates that the annual income of less than 4,000 RMB is absolute poverty. The SDG 1 China localization evaluation method proposed in this paper can realize long-term monitoring of the implementation of SDG 1 in China’s districts and counties and can also provide references for China’s rural revitalization strategy and regional balanced development.
2.2. Data sources
The experimental data in this paper include Shuttle Radar Topography Mission (SRTM) Digital Elevation Model (DEM) data, NPP-VIIRS-like nighttime light data, land cover data, and socio-economic statistics.
(1) SRTM DEM data
The DEM data of the experimental area are derived from the US SRTM dataset, which is based on the most recent acquisition (SRTM V4.1) and created by collating and splicing DEM data at a resolution of 250 meters (). Topography is an important natural factor that affects socio-economic development and that is somewhat related to the distributed generation of poverty (Zhou et al. Citation2017; Tian et al. Citation2018). Natural topography can directly affect the construction of transportation networks, logistics trade, survival conditions and so on, which are closely related to poverty.
(2) NPP-VIIRS-like nighttime light data
Nighttime light data is sourced from an extended time series (2000–2018) of global NPP-VIIRS-like nighttime light data generated by Chen et al. (Citation2021) (). Chen et al. (Citation2021) built an extended time series (2000–2018) of NPP-VIIRS-like nighttime light data through a new cross-sensor calibration from DMSP-OLS nighttime light data (2000–2012) and a composition of monthly NPP-VIIRS nighttime light data (2013–2018). The NPP-VIIRS-like nighttime light data have an excellent spatial pattern and temporal consistency, which are similar to the composited NPP-VIIRS nighttime light data (Chen et al. Citation2021). Nighttime light can reflect the level of economic development of the region and is widely transported to the inversion of socioeconomic parameters (Lopez-Ruiz, Blazquez, and Hasanov Citation2019; Lin and Xu Citation2020).
(3) Land cover data
The land cover data are obtained from the European Space Agency (ESA) Climate Change Initiative (CCI) project. The land cover data include data on 22 types of land, including urban land, water bodies, and grassland, with a resolution of 300 meters. We integrated land cover types into six categories: urban, cropland, vegetation, bare areas, permanent snow and ice, and water (). This research uses mainly urban land areas to represent construction land. Land cover data can reflect the direction and intensity of land use in a region, and different land-use structures can reflect different landscape compositions (Arowolo and Deng Citation2017; Simwanda and Murayama Citation2018).
(4) Socio-economic statistics
The social and economic statistics of the experimental area are derived from 2012, 2014, 2016, and 2018 Statistical Yearbooks of Prefecture-level Cities in China and the National Economic and Social Development Statistical Communiques of Districts and Counties. These statistics mainly include the incidence of poverty, the number of urban and rural residents with minimum living allowances, the disposable income of urban and rural residents, general public budget expenditures, and the number of registered residents. Due to the difficulty of obtaining social and economic statistics in some districts and counties, 116 districts and counties with complete data for model construction and accuracy verification were selected for this research.
2.3. Research methods
This paper establishes SDG 1 China’s district and county localization indicator system for the SDGs global indicator framework and combines the multisource geographic data to construct the SDG 1 China localization estimation model. Finally, it analyses the spatial distribution pattern of the SDG 1 evaluation value of China’s districts and counties. The specific process is shown in .
2.3.1. SDG 1 China’s district and county localization indicator system
To promote the implementation of SDG 1 in China, this paper combines China’s social development stage and statistical data acquisition to design and construct SDG 1 China’s district and county localization indicator system. The SDGs global indicator framework proposes 13 indicators for the design of the seven sub targets of SDG 1. The report “2018 China SDGs Index Construction and Progress Evaluation Report” jointly completed by the China Academy of Environmental Planning and the World Wide Fund for Nature proposes that there are only seven indicators in the SDGs global indicator framework that have established methods and can be monitored (Wang et al. Citation2021a). Based on the SDGs global indicator framework, the “2018 China SDGs Index Construction and Progress Evaluation Report” builds an SDG 1 China’s localization indicator system with six indicators (Wang et al. Citation2021a). This paper is oriented to the SDGs global indicator framework, and considers China’s national conditions and data continuity issues. Based on the selection of the seven SDG 1 indicators in the SDGs global indicator framework, three indicators that can better reflect the poverty level of China’s districts and counties are added ().
Table 1. SDG 1 China’s district and county localization indicator system.
SDG 1 China’s district and county localization indicator system is oriented to the SDGs global indicator framework, where indicator 1 corresponds to SDG 1’s indicators 1 and 2 in the SDGs global indicator framework, and indicators 2 and 3 correspond to SDG 1’s indicators 3 and 4 in the SDGs global indicator framework. Since it is difficult to collect data on indicators 5, 6, and 7 of SDG 1 in the SDGs global indicator framework of China’s districts and counties, this paper adds three items: the disposable income of urban and rural residents and the balance of savings deposits per capita to SDG 1 China’s district and county localization indicator system. SDG 1 China’s district and county localization indicator system can better reflect the living standards of urban and rural residents and strengthen its ability of it to reflect the poverty level of China’s districts and counties. In addition, SDG 1 China’s district and county localization indicator system can fully consider the seven sub goals of SDG 1.
After constructing SDG 1 China’s district and county localization indicator system, it is necessary to standardize the indicators and assign weights to obtain the true SDG 1 evaluation value. The index data of different magnitudes are uniformly transformed into the same magnitude to ensure the comparability of the index data. In view of the uncertain value range of the SDG 1 Chinese localized index data, this paper uses the Z score standardization method to convert the index data into the same magnitude. The methods for determining weights can be divided into three categories: subjective methods, objective methods, and combined subjective and objective methods. Due to the relatively rapid social and economic development in China from 2012 to 2018, it is difficult for subjective components to obtain a scientific and reasonable indicator weight from the six years of change. The importance of indicators cannot be evaluated well, so subjective components may make the SDG 1 evaluation value not a good reflection of the poverty level of China’s districts and counties. Therefore, this paper chooses the entropy method as the objective method to determine the weights of SDG 1 Chinese localization indicators (). The entropy method only relies on the information of the median dispersion of the index data to determine the index weights. The greater the dispersion of the indicators, the greater the index weight. The greater the impact of the indicator on the evaluation results of poverty in China’s districts and counties, that is, the greater the weight of the indicator, the better the objective information of the indicator data itself. Therefore, it is more objective to determine the weight of SDG 1 China’s localization index. The specific steps to determine the index weight are shown in EquationEquations (1)(1)
(1) –(Equation5
(5)
(5) ).
Table 2. Localized SDG 1 index weight for districts and counties.
where is the value of the j-th index of district i, and
is the value of the j-th index of district i after standardization, and min
and max
are the minimum and maximum values of the j-th index in all districts and counties.
where is the index proportion of the j-th index of district i, and m is the number of districts and counties.
where is the index information entropy of the j-th index.
where is the information entropy redundancy of the
-th index.
where is the weight of the
-th index, and
is the number of indexes.
After standardization and weight assignment processing, the true SDG 1 evaluation value of 116 districts and counties in China can be obtained through EquationEquation (6)(6)
(6) . The lower the SDG 1 evaluation value is, the deeper the poverty level of the district and county.
where is the true SDG 1 evaluation value of China’s districts and counties based on SDG 1 of China’s district and county localization indicator system,
is the weight value of the
-th index, and
is the
-th index after standardization.
This paper collected index data for 37 districts and counties in 2014, including 21 NPCs and 16 non-NPCs. This paper calculates the SDG 1 evaluation values of 37 districts and counties based on SDG 1 China’s district and county localization indicator system and the indicator system constructed by Wang’s et al. (Citation2021a) research. These 37 districts and counties were ranked based on the SDG 1 evaluation value and show whether they are NPCs (). The results show that the two indicator systems can reflect the poverty situation of China’s districts and counties as a whole. However, SDG 1 China’s localization indicator system for districts and counties proposed in this paper better reflects China’s poverty. Therefore, this paper finally selects the incidence of poverty, the proportion of urban residents with minimum living security in the urban population, the proportion of rural residents with minimum living security in the rural population, the disposable income of urban residents, the disposable income of rural residents and the per capita household savings deposit balance.
Figure 4. SDG 1 evaluation value ranking of some districts and counties in China in 2014. (a) and (b) are calculated based on SDG 1 China’s district and county localization indicator system proposed in this study and by Wang et al. (Citation2021a) research, respectively. If the rank of the district/county is lower, it means that the SDG 1 evaluation value of the district/county is lower.

2.3.2. SDG 1 China’s localized partial least squares estimation model
This paper selects the four aspects of topography, land cover, social economy and urban accessibility to analyze the distribution of poverty in China’s districts and counties. Topography is an important natural factor that affects the socio-economic development of a region. Complex topographic conditions will increase the cost of regional development. Topography will affect the distribution of poverty in the region. Surface cover can describe the composition of the surface landscape of the area and reflect the direction of land use and the intensity of development. The social economy can reflect the active degree of regional economic exchanges. Generally, the higher the socioeconomic level is, the more it can promote employment and eliminate poverty. Urban accessibility reflects the convenience of the place of residence to reach the town because most of the infrastructure, such as education, medical care, and shopping malls, are located in the town, so areas with high urban accessibility can enjoy more infrastructure services.
DEM data can reflect the topography and surface morphology with certain accuracy and can derive surface morphological features such as slope and topographic undulation from it. DEM data can be selected as the topography and geomorphology data source. Land cover data can quantitatively describe the difference in the area of different types of land, such as the proportion of forest land area. Land cover data can be selected as the data source for land cover. At the same time, it can extract urban land information from land cover data, and it can also be used as a data source for urban accessibility. Nighttime light images can explore the spatial details of the economy and society and realize long-term monitoring of the temporal and spatial changes in human activity intensity (Xu, Mo, and Zhu Citation2021b). Nighttime light images are selected as the socioeconomic data source. The characteristic factors extracted from the four aspects of topography, land cover, social economy, and urban accessibility are shown in . The feature factors extracted from DEM data can reflect the difficulty of SDG 1 realization. The feature factors extracted from land cover data can detect the infrastructure conditions to achieve SDG 1. And the feature factors extracted from nighttime light images can monitor the realization of SDG 1 to a certain extent.
Table 3. Multidimension feature factors based on geographic data.
This paper selects the partial least squares method to construct the SDG 1 Chinese localization estimation model as Model I. The partial least squares method solves the problem of multicollinearity of feature factors and can obtain the importance of each feature factor (Guven and Samkar Citation2018; Liu, Peng, and Wang Citation2018). SDG 1 China’s localized partial least squares estimation model is as follows:
where is the estimated value based on SDG 1 China’s localized partial least squares estimation model;
is the regression coefficient;
is the regression constant; and
is the
-th principal component extracted from the feature factor;
is the model error term.
The principal components of the characteristic factors are independent of each other, which eliminates the multicollinearity of the characteristic factors to a certain extent and carries the information of the characteristic factors as much as possible (Afanador, Tran, and Buydens Citation2013), and the selected principal components are correlated with the real SDG 1 evaluation value as much as possible.
In addition, the partial least squares method will also provide the importance of each characteristic factor to the model estimation result (Chong and Jun Citation2005), and the importance equation of the characteristic factor is as follows:
where is the importance of feature factor
;
is the number of feature factors participating in model construction;
is the number of model iteration calculations, which is also the number of principal components; and
is the weight used by feature factor
when extracting principal component
, that is, the degree of interpretation of principal component
by feature factor
.
the degree of interpretation of the principal component
to
.
Since the square mean of the VIP values of all feature factors is equal to 1, the feature factors with VIP values greater than 1 are considered to be important variables. In addition to using 12 feature factors to build SDG 1 China’s localized partial least squares estimation model, this paper will select some of the more important feature factors to build the model and compares the estimation accuracy of the two models to determine the selection of feature factors.
2.3.3. SDG 1 China’s localized machine learning estimation model
In the absence of a definitive SDG 1 Chinese localized estimation model, machine learning regression methods can analyze and extract key feature information in known datasets, inspire complex correlations between search data, and repeatedly train and verify a large number of samples (Li et al. Citation2019a; Alsharkawi et al. Citation2021). Machine learning can also conduct precise simulation and prediction to build SDG 1 China’s localized machine learning estimation model. Based on the existing statistical data of 116 Chinese districts and counties and the feature factor data of geographic data, this paper constructs four SDG 1 China’s localized machine learning estimation models of regression tree, support vector machine, Gaussian process regression, and random forest.
In this paper, the four SDG 1 China’s localized machine learning estimation models are adjusted for multiple sample training and the model accuracy is tested using the fivefold cross-validation method. Finally, the four SDG 1 China’s localized machine learning estimation models have the highest Model parameters for the goodness of fit. Among them, the parameters of the regression tree and random forest model are the minimum leaf sizes, which are 12 and 8, respectively; the parameters of the support vector machine and Gaussian process regression model are kernel functions, which are exponential and Gaussian kernel functions, respectively ().
Table 4. Important parameters of the machine learning model.
3. Results
3.1. Index selection and model accuracy verification
Based on constructing the SDG 1 China’s localized partial least squares estimation model of 12 characteristic factors, this paper selects the characteristic factors according to the importance of the characteristic factors provided by the partial least square method () and performs multiple models. After training, six feature factors with importance greater than or close to 1 are selected to construct SDG 1 China’s localized partial least squares estimation model as Model II. The six feature factors are urban land area, average elevation, proportion of Nighttime Light (NTL) area, average slope, average undulation, and average NTL.
The estimation effect of the SDG 1 Chinese localization estimation model based on the partial least squares method and machine learning is shown in . This paper uses five-fold cross-validation to test the machine learning estimation model, and at the same time performs the F test for the partial least squares estimation model, and passes the 1% significance test. The results show that SDG 1 China’s localized partial least squares estimation model has the best estimation effect, which uses 12 feature factors, where six feature factors have the same goodness of fit R2. Considering the issue of data redundancy, this paper selects six feature factors to construct the SDG 1 China localization estimation model.
Table 5. Results of SDG 1 China’s district and county localization estimation model.
To further test the generalization ability of the model, this paper uses SDG 1 China’s localized partial least squares estimation model and SDG 1 China’s localized machine learning estimation models with six feature factors to obtain the SDG 1 evaluation value of China’s districts and counties in 2014. The SDG 1 evaluation value estimated by the model is compared with the NPCs list, and the districts and counties with the SDG 1 evaluation value ranking in the last 832 are extracted. Among them, the districts and counties that belong to the NPCs are the numbers of successful model identifications (). The results show that SDG 1 China’s localized partial least squares estimation model has identified the most impoverished counties in the country. There are 604 impoverished counties in the country, accounting for 72.77% of the total impoverished counties in the country. Therefore, this paper chooses Model II to retrieve the SDG 1 evaluation values of China’s districts and counties in 2012, 2014, 2016, and 2018.
3.2. Spatiotemporal distribution characteristics of SDG 1 evaluation value in China’s districts and counties
Based on SDG 1 China’s localized partial least squares estimation model, combined with the feature factors of multisource geographic data of China’s districts and counties, inversion was performed to obtain the SDG 1 evaluation values of China’s districts and counties in 2012, 2014, 2016 and 2018. This paper Combined the list of NPCs, in order to make the low-value area of SDG 1 evaluation value include NPCs as much as possible. Using the quantile method, the SDG 1 evaluation value of China’s districts and counties is divided into the lowest value area, low-value area, lower value area, higher value area, high-value area, and highest value area ().
Figure 7. The spatial distributions of SDG 1 assessment values of China’s districts and counties in 2012, 2014, 2016, and 2018, respectively.

The results show that the SDG 1 assessment values of China’s districts and counties in 2012, 2014, 2016, and 2018 have similar spatial distribution patterns. High-value areas and highest-value areas are concentrated in the coastal and northeastern regions of China, while higher-value areas extend inland along the high-value areas and highest-value areas, and are mixed with lower-value areas are mainly distributed in central and northeastern China. The low-value area extends along the higher and lower-value areas inland of western China and is mainly distributed in the southwest and northern China. The lowest value areas continue to extend along the low-value areas inland of western China and are mainly distributed in the six provinces of Qinghai, Tibet, Sichuan, Gansu, Xinjiang, and Yunnan in China. Among them, eastern Sichuan and western Chongqing constitute a higher circled area of SDG 1 evaluation value. At the same time, there were differences in the spatial distribution patterns of the SDG 1 assessment values of China’s districts and counties in 2012, 2014, 2016, and 2018. The more obvious difference is that the SDG 1 assessment values of China’s districts and counties increase with the year. From 2012 to 2014, the average SDG 1 evaluation value of China’s districts and counties increased by 11.08%, the average value of China’s districts and counties SDG 1 evaluation value increased by 1.48% from 2014 to 2016, and the average value of China’s districts and counties SDG 1 evaluation value increased by 1.48% from 2016 to 2018. The average SDG 1 evaluation value of China’s districts and counties increased by 22.67% from 2012 to 2018. The abovementioned increase in the average SDG 1 evaluation value of China’s districts and counties is spatially manifested more significantly. The SDG 1 evaluation value of many Chinese districts and counties switches to a higher partition.
To further analyze the differences in the trend of the SDG 1 evaluation value of China’s districts and counties, the differences in the SDG 1 evaluation values of China’s districts and counties in 2012–2014, 2014–2016, 2016–2018, and 2012–2018 were calculated. In this paper, the difference between the SDG 1 evaluation values is divided into positive and negative categories, and then the natural discontinuity point classification method is used to divide different growth rates (). The results show that the SDG 1 assessment value of most districts and counties in China from 2012 to 2018 showed a low-speed positive growth trend. Among them, the Pearl River Delta and Yangtze River Delta urban agglomerations have more high-speed positive growth regions. However, there were many low-speed negative growth regions in China from 2014 to 2016.
Figure 8. The changes in the SDG 1 assessment value of China’s districts and counties in 2012–2014, 2014–2016, 2016–2018, and 2012–2018, respectively.

To study the spatial aggregation effect of China’s SDG 1 evaluation value from 2012 to 2018, this paper conducted a global autocorrelation analysis of China’s SDG 1 evaluation value in 2012, 2014, 2016, and 2018. The results show that the global Moran’s I index in 2012 was 0.26, 2014, 2016, and 2018 were both 0.28, the Z scores were all greater than 2.58, and the P values were all 0.00. The results reject the null hypothesis at a significance level of 1%, indicating that there is a spatial agglomeration effect in the evaluation value of China’s SDG 1 in 2012, 2014, 2016, and 2018. Based on global autocorrelation analysis, this paper also carried out a local autocorrelation analysis of China’s SDG 1 evaluation values in 2012, 2014, 2016, and 2018 (). The results showed that there were a large number of low values in the western part of China in the past four years. Clustering, high-low clustering, and low-low clustering are also basically distributed in western China; most of the high-high clusters are distributed in the coastal areas of China, while low-high clustering is accompanied by high-high clusters distributed around its surroundings.
Figure 9. The local autocorrelation results of the SDG 1 evaluation value of China’s districts and counties in 2012, 2014, 2016, and 2018, respectively.

This paper conducts a first-level standard deviation ellipse analysis on the six grading areas of China’s SDG 1 evaluation value in 2012, 2014, 2016, and 2018. The number of districts and counties in the first-level standard deviation ellipse accounts for approximately 68% of the total number of districts and counties in the study area. This paper uses the standard deviation ellipse tool to analyze the spatiotemporal distribution of the center of gravity and direction of the 6 graded regions of China’s SDG 1 assessment value (). The results show that in 2012, 2014, 2016, and 2018, the long axis of the ellipse of the lowest value and low-value area of China’s SDG 1 evaluation value has a southeast-northwest distribution, indicating that these two regions have a southeast-northwest spatial distribution pattern. The major axes of the ellipses in the other four regions are all distributed from southwest to northeast, indicating that these four regions have a southwest-northeast spatial distribution pattern.
Figure 10. The standard deviation ellipse spatial distributions of the six graded areas of the SDG 1 evaluation value of China’s districts and counties in 2012, 2014, 2016, and 2018, respectively.

In addition, this paper further analyses the temporal and spatial distribution of the ellipse’s center of gravity (). The arrow directions range from lowest to highest values. The results show that the ellipse centroids of the six grading regions of China’s SDG 1 evaluation value in 2012, 2014, 2016, and 2018 moved from lowest to highest values as you move eastward, indicating that the spatial distribution pattern from lowest to highest value areas is distributed from the west to the east of China.
3.3. Spatiotemporal distribution characteristics of the evaluation results of China’s SDG poverty counties
This paper identifies the lowest- and low-value areas of China’s SDG 1 assessment value as SDG poverty counties and uses China’s NPCs list to compare the 2014 SDG poverty counties () to test the scientific rationality of SDG poverty counties. The results show that there were 852 SDG poverty counties in China in 2014, of which 611 were NPCs, accounting for 71.71% of the total number of SDG poverty counties. The current situation is generally consistent, and this method can be used to determine China’s SDG poverty counties in 2012, 2014, 2016, and 2018 ().
Figure 13. The spatial distribution of China’s SDG poverty counties’ estimated results in 2012, 2014, 2016, and 2018, respectively.

The results show that the number of SDG poverty counties in China in 2012, 2014, 2016, and 2018 was 951, 852, 843, and 746, respectively. The number of SDG poverty counties in China has been on a decreasing trend from 2012 to 2018, and the number of SDG poverty counties in China from 2014 to 2016 is much smaller than that in 2014–2016, 2012–2014, and 2016–2018. China’s SDG poverty counties are mainly distributed in the central and western regions of China and are especially concentrated in the six provinces of Qinghai, Tibet, Xinjiang, Yunnan, Gansu, and Guizhou. The SDG poverty counties in these six provinces not only account for a large proportion, but the number of SDG poverty counties reduced from 2012 to 2018 is too small, indicating that it is difficult to eliminate poverty in these regions.
4. Discussion
(1) The concept of poverty areas and counties and their measurement
Monitoring regional poverty first requires building a scientific and reasonable poverty evaluation indicator system, which requires a clear definition of poverty and the current socioeconomic development of the study area. The definition of poverty is not static. In the beginning, scholars only defined poverty in terms of income (Kingdon and Knight Citation2006; Rojas Citation2008). At the beginning of the 20th century, S. Rowntree proposed the concept of absolute poverty for the first time in his book “Poverty: A Study of Town Life”. He believes that a family is in poverty because the income it has is not enough to maintain the minimum needs for its physiological functions; however, relying on income level alone is not enough to comprehensively measure the degree of poverty, and a low-income level is only a sign of poverty. In the 1970s, Amartya Sen proposed the concept of ability poverty, trying to understand poverty from its root causes. Amartya Sen believes that the essence of poverty is the lack of the poor people’s ability to change their living conditions, resist various production or life risks, seize economic opportunities and obtain economic benefits. In addition, he believes that the poor lack equal access to many products and services, thus establishing a multidimensional poverty measurement system. However, income poverty and ability poverty are not substitute relationships but complementary relationships. The first goal of the SDGs is to eliminate all forms of poverty in the world. It is possible to measure poverty more comprehensively. Considering the actual situation of Chinese society and the difficulty of collecting statistical data at the district and county levels, this paper proposes the SDG 1 China district and county localization indicator system for the design of the SDGs global indicator framework, which can better reflect the poverty level of China’s districts and counties.
(2) Selection of remote sensing data in poverty monitoring based on geospatial perspective
Geographic data have obvious advantages in long-term and large-scale socioeconomic monitoring. There have been many studies on regional poverty monitoring based on multisource geographic data. These geographic data mainly include nighttime light images, land cover data, road network data, DEM data, and Normalized Difference Vegetation Index (NDVI) data (Zhao et al. Citation2019; Xu et al. Citation2021a). Due to the lack of road network data in China’s districts and counties in 2012, this paper did not select road network data as the data source for poverty monitoring. The feature factors extracted from the NDVI data in this paper did not improve the accuracy of the model’s estimation. In contrast, the 2014 SDG 1 evaluation value of China’s districts and counties obtained by the model’s estimation deviated from the actual poverty situation of China’s districts and counties. The SDG 1 Chinese localized estimation model is used as the data source to select nighttime light images, land cover data, DEM data, and NDVI data as data source to estimate the 2014 China district and county SDG 1 evaluation value (). Among the districts and counties in the bottom 832 of the evaluation value, only 236 belong to the NPCs, accounting for only 28.37%. Therefore, this paper does not select NDVI data for poverty monitoring.
(3) Comparison of the evaluation model results in poverty areas and counties
This paper uses nighttime light images, land cover data, and DEM data as data sources to estimate poverty in China’s districts and counties. The results can better reflect the actual poverty situation in China. There are also many studies estimating poverty in China’s districts and counties based on geographic data. Yu et al. (Citation2015) used NPP-VIIRS nighttime light images to estimate the poverty level in China’s districts and counties and achieved good results (). The results show that this paper can correctly identify more NPCs than Yu et al. (Citation2015) research. Li et al. (Citation2019b) extracted information from DMSP-OLS nighttime light data to identify poverty counties in China. The identified NPCs accounted for 73.10% of the total NPCs. Among the SDG poverty counties identified in this paper, 611 belong to the NPCs, accounting for 73.61% of the total NPCs.
Table 6. Estimated results of related poverty monitoring studies.
In this paper, the partial least squares method is selected as the SDG 1 Chinese localization estimation model. The estimation effect of SDG 1 China’s localized partial least squares estimation model is better than the commonly used machine learning regression models such as support vector machine, random forest, Gaussian process regression, and regression tree. The partial least squares method can better solve the multicollinearity problem based on retaining all the feature factors of geographic data, and the model will extract as much feature factor information related to the real SDG 1 evaluation value as possible and can also obtain features. The importance of factors for estimating the model, in addition, the positive or negative of the regression coefficient of each characteristic factor, is more reliable and in line with reality.
However, how to monitor China’s SDG 1 evaluation value with longer time series and higher precision still needs to be studied in depth. Based on complete statistical data, we will further improve SDG 1 China’s district and county localization indicator system and select more geographic data that can reflect poverty, such as road network data, to add to the model construction. The Chinese government announced in 2020 that it eliminated the long-standing absolute poverty in China, but there is a need for further research on the balanced development of the region in the later period in China.
5. Conclusions
This study proposes the SDG 1 China localization assessment method for the United Nations sustainable development framework design. The ability of this assessment method to successfully identify the NPCs is stronger than that of Yu et al. (Citation2015) and Li et al. (Citation2019b). It analyses the assessment value of China’s SDG 1 in 2012, 2014, 2016, and 2018 and the spatial distribution pattern of China’s SDG poverty counties. The following conclusions can be drawn: (1) SDG 1 China’s localized partial least squares estimation model has a good estimation effect, R2 is 0.65, and can successfully identify 72.77% of the NPCs. (2) The spatial distribution patterns of China’s SDG 1 assessment values in 2012, 2014, 2016, and 2018 are similar. China’s SDG 1 evaluation value has a spatial clustering effect. The spatial distribution pattern from lowest to highest value areas is distributed from the west to the east of China. At the same time, China’s SDG 1 evaluation value shows an overall increasing trend from 2012 to 2018. (3) The number of SDG poverty counties in China declined in 2012–2018, and the number of SDG poverty counties in China decreased significantly in 2012–2014 and 2016–2018.
From the above conclusions, it can be found that China has made great progress in poverty alleviation in recent years, and the number of poverty districts and counties has decreased significantly, but the distribution pattern of poverty districts and counties in China has not changed. China’s poverty districts and counties are still mainly located in the western region of China, so China’s follow-up poverty alleviation and poverty prevention work should be located in the western part of China. According to the research of this paper, the following policy suggestions are put forward: (1) In contiguous poverty areas, industrial development should be vigorously promoted, to help solve the employment problem in poverty areas. (2) Increase investment in basic education in poverty areas. The improvement of basic education facilities can effectively attract human resources and provide labor resources for industrial development. (3) Improve the connectivity between poverty areas and economically developed areas to give better play to the advantages of characteristic resources and industries in poverty areas.
This study provides an SDG 1 China localization assessment method based on multisource geographic data, which has a certain reference value for the implementation of China’s 2030 Agenda for Sustainable Development. In addition, this quantitative assessment method can also provide a reference for China’s medium- and long-term sustainable development strategies such as rural revitalization strategies.
Disclosure statement
No potential conflict of interest was reported by the author(s).
Data availability statement
The data that support the findings of this study are available in the public domain:
SRTM DEM data: (https://doi.org/10.1080/10095020.2022.2108346)
NPP-VIIRS-like nighttime light data: (https://doi.org/10.1080/10095020.2022.2108346)
Land cover data: (https://doi.org/10.1080/10095020.2022.2108346)
Socio-economic statistics data: (https://doi.org/10.1080/10095020.2022.2108346)
Consent for publication
All the coauthors consent the publication of this work.
Additional information
Funding
Notes on contributors
Mengjie Wang
Mengjie Wang is currently a master’s student at Hunan University of Science and Technology. His research interests include nighttime light remote sensing image processing and geographic modeling, socio-economic and urban ecological environment monitoring.
Yanjun Wang
Yanjun Wang is currently a professor at Hunan University of Science and Technology. He received the PhD in Photogrammetry and Remote Sensing at Wuhan University in 2012. His research interests include spatiotemporal geographic information analysis and mining, lidar remote sensing data processing, and modeling applications.
Fei Teng
Fei Teng is currently a master’s student at Hunan University of Science and Technology. His research interests include remote sensing data processing and geographic modeling, socioeconomic and urban ecological environment monitoring.
Shaochun Li
Shaochun Li is currently a master’s student at Hunan University of Science and Technology. His research interests include multi-source remote sensing data processing analysis and modeling analysis.
Yunhao Lin
Yunhao Lin is currently a master’s student at Hunan University of Science and Technology. His research interests include lidar point cloud intelligent processing and remote sensing image processing.
Hengfan Cai
Hengfan Cai is currently a master’s student at Hunan University of Science and Technology. His research interests include lidar point cloud intelligent processing.
References
- Afanador, N., T. Tran, and L. Buydens. 2013. “Use of the Bootstrap and Permutation Methods for a More Robust Variable Importance in the Projection Metric for Partial Least Squares Regression.” Analytica Chimica Acta 768: 49–56. doi:10.1016/j.aca.2013.01.004.
- Allen, C., G. Metternicht, and T. Wiedmann. 2018. “Initial Progress in Implementing the Sustainable Development Goals (SDGs): A Review of Evidence from Countries.” Sustainability Science 13: 1453–1467. doi:10.1007/s11625-018-0572-3.
- Alsharkawi, A., M. Al-Fetyani, M. Dawas, H. Saadeh, and M. Alyaman. 2021. “Poverty Classification Using Machine Learning: The Case of Jordan.” Sustainability 13: 1412. doi:10.3390/su13031412.
- Antoniades, A., I. Widiarto, and A. Antonarakis. 2019. “Financial Crises and the Attainment of the SDGs: An Adjusted Multidimensional Poverty Approach.” Sustainability Science 15: 1683–1698. doi:10.1007/s11625-019-00771-z.
- Arowolo, A., and X. Deng. 2017. “Land Use/Land Cover Change and Statistical Modelling of Cultivated Land Change Drivers in Nigeria.” Regional Environmental Change 18: 247–259. doi:10.1007/s10113-017-1186-5.
- Avtar, R., R. Aggarwal, A. Kharrazi, P. Kumar, and T. Kurniawan. 2019. “Utilizing Geospatial Information to Implement SDGs and Monitor their Progress.” Environmental Monitoring and Assessment 192: 35. doi:10.1007/s10661-019-7996-9.
- Avtar, R., A. Komolafe, A. Kouser, D. Singh, A. Yunus, J. Dou, and P. Kumar. 2020. ”Assessing Sustainable Development Prospects Through Remote Sensing: A Review”. Remote sensing applications : Society and environment 20: 100402. doi:10.1016/j.rsase.2020.100402.
- Biggeri, M., D. Clark, A. Ferrannini, and V. Mauro. 2019. “Tracking the SDGs in an ‘Integrated’ Manner: A Proposal for a New Index to Capture Synergies and Trade-Offs between and within Goals.” World Development 122: 628–647. doi:10.1016/j.worlddev.2019.05.022.
- Caizhen, L. 2010. “Who is Poor in China? A Comparison of Alternative Approaches to Poverty Assessment in Rural Yunnan.” The Journal of Peasant Studies 37: 407–428. doi:10.1080/03066151003595242.
- Chen, M., L. Liang, Z. Wang, W. Zhang, J. Yu, and Y. Liang. 2020b. “Geographical Thoughts on the Relationship Between ‘Beautiful China’ and Land Spatial Planning.” Journal of Geographical Sciences 30: 705–723. doi:10.1007/s11442-020-1751-6.
- Chen, J., S. Peng, H. Chen, X. Zhao, Y. Ge, and Z. Li. 2020a. “A Comprehensive Measurement of Progress Toward Local SDGs with Geospatial Information: Methodology and Lessons Learned.” ISPRS International Journal of Geo-Information 9: 522. doi:10.3390/ijgi9090522.
- Chen, Z., B. Yu, C. Yang, Y. Zhou, S. Yao, X. Qian, and C. Wang. 2021. ”An Extended Time Series (2000–2018) of Global NPP-VIIRS-Like Nighttime Light Data from a Cross-Sensor Calibration.” Earth System Science Data 13: 889–906. doi:10.5194/essd-13-889-2021.
- Chong, I. -G., and C. -H. Jun. 2005. “Performance of Some Variable Selection Methods when Multicollinearity is Present.” Chemometrics and Intelligent Laboratory Systems 78: 103–112. doi:10.1016/j.chemolab.2004.12.011.
- Cohen, A. 2010. “The Multidimensional Poverty Assessment Tool: A New Framework for Measuring Rural Poverty.” Development in Practice 20: 887–897. doi:10.1080/09614524.2010.508111.
- Dickens, C., V. Smakhtin, M. McCartney, G. O’Brien, and L. Dahir. 2019. “Defining and Quantifying National-Level Targets, Indicators and Benchmarks for Management of Natural Resources to Achieve the Sustainable Development Goals.” Sustainability 11: 462. doi:10.3390/su11020462.
- Dizdaroglu, D. 2017. “The Role of Indicator-Based Sustainability Assessment in Policy and the Decision-Making Process: A Review and Outlook.” Sustainability 9: 1018. doi:10.3390/su9061018.
- Echendu, A. J. 2020. “The Impact of Flooding on Nigeria’s Sustainable Development Goals (SDGs).” Ecosystem Health and Sustainability 6: 1791735. doi:10.1080/20964129.2020.1791735.
- Fisher, A., and S. Fukuda-Parr. 2019. “Introduction—Data, Knowledge, Politics and Localizing the SDGs.” Journal of Human Development and Capabilities 20: 375–385. doi:10.1080/19452829.2019.1669144.
- Geng, W., J. Chen, H. Zhang, and K. Xu. 2018. “Task and Progress of Iaeg-SDGs: Wggi in Monitoring SDGs through a ‘Geographic Location’ Lens.” The International Archives of the Photogrammetry, Remote Sensing and Spatial Information Sciences XLII-3: 385–390. doi:10.5194/isprs-archives-XLII-3-385-2018.
- Ghasemkhani, N., S. S. Vayghan, A. Abdollahi, B. Pradhan, and A. Alamri. 2020. “Urban Development Modeling Using Integrated Fuzzy Systems, Ordered Weighted Averaging (OWA), and Geospatial Techniques.” Sustainability 12: 809. doi:10.3390/su12030809.
- Ghosh, T., L. Coscieme, S. J. Anderson, and P. C. Sutton. 2020. “Building Volume per Capita (BVPC): A Spatially Explicit Measure of Inequality Relevant to the SDGs.” Frontiers in Sustainable Cities 2. doi:10.3389/frsc.2020.00037.
- Giles-Corti, B., M. Lowe, and J. Arundel. 2020. “Achieving the SDGs: Evaluating Indicators to be Used to Benchmark and Monitor Progress Towards Creating Healthy and Sustainable Cities.” Health Policy 124: 581–590. doi:10.1016/j.healthpol.2019.03.001.
- Giuliani, G., P. Mazzetti, M. Santoro, S. Nativi, J. Van Bemmelen, G. Colangeli, and A. Lehmann. 2020. “Knowledge Generation Using Satellite Earth Observations to Support Sustainable Development Goals (SDG): A Use Case on Land Degradation.” International Journal of Applied Earth Observation and Geoinformation 88: 102068. doi:10.1016/j.jag.2020.102068.
- Guo, Y., Y. Zhou, and Y. Liu. 2019. “Targeted Poverty Alleviation and its Practices in Rural China: A Case Study of Fuping County, Hebei Province.” Journal of Rural Studies 93: 430–440. doi:10.1016/j.jrurstud.2019.01.007.
- Guven, G., and H. Samkar. 2018. “Examination of Dimension Reduction Performances of PLSR and PCR Techniques in Data with Multicollinearity.” Iranian Journal of Science and Technology, Transactions A: Science 43: 969–978. doi:10.1007/s40995-018-0565-1.
- Heimann, T. 2019. “Bioeconomy and SDGs: Does the Bioeconomy Support the Achievement of the SDGs?” Earth’s Future 7: 43–57. doi:10.1029/2018ef001014.
- Holloway, J., and K. Mengersen. 2018. “Statistical Machine Learning Methods and Remote Sensing for Sustainable Development Goals: A Review.” Remote Sensing 10: 1365. doi:10.3390/rs10091365.
- Hossain, M. S., F. A. Johnson, J. A. Dearing, and F. Eigenbrod. 2016. “Recent Trends of Human Wellbeing in the Bangladesh Delta.” Environmental Development 17: 21–32. doi:10.1016/j.envdev.2015.09.008.
- Im, J. 2020. “Earth Observations and Geographic Information Science for Sustainable Development Goals.” GIScience & Remote Sensing 57: 591–592. doi:10.1080/15481603.2020.1763041.
- Janoušková, S., T. Hák, and B. Moldan. 2018. “Global SDGs Assessments: Helping or Confusing Indicators?” Sustainability 10: 1540. doi:10.3390/su10051540.
- Khalid, A. M., S. Sharma, and A. K. Dubey. 2018. “Developing an Indicator Set for Measuring Sustainable Development in India.” Natural Resources Forum 42: 185–200. doi:10.1111/1477-8947.12151.
- Khan, A. U., A. Saboor, A. Hussain, S. Sadiq, and A. Q. Mohsin. 2014. “Poverty Assessment as a Multidimensional Socio-Economic Concept: The Case of the Rawalpindi Region in Pakistan.” Asia Pacific Journal of Social Work and Development 24: 238–250. doi:10.1080/02185385.2013.844724.
- Kingdon, G. G., and J. Knight. 2006. “Subjective Well-Being Poverty vs. Income Poverty and Capabilities Poverty?” The Journal of Development Studies 42: 1199–1224. doi:10.1080/00220380600884167.
- Li, G., Z. Cai, X. Liu, J. Liu, and S. Su. 2019a. “A Comparison of Machine Learning Approaches for Identifying High-Poverty Counties: Robust Features of DMSP/OLS Night-Time Light Imagery.” International Journal of Remote Sensing 40: 5716–5736. doi:10.1080/01431161.2019.1580820.
- Li, G., L. Chang, X. Liu, S. Su, Z. Cai, X. Huang, and B. Li. 2019b. “Monitoring the Spatiotemporal Dynamics of Poor Counties in China: Implications for Global Sustainable Development Goals.” Journal of Cleaner Production 227: 392–404. doi:10.1016/j.jclepro.2019.04.135.
- Liang, H., Z. Guo, J. Wu, and Z. Chen. 2020. “GDP Spatialization in Ningbo City Based on NPP/VIIRS Night-Time Light and Auxiliary Data Using Random Forest Regression.” Advances in Space Research 65: 481–493. doi:10.1016/j.asr.2019.09.035.
- Lin, Z., and H. Xu. 2020. “Anthropogenic Heat Flux Estimation Based on Luojia 1-01 New Nighttime Light Data: A Case Study of Jiangsu Province, China.” Remote Sensing 12: 3707. doi:10.3390/rs12223707.
- Liu, S., J. Bai, and J. Chen. 2019b. “Measuring SDG 15 at the County Scale: Localization and Practice of SDGs Indicators Based on Geospatial Information.” ISPRS International Journal of Geo-Information 8: 515. doi:10.3390/ijgi8110515.
- Liu, H., S. Leng, C. He, J. Peng, S. Piao, and X. Wang. 2019a. “China’s Road Towards Sustainable Development: Geography Bridges Science and Solution.” Progress in Physical Geography: Earth and Environment 43: 694–706. doi:10.1177/0309133319851026.
- Liu, Y., J. Peng, and Y. Wang. 2018. “Application of Partial Least Squares Regression in Detecting the Important Landscape Indicators Determining Urban Land Surface Temperature Variation.” Landscape Ecology 33: 1133–1145. doi:10.1007/s10980-018-0663-7.
- Liu, Q. -Q., M. Yu, and X. -L. Wang. 2015. “Poverty Reduction Within the Framework of SDGs and Post-2015 Development Agenda.” Advances in Climate Change Research 6: 67–73. doi:10.1016/j.accre.2015.09.004.
- Liverman, D. M. 2018. “Geographic Perspectives on Development Goals.” Dialogues in Human Geography 8: 168–185. doi:10.1177/2043820618780787.
- Lopez-Ruiz, H. G., J. Blazquez, and F. Hasanov. 2019. “Estimating the Saudi Arabian Regional GDP Using Satellite Nighttime Light Images.” No. ks–2019-dp80, Riyadh: King Abdullah Petroleum Studies and Research Center. doi:10.2139/ssrn.3382748.
- Lu, H., and G. Liu. 2014. “Spatial Effects of Carbon Dioxide Emissions from Residential Energy Consumption: A County-Level Study Using Enhanced Nocturnal Lighting.” Applied Energy 131: 297–306. doi:10.1016/j.apenergy.2014.06.036.
- Ma, L., X. Che, J. Zhang, F. Fang, and M. Chen. 2019. “Rural Poverty Identification and Comprehensive Poverty Assessment Based on Quality-of-Life: The Case of Gansu Province (China).” Sustainability 11: 4547. doi:10.3390/su11174547.
- Menton, M., C. Larrea, S. Latorre, J. Martinez-Alier, M. Peck, L. Temper, and M. Walter. 2020. “Environmental Justice and the SDGs: From Synergies to Gaps and Contradictions.” Sustainability Science 15: 1621–1636. doi:10.1007/s11625-020-00789-8.
- Molla, Y. B., B. Rawlins, P. T. Makanga, M. Cunningham, J. E. H. Ávila, C. W. Ruktanonchai, and K. Singh. 2017. “Geographic Information System for Improving Maternal and Newborn Health: Recommendations for Policy and Programs.” BMC Pregnancy and Childbirth 17: 26. doi:10.1186/s12884-016-1199-y.
- Moreno Pires, S., T. Fidélis, and T. B. Ramos. 2014. “Measuring and Comparing Local Sustainable Development Through Common Indicators: Constraints and Achievements in Practice.” Cities 39: 1–9. doi:10.1016/j.cities.2014.02.003.
- Mori, K., and A. Christodoulou. 2012. “Review of Sustainability Indices and Indicators: Towards a New City Sustainability Index (CSI).” Environmental Impact Assessment Review 32: 94–106. doi:10.1016/j.eiar.2011.06.001.
- Nagabhatla, N., and R. Brahmbhatt. 2020. “Geospatial Assessment of Water-Migration Scenarios in the Context of Sustainable Development Goals (SDGs) 6, 11, and 16.” Remote Sensing 12: 1376. doi:10.3390/rs12091376.
- Niu, T., Y. Chen, and Y. Yuan. 2020. “Measuring Urban Poverty Using Multi-Source Data and a Random Forest Algorithm: A Case Study in Guangzhou.” Sustainable Cities and Society 54: 102014. doi:10.1016/j.scs.2020.102014.
- Niu, S., X. Lyu, G. Gu, X. Zhou, and W. Peng. 2021. “Sustainable Intensification of Cultivated Land Use and its Influencing Factors at the Farming Household Scale: A Case Study of Shandong Province, China.” Chinese Geographical Science 31: 109–125. doi:10.1007/s11769-021-1178-8.
- Pizzi, S., A. Caputo, A. Corvino, and A. Venturelli. 2020. “Management Research and the UN Sustainable Development Goals (SDGs): A Bibliometric Investigation and Systematic Review.” Journal of Cleaner Production 276: 124033. doi:10.1016/j.jclepro.2020.124033.
- Reid, A. J., J. L. Brooks, L. Dolgova, B. Laurich, B. G. Sullivan, P. Szekeres, et al. 2017. “Post-2015 Sustainable Development Goals Still Neglecting Their Environmental Roots in the Anthropocene.” Environmental Science & Policy 77: 179–184. doi:10.1016/j.envsci.2017.07.006.
- Ren, Q., Q. Huang, C. He, M. Tu, and X. Liang. 2018. “The Poverty Dynamics in Rural China During 2000–2014: A Multi-Scale Analysis Based on the Poverty Gap Index.” Journal of Geographical Sciences 28: 1427–1443. doi:10.1007/s11442-018-1554-1.
- Rojas, M. 2008. “Experienced Poverty and Income Poverty in Mexico: A Subjective Well-Being Approach.” World Development 36: 1078–1093. doi:10.1016/j.worlddev.2007.10.005.
- Rosati, F., and L. G. D. Faria. 2019. “Addressing the SDGs in Sustainability Reports: The Relationship with Institutional Factors.” Journal of Cleaner Production 215: 1312–1326. doi:10.1016/j.jclepro.2018.12.107.
- Shi, K., Z. Chang, Z. Chen, J. Wu, and B. Yu. 2020. “Identifying and Evaluating Poverty Using Multisource Remote Sensing and Point of Interest (POI) Data: A Case Study of Chongqing, China.” Journal of Cleaner Production 255: 120245. doi:10.1016/j.jclepro.2020.120245.
- Simwanda, M., and Y. Murayama. 2018. “Spatiotemporal Patterns of Urban Land Use Change in the Rapidly Growing City of Lusaka, Zambia: Implications for Sustainable Urban Development.” Sustainable Cities and Society 39: 262–274. doi:10.1016/j.scs.2018.01.039.
- Solís, P., B. McCusker, N. Menkiti, N. Cowan, and C. Blevins. 2018. “Engaging Global Youth in Participatory Spatial Data Creation for the UN Sustainable Development Goals: The Case of Open Mapping for Malaria Prevention.” Applied Geography 98: 143–155. doi:10.1016/j.apgeog.2018.07.013.
- Steele, J. E., P. R. Sundsoy, C. Pezzulo, V. A. Alegana, T. J. Bird, J. Blumenstock, and J. Bjelland. 2017. ”Mapping Poverty Using Mobile Phone and Satellite Data”. Journal of the Royal Society Interface 14: 20160690. doi:10.1098/rsif.2016.0690.
- Tian, Y., Z. Wang, J. Zhao, X. Jiang, and R. Guo. 2018. “A Geographical Analysis of the Poverty Causes in China’s Contiguous Destitute Areas.” Sustainability 10: 1895. doi:10.3390/su10061895.
- Vanham, D., A. Leip, A. Galli, T. Kastner, M. Bruckner, A. Uwizeye, and K. van Dijk. 2019. “Environmental Footprint Family to Address Local to Planetary Sustainability and Deliver on the SDGs.” The Science of the Total Environment 693: 133642. doi:10.1016/j.scitotenv.2019.133642.
- Vaziri, M., M. Acheampong, J. Downs, and M. Rafee Majid. 2018. “Poverty as a Function of Space: Understanding the Spatial Configuration of Poverty in Malaysia for Sustainable Development Goal Number One.” GeoJournal 84: 1317–1336. doi:10.1007/s10708-018-9926-8.
- Wang, W., H. Cheng, and L. Zhang. 2012. “Poverty Assessment Using DMSP/OLS Night-Time Light Satellite Imagery at a Provincial Scale in China.” Advances in Space Research 49: 1253–1264. doi:10.1016/j.asr.2012.01.025.
- Wang, Y., M. Wang, B. Huang, S. Li, and Y. Lin. 2021a. “Evaluation and Analysis of Poverty-Stricken Counties Under the Framework of the UN Sustainable Development Goals: A Case Study of Hunan Province, China.” Remote Sensing 13: 4778. doi:10.3390/rs13234778.
- Wang, Y., M. Wang, B. Huang, S. Li, and Y. Lin. 2021b. “Estimation and Analysis of the Nighttime PM2.5 Concentration Based on LJ1-01 Images: A Case Study in the Pearl River Delta Urban Agglomeration of China.” Remote Sensing 13: 3405. doi:10.3390/rs13173405.
- Wu, B., F. Tian, M. Zhang, H. Zeng, and Y. Zeng. 2020. “Cloud Services with Big Data Provide a Solution for Monitoring and Tracking Sustainable Development Goals.” Geography and Sustainability 1: 25–32. doi:10.1016/j.geosus.2020.03.006.
- Xu, L., X. Deng, Q. Jiang, and F. Ma. 2021a. “Identification and Alleviation Pathways of Multidimensional Poverty and Relative Poverty in Counties of China.” Journal of Geographical Sciences 31: 1715–1736. doi:10.1007/s11442-021-1919-8.
- Xu, X., Z. Kuang, J. Han, Y. Meng, L. Li, H. Luan, P. Xu. 2019. “Development and Characterization of a Fluorescent Probe for GLS1 and the Application for High-Throughput Screening of Allosteric Inhibitors.” Journal of Medicinal Chemistry 62: 9642–9657. doi:10.1021/acs.jmedchem.9b01035.
- Xu, Y., Y. Mo, and S. Zhu. 2021b. “Poverty Mapping in the Dian-Gui-Qian Contiguous Extremely Poor Area of Southwest China Based on Multi-Source Geospatial Data.” Sustainability 13: 8717. doi:10.3390/su13168717.
- Yu, B., K. Shi, Y. Hu, C. Huang, Z. Chen, and J. Wu. 2015. “Poverty Evaluation Using NPP-VIIRS Nighttime Light Composite Data at the County Level in China.” IEEE Journal of Selected Topics in Applied Earth Observations and Remote Sensing 8: 1217–1229. doi:10.1109/jstars.2015.2399416.
- Yuan, Y., M. Xu, X. Cao, and S. Liu. 2018. “Exploring Urban-Rural Disparity of the Multiple Deprivation Index in Guangzhou City from 2000 to 2010.” Cities 79: 1–11. doi:10.1016/j.cities.2018.02.016.
- Zhao, X., B. Yu, Y. Liu, Z. Chen, Q. Li, C. Wang, and J. Wu. 2019. “Estimation of Poverty Using Random Forest Regression with Multi-Source Data: A Case Study in Bangladesh.” Remote Sensing 11: 375. doi:10.3390/rs11040375.
- Zhou, Y., F. Zhang, Z. Du, X. Ye, and R. Liu. 2017. “Integrating Cellular Automata with the Deep Belief Network for Simulating Urban Growth.” Sustainability 9: 1786. doi:10.3390/su9101786.