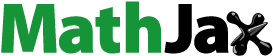
ABSTRACT
Spatial equity in access to urban services is a significant element of sustainable urban planning. To date, a service-based index is used to measure service attractiveness, considering attractiveness as a static concept. While from the user side view, service attractiveness is a dynamic concept related to the variation of different opportunities of users to use the services and capacities. In this paper, a user-based method for measuring service attractiveness is proposed. In this approach, the attractiveness of service for a user is modeled as a function of the user’s Travel Times (TT), Free Times (FTs), park size and relative positions of the user, local demanding population, and service. Then, the inequity of attractiveness of services for different users has been examined using three inequity indices including the Duration of Use (DU), Frequency of Visit (FV), and the Chance of Space Choice (CSC). The proposed method is applied to urban regional parks of Tehran municipality. Traffic Analysis Zones (TAZs) and regional parks are respectively regarded as the users and services. In addition to considering inequity of access to the first closest park, the additional cost of accessing to the other two closest parks is also considered. Results show that the proposed method leads to the exploration of the existing inequities of DU, FV, and CSC among residents in local scales, whereas in the service-based method, all users of a service are highlighted with a single value of attractiveness. Comparison of the user-based and service-based attractiveness indices show significant differences. As an example, user-based attractiveness values of about 14% and 20% of TAZs are respectively 5 and 3 square meters more than that of the service-based attractiveness index. However, the service-based method is suitable for regional-scale comparison of the inequities. Therefore, the proposed index can complement the service-based index for inequity mapping and management in varying scales.
1. Introduction
Achievement of spatial equity in the distribution of urban services is one of the most significant elements of sustainable urban development (Talen Citation1998). The concept of spatial equity concerns the distribution of services (Monzón, Ortega, and López Citation2013) and considers equity to avoid regional disparity (Wismadi Citation2015). Accessibility is a key concept in assessing spatial equity in the allocation of services, worldwide (Van Wee Citation2016). Distance and attractiveness are the two most frequently used elements for measuring the accessibility index of a neighborhood or group to urban services. The distance between a service and a user may be measured by the physical distance, travel time, or any associated parameter representing the travel costs. The attractiveness of a service refers to its quality, size, or capacity (Omer Citation2006).
Service attractiveness is generally tested using the covering model of accessibility (Hodgart Citation1978) in which a service radius defined by municipalities and urban planners is used, and as a common practice, residents who are covered by the specified radius are considered as the demanding population Then, a single attractiveness index is calculated for each service by dividing its size or capacity values into the total number of demanding populations within the service catchment. This is hereinafter referred to as the service-based approach for estimating attractiveness.
The service-based approach creates several problems. First, the specified radius represents only an approximation of the service catchment of a park, and the real service radius can be different depending on the density and condition of the demanding population surrounding the service. Second, computation of the attractiveness indices in service-based approaches is based on the assumption that each service is used only by residents within the predefined service catchments. But in reality, residents may use any available service, regardless of their service catchment. Third, the service-based approach considers service attractiveness as a function of static concepts such as the service area/size and the total demanding population living in the corresponding service catchments. This definition of attractiveness results in a single value for the attractiveness of a center for all of its users. Therefore, allocation of a single attractiveness index for each service through a service-based approach, as in the previous studies (e.g. Nicholls Citation2001; Smoyer‐tomic, Hewko, and Hodgson Citation2004; Rosero-Bixby Citation2004; Tsou, Hung, and Chang Citation2005; Omer Citation2006; Oh and Jeong Citation2007; McGrail and Humphreys Citation2009; Chang and Liao Citation2011; Laghai and Bahmanpour Citation2012; Lee and Hong Citation2013; Taleai, Sliuzas, and Flacke Citation2014; So Citation2016; Dadashpoor and Rostami Citation2017; Tan and Samsudin Citation2017; Xiao et al. Citation2017; Stępniak and Goliszek Citation2017; Hu et al. Citation2020; Arranz-López, Soria-Lara, and Pueyo-Campos Citation2019; Lu, Zhang, and Lan Citation2019; Mooney et al. Citation2019; Zhang, Yang, and Pan Citation2019), does not take into account the distribution and positions of users in determining the attractiveness of the service which can lead to hindering the existing spatial inequities among the users.
In a service-based approach, it is assumed that all residents of each service catchment are interested to use a single and similar service; therefore, the only discriminating factors inside each service catchment are the travel costs or the distances between the users and services. Because of using a single value for the attractiveness of each service center, the highlighted inequities inside each service catchment only reflects the distance-related inequities, neglecting the inequities caused by the heterogeneous space and dynamic nature of the service attractiveness.
Recently, alternative definitions of attractiveness have been addressed by some researchers. For example, the temporal dynamics of the attractiveness of service centers have been considered by García-Albertos et al. (Citation2019). In their study, the instant attraction mass of each zone like j was defined by a single one-dimensional value as the total number of trips with a destination in that transport zone at a time of the day. Zheng et al. (Citation2019) assessed the spatial accessibility to hospitals and defined the attraction of hospitals as the level of medical technology available to service residents. They used the grade weightings proposed by Zeng et al. (Citation2017), adopting a weight of 0.6 for high-quality medicine, 0.3 for a basic hospital, and 0.1 for a primary hospital. Panagiotopoulos and Kaliampakos (Citation2019) used the Accessibility/Remoteness Index of Australia (ARIA) in Greece to measure the accessibility index in which the general attractiveness function of each service j for a location i is considered to be one or zero. If the service center j is higher (in terms of services) than the locality i, then the general attractiveness function is one otherwise it is zero. Despite these studies, particularly the concept of temporally dynamic attractiveness proposed by García-Albertos et al. (Citation2019), revealing additional dimensions of attractiveness, they still assume a single attractiveness index for each service and for all of its users.
Another important deficiency of many of the previous studies is the consideration of the inequities of users in accessing a single closest service, based on the assumption of one-to-one allocation; each resident uses or has to use only one service. While this concept is quite attractive for planners, often it may not reflect the realities. This problem has been addressed by Zhang, Lu, and Holt (Citation2011). They considered distances of users to seven alternative nearby parks in the USA. The probability of using a park was modeled based on distance decay theory by considering the distance of users to different nearby parks and using the distances as the weights for use of different parks. Finally, the expected distance of census blocks to their nearby parks named as the population-weighted distance (PWD) was modeled, and the resulting maps of inequities of PWDs were produced. Only considering the distance of census blocks from the neighborhood parks and size of the parks, the proposed PWD index is mainly concerned with the potential access modeling, and does not consider park attractiveness (Zhang, Lu, and Holt Citation2011).
Using distance decay theory to determine the demand for services has become common, recently. Gao et al. (Citation2021) investigated the spatial heterogeneity in the distance decay of using dockless bike-sharing systems (DLBS) using transaction data. They generated the distance decay function using the number of trips made at different distances and proved that mobility flows decrease along with travel distance. Finally, the effect of various built-up environmental factors including the population density, land use entropy, branch road density, metro station, commercial land use ratio, industrial land use ratio, and motorway density on the distance decay of DLBS was explored. In another study, Łaszkiewicz et al. (Citation2022) investigated the relationship between the walking accessibility to amenities from green spaces and apartment prices in Oslo, using non-linear distance decay functions. Similarly, the decline in the willingness of using services located at higher distance from the user locations has been considered in many other published works (e.g. Badura et al. Citation2020; Bateman et al. Citation2006; Johnston, Besedin, and Holland Citation2019; Schaafsma et al. Citation2013; Söderberg and Barton Citation2014; Yamaguchi and Shah Citation2020; Goel Citation2018; Martínez and Viegas Citation2013; Halás, Klapka, and Kladivo Citation2014). Previous studies of the distance decay are mainly focused on the effects of the distance decay on the accessibility and service choice by the users. Influences of the distance, spatial distribution and relative positions of the potential users on the attractiveness of each service and its variability among the different neighborhoods have not been considered.
Generally, spatial equity may be measured using the objective and subjective methods. Subjective evaluation usually includes field studies such as the design of a questionnaire to understand and analyze the geographical tendencies and perceptions of citizens. It is mainly concerned with the tendencies and personal characters of citizens such as the age, sex and physical abilities. The objective evaluation is usually based on the quantitative indicators related to the physical environmental characteristics. At the other hand, the concept of spatial equity in the distribution of services can be divided into the two categories of horizontal and vertical spatial equities (Ashik, Mim, and Neema Citation2020). Horizontal spatial equity is concerned with the equal distribution of services among all the citizens regardless of their socio-economic status and conditions (Bennett Citation1983; Tsou, Hung, and Chang Citation2005). Whereas, in vertical spatial equity, equal access to services is regarded by considering the special needs and demands of people in different situations (Litman Citation2017; Wang and Yaung Citation2013). Due to lack of data and the need for costly and time consuming field surveys for the subjective and vertical evaluation of spatial equity (Lotfi and Koohsari Citation2009), the objective and horizontal methods have been adapted to investigate the spatial equity in this study.
To fill the above-mentioned shortcomings, a user-based approach for estimating service attractiveness is proposed in this paper. In this approach, service catchment is considered to change in time, based on the temporal Patterns of Travel Times (TTs) and Free Times (FTs) of users. In this regard, besides considering accessibility to alternative parks, service attractiveness is considered as a dynamic variable depending on the TT and FT and positions of user, service, and other local demanders. Therefore, instead of assuming a unique value for the attractiveness of users of a service, a vector of values showing the attractiveness of service for each user is produced. Also, consideration of destinations is not confined to a single center. Instead, inequity of additional access costs to the three closest services is considered and the inequity of extra costs for use of the second and third closest services by each user is compared.
By considering the deficiencies of the conventional stationary approaches for defining the attractiveness of a park, the main objective of this paper is to explore the problem of the attractiveness of a public service like a park and its variability for the corresponding users. In this regard, by developing the three related indices of DU, FV and CSC, an efficient approach for modeling the variability of the attractiveness of a park for different users is proposed. Examination of the results shows that the proposed approach leads to enhancement and visualization of the important spatial inequities of attractiveness within the park service catchment which are usually neglected in the conventional static service-based attractiveness measures.
The rest of the paper is organized as follows. In Section 2, the proposed approach and methods of research are specifically explained. Methods and results of practical examination of the proposed models and their comparison with the conventional service-based approach are presented in Section 3. The discussion and conclusion of the research are put forward in the last two sections.
2. Accessibility and attractiveness measurement
2.1. Accessibility measurement
There are two types of place-based and people-based accessibility evaluation techniques (Neutens et al. Citation2010). Place-based measures investigate the proximity of service receivers to desired service providers. While people-based measures are based on detailed observations of an individual’s activity schedule and space-time constraints (e.g. Delafontaine et al. Citation2011) which requires a vast database which is not available in many developing countries. So, the use of place-based or so-called distance-based methods is more prevalent among researchers. The most commonly used distance-based models for evaluating spatial equity include the Container model (e.g. Talen and Anselin Citation1998; Tsou, Hung, and Chang Citation2005; Kelobonye et al. Citation2019), Gravity model (e.g. Talen and Anselin Citation1998; Makri and Folkesson Citation1999; Karner Citation2018), Minimum distance model (Talen and Anselin Citation1998) and Covering objective model (Talen and Anselin Citation1998). Anjomshoaa et al. (Citation2017) provide a comprehensive review of different accessibility measurement techniques in urban studies.
In this paper, the Gravity model concept proposed by Hansen (Citation1959) is used to test the accessibility index, as it allows accessibility to be examined from both aspects of access costs and benefits. EquationEquation (1)(1)
(1) calculates the accessibility index of the user i to a given urban service type according to the Gravity model.
where is the accessibility index of user i to service j;
is the normalized travel time from residential parcel i to service j and
is the normalized attractiveness index of service j that is usually calculated using EquationEquation (2).
(2)
(2)
where is the area of service j and
is the total demanding population resident in the service catchment of the service j.
In most of the previous studies, the total population of each service catchment is directly embedded in the above equation (e.g. Rich Citation1978; Omer Citation2006; Lotfi and Koohsari Citation2009; Taleai, Sliuzas, and Flacke Citation2014; Dadashpoor, Rostami, and Alizadeh Citation2016; Brook Citation2016; Arranz-López et al. Citation2017; Hu et al. Citation2020; Tahmasbi et al. Citation2019; Ashik, Mim, and Neema Citation2020; Kompil et al. Citation2019; Fasihi and Parizadi Citation2020). So, because of the overlap of service catchments, using the population of these catchments would lead to repeated consideration of each recipient population in calculating the attractiveness indices. Although this may be valid by assuming each user to use more than a single service, it leads to an overestimation of the population in attractiveness estimation. On the other hand, solving the problem of overlapping service catchments by algorithms such as the minimum distance, the minimum cost allocation or other innovative methods (e.g. Fasihi and Parizadi Citation2020 or Whitehead et al. Citation2020) lead to an unrealistic assumption of limiting each resident to use a single service. Whereas, each service may not be necessarily used by all the neighborhoods covered by each service catchment. Because, choice of residents can be influenced by a combination of many factors such as the attractiveness, access costs, free times, travel times, and demand times. On the other hand, the competition for limited sources is not considered in EquationEquations (1)(1)
(1) and (Equation2
(2)
(2) ); a fact that Joseph and Bantock Citation1982 has taken into account by developing a modified gravitational potential model which was later completed by Zhang, Lu, and Holt (Citation2011) and used by many researchers (e.g. Mishra et al. Citation2019; Leung et al. Citation2020). Thus, by assuming each resident to consider more than a single service and that access costs (travel time, travel distance, etc.) are the most important factors affecting the choice of service by residents, a proportional distance function known as distance decay theory has been adopted in this research by modification of the definition of
as in EquationEquation (3).
(3)
(3)
where
In which is the proportion of the population living in neighborhood i who visit service j,
is the amount of population living in neighborhood i and n is the total number of surrounding services which the population living in neighborhood i are interested to visit.
2.2. The proposed user-based attractiveness approach
Calculation of the attractiveness index for each service center, as described in EquationEquation (2)(2)
(2) , is mainly based on the static parameters of size (or capacity) and population. This leads to a static index for attractiveness, independent of the user to user and user to service positions. This model may be applicable for some services such as the schools, where a fixed number of students are allocated to each class according to its size, capacity, or the existing standards. Then, the attractiveness of each school or each class can be defined by the ratio of the size to the number of students or the number of teachers to students. These static attractiveness values, the school models, well fit some service centers such as the schools or cinemas. Because, in these centers, the existing capacities and spaces are already allocated and simultaneously used by the users. That is all students attend and use the school in pre-defined times and spaces. From per capita management points of view, static models of attractiveness and service areas of public services provide useful long-term concepts for urban planners and managers. But these models of attractiveness may not be useful for modeling of the real-life and user to user attractiveness of public service centers such as the parks or health centers. Because, both the usable spaces and demanding population are dynamic, showing high variability with space and time. The followings are some particular characteristics of many public services which discriminate them from the simple school models of attractiveness:
Usable spaces of most public services are not homogeneous, and they are composed of heterogeneous units in finer scales of individual user spaces. As an example, spatial distribution and temporal variation of light, temperature (e.g. sunny and shady areas), facilities, scenic values of landscapes, and their interactions at different times of a day and seasons in a park are very complex and variable. This leads to considerable heterogeneities and the creation of various dynamic characteristics affecting the local variation of attractiveness for users.
Because of the heterogeneity of usable spaces of public services and diversity of the demands of users, attractiveness of services can be very variable. Due to the diversity of the quality of available usable spaces and the decrease of the alternative suitable spaces, the attractiveness of a park is not constant and declines with the increase of the users and occupied spaces. It is usually maximum for the early coming users and expected to decline with an increase of user and space occupations.
Distribution patterns and travel costs of the demanding population of service areas are variable and heterogeneous. So, users in closer distances to services have a higher chance of selecting and enjoying the best spaces and services (e.g. best spaces in a park or quicker services in a health center) before the others. Therefore, by defining equity of attractiveness as the equity of opportunities for selection and better use of the capacities of service, the real attractiveness of a park is not equal for different users. It is higher for closer users than those located in farther distances from the service.
Demand to use services such as parks is highly related to temporal patterns of FT of the users. FTs may be divided in two classes of private and public. Depending on the specific habits and temporal activities of the users, short FTs can be mostly regarded as the private. Whereas, longer FTs such as a couple of days and hours or a full day can be attributed to public FTs or holidays. These FTs lead to higher demands to use public services such as the parks. It is reasonable to assume that the frequency or probability of short FTs is higher than those of the longer FTs. Then, relations between the frequency and length of FTs can be represented with a non-linear function decreasing with lengths of FTs as highlighted in .
Figure 1. Conceptual model of the variation of frequencies of FT and demand to use a park as a function of FT length.

In contrast to this, demand for park use is expected to non-linearly increase with the increase of FT length in weekends and holidays. In longer FTs, users have more time to use services and also more options to choose. Based on this concept, the service catchment of a service is directly related to the FTs of users. In the other word, a specific park may be visited only by its neighboring users in short FTs. While only in longer FTs, farther users are able to choose and visit it. Therefore, service catchment is variable in time depending on the user’s FT and TT.
2.2.1. Modeling of DU
Considering an equal FT for all users, service j can be visited by user i if the difference between FT and TT from user i to service j and vice versa is greater than a specified threshold. Assuming that
and
respectively represent the travel times of a user from location i to service j and vice versa at time t, and
represent their average, then, the instant catchment and possibility of visiting a service such as j by user i at time t can be expressed as in EquationEquation (5)
(5)
(5) :
where is the free time of user i at time t, and threshold is the minimum acceptable DU of parks by the user i (
).
Visit Duration (VD) is another critical concept for deciding to visit a park such as j by different service users. Because, resident i can decide to visit park j only if equals or exceeds
. Where
is a function of travel time (
) and
of park j by resident i at time t as follows:
Therefore, to visit a service such as j, at time t there is a need to have:
2.2.2. Modeling of FV
The inequity of attractiveness of service for users may be considered by inequities in the FV, DU, and CSC. Inequities of FV among the different users of a service can be modeled for a given period of time (e.g. a week) or a given total free time. Let TFT represent an equal total free times of each user of service j. TFT for a user such as i can be expressed as a combination of n instant free times:
According to EquationEquation (5)(5)
(5) , each instant FT can be sufficient to visit service j only if:
By selecting a constant value for (minimum DU of users) FV of users for the given
and variability of FVs for different scenarios of
can be modeled as follows:
where if
meets the condition of EquationEquation (9)
(9)
(9) , otherwise
EquationEquation (10)(10)
(10) highlights the fact that the frequency or number of visits of users are inversely related to their TT and assuming equal TFTs for different users of a service, residents in positions with lower TTs to services have a higher chance of frequent use of a park.
2.2.3. Modeling of CSC
It can be seen from EquationEquation (6)(6)
(6) that
, therefore for a given FT, DU is negatively related to TT and residents with lower TTs can have higher DUs and vice versa. In the same way as for DUs, it can be shown that residents with lower TTs have a higher CSC and selecting better spaces. Choices of space for the user i, at any time, depends on the number of all users who are present in the park before user i (
).
Considering the inverse relations between the FT length and frequency of park use as in , the frequency of park visits for residents in lower TTs to parks is higher than those in higher TTs. In addition, because of the condition of FTs larger than VDs, longer FTs are required for park visits of residents in positions with longer TTs. Therefore, can be modeled by the set of all residents such as k (
) that their TTs are less than or equal to that of the resident i:
Although the total size of a park may be a useful concept for planning purposes, it may not be a good indicator of its daily and hourly use. Because of space availability and space choice points of view, the total usable space of a park is available for use, only when there is not a user. Therefore, two user-based attractiveness indices are proposed here. One is the local index considering only the local population as the competitors. Another is considering the population in both local and within service area scales. The first user-based attractiveness index of a service such as j for a coming user i () can be modeled by replacing
with
, in EquationEquation (2).
(2)
(2)
By assuming to represent the maximum number of park users
≥
, because
is a subset of
≤
. So, the actual attractiveness of a park for a user is usually higher than those of the constant average values calculated by using the whole population of the service catchment as in EquationEquation (2).
(2)
(2) Only when all users of the service catchment are simultaneously present in the park,
and two attractiveness values are equal
.
As mentioned before, the first user-based attractiveness index as in EquationEquation (12)(12)
(12) is a local model of attractiveness which is suitable for local and within the service catchment application. Because, for a user such as i, only populations with TTs lower than that of i are considered
. However in larger scales of between service areas, there are significant differences between the size, quality and number of the users of different parks and the total competitive population of service catchments are usually composed of residents either with lower
or higher
TTs than each user such as i to a park. The contribution of these residents in
can be modeled by nonlinear or linear functions. However, for simplicity, a linear combination function, with an inverse weighting of the average TTs of the close and far population from the park are proposed here, as follows.
where
and
.
Parameters and
are weights representing the effects of
and
on the attractiveness of the park for user i (
+
= 1) and defined as:
where and
respectively represent the average TTs of
and
to visit the destination park j. Substituting new definition of
from EquationEquation (13)
(13)
(13) in EquationEquation (12)
(12)
(12) , the second user-based index of attractiveness
is defined as:
By substituting for and
from EquationEquation (14)
(14)
(14) :
Two definitions of user-based attractiveness and
, in EquationEquations (12)
(12)
(12) and (Equation16
(16)
(16) ) provide complementary roles for assessment and comparison of the attractiveness of public services and their equity for different users in the local and service catchment scales. It should be noted that for users on the boundary of instant service catchments, the value of
would be equal to one and
That is, the minimum user-based attractiveness of each service center occurs at its service catchment boundary in which
and
. Then, the attractiveness indices can be estimated using either the EquationEquations (1)
(1)
(1) or Equation(12)
(12)
(12) . However, for brevity, in this research, only the second proposed index (
) has been used for practical examination and comparison with the conventional service-based index.
2.3. Accessibility to alternative parks
For consideration of the alternative accessibilities, there is a need to define destination sets of alternative parks for each residential parcel. These sets may be defined by using either a fixed distance or a fixed number of parks. The first approach considers all the services in less than a pre-defined distance or travel time from the user. The second approach defines the locations of pre-defined number of alternative centers regardless of their distances from the user. An important deficiency of the first approach is its sensitivity to the effects of the uneven distribution of service centers, leading to considerable differences in the number of considered centers in different parts of the study areas. Sometimes, an area may not even contain a single center. Although this can reflect some kind of the existing inequities caused by the uneven distribution of service centers, consideration of accessibility to an equal number of alternative centers as in the second approach seems to provide more in-depth and useful information on the inequity. Because, it leads to answer the question that which alternatives with how much additional costs (money, time, etc.) are available for each resident? This means that definition of access costs as a function of the positions of the existing alternative parks is a valuable information.
By considering the accessibilities to the equal number of services, inequities in access costs for an equal number of alternative services can be highlighted. So, in this paper, a destination set of three nearest parks is used for assessing the inequity of using the second and third closest services. Because of correlations between the distances to the first, second, and third closest parks, computation of the additional costs represented by the additional travel distances required to access the second and third nearest parks are used as the indicators of inequity. Let,
and
respectively represent distances of the residential parcel i to the first, second, and third service centers. Additional costs (AC) of visiting centers 2
and 3
by user i are computed as:
Inequity of ACs can then be considered by consideration of their variability among the residents.
2.4. Workflow steps for the accessibility and proposed user-based attractiveness modeling
The steps of modeling the proposed user-based attractiveness indices are as follows:
Collection, preprocessing and organization of the required data (base maps, parks, traffic, street networks, population)
Calculation and mapping of TTs from TAZs to the closest public park locations using the network analysis tools and traffic data.
Calculation of the distance and additional costs of accessing the first, second and third closest parks
Calculation of DUs and their inequities by using EquationEquations (5)
(5)
(5) and (Equation6
(6)
(6) ) and considering different lengths of FTs.
Definition of the
of parks (threshold) for calculation of FV.
Calculation of FVs and their inequities using a
of 15 min as an example, at different FTs using the EquationEquations (9)
(9)
(9) and (Equation10)
(10)
(10) .
Calculation of the second user-based attractiveness index (CSCs) and their inequities using the EquationEquation (16)
(16)
(16) and comparison with the conventional service-based approach.
The workflow of the proposed approach is outlined in . In the rest of the paper, practical tests and results are presented. The proposed user-based attractiveness indices have been measured and new dimensions of inequity have been revealed. Then, the service-based and the proposed user-based attractiveness indices in terms of DU, FV and CSC are computed and compared. Finally, the inequity of distance from the three closest parks is considered and additional access costs for the second and third alternative parks are measured and evaluated.
3. Practical experiments and results
3.1. Case study area
The case study area is Tehran megacity, the capital of Iran. Tehran has been divided into 22 metropolitan regions. Each region is divided into several districts, and finally, each district includes several local neighborhoods. In the latest official national census in 2016, Tehran had a population of 8,737,510 extended in over 640 km2. The city is divided into 560 TAZs. shows geographic location and regional park distribution in Tehran megacity as the case study area.
3.2. Data sets
3.2.1. Parks
Data of urban parks were obtained from the spatial information department of Tehran municipality and website of the Municipality (https://www.tehran.ir). Size and centroids of parks were then defined by the QGIS software. Only regional parks which are accessible by car were included in the assessment of the inequity.
3.2.2. Spatial unit of analysis
Because of the variability of working scales of the city planners ranging from the micro to macro scales, various scales and areal units have been used by researchers for spatial equity studies. As an example, Traffic Area Zones (TAZs) have been used in some other spatial accessibility and equity studies (e.g. García-Albertos et al. Citation2019; Yan-Yan et al. Citation2016). These zones together with the regional parks respectively have been used as the areal units of users and services in this study. Selection of traffic area zones as our basic geographic neighborhood units of analysis was for two reasons: 1) Computation of the inequity indices such as the frequency and duration of park use was based on the availability of travel times of the users. So, data of TTs, as one of the most critical parameters of this study, required traffic volume data, and TAZs are the most appropriate geographic units delineated based on the traffic volumes. 2) Resident population statistics were only available in the district and TAZs level in the study area.
3.2.3. Computation of TTs of residents
The Network Analyst, OD (origin-destination) Cost Matrix tool of QGIS were used to measure network travel distances between TAZs and regional parks by considering length as the impedance function. Due to the lack of reliable data on the exact location of city park entrances, centroids of TAZs and regional parks were used as the origins and destinations, respectively. Real-time traffic conditions of the study area were monitored and mapped for five samples of varying traffic conditions during the working day times at 7:00 a.m., 9:00 a.m., 12:00 a.m., 3:00 p.m., and 6:00 p.m., using the Google Maps. Different traffic patterns and representativeness of these times in the study area, were the main reason for their selection. In Google traffic maps, street segments are constantly labeled with three traffic classes named “Light”, “Moderate” and “Heavy” which are respectively equivalent to maximum speeds of 80, 50 and 30 km/h according to the local traffic control agencies. For the computation of TTs in different ODs, the existing web-based local route planning tools based on the Google maps were used to calculate the average speeds in each of the three traffic classes. These respectively resulted in average speeds of 10, 24, and 58 km/h for the heavy, medium, and light traffic classes. Then, the calculated average speeds and traffic maps of five different times were used to compute the total TTs for ODs by measuring and summation of the TTs of different segments of the networks connecting each TAZ to the regional parks.
3.3. Evaluation of the spatial distribution and inequities of the user-based attractiveness indices
To evaluate the inequity of TAZs in terms of their DUs, the network distances between each TAZ and its nearest park were calculated and converted to TT based on the traffic volume data as explained in Section 3.2.3. Results indicated that the maximum TT from the TAZs to their nearest neighborhood park is 20 minutes.
3.3.1. Spatial distribution and inequities of DU
By assuming equal TTs for going to and coming back from their nearest park, in the worst case, TAZs need about 40 minutes to visit their nearest park. So, double of this TT, 80 min, was selected as the maximum FT for simulation of the variability of DUs among the TAZs using EquationEquations (5)(5)
(5) and (Equation6
(6)
(6) ) and equity of VDs and FTs. Simulation included consideration of the spatiotemporal variability of DUs resulting from application of five FTs of 20, 30, 40, 60 and 80 min for TAZs. highlights the five resulting maps of DUs.
Figure 4. DUs of TAZs at different equal FTs of 20 min (1st row, left), 30 min (1st row, right), 40 min (2nd row, left), 60 min (2nd row, right) and 80 min (3rd row).

Observation of the maps of DU shows significant differences between DUs of different TAZs for equal FTs. For instance, considering a public FT of 40 min, some TAZs with DU = 0, are not able to visit their nearest park. Whereas, in equal condition of FT, some others are highlighted with DUs of at least 30 min to visit their nearest parks (, 2nd row, left). Almost in all FTs, the shortest DUs belong to the TAZs located in the east, south west, and some scattered parts of north and west of Tehran. By increasing FTs from 20 to 80 min, the minimum DU of TAZs increases from 0 to 39 min. The range of DUs (differences between the minimum and maximum values) increase from 18 to 39 min respectively at FTs of 20 and 80 min. These results demonstrate the increase of the number of visitors of parks and at the same time increase of the inequity of DUs in higher FTs.
3.3.2. Spatial distribution and inequities of FV
For the purpose of highlighting the frequency of visit of the park (FV) and the related inequities among the users, different values of DU may be used as the threshold, reflecting the minimum acceptable value of DU, to visit a park. As an example, we used a threshold value of 15 minutes for DU to convert all five maps of to binary maps. Each map shows two classes of i-visit and ii-no visit, respectively showing TAZs with DUs of greater or equal to and less than the threshold DU of 15 minutes. The resulting maps were then combined using the EquationEquation (10)(10)
(10) to produce the map of FVs and their spatial inequities, in FT ranges of 20 to 80 minutes in the study area ().
Figure 5. Frequency of the closest park visit (FV) by TAZs in FT ranges of 20 to 80 minutes with minimum DU of 15 minutes.

Results of mapping the spatial distribution of FV, as highlighted in , show that majority of TAZs (about 66%) in the study area are classified with FVs of 4. On the other hand, about 25% of TAZs are highlighted with maximum value of 5 for their FVs. TAZs with smaller values of 2 and 3 for their FVs occupy about 9% of the area and highlight the most deprived TAZs in the area.
3.3.3. Spatial distribution and inequities of CSC
Service-based and user-based attractiveness indices for the first nearest parks are respectively computed using EquationEquations (2)(2)
(2) and (Equation16
(16)
(16) ), and their results are compared in . As discussed in Section 2.2, service-based and minimum values of the user-based attractiveness indices show a one-to-one correspondence. As compared to the static values of service-based index, the user-based index shows higher variability and discrimination power of the attractiveness between different users of a service. This is more significant in service catchments with higher density of users, where the service-based index shows a single value of low attractiveness and it is not able to discriminate the variability of attractiveness among the users located in the close neighborhood or the boundary of the service catchments. Whereas, the user-based index, shows high variability in lower values of the service-based approach. Therefore, consideration of relations between the differences of the two indices and service-based index as in shows negative relations.
Figure 6. Comparison of the user-based (vertical axis) and service-based (horizontal axis) attractiveness indices (CSC) of the first nearest parks.

Figure 7. Relations between the service-based (horizontal axis) and differences of the two service-based and user-based indices (vertical axis).

3.3.3.1. Evaluation of the accessibility to alternative parks
For measuring inequity of the accessibility of residents to their closest parks, travel distances between pairs of TAZs and their 3-nearest parks are measured. Results show that distances vary between 0.1 to 18 kilometers and average distances to the first, second, and third nearest parks are respectively 3, 4, and 5 kilometers. However, variance of access distances to the first, second, and third nearest parks are approximately similar and equal to 1.8 kilometers which are considerably high.
For identification of hot spots, the areas with interminable distances to the first, second, and third closest parks, TAZs were classified in three distance classes. These included the short (SD), medium (MD), and long (LD) respectively with distances of less than 5, 5 to 7.5, and over 7.5 km. By assuming the minimum driving speed of 30 km/hour, 7.5 km represents the local standard TT of 15 minutes driving to the closest park in Tehran. Therefore, the LD class can represent the deprived areas or hotspots. Because of the focus of the study on hotspots, only maps of hotspots to the three closest parks were produced by intersection of the LDs of the three corresponding maps . These classes are highlighted as the LD1, LD2 and LD3 respectively representing hotspots for the first, second, and third closest parks. Because of correlations between the distances to the first, second and third closest parks, (e.g. distance for the first park is shorter than the distances for other parks), LD1 coincides with LD2 and LD3 classes and therefore hotspot for the first closest park represents the most deprived areas. LD2-LD3 represents areas with less than 7.5 km for the first closest park and over 7.5 km to the second and third closest parks. Finally, LD3 shows areas with interminable distances only to the third closest park.
Figure 8. Hot spots showing areas in interminable distances (over 7.5 km) to the first (LD1), second (LD2) and third (LD3) nearest parks.

Besides considering distances to the three closest parks, the inequity of Additional Costs (ACs) for use of the second and third closest parks by each user was computed and compared using EquationEquation (17).(17)
(17) Relations between ACs for use of the second and third parks are shown in .
Figure 9. Relations between ACs for use of the second (horizontal axis) and third closest parks (vertical axis) in the study area.

Because of the uneven distribution and concentration of services in some parts of the study area, a large number of ACs occur in 1000–1500 and 1000–2500 m respectively for use of the second and third nearest parks . So, about 80% of the population have to travel the maximum additional distances of 1500 and 2500 m, respectively, to access their second and third nearest parks.
highlights the classified map of ACs in three classes of low (less than 1.5 km), medium (1.5 to 3 km) and high (over 3 km). Majority of the deprived residential parcels for use of the second and third alternatives are located on the margins of the study area, because of the higher density of parks in the central parts of the study area.
4. Discussion
Examination of the spatial distribution of regional parks shows that a considerable number of regional parks are in areas with lower population densities, especially in the western parts of the city. This may be because of the large bare lands available in the western parts of Tehran (regions 21 and 22) and construction of parks and lunar cities around the city to accommodate the overflow population.
Although in the conventional service-based accessibility studies, different users of a service are discriminated by their distance from the closer service centers, but the distance is more related to the costs rather than the attractiveness. Whereas, principal concern of the proposed user-based approach is the attractiveness, or what one receives and pays the costs for. As an example, two residents in a service catchment may be in similar conditions of distances or costs to reach their closest park. But the similarity of these costs does not mean they will face with similar services, attractions and opportunities to choose and enjoy the park facilities. The proposed user-based attractiveness introduces three novel parameters including the DU, FV and CSC based on three concepts of TT, FT and population distribution.
Comparison of DUs of users proves that there is a deep inequity among users in terms of their durations of using parks. In a way that, in some cases, this inequity reaches about 40 min . Considering a minimum acceptable DU for visiting regional parks, another dimension of inequity called inequity in FV is explored. Longer FTs and shorter TTs will increase the FVs. Therefore, considering equal FTs for all users, users with low TTs have a higher chance to more frequently use their services.
The presented analysis showed that the service catchment is variable in time depending directly on the TTs and FTs of the users. Then the instant service catchments can be larger or even smaller than the conventional distance-based service catchments. However, the conventional service-based methods are based on an unrealistic fixed service catchment resulting from the distance-based definition.
Comparison of the user-based and service-based methods of attractiveness measurement proves that in contrast to the service-based approach, results of the user-based approach show higher variability for a value of the service-based index which decreases with the increase of the service-based index. This shows the discrimination power of the proposed approach for highlighting attractiveness inequities inside the service areas. High variability of the user-based approach in lower values of the service-based index can be attributed to a higher population and larger service areas leading to the high variation of both the, and user-based index and low values of the service-based indices. Lower population rates and smaller service areas lead to higher values of the service-based and low variation of the user-based indices. Such that, large values of the service-based index coincide with higher values and lower data range of the user-based index.
As expected, the service-based and minimum values of the user-based indices show inverse linear relations. This happens in service area boundaries where the number of populations used in calculation of both indices are equal. The principal reason for the linear relation between data of service-based and minimum values of user-based approaches is because of similarities of their mathematical structures. User-based approach is minimized when is maximized (or
is minimized) and this is when users are in boundaries of service areas and max (
) =
.
These results show particular usefulness of the user-based approach in areas highlighted with the low attractiveness indices in the service-based approach. By assigning variable values for the attractiveness of a service center, the user-based index leads to the discrimination of users according to their position in relation to the center and to the other users inside the service areas.
It may be argued that these inequities can be discriminated by considering the distance/TT of different users to the closest service centers. But two important factors of the accessibility including the i- distance or TT as the spatial impedance between demand and service (supply), and ii-attractiveness or availability of services should be discriminated. Attractiveness of a service is mainly concerned with what service such as the space, how long (DU) and how often (FV) is available which is quite different and independent of the one dimensional data of network connecting a user to a service.
An important advantage of the proposed approach is its sensitivity to the two-dimensional data of population distribution and their relative positions within the service areas. Because, population of users have important impacts on the quality and availability of services and show irregular patterns of distribution which rarely follow those of the even distribution patterns. As an example, shows relations between the population and TT from the closest parks of users in the study area. Results show the irregular patterns of population distribution resulting in nonlinear relations between the population and TT from the first closest parks in the study area.
Figure 11. Distribution of the number of close) and far
) residents (respectively shown by the red and blue colors in vertical axis) in relation to TT of users (horizontal axis) from the first closest parks.

verifies that distribution of population around services shows a complicated, uneven and anisotropic pattern of distribution. As shown in , the number of far residents in relation to TT of a user from the closest park show high variability in closer distances of less than 5,000 meters. In contrast, number of close residents in relation to TT of a user from the closest park show high variability in longer TTs of over 5 minutes. Therefore, consideration of population distribution in varying TTs as in the proposed user-based attractiveness approach provides detailed and additional information on inequity of CSC, because of the interactions between the demanding population and TT. This information is considerably different with what is obtained by separate consideration of the TT of users from the corresponding services and the whole demanding population in the service areas. As an example, highlights variance of user-based attractiveness for the first, second and third closest parks. These parks have been selected by having a variance of over 3 in their user-based index. Single values of service-based attractiveness for these parks are also provided for the purpose of comparison. As seen, the variance of attractiveness within service areas varies between 3 and 12 and shows high variability and considerable inequities within the service areas.
Table 1. Higher variability of the user-based attractiveness indices as compared to the single value provided by the service-based approach for attractiveness of some selected parks as the first, second and third closest parks.
For spatial visualization of the differences between the service-based and user-based attractiveness indices (CSC), two resulting maps of these indices were subtracted and their differences were classified into two classes of high (over 3 square meters per capita) and low differences (less than 3 square meters per capita). highlights the results. As expected, the user-based index is mostly higher or equal with the service-based attractiveness index. The highest difference between the two indices occurs around the services with higher population densities in their service areas, where differences between the and
is maximum. This shows considerable shortcomings of the service-based attractiveness index in underestimating the attractiveness for residents in closer TTs to services. While by moving away from services and approaching the boundaries of service areas and also at TAZs with lower population densities, values of
and
get closer and hence their differences are reduced.
Figure 12. Spatial distribution of two difference classes (more than and less than 3 square meters, respectively highlighted with red and white colors) resulting from the subtraction of the user-based and service-based attrativeness indices in the study area.

Finally, assessing the accessibility to alterative parks shows that the most deprived areas in terms of the access distance to the three nearest parks are in the margins of the study area, mostly at eastern and southwestern parts. Residents should pass average distances of 1100 and 1800 meters to reach their second and third nearest parks.
5. Conclusions
In this paper, a user-based approach for evaluating the spatial inequity of attractiveness of services is developed to address the deficiencies of defining service attractiveness by a single static value for each service. In the proposed approach, the attractiveness of services is considered as a dynamic parameter that depends on the user position in relation to the service and varies with their positions. In this definition, the attractiveness of service for a particular user is modeled using the TT and FT of users and positions of the demanding population in relation to the service and user. Compared to the traditional service-based approach, the proposed approach is based on a more realistic assumption, in which service would be more attractive for its neighboring users than those in farther locations. So, instead of using a unique value for the attractiveness of service, a matrix of values showing the attractiveness of service for different users is presented.
In the proposed user-based approach, we considered two innovative indices of DU and FV to explore the other important aspects of inequities among residents in local scales along with consideration of the user-based CSC in comparison to the traditional service-based CSC. However, the proposed approach is more suitable for highlighting inequities in local scales within and between the services. It may not replace but can complement the well-known service-based attractiveness index which is more adapted for comparison of attractiveness and per capita space of different service centers in regional scales. A comparison of relations between the proposed user-based and service-based indices shows the ability of the user-based approach for highlighting the variability of attractiveness within the service areas. The variability of the user-based index is higher for low values of service-based indices in highly populated larger service areas. This variability decreases with the increase of the service-based index. These show capabilities of the proposed approach for highlighting inequities in deprived service areas. Two indices tend to become equal when the user-based index reaches its minimum, for residents in boundaries of the service areas. Therefore, it can be concluded that the service-based method provides a pessimistic view and assumption that all residents will simultaneously attend the park, resulting in assignment of the minimum values of attractiveness to each service and ignorance of the inequities and underestimation of the attractiveness of a park for different users.
An important application of the proposed user-based approaches is the effective mapping of the spatial inequities in local scales, which can be of interest for more efficient decision-making and inequity management by urban planners and municipalities. Also, this information can be of concern for the public and residents of service areas. Comparison of the additional access costs for use of the second and third alternative parks reveals significant inequities. As an example, only about 40% of the population can reach their third nearest parks within the additional distances of less than 2 kilometers, and the others should travel longer additional distances.
Lack of detailed information about the three factors of parks as the source, users as the demanders, and travel times as the costs, can be regarded as the most important limiting factors for wider applicability of the proposed user-based attractiveness index. Employment of the size, as an indicator of the existing capacities of a park, may lead to unrealistic results. However, use of this indicator is common and may be justifiable, due to lack of detailed information about the space and service characteristics of the parks and the fact that usually larger parks have more diverse and attractive facilities and services. There is need to collect and classify detailed information which well represents the capacities of parks for different activities and users. Collection and incorporation of detailed information about the demographic characteristics of service users, their free times, spatial distribution, interests and preferences can lead to more efficient analysis and classification of different user groups. Real time spatial data of park use and travel times are also required for spatiotemporal user preference analysis and real time demand and attractiveness assessment. This study, has been focused on the regional parks, and can be extended to other park types and mapping of the variability of their attractiveness.
Disclosure statement
No potential conflict of interest was reported by the author(s).
Data availability statement
The data that support the findings of this study were derived from the following resources available in the public domains: “https://map.tehran.ir”; “https://www.google.com/maps/@35.7138432,51.3396774,12z” and “https://www.amar.org.ir”. These data are also available from the corresponding author, Alimohammadi, A. upon reasonable request.
Additional information
Funding
Notes on contributors
Tahere Ghaemi Rad
Tahereh Ghaemi Rad is a PhD candidate in GIS Dept. of the Faculty of Geomatics Engineering. She received the Master degree from the Center of Excellence in Geospatial Information Technology, K.N.Toosi University of Technology. Her research interests are spatial/social equity analysis and smart city problems.
Abbas Alimohammadi
Abbas Alimohammadi is an emeritus professor in GIS Dept. and Center of Excellence in Geospatial Information Technology, Faculty of Geomatics Engineering, K. N. Toosi University of Technology. He received the PhD degree from the Australian National University. His research interests are spatial data science, GIS/spatial analysis and modeling, spatial optimization and data mining in urban and environmental modeling problems.
References
- Anjomshoaa, E., B. H. Lamit, A. Shafaghat, T. Hayat Khan, and S. Mahdzar. 2017. “Accessibility Measurement Techniques in Urban Studies:A Comprehensive Review.” Journal of Biodiversity and Environmental Sciences (JBES) 10 (6): 92–106.
- Arranz-López, A., J. A. Soria-Lara, C. López-Escolano, and Á. P. Campos. 2017. “Retail Mobility Environments: A Methodological Framework for Integrating Retail Activity and Non-Motorized Accessibility in Zaragoza, Spain.” Journal of Transport Geography 58: 92–103. doi:10.1016/j.jtrangeo.2016.11.010.
- Arranz-López, A., J. A. Soria-Lara, and Á. Pueyo-Campos. 2019. “Social and Spatial Equity Effects of Non-Motorized Accessibility to Retail.” Cities 86: 71–82. doi:10.1016/j.cities.2018.12.012.
- Ashik, F. R., S. A. Mim, and M. N. Neema. 2020. “Towards Vertical Spatial Equity of Urban Facilities: An Integration of Spatial and Spatial Accessibility.” Journal of Urban Management 9 (1): 77–92. doi:10.1016/j.jum.2019.11.004.
- Badura, T., S. Ferrini, M. Burton, A. Binner, and I. J. Bateman. 2020. “Using Individualized Choice Maps to Capture the Spatial Dimensions of Value Within Choice Experiments.” Environmental and Resource Economics 75 (2): 297–322. doi:10.1007/s10640-019-00358-3.
- Bateman, I. J., B. H. Day, S. Georgiou, and I. Lake. 2006. “The Aggregation of Environmental Benefit Values: Welfare Measures, Distance Decay and Total WTP.” Ecological Economics 60 (2): 450–460. doi:10.1016/j.ecolecon.2006.04.003.
- Bennett, R. J. 1983. “Individual and Territorial Equity.” Geographical Analysis 15 (1): 50–57. doi:10.1111/j.1538-4632.1983.tb00763.x.
- Brook, O. 2016. “Spatial Equity and Cultural Participation: How Access Influences Attendance at Museums and Galleries in London.” Cultural Trends 25 (1): 21–34. doi:10.1080/09548963.2015.1134098.
- Chang, H. S., and C. H. Liao. 2011. “Exploring an Integrated Method for Measuring the Relative Spatial Equity in Public Facilities in the Context of Urban Parks.” Cities 28 (5): 361–371. doi:10.1016/j.cities.2011.04.002.
- Dadashpoor, H., and F. Rostami. 2017. “Measuring Spatial Proportionality Between Service Availability, Accessibility and Mobility: Empirical Evidence Using Spatial Equity Approach in Iran.” Journal of Transport Geography 65: 44–55. doi:10.1016/j.jtrangeo.2017.10.002.
- Dadashpoor, H., F. Rostami, and B. Alizadeh. 2016. “Is Inequality in the Distribution of Urban Facilities Inequitable? Exploring a Method for Identifying Spatial Inequity in an Iranian City.” Cities 52: 159–172. doi:10.1016/j.cities.2015.12.007.
- Delafontaine, M., T. Neutens, T. Schwanen, and N. Van de Weghe. 2011. “The Impact of Opening Hours on the Equity of Individual Space–Time Accessibility.” Computers, Environment and Urban Systems 35 (4): 276–288. doi:10.1016/j.compenvurbsys.2011.02.005.
- Fasihi, H., and T. Parizadi. 2020. “Analysis of Spatial Equity and Access to Urban Parks in Ilam, Iran.” Journal of Environmental Management 260: 110–122. doi:10.1016/j.jenvman.2020.110122.
- Gao, K., Y. Yang, A. Li, and X. Qu. 2021. “Spatial Heterogeneity in Distance Decay of Using Bike Sharing: An Empirical Large-Scale Analysis in Shanghai.” Transportation Research Part D: Transport and Environment 94: 102814. doi:10.1016/j.trd.2021.102814.
- García-Albertos, P., M. Picornell, M. H. Salas-Olmedo, and J. Gutiérrez. 2019. “Exploring the Potential of Mobile Phone Records and Online Route Planners for Dynamic Accessibility Analysis.” Transportation Research Part A: Policy and Practice 125: 294–307. doi:10.1016/j.tra.2018.02.008.
- Goel, R. 2018. “Distance-Decay Functions of Travel to Work Trips in India.” Data in Brief 21: 50–58. doi:10.1016/j.dib.2018.09.096.
- Halás, M., P. Klapka, and P. Kladivo. 2014. “Distance-Decay Functions for Daily Travel-To-Work Flows.” Journal of Transport Geography 35: 107–119. doi:10.1016/j.jtrangeo.2014.02.001.
- Hansen, W. G. 1959. “How Accessibility Shapes Land Use.” Journal of the American Institute of Planners 25 (2): 73–76. doi:10.1080/01944365908978307.
- Hodgart, R. 1978. “Optimizing Access to Public Services: A Review of Problems, Models and Methods of Locating Central Facilities.” Progress in Geography 2 (1): 17–48. doi:10.1177/030913257800200103.
- Hu, W., J. Tan, M. Li, J. Wang, and F. Wang. 2020. “Impact of Traffic on the Spatiotemporal Variations of Spatial Accessibility of Emergency Medical Services in Inner-City Shanghai.” Environment and Planning B: Urban Analytics and City Science 47 (5): 841–854. doi:10.1177/2399808318809711.
- Johnston, R. J., E. Y. Besedin, and B. M. Holland. 2019. “Modeling Distance Decay Within Valuation Meta-Analysis.” Environmental and Resource Economics 72 (3): 657–690. doi:10.1007/s10640-018-0218-z.
- Joseph, A. E., and P. R. Bantock. 1982. “Measuring Potential Physical Accessibility to General Practitioners in Rural Areas: A Method and Case Study.” Social Science & Medicine 16 (1): 85–90. doi:10.1016/0277-9536(82)90428-2.
- Karner, A. 2018. “Assessing Public Transit Service Equity Using Route-Level Accessibility Measures and Public Data.” Journal of Transport Geography 67: 24–32. doi:10.1016/j.jtrangeo.2018.01.005.
- Kelobonye, K., G. McCarney, J. C. Xia, M. S. H. Swapan, F. Mao, and H. Zhou. 2019. “Relative Accessibility Analysis for Key Land Uses: A Spatial Equity Perspective.” Journal of Transport Geography 75: 82–93. doi:10.1016/j.jtrangeo.2019.01.015.
- Kompil, M., C. Jacobs-Crisioni, L. Dijkstra, and C. Lavalle. 2019. “Mapping Accessibility to Generic Services in Europe: A Market-Potential Based Approach.” Sustainable Cities and Society 47: 101372. doi:10.1016/j.scs.2018.11.047.
- Laghai, H., and H. Bahmanpour. 2012. “GIS Application in Urban Green Space per Capita Evaluation: (Case Study: City of Tehran).” Annals of Biological Research 3 (5): 2439–2446.
- Łaszkiewicz, E., A. Heyman, X. Chen, Z. Cimburova, M. Nowell, and D. N. Barton. 2022. “Valuing Access to Urban Greenspace Using Non-Linear Distance Decay in Hedonic Property Pricing.” Ecosystem Servicess 53: 101394. doi:10.1016/j.ecoser.2021.101394.
- Lee, G., and I. Hong. 2013. “Measuring Spatial Accessibility in the Context of Spatial Disparity Between Demand and Supply of Urban Park Service.” Landscape and Urban Planning 119: 85–90. doi:10.1016/j.landurbplan.2013.07.001.
- Leung, M., C. B. Chow, P. K. P. Ip, and P. Y. Siu-Fai. 2020. “Geographical Accessibility of Community Social Services and Incidence of Self-Harm.” Spatial and Spatio-Temporal Epidemiology 33: 100334. doi:10.1016/j.sste.2020.100334.
- Litman, T. 2017. “Evaluating Transportation Equity.” World Transport Policy & Practice 8 (2): 50-65.
- Lotfi, S., and M. J. Koohsari. 2009. “Measuring Objective Accessibility to Neighborhood Facilities in the City (A Case Study: Zone 6 in Tehran, Iran).” Cities 26 (3): 133–140. doi:10.1016/j.cities.2009.02.006.
- Lu, C., Z. Zhang, and X. Lan. 2019. “Impact of China’s Referral Reform on the Equity and Spatial Accessibility of Healthcare Resources: A Case Study of Beijing.” Social Science & Medicine 235: 112386. doi:10.1016/j.socscimed.2019.112386.
- Makri, M. C., and C. Folkesson. 1999. “Accessibility Measures for Analyses of Land Use and Travelling with Geographical Information Systems.” In Proceedings from the Annual Transport Conference at Aalborg University Proceedings from the Annual Transport Conference at Aalborg University 6 (1): 1-17.
- Martínez, L. M., and J. M. Viegas. 2013. “A New Approach to Modelling Distance-Decay Functions for Accessibility Assessment in Transport Studies.” Journal of Transport Geography 26: 87–96. doi:10.1016/j.jtrangeo.2012.08.018.
- McGrail, M. R., and J. S. Humphreys. 2009. “Measuring Spatial Accessibility to Primary Care in Rural Areas: Improving the Effectiveness of the Two-Step Floating Catchment Area Method.” Applied Geography 29 (4): 533–541. doi:10.1016/j.apgeog.2008.12.003.
- Mishra, S., P. K. Sahu, A. K. Sarkar, B. Mehran, and S. Sharma. 2019. “Geo-Spatial Site Suitability Analysis for Development of Health Care Units in Rural India: Effects on Habitation Accessibility, Facility Utilization and Zonal Equity in Facility Distribution.” Journal of Transport Geography 78: 135–149. doi:10.1016/j.jtrangeo.2019.05.017.
- Monzón, A., E. Ortega, and E. López. 2013. “Efficiency and Spatial Equity Impacts of High-Speed Rail Extensions in Urban Areas.” Cities 30: 18–30. doi:10.1016/j.cities.2011.11.002.
- Mooney, S. J., K. Hosford, B. Howe, A. Yan, M. Winters, A. Bassok, and J. A. Hirsch. 2019. “Freedom from the Station: Spatial Equity in Access to Dockless Bike Share.” Journal of Transport Geography 74: 91–96. doi:10.1016/j.jtrangeo.2018.11.009.
- Neutens, T., T. Schwanen, F. Witlox, and P. De Maeyer. 2010. “Equity of Urban Service Delivery: A Comparison of Different Accessibility Measures.” Environment & Planning A 42 (7): 1613–1635. doi:10.1068/a4230.
- Nicholls, S. 2001. “Measuring the Accessibility and Equity of Public Parks: A Case Study Using GIS.” Managing Leisure 6 (4): 201–219. doi:10.1080/13606710110084651.
- Oh, K., and S. Jeong. 2007. “Assessing the Spatial Distribution of Urban Parks Using GIS.” Landscape and Urban Planning 82 (1–2): 25–32. doi:10.1016/j.landurbplan.2007.01.014.
- Omer, I. 2006. “Evaluating Accessibility Using House-Level Data: A Spatial Equity Perspective.” Computers, Environment and Urban Systems 30 (3): 254–274. doi:10.1016/j.compenvurbsys.2005.06.004.
- Panagiotopoulos, G., and D. Kaliampakos. 2019. “Accessibility and Spatial Inequalities in Greece.” Applied Spatial Analysis and Policy 12 (3): 567–586. doi:10.1007/s12061-018-9256-8.
- Rich, D. C. 1978. “Population Potential, Potential Transportation Cost and Industrial Location.” Area 10: 222–226.
- Rosero-Bixby, L. 2004. “Spatial Access to Health Care in Costa Rica and Its Equity: A GIS-Based Study.” Social Science & Medicine 58 (7): 1271–1284. doi:10.1016/S0277-9536(03)00322-8.
- Schaafsma, M., R. Brouwer, A. Gilbert, J. Van Den Bergh, and A. Wagtendonk. 2013. “Estimation of Distance-Decay Functions to Account for Substitution and Spatial Heterogeneity in Stated Preference Research.” Land Economics 89 (3): 514–537. doi:10.3368/le.89.3.514.
- Smoyer‐tomic, K. E., J. N. Hewko, and M. J. Hodgson. 2004. “Spatial Accessibility and Equity of Playgrounds in Edmonton, Canada.” Canadian Geographer/Le Géographe Canadien 48 (3): 287–302. doi:10.1111/j.0008-3658.2004.00061.x.
- So, S. W. 2016. “Urban Green Space Accessibility and Environmental Justice: A GIS-Based Analysis in the City of Phoenix, Arizona.” A Thesis Presented to the Faculty of the USC Graduate School. University of Southern California.
- Söderberg, M., and D. N. Barton. 2014. “Marginal WTP and Distance Decay: The Role of ‘Protest’ and ‘True Zero Responses in the Economic Valuation of Recreational Water Quality.” Environmental and Resource Economics 59 (3): 389–405. doi:10.1007/s10640-013-9735-y.
- Stępniak, M., and S. Goliszek. 2017. “Spatiotemporal Variation of Accessibility by Public Transport—the Equity Perspective.” In the Rise of Big Spatial Data Springer, Cham 241–261.
- Tahmasbi, B., M. H. Mansourianfar, H. Haghshenas, and I. Kim. 2019. “Multimodal Accessibility-Based Equity Assessment of Urban Public Facilities Distribution.” Sustainable Cities and Society 49: 101633. doi:10.1016/j.scs.2019.101633.
- Taleai, M., R. Sliuzas, and J. Flacke. 2014. “An Integrated Framework to Evaluate the Equity of Urban Public Facilities Using Spatial Multi-Criteria Analysis.” Cities 40: 56–69. doi:10.1016/j.cities.2014.04.006.
- Talen, E. 1998. “Visualizing Fairness: Equity Maps for Planners.” Journal of the American Planning Association 64 (1): 22–38. doi:10.1080/01944369808975954.
- Talen, E., and L. Anselin. 1998. “Assessing Spatial Equity: An Evaluation of Measures of Accessibility to Public Playgrounds.” Environment & Planning A 30 (4): 595–613. doi:10.1068/a300595.
- Tan, P. Y., and R. Samsudin. 2017. “Effects of Spatial Scale on Assessment of Spatial Equity of Urban Park Provision.” Landscape and Urban Planning 158: 139–154. doi:10.1016/j.landurbplan.2016.11.001.
- Tsou, K. W., Y. T. Hung, and Y. L. Chang. 2005. “An Accessibility-Based Integrated Measure of Relative Spatial Equity in Urban Public Facilities.” Cities 22 (6): 424–435. doi:10.1016/j.cities.2005.07.004.
- Van Wee, B. 2016. “Accessible Accessibility Research Challenges.” Journal of Transport Geography 51: 9–16. doi:10.1016/j.jtrangeo.2015.10.018.
- Wang, S. I., and C. L. Yaung. 2013. “Vertical Equity of Healthcare in Taiwan: Health Services Were Distributed According to Need.” International Journal for Equity in Health 12 (1): 1–8. doi:10.1186/1475-9276-12-12.
- Whitehead, J., A. L. Pearson, R. Lawrenson, and P. Atatoa-Carr. 2020. “Defining General Practitioner and Population Catchments for Spatial Equity Studies Using Patient Enrolment Data in Waikato, New Zealand.” Applied Geography 115: 102137. doi:10.1016/j.apgeog.2019.102137.
- Wismadi, A. 2015. Equity-Based Resource Allocation for Infrastructure Development. Netherlands: University of Twente.
- Xiao, Y., Z. Wang, Z. Li, and Z. Tang. 2017. “An Assessment of Urban Park Access in Shanghai–Implications for the Social Equity in Urban China.” Landscape and Urban Planning 157: 383–393. doi:10.1016/j.landurbplan.2016.08.007.
- Yamaguchi, R., and P. Shah. 2020. “Spatial Discounting of Ecosystem Services.” Resource and Energy Economics 62: 101186. doi:10.1016/j.reseneeco.2020.101186.
- Yan-Yan, C., W. Pan-Yi, L. Jian-Hui, F. Guo-Chen, L. Xin, and G. Yi. 2016. “An Evaluating Method of Public Transit Accessibility for Urban Areas Based on GIS.” Procedia Engineering 137: 132–140. doi:10.1016/j.proeng.2016.01.243.
- Zeng, W., L. Xiang, H. Li, X. Zhang, and H. Zhang. 2017. “Spatial Pattern of Access to Health Care Facilities and Its Formation Mechanism in Nanjing.” Economic Geography 37: 136–143.
- Zhang, X., H. Lu, and J. B. Holt. 2011. “Modeling Spatial Accessibility to Parks: A National Study.” International Journal of Health Geographic 10 (1): 31. doi:10.1186/1476-072X-10-31.
- Zhang, Y., H. Yang, and J. Pan. 2019. “Maximum Equity in Access of Top-Tier General Hospital Resources in China: A Spatial Optimization Model.” The Lancet 394: S90. doi:10.1016/S0140-6736(19)32426-2.
- Zheng, Z., H. Xia, S. Ambinakudige, Y. Qin, Y. Li, Z. Xie, L. Zhang, and H. Gu. 2019. “Spatial Accessibility to Hospitals Based on Web Mapping API: An Empirical Study in Kaifeng, China.” Sustainability 11 (4): 1160. doi:10.3390/su11041160.