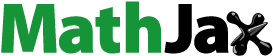
ABSTRACT
Marine aquaculture is one of the most important marine resource utilization types, widely distributed in seashore areas. Because mass marine aquaculture development will lead to the eutrophication disaster of macroalgae blooms (green tide), it is imperative to discover the latent relationship. Inspired by the big data analysis theory, the spatiotemporal correlation change in 2017 and 2019 of the marine aquaculture and macroalgae blooms in Jiangsu Shoal in the West of the Yellow Sea and its adjacent seas were monitored and extracted respectively based on multi-source remote sensing images. Through optical and Synthetic Aperture Radar (SAR) images, the imaging characteristics of marine aquaculture in different growth cycles and sea conditions are analyzed. The relationship between the green tide and marine aquaculture harvest was explored from the early green algae distribution and spatiotemporal distribution changes. The earliest harvest area of marine aquaculture is the exact location of the outbreak of green tide. Along with the rapid reduction of aquaculture area, the distribution range of green tide gradually increased. The two showed opposite trends through many multi-source remote sensing data statistical analyses. Therefore, they have a strong correlation, which can be utilized to offer a significant value for the balance of marine environmental protection and resource development.
1 Introduction
China has the world’s most significant marine aquaculture industry (FAO Citation2018), which provides enormous marine economic benefits. At the same time, the quick expansion of the marine aquaculture industry also brings related environmental eutrophication issues. Driven by economic interests, some regions have developed aquaculture disorderly or irrationally according to the local environmental carrying capacity. Large-scale aquacultural reclamation or offshore aquaculture has decreased the area of the sea and reduced the amount of tidal absorption, which has weakened the self-purification ability of the ocean and aggravated the deterioration of the water environment (Ottinger, Clauss, and Kuenzer Citation2016). The owner blindly constructs net cages and erects hanging rafts on the sea, resulting in excessive breeding density, which far exceeds the marine ecosystem’s bearing capacity, leading to obstruction and disorder of the logistics and energy flow cycle in the marine aquaculture ecosystem. Since bait, debris, fish, shrimp feces, and domestic waste by breeding workers decompose, it produces a large amount of nitrogen and phosphorus nutrients, which leads to eutrophication in the breeding waters, and provides a suitable ecological environment for green tide organisms (Cao et al. Citation2007).
Remote sensing technology has become a proven and integral approach to monitoring marine aquaculture and other marine environmental applications (Lee et al. Citation2006; Zhao et al. Citation2023), with the advantages of wide-range synchronous observation. Synthetic Aperture Radar (SAR) is active remote sensing, which has the ability of wide-area coverage and all-weather observation of the earth. In cloudy and foggy coastal areas, SAR has a significant advantage in monitoring marine aquaculture (Fan et al. Citation2015, Citation2019). Many researchers have carried out the imaging analysis and automatic extraction of marine aquaculture based on different satellites, such as ENVISAT, Radarsat-2, GF-3, etc. The image contrast between the laver targets and the seawater is evaluated by using various polarimetric features with the laver cultivation as the research area (Sugimoto, Ouchi, and Nakamura Citation2012). Taking marine floating rafts in Beidaihe as the research area, wavelet transform and gray-level co-occurrence matrix are used to detect it, and then the joint sparse representation classification method is used to achieve high-precision detection of aquaculture areas (Fan et al. Citation2015). Compared to SAR images, optical satellite remote sensing images have vibrant spectral features. Through the analysis of spectral data and the combination of representative bands, marine aquaculture information is extracted (Hou et al. Citation2022; Sun et al. Citation2021). Although the optical remote sensing imagery is influenced by weather conditions, it has fewer speckle noises and more semantic information. By analyzing the characteristics of shape regularity and extracting the boundary information, the offshore marine aquaculture areas of China are extracted (Kuang et al. Citation2022; Liu et al. Citation2020; Wang et al. Citation2017). Some recognition methods (Xu, Zhang, and Wang Citation2018; Liu et al. Citation2019; Shi et al. Citation2018), such as human-computer interaction interpretation, nearest neighborhood analysis, threshold, object-oriented, and deep learning neural networks, are proposed to conduct high-precision remote sensing monitoring of marine aquaculture.
Recently, deep learning neural networks have received extensive attention in the remote sensing image processing field because of their accuracy, stability, and robustness in image segmentation tasks (Bai et al. Citation2022; Oktay et al. Citation2018). U-net (Ronneberger, Fischer, and Brox Citation2015) was originally developed based on semantic segmentation of biomedical images, but its advantages in feature detection of remote sensing images have been demonstrated in the study (Cui et al. Citation2019; Shamsolmoali et al. Citation2019). Through the integration and optimization of remote sensing image features, the deep learning network has realized the automatic extraction of cage and floating raft aquaculture information (Hu, Fan, and Wang Citation2018; Geng, Fan, and Wang Citation2017; Sui et al. Citation2020).
In 2021, the largest Enteromorpha disaster in history broke out in Qingdao, China. From the remote sensing, biological, and chemical perspectives, researchers have studied and analyzed the green tide. Based on the methods of machine learning and deep learning, the green tide in the Yellow Sea is automatically extracted from multi-source satellite data such as optics and SAR data, and the change information of its distribution range is analyzed by trajectory model (Jin et al. Citation2020; Liang et al. Citation2020; Xu et al. Citation2016; Yu et al. Citation2021). The temporal and spatial dynamics of laver cultivation and green tides in the South Yellow Sea are studied through long-time satellite observation and numerical simulation of the relationship between the time to recover aquaculture facilities and the number of green tides (Xing et al. Citation2019). Through the long-term trajectory of cyanobacteria bloom, the influence of the environment on the spatio-temporal dynamics of cyanobacteria bloom is studied (Yang et al. Citation2023).
Through observing the distribution of floating green algae in the Yellow Sea by satellite remote sensing (Hu, Hu, and He Citation2017; Xu et al. Citation2016), comparing the biomass between the floating green algae on the coast near Qingdao and the fixed green algae on the rafts and analyzing their respective compositions by experiments (Fan et al. Citation2015; Han et al. Citation2019; Li et al. Citation2014; Zhang et al. Citation2018), and the on-site continuous tracking observation studies (Fan et al. Citation2012; Fletcher Citation1996; Liu et al. Citation2015), it is found that there is a particular connection between the green tide that broke out in the Yellow Sea and the seaweed culture in the Jiangsu Shoal. The rafts are recovered from late April to early June, and a large number of green algae adhered to the aquaculture facilities are removed and finally entered the ocean. The floating Enteromorpha prolifera is first scattered near the coast of Jiangsu Shoal, forming floating green tide patches on the surface under the action of the summer monsoon and drifting to the northern part of the Yellow Sea under the impetus of ocean currents. Under suitable conditions, the biomass expanded rapidly, and finally, a large-scale green tide broke out in (Wang et al. Citation2018; Xing et al. Citation2018).
Because of the great influence of the Enteromorpha prolifera outbreak, scholars have paid significant attention to it since the large-scale green tide broke out in the Yellow Sea in 2008. In recent years, even though multidisciplinary investigations and experimental studies have been carried out around its origin, development process, and environmental characteristics, the research on its relationship with aquaculture primarily focuses on the biological field. Because of this, this paper will accurately extract marine aquaculture in northern Jiangsu shoal based on multi-source remote sensing data, and then analyze its area changes and distribution characteristics, and study the correlation between the marine aquaculture in the north of Jiangsu shoal and the green tide in the Yellow Sea from multiple angles.
In this paper, concentrating on the above-mentioned consideration, the main contributions are as follows,
Different aquaculture imaging characters of optical and SAR remote sensing imagery in the Jiangsu Shoal are analyzed with varying growth and sea conditions cycles, where month-to-month changes are extracted during the aquaculture cycle.
In 2017 and 2019, the entire dynamic process of the green tide outbreak was monitored through multi-source satellite remote sensing images, respectively.
The correlation between the green tide and marine aquaculture harvest in the Jiangsu Shoal is explored from the initial outbreak places and the principle of early green tide spatial distribution changes, based on the statistical analyses of many multi-source remote sensing images.
The rest of this paper is organized as follows. The methodology, including the study area, marine aquaculture imaging characters in different satellite and growth cycles, extraction, and spatiotemporal correlation analysis methods, is introduced in Section 2. The results of the change in marine aquaculture and green tide are presented in Section 3. The discussion of the spatiotemporal correction from the headstream and early change trend is presented in Section 4. Finally, the conclusions are given in Section 5.
2 Methodology
The overall idea of the research is shown in . Based on optical and SAR remote sensing images, the characteristics of different types of marine aquaculture and green tides are analyzed. A number of methods have been proposed by our research team (Fan et al. Citation2015, Citation2019; Geng, Fan, and Wang Citation2017; Hu, Fan, and Wang Citation2018; Wang, Fan, and Wang Citation2022; Wang et al. Citation2022) to extract offshore marine aquaculture, which can solve different types of marine aquaculture in multi-source remote sensing images. The MDOAU-net (Wang, Fan, and Wang Citation2022) is the newest approach for marine aquaculture extraction. Firstly, it extracts features from the input image through a multi-scale feature fusion block. Then divides the image and the boundary in the U-net network with the attention module, extended convolution block, and offset convolution block added and obtains the semantic features of the potential features and speckle noise in the image. Finally, the information about aquaculture is extracted by combining various features. Our previous research (Liang et al. Citation2020) showed macroalgae as promising targets in SAR images with irregular but clear boundaries. Therefore, adaptively, the Otsu algorithm with expansion and corrosion post-processing determines the optimal segmentation threshold and accurately extracts macroalgae bloom information. The corrosion expansion algorithm is used to optimize the extraction results. In the optical image, the water spectrum of the surrounding sea area is similar to the vegetation spectrum, so the enhanced vegetation index (EVI) is used to extract the size of macroalgae. The image is divided into several small windows for more accurate extraction, and the EVI threshold is set to pull large macroalgae one by one. The code of these works will be provided on http://github.com/jcfan for the sake of reproducibility. This paper mainly focuses on monitoring the monthly changes in Jiangsu Shoal aquaculture and the typical changes in the green tide distribution in the Yellow Sea in 2017 and 2019. Then, clarify the spatiotemporal correlation between them and diagnose the inherent marine aquaculture changes that drive the macroalgae blooms through big data analysis.
Figure 1. The whole framework of spatiotemporal correlation analysis between marine aquaculture and macroalgae blooms.

2.1 Research area
Jiangsu Shoal, as a typical muddy tidal flat in China (N,
E), located in the Yangtze River estuary to the north of the Sheyang River, covering an area of about 22,000 square kilometers, as shown in . The topography of the northern Jiangsu Shoal is complex, with many sand ridges and sand troughs alternately distributed in the middle. The water body is turbid for a long time, greatly disturbed by tides. Ocean current is mainly reciprocating flow. At high tide, algae float on the sea surface, the tidal flat is submerged under water, and the background of the algae culture area is seawater. At low tide, the raft frame is exposed to the water, and the experience of the seaweed culture area above the tidal flat changes from seawater to bare tidal flat. Its suitable geographical location, hydrology, meteorology, and other conditions make it an important area for marine aquaculture.
Figure 2. Jiangsu Shoal, the research area of marine aquaculture and macroalgae blooms spatiotemporal correlation.

In extracting marine aquaculture and green tide from multi-source remote sensing images, SAR images like GF-3 usually adopt a UFS sensor of 3 m resolution, an FSI sensor of 5 m resolution, and an FSII sensor of 10 m resolution. Optical images commonly use Sentinel-2 visible light bands 4, 3, and 2 with 10 m resolution and GF-1 WFV sensor with 16 m resolution.
2.2 Marine aquaculture imaging characters in optical and sar remote sensing images
By comparing the synchronized SAR and optical images, macroalgae blooms are represented obviously in the GF-2 optical true-color image, as shown in the Feature Analysis part of . In SAR images, the brightness of the green tide targets can be detected by the backscattered intensity. It is found that the marine aquaculture area will show different states. It is seen that after Freeman polarimetric decomposition, marine aquaculture in the Jiangsu Shoal has a significant difference. Various factors such as sea conditions, materials of aquaculture facilities, as well as the spatial distribution of cultured crops will affect the intensity of backscattering. Compared with the background seawater, the laver cultivation areas are rougher, so the even-scattering signals are stronger. Therefore, in the SAR satellite remote sensing image, the laver cultivation area is brighter than the sea surface background, and the texture characteristics are more obvious, which is shown as a regular strip. The laver raft culture area is pink and white, a mixture of dihedral scattering and volume scattering, and rough sea surface waves form a very typical surface scattering and appear blue.
The idea of using optical images to identify laver aquaculture is that its spectral features are sensitive to Chl-a pigments in the algae, and the “red edge” phenomenon is obvious, which is easy to distinguish from the background seawater. However, in optical images of Jiangsu Shoal, aquaculture targets are very similar to the seawater background, indicated as long dark strips. Considering the geomorphological characteristics of the Jiangsu Shoal, it can be found that the main reason is that the total suspended matter in the seawater is very high. The spectral reflection curve of the turbid water body is overall higher than that of the clear water. Thus, laver aquaculture targets in the turbid seawater are also affected, and the differences in spectral reflectance between them are smaller.
2.3 Marine aquaculture imaging characters in the different growth cycle and sea conditions
During the growth cycle of marine laver aquaculture with the facility recycle, marine aquaculture targets have distinguished differences, as shown in . The first row is the optical images under the corresponding situation, and the second row is the related quasi-synchronous SAR images. From the end of April to the beginning of June each year, the cultivation equipment (net curtains, bamboo poles, cables), as shown in , needs to be recycled after the harvest of mature laver. Generally, the net curtains are collected first, and then the bamboo poles and cables are ordered subsequently. In , the laver and the raft have not been recovered. Aquaculture areas can be seen in the optical and SAR images at low tide, which means most part of the rafts can be exposed to the sea. also show that laver and rafts have not been recovered in the high tide. The difference is that the cultivation can be seen in the optical image, but the cultivation cannot be seen in the SAR image. The reason may be that some parts of the cultivation area are submerged by seawater, which cannot be detected in the SAR image observably. But there are still some sporadic aquaculture areas to be found in .
Figure 3. Image characters of the aquaculture area are chosen from the Jiangsu Shoal research area under optical and SAR images during the different periods and sea conditions. In the first row are optical images, and in the second row are synchronous SAR images. (a) and (e) indicate the laver and raft in the low tide, (b) and (f) are the laver and raft in the high tide, (c) and (g) describe only existing rafts in the high tide, (d) and (h) show the laver and raft recovered.

In , it is shown that the laver has been harvested, but the rafts have not been recovered in the high tide. The aquaculture areas are visible in the optical image but not prominent in the SAR image. This situation speculated that rafts had changed the topography of the shoal, and the sediment became strips like the shape of aquaculture. So it causes the water body to change in surrounding spectral reflectance, which is more evident in the optical image. On the contrary, this topography change is too slight to affect seawater surface roughness, so it can be detected and identified by SAR images. show that the laver and the raft have been recovered, and the culture area is not visible in the optical and SAR images. The analysis of the differences between the optical and SAR images in the display of the aquaculture area is of great significance for the precision extraction of marine aquaculture and the recovery of the laver cultivation equipment under different sea conditions and growth cycles.
2.4 Spatiotemporal correlation
The correlation coefficient is employed to quantify a specific measure of the linear relationship between the two variables in the correlation analysis to analyze the relationship between marine aquaculture and macroalgae blooms. The coefficient of correlation is defined as
where represent the area of marine aquaculture and macroalgae, respectively;
are the mean of these variables. It compares the distance between each data point and the average of variables and describes the degree of correlation between variables. The coefficient of correlation
takes a value between −1 and 1. As
approaches 0, it means that the linear relationship between two variables is weaker. A positive value of
means a positive correlation, and the values of two variables will increase together. A negative value of
indicates a negative correlation, as the value of one variable decreases, the value of another variable will gradually increase. The degree of correlation can be judged by the following formula,
The significance value can also evaluate the correlation between marine aquaculture and green tide.
where is the statistic of the test,
is the test statistics calculated from sample data, and
is the probability of the size between the test statistic and the sample statistic. Theoretically, if
is less than 0.05, there is a correlation relationship. Therefore, the level of spatiotemporal correlation between marine aquaculture and macroalgae Blooms can be analyzed based on the statistics theory.
3 Results
3.1 Changing trend of green tide disaster outbreak
Due to the influence of many factors, there are great differences in the area size and shape characteristics of the green tide patches in different periods of green tide disaster outbreak, especially in the early stages of the green tide outbreak. The green tide is generally distributed in fine filaments. The initial spatiotemporal features of the green tide in 2017 and 2019 are shown in , respectively.
Its outbreak cycle characteristics can be summarized as four stages of initial birth-development-outbreak-death. The first three stages are the main focus. The green tide areas in different periods in 2017 and 2019 are tabulated in . In early May, the green tide began to form and sporadically floated in the muddy water tongue along the North Jiangsu coast. In the middle and late May, under the action of the sea surface wind field, the distribution area of green tide further expanded and gradually drifted northward. The green tide gathered on the sea surface to form strips, and it is estimated that some strips stretched for 2.5 km. It is the main period of rapid growth of green tide. In early June, the green tide grew explosively and gathered in a large area. The biomass also increased rapidly, and the distribution area continued to expand and drift northward. In the middle and late June, the green tide gradually approached the coastal area in the south of the Shandong Peninsula and finally landed in Weihai, Qingdao, Rizhao, and other places and accumulated on the beach.
Table 1. Green tide areas of different periods in the Jiangsu Shoal (km2).
3.2 Marine aquaculture area change in the whole period
The marine aquaculture areas of Subei Shoal were extracted using optical and SAR remote sensing images. The main spatiotemporal distribution change is shown in during the entire cultivation period. The specific marine aquaculture areas of different months are tabulated in . In 2019, the largest area of marine aquaculture was 221.3 km, distributed along the beach areas near Dafeng, Dongtai, and Rudong in a flaky shape from north to south. Besides, there were also a fraction of laver rafts on the coastland of Rudong County, but the scale is not large, where the southernmost point can reach the coast of the south-central south.
Figure 5. Monthly changes of spatiotemporal distribution in laver aquaculture area in the Jiangsu Shoal in 2019.

Table 2. Marine aquaculture areas of different months in the Jiangsu Shoal (km2).
In the Jiangsu Shoal, the laver cultivation activities usually begin in September and are harvested next April after 6–7 months of cultivation. It revealed that the distribution and area of laver cultivation areas in January, February, and March were relatively stable, maintaining around 220 km2, according to . The area of cultivation distribution began to decrease in April and dropped to about 162.3 km2. It was because the period from December to next April was the growing and harvesting stage of laver cultivation activities in the Jiangsu Shoal. The distribution and area of laver cultivation areas remain unchanged during the growth period of laver. From the end of April to the beginning of June each year, after the seaweed was harvested, the area of the seaweed breeding area could decrease with the recovery of breeding equipment due to the recycling of breeding equipment. The breeding area significantly reduced in May and June. The laver aquaculture was recovered with only about 2.5 km2 distribution in June. July-August was the facility preparation stage. During this period, the net curtains were cleaned and maintained, rafts were repaired, and pilings and cables were laid on the tidal flats, so there was almost no laver cultivation distribution. However, shows that there was sporadic laver cultivation in the northern area far from the shore in July, and it was guessed that the laver cultivation was missed during the shoal farming operation. September-November was the emergence stage of laver cultivation activities. Laver cultivation area gradually increased, mainly north of Rudong City. From November to December, the size and distribution were shown with a significant expansion trend, and cultivation areas increased to 138.9 km2. As the local laver cultivation cycle was relatively stable, the changes in the cultivation area in 2017 and 2019 were the same.
4 Discussion
4.1 Headstream discussion on marine aquaculture and macroalgae blooms occurrence
Since the serious green tide broke out in the Yellow Sea in 2008, scholars have paid significant attention to it. Exploring the correlation between the laver breeding raft and the source of green tide has tremendous significance and scientific value for mitigating green tide disasters. The correlation between the initial position of the green tide outbreak and the marine aquaculture area is obtained through extensive monitoring data analysis, as shown in . The red part in the figure is the initial stage of the cultivation facility recycling in 2017 and 2019. In other words, these are the areas of laver cultivation and harvesting from late April to early May. The green tides in 2017 and 2019 were first discovered and marked as the green part in .
Figure 6. Relationship between initial green tide occurrence and marine aquaculture recycling in 2017 (a) and 2019 (b).

After the aquaculture activities, aquaculture facilities will be recycled for further use in the next aquaculture cycle. The recovery of aquaculture facilities includes three processes. The bamboo poles and net curtains are directly recovered, and the stem rope is finally recovered. The stem rope is the primary attachment base of the green algae. The fishermen usually manually clean the settled green algae and then recover the stem rope. Part of the Enteromorpha in the green algae that was manually cleaned and abandoned in the shallows due to its strong floating ability was floated by the tide during the subsequent high tide and became a randomly distributed floating Enteromorpha. It reveals that the floating green algae are primarily strips and broken patches, with scattered regional distribution in the early stage of the growth in early May. Expanding from the area of the laver breeding raft to the outside in a fan-shaped along the tides. Floating green algae can be traced back to the edge of the raft culture region in the shoal center, and then extended along the tidal channel. During the ebb tide, the floating Enteromorpha is gradually gathered into strips of different sizes under the action of strong tidal currents and transported to deep water areas by the tidal currents. The unique tidal current system and radiant sandbank landform features of the Jiangsu Shoal are conducive to the belt-shaped accumulation of floating green algae.
At this time, it coincides with the Asian summer monsoon season. Therefore, under the action of the summer monsoon and surface currents, the floating Enteromorpha gradually drifts northward. Due to suitable environmental conditions, the biomass increases hundreds of times during the long-distance floating process. Finally, a large-scale green tide is formed in coastal waters. It can be inferred that the Jiangsu Shoal is the source of the outbreak of the green tide in the Yellow Sea. Enteromorpha, which developed in the coastal area of the Jiangsu Shoal, spread to the sea under the drive of tidal channel currents and eventually piled up in large-scale coastal areas of Shandong.
4.2 Marine aquaculture change and early green tide distribution
In the laver aquaculture area of Jiangsu Shoal, the Yellow Sea green tide broke out first. During the recovery related to the cultivation facilities in the cultivation area, the green algae adhered to the raft is artificially scraped into the shallows and entered under the action of the tide. It is the primary way for the green algae to enter the ocean in seawater. The floating green algae grow and proliferate under suitable conditions and drift northward, and finally, in the Yellow Sea, a large-scale green tide disaster erupts. The first green tides usually occur in early May, and they are only distributed sporadically. During the recovery of the marine aquaculture facilities in the north of Jiangsu Shoal in 2017 and 2019, the spatiotemporal distribution of the breeding areas and the green tides are shown in .
Figure 7. Spatiotemporal dynamical distribution of the early green tides and marine aquaculture in the Jiangsu Shoal. (a)-(c) 2017, (d)-(f) 2019.

From the time and space distribution of the marine aquaculture area and green tide, it can be found that with the decrease of the breeding areas, the distribution of green tides increases. The remaining marine aquaculture areas are minor, which means more breeding equipment is recycled, and more green tides are generated. On the contrary, the more remaining in the breeding areas, the less the breeding equipment is recycled, and the fewer green tides will be produced. Therefore, the later the aquaculture equipment is recycled, the smaller the amount of early green tides. Green tides mainly appear in the northern laver cultivation area in the Jiangsu Shoal. The north area is located on the beach about 300–600 km from the coastline. The transportation cost of recycling the breeding facilities in these areas is high. Workers are more inclined to use the facilities to clean up the green algae to reduce weight instead of collecting them. The scraped-off green algae are directly left on the tidal flat, thereby increasing the chance of releasing the green algae into the seawater, providing a large amount of initial biomass for the green tides outbreak.
The changes in green tides and marine aquaculture areas in 2017 and 2019 are shown in . The scope of green tides and marine aquaculture show the opposite change trend. With the decrease in the breeding area, the area covered by the green tides increases. Compared to the displayed regions covered by the early green tides, the area in 2019 is more than in 2017. The area covered by the green tides in early June 2019 reached 158,226.8 km2, nearly twice in the same period in 2017, while the aquaculture area was 2.49 km2, about half that of the same period in 2017. shows that after the aquaculture equipment is recycled, the aquaculture area decreases, and the green tide area increases. When the recovery time of laver cultivation equipment was earlier, the remaining laver cultivation area was less, and the cultivation area was recovered more, leading to more green tides. When the cultivation equipment was recycled later, the aquaculture area’s remaining size was more prominent, the reclaimed aquaculture area was less, and the number of green tides produced was less. On the other hand, according to EquationEquation 1(1)
(1) , the correction coefficients were
in 2017 and
in 2019, respectively. So the area of marine aquaculture and macroalgae blooms has significant or high correction according to EquationEquation 2
(2)
(2) . What’s more,
in 2017 and
in 2019. Based on the above studies, it is shown that there is a strong correction relationship between marine laver aquaculture activities and macroalgae blooms.
4.3 Other factors of the green tide outbreak
The green tide outbreak is affected not only by aquaculture activities but also by other factors such as temperature and nutrient salt, etc.. For example, the germination of Enteromorpha is related to temperature, and the highest germination rate is when the water temperature reaches 15 degrees Celsius. Jiangsu shoal area is rich in nutrients, and its high concentration of nutrients such as nitrogen and phosphorus provides a material basis for forming the green tide.
5 Conclusion
Marine aquaculture and green tide have different characteristics on satellite remote sensing images. Through long-time monitoring and analysis of the characteristics of marine aquaculture activities in the Jiangsu Shoal and the green tide outbreak, it has been found that there is a specific correlation between these two. From the two aspects of the place of origin and the early outbreak trend, the relationship between the two can be further determined based on 2017 and 2019 remote sensing data. The green tide was born in the laver breeding raft area of the radiation sand ridge group in Jiangsu from the end of April to the beginning of May. From the time and space distribution of the marine aquaculture area and green tide, it can be found that with the decrease of the breeding areas, the distribution of green tides increases. The earlier the laver aquaculture equipment is recycled, the earlier green tides are produced. Moreover, the later the cultivation equipment is recycled, the fewer early green tides are produced. The preliminary stage characteristics of green tides in different periods show the apparent opposite trend of the marine aquaculture area change. To reduce marine green tide outbursts from the root cause, adjusting the recycling process of aquaculture equipment in the Jiangsu Shoal aquaculture area might be an effective way to a certain extent. This study only analyzed remote sensing big data from the temporal and spatial correlation of mariculture and green tide outbreaks but did not consider other environmental factors, such as temperature and nutrients. Therefore, correction analysis from more dimensions between marine aquaculture and macroalgae blooms and theoretical verification in other sea areas can be conducted in the future.
Disclosure statement
No potential conflict of interest was reported by the author(s).
Data availability statement
The data that support the findings of this study are available from the corresponding author upon reasonable request.
Additional information
Funding
Notes on contributors
Jianchao Fan
Jianchao Fan received the B.S. and Ph.D. degrees from the School of Control Science and Engineering, Dalian University of Technology, Dalian, China, in 2007 and 2012, respectively. He was a Postdoctoral Fellow jointly cultivated by The Chinese University of Hong Kong and Dalian University of Technology during 2013-2016, and also a Visiting Scholar with the Department of Computer Science and Engineering, Washington University in St. Louis, St. Louis, MO, USA, during 2018-2019. He has been a Professor at the School of Control Science and Engineering, Dalian University of Technology, Dalian, China. He has authored/co-authored three books and over 90 scientific papers in international journals and conference proceedings. His current research interests include marine SAR image processing, polarimetric SAR, deep learning neural networks, and heuristic swarm intelligent optimization.
Yujuan Ma
Yujuan Ma received the B.S. and Ph.D. degrees from the School of Marine Environment, Ocean University of China, Qingdao, China, in 2007 and 2012, respectively. She has been a research assistant with the Department of Ocean Remote Sensing, National Marine Environmental Monitoring Center, Dalian, China, since 2012. She has authored/coauthored two books, and over 20 scientific papers in Chinese journals, international journals, and conference proceedings. Her current research interests include monitoring marine ecological disasters and marine atmospheric deposition.
Tingting Liang
Tingting Liang received the B.S. and M.S. degrees from the Institute of Geography Science, Liaoning Normal University, Dalian, China, in 2018 and 2021, respectively. Her research interests include satellite remote sensing, analysis of marine aquaculture, and green tide disasters.
Guangyan Cui
Guangyan Cui received the B.S. degree from the School of Aerospace Engineering, Zhengzhou University of Aeronautics, Zhengzhou, China, in 2021. He is currently pursuing an M.S. degree with the School of Information at Shanghai Ocean University, Shanghai, China. His research interests include transfer learning and ocean remote sensing image processing.
References
- Bai, T., L. Wang, D. Yin, K. Sun, Y. Chen, and W. Li. 2022. “Deep learning for change detection in remote sensing: a review.” Geo-Spatial Information Science 26 (3): 262–288. https://doi.org/10.1080/10095020.2022.2085633.
- Cao, L., W. Wang, Y. Yang, C. Yang, Z. Yuan, S. Xiong, and J. Diana. 2007. “Environmental impact of aquaculture and countermeasures to aquaculture pollution in China.” Environmental Science and Pollution Research International 14 (7): 452–462. https://doi.org/10.1065/espr2007.05.426.
- Cui, B., D. Fei, G. Shao, Y. Lu, and J. Chu. 2019. “Extracting Raft Aquaculture Areas from Remote Sensing Images via an Improved U-Net with a PSE Structure.” Remote Sensing 11 (17): 2053. https://doi.org/10.3390/rs11172053.
- Fan, J., J. Chu, J. Geng, and F. Zhang. 2015. “Floating Raft Aquaculture Information Automatic Extraction Based on High Resolution SAR Images.” In 2015 IEEE International Geoscience and Remote Sensing Symposium (IGARSS), 92–98.
- Fan, J., J. Zhao, W. An, and Y. Hu. 2019. “Marine Floating Raft Aquaculture Detection of GF-3 PolSar Images Based on Collective Multikernel Fuzzy Clustering.” IEEE Journal Selected Topic Applied Earth Observation Remote Sensing 12 (8): 2741–2754. https://doi.org/10.1109/JSTARS.2019.2910786.
- Fan, S., M. Fu, Y. Li, Z. Wang, S. Fang, H. Wang, P. Sun, and P. Qu. 2012. “Origin and Development of Huanghai(Yellow) Sea Green-Tides in 2009 and 2010.” Haiyang Xuebao 34 (6): 187–194.
- Fan, S., M. Fu, Z. Wang, X. Zhang, W. Song, Y. Li, G. Liu, X. Shi, X. Wang, and M. Zhu. 2015. “Temporal Variation of Green Macroalgal Assemblage on Porphyra Aquaculture Rafts in the Subei Shoal, China.” Estuarine, Coastal and Shelf Science 163 (9): 23–28. https://doi.org/10.1016/j.ecss.2015.03.016.
- FAO. 2018. “The State of World Fisheries and Aquaculture (SOFIA).” FAO Fisheries and Aquaculture Department.
- Fletcher, R. L. 1996. The Occurrence of ”Green Tides” | A Review. Berlin Heidelberg: Springer.
- Geng, J., J. Fan, and H. Wang. 2017. “Weighted Fusion-Based Representation Classifiers for Marine Floating Raft Detection of SAR Images.” IEEE Geoscience Remote Sensing Letter 14 (3): 444–448. https://doi.org/10.1109/LGRS.2017.2648641.
- Han, X., T. Zhao, H. Miao, B. Sun, W. Zhao, and T. Yan. 2019. “Growth Characteristics of Algae from Attached to Floated Growth Process in the Subei Shoal Detached from Aquaculture Rafts into Seawater Resulted in Green Tide Occurrence in the Yellow Sea.” Oceanologia Et Limnologia Sinica 50 (2): 309–315.
- Hou, T., W. Sun, C. Chen, G. Yang, X. Meng, and J. Peng. 2022. “Marine Floating Raft Aquaculture Extraction of Hyperspectral Remote Sensing Images Based Decision Tree Algorithm.” International Journal of Applied Earth Observation and Geoinformation 111:102846. https://doi.org/10.1016/j.jag.2022.102846.
- Hu, L., C. Hu, and M. He. 2017. “Remote Estimation of Biomass of Ulva Prolifera Macroalgae in the Yellow Sea.” Remote Sensing of Environment 192:217–227. https://doi.org/10.1016/j.rse.2017.01.037.
- Hu, Y., J. Fan, and J. Wang. 2018. “Classification of PolSar Images Based on Adaptive Nonlocal Stacked Sparse Autoencoder.” IEEE Geoscience and Remote Sensing Letters 15 (7): 1050–1054. https://doi.org/10.1109/LGRS.2018.2829182.
- Jin, X., J. Wan, W. Hu, Y. Song, and B. Lu. 2020. “Retrieval of Green Tide Concentration and Interannual Variation Analysis in Yellow Sea Based on Multi-Source Remote Sensing Monitoring.” In Global Oceans 2020: Singapore – U.S. Gulf Coast, 1–5. https://doi.org/10.1109/IEEECONF38699.2020.9388992.
- Kuang, G., J. Wang, J. Fan, and J. Wang. 2022. “Marine Aquaculture Information Extraction from Optical Remote Sensing Images via MDOAU2-Net.” In 2022 12th International Conference on Information Science and Technology (ICIST), 167–170. https://doi.org/10.1109/ICIST55546.2022.9926847.
- Lee, S., S. Hong, S. Kim, Y. Yamaguchi, and J. Won. 2006. “Polarimetric Features of Oyster Farm Observed by AIRSAR and JERS-1.” IEEE Transactions on Geoscience & Remote Sensing 44 (10): 2728–2735. https://doi.org/10.1109/TGRS.2006.879107.
- Li, Y., W. Song, J. Xiao, Z. Wang, M. Fu, M. Zhu, R. Li, X. Zhang, and X. Wang. 2014. “Tempo-Spatial Distribution and Species Diversity of Green Algae Micro-Propagules in the Yellow Sea During the Large- Scale Green Tide Development.” Harmful Algae 39:40–47. https://doi.org/10.1016/j.hal.2014.05.013.
- Liang, T., L. Ke, J. Fan, and J. Zhao. 2020. “Green Tide Information Extraction Based on Multi-Source Remote Sensing Data.” In 2020 12th International Conference on Advanced Computational Intelligence (ICACI), 460–465.
- Liu, Q., R. Yu, T. Yan, Q. Zhang, and M. Zhou. 2015. “Laboratory Study on the Life History of Bloom- Forming Ulva Prolifera in the Yellow Sea.” Estuarine, Coastal and Shelf Science 163 (9): 82–88. https://doi.org/10.1016/j.ecss.2014.08.011.
- Liu, Y., Z. Wang, X. Yang, Y. Zhang, F. Yang, B. Liu, and P. Cai. 2020. “Satellite-Based Monitoring and Statistics for Raft and Cage Aquaculture in China Offshore Waters.” International Journal of Applied Earth Observation and Geoinformation 91:102–118. https://doi.org/10.1016/j.jag.2020.102118.
- Liu, Y., X. Yang, Z. Wang, C. Lu, and Z. Li. 2019. “Aquaculture Area Extraction and Vulnerability Assessment in Sanduao Based on Richer Convolutional Features Network Model.” Journal of Oceanology and Limnology 37 (6): 153–166. https://doi.org/10.1007/s00343-019-8265-z.
- Oktay, O., J. Schlemper, L. L. Folgoc, M. Lee, M. Heinrich, K. Misawa, K. Mori, S. McDonagh, N. Y. Hammerla, and B. Kainz. 2018. “Attention U-Net: Learning Where to Look for the Pancreas.” In Proceedings of Conference on Medical Imaging with Deep Learning, 1–10.
- Ottinger, M., K. Clauss, and C. Kuenzer. 2016. “Aquaculture: Relevance, Distribution, Impacts and Spatial Assessments − A Review.” Ocean and Coastal Management 119:244–266. https://doi.org/10.1016/j.ocecoaman.2015.10.015.
- Ronneberger, O., P. Fischer, and T. Brox. 2015. “U-Net: Convolutional Networks for Biomedical Image Segmentation.” In The IEEE Conference on Computer Vision and Pattern Recognition (CVPR), 234–241.
- Shamsolmoali, P., M. Zareapoor, R. Wang, H. Zhou, and J. Yang. 2019. “A Novel Deep Structure U-Net for Sea-Land Segmentation in Remote Sensing Images.” IEEE Journal of Selected Topics in Applied Earth Observations and Remote Sensing 12 (9): 3219–3232. https://doi.org/10.1109/JSTARS.2019.2925841.
- Shi, T., Q. Xu, Z. Zou, and Z. Shi. 2018. “Automatic Raft Labeling for Remote Sensing Images via Dual- Scale Homogeneous Convolutional Neural Network.” Remote Sensing 10 (7): 1130. https://doi.org/10.3390/rs10071130.
- Sugimoto, M., K. Ouchi, and Y. Nakamura. 2012. “Comparison of Contrast Improvement of Extracted Laver Cultivation Area Using Parameters Derived from Polarimetric SAR Data.” In Remote Sensing of the Marine Environment II, 134–143. Vol. 8525. SPIE.
- Sui, B., T. Jiang, Z. Zhang, X. Pan, and C. Liu. 2020. “A Modeling Method for Automatic Extraction of Offshore Aquaculture Zones Based on Semantic Segmentation.” ISPRS International Journal of Geo- Information 9 (3): 145. https://doi.org/10.3390/ijgi9030145.
- Sun, W., K. Liu, G. Ren, W. Liu, G. Yang, X. Meng, and J. Peng. 2021. “A Simple and Effective Spectral- Spatial Method for Mapping Large-Scale Coastal Wetlands Using China ZY1-02D Satellite Hyperspectral Images.” International Journal of Applied Earth Observation and Geoinformation 104:102572. https://doi.org/10.1016/j.jag.2021.102572.
- Wang, J., J. Fan, and J. Wang. 2022. “MDOAU-Net: A Lightweight and Robust Deep Learning Model for SAR Image Segmentation in Aquaculture Raft Monitoring.” IEEE Geoscience & Remote Sensing Letters 19 (4504505): 1–5. https://doi.org/10.1109/LGRS.2022.3147355.
- Wang, M., Q. Cui, J. Wang, D. Ming, and G. Lv. 2017. “Raft Cultivation Area Extraction from High Resolution Remote Sensing Imagery by Fusing Multi-Scale Region-Line Primitive Association Features.” ISPRS Journal of Photogrammetry and Remote Sensing 123:104–113. https://doi.org/10.1016/j.isprsjprs.2016.10.008.
- Wang, X., Q. Deng, J. Wang, and J. Fan. 2022. “Deep Semantic Segmentation MRF Model for Information Extraction of Marine Floating Raft Aquaculture.” Journal of Shandong University(engineering Science) 52 (2): 89–98.
- Wang, Z., M. Fu, J. Xiao, X. Zhang, and W. Song. 2018. “Process on the Study of the Yellow Sea Green Tides Caused by Ulva Prolifera.” Haiyang Xuebao 40 (2): 1–13.
- Xing, Q., D. An, X. Zheng, Z. Wei, and J. Chen. 2019. “Monitoring Seaweed Aquaculture in the Yellow Sea with Multiple Sensors for Managing the Disaster of Macroalgal Blooms.” Remote Sensing of Environment 231:1–12. https://doi.org/10.1016/j.rse.2019.111279.
- Xing, Q., L. Wu, L. Tian, T. Cui, and M. Wu. 2018. “Remote Sensing of Early-Stage Green Tide in the Yellow Sea for Floating-Macroalgae Collecting Campaign.” Marine Pollution Bulletin 133:150–156. https://doi.org/10.1016/j.marpolbul.2018.05.035.
- Xu, Q., H. Zhang, Y. Cheng, S. Zhang, and W. Zhang. 2016. “Monitoring and Tracking the Green Tide in the Yellow Sea with Satellite Imagery and Trajectory Model.” IEEE Journal of Selected Topics in Applied Earth Observations and Remote Sensing 9 (11): 5172–5181. https://doi.org/10.1109/JSTARS.2016.2580000.
- Xu, Y., Z. Zhang, and X. Wang. 2018. “Remote Sensing Monitoring Analysis of Coastal Farming Land in Shandong Province in Recent 30 Years.” Journal of Geo-Information Science 16 (3): 482–489.
- Yang, S., J. Lu, X. Chen, X. Hou, Z. Wei, and J. Wu. 2023. “Unraveling Environmental Influences on the Spatial and Temporal Dynamics of Cyanobacterial Blooms in Lake Erhai During Its Early Stage of Eutrophication.” Geo-Spatial Information Science 1–19. https://doi.org/10.1080/10095020.2023.2217860.
- Yu, H., C. Wang, J. Li, and Y. Sui. 2021. “Automatic Extraction of Green Tide from GF-3 SAR Images Based on Feature Selection and Deep Learning.” IEEE Journal of Selected Topics in Applied Earth Observations and Remote Sensing 14:10598–10613. https://doi.org/10.1109/JSTARS.2021.3118374.
- Zhang, Q., F. Kong, T. Yan, R. Yu, X. Hu, and M. Zhou. 2018. “Green Algae Detached from Aquaculture Rafts into Seawater Resulted in Green Tide Occurrence in the Yellow Sea.” Oceanologia Et Limnologia Sinica 49 (5): 1015–1019.
- Zhao, Q., A. Pepe, V. Zamparelli, P. Mastro, F. Falabella, S. Abdikan, C. Bayik, et al. 2023. “Innovative Remote Sensing Methodologies and Applications in Coastal and Marine Environments.” Geo-Spatial Information Science 1–18. https://doi.org/10.1080/10095020.2023.2244006.