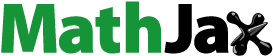
Abstract
Despite availability of in vivo knee loads and kinematics data, conventional load- and displacement-controlled configurations still can’t accurately predict tibiofemoral loads from kinematics or vice versa. We propose a combined load- and displacement-control method for joint-level simulations of the knee to reliably reproduce in vivo contact mechanics. Prediction errors of the new approach were compared to those of conventional purely load- or displacement-controlled models using in vivo implant loads and kinematics for multiple subjects and activities (CAMS-Knee dataset). Our method reproduced both loads and kinematics more closely than conventional models and thus demonstrates clear advantages for investigating tibiofemoral contact or wear.
Introduction
Knowledge of in vivo tibiofemoral loads and kinematics is essential not only to understand knee biomechanics, but also for numerical simulations or experimental setups to reproduce clinical conditions. Accurate joint-level data is required to investigate soft-tissue loading (Hosseini Nasab et al. Citation2021), predict implant wear (Fregly et al. Citation2005b), and validate computational models (Imani Nejad et al. Citation2020).
Many studies have measured tibiofemoral kinematics in vivo using static (Pfitzner et al. Citation2018) or mobile (Schütz et al. Citation2019; Gray et al. Citation2019; Postolka et al. Citation2020) fluoroscopy. Root-mean-square-errors (RMSE) of relative tibiofemoral kinematics measured in vitro using single-plane fluoroscopy have been reported to be in the range of 0.2–1.3 mm for in-plane translations, 1.9–4.7 mm for out-of-plane translations, and 0.3–2.2° for all rotations (Banks and Hodge Citation1996; Hoff et al. Citation1998; Mahfouz et al. Citation2003; Fregly et al. Citation2005a; Acker et al. Citation2011; Prins et al. Citation2011; Taylor et al. Citation2017).
Nevertheless, these ostensibly small errors still make it almost impossible to use fluoroscopy kinematics for predictions of knee joint contact mechanics. For one, the contact loads are highly sensitive to errors in superior-inferior and adduction-abduction kinematics, which can cause interface overclosure or loss of contact in models of the implanted knee (Fregly et al. Citation2008; Lin et al. Citation2010). To enable model convergence, these degrees of freedom (DoFs) are commonly driven by a compressive load and sometimes an adduction-abduction moment instead. However, errors in the other DoFs are also critical: Upon introduction of kinematic errors of ±1° and ±1 mm, relative contact force errors exceeding 100% have been reported (Fregly et al. Citation2008; Fitzpatrick and Rullkoetter Citation2014). Moreover, even less than 1 mm of tibiofemoral positioning error can introduce more than 3 mm error in the implant contact point location (Catani et al. Citation2010) and significantly alter the center of pressure (CoP) location (Navacchia et al. Citation2016).
As an alternative approach, kinematics can be predicted from measured loads. The necessary direct measurements of tibiofemoral contact loads are difficult, but have been performed using a small number of telemetric load-measuring implants (Kirking et al. Citation2006; Heinlein et al. Citation2007). Using such in vivo loads, D’Lima et al. (Citation2011) were able to reproduce in vivo kinematics to within average absolute errors of 1.2 mm and 1.6°. These values, however, significantly exceeded the reported fluoroscopy measurement errors (Varadarajan et al. Citation2008) and, as outlined above, may not yield accurate contact locations.
Despite the increased availability of high-quality in vivo data, the reproduction of measured tibiofemoral loads from kinematics or vice versa remains challenging. Consequently, there is an unmet need for models that can accurately reproduce both in vivo tibiofemoral loads and kinematics. This could be achieved by developing modelling techniques that enforce both a load and a motion on the same DoF.
Thus, to reconcile and reproduce both known loads and kinematics within a single computational model, we here present a novel combined load and displacement control approach with springs (LDCS). In the DoFs this penalty-like method is applied to, the springs keep the error between computed output and measured input kinematics below a defined threshold such as the input kinematics’ measurement error while also reducing contact load errors. The LDCS method is demonstrated using finite element (FE) models of the CAMS-Knee datasets (Taylor et al. Citation2017), which contain both in vivo implant loads and kinematics of six subjects for level and downhill walking, stair descent, squat, and sit-to-stand-to-sit. Here, our method has the additional advantage of not disregarding either measured load or kinematic data for each DoF. For validation, we compare prediction errors of conventional load- and displacement-controlled simulations to our LDCS method. The proposed LDCS method has several practical applications with boundary conditions generated from published in vivo datasets, laboratory knee simulators, and computational models such as musculoskeletal simulations.
Methods
In this study, we propose the LDCS method to reproduce both measured in vivo loads and kinematics in a joint-level simulation of the knee. We investigated load control (LC), displacement control (DC), and LDCS setups in FE models of the resurfaced tibiofemoral joint. The resulting contact loads and kinematics were quantitatively validated against in vivo data from the CAMS-Knee datasets.
FE models of the CAMS-Knee prosthesis’ (Innex FIXUC, Zimmer Biomet, Switzerland) tibial inlay and femoral component were constructed in Abaqus/Standard 6.21 (Dassault Systemes, USA). A proprietary elastic-plastic material model supplied by the manufacturer was assigned to the tibial inlay. It was meshed using linear tetrahedral elements with an average element size of 0.9 mm (Godest et al. Citation2002), resulting in 43308 elements. The femoral component was considered to be rigid (Carr and Goswami Citation2009) with an element size of 0.5 mm, resulting in 20754 shell elements. Penalty contact was defined with a coefficient of friction of 0.04 (Godest et al. Citation2002).
Reference points (RPs) were created on the coordinate system origins of the femoral and tibial components as defined in the CAMS-Knee dataset (Dreyer et al. Citation2022) and constrained to the femoral component and the inferior planar surface of the tibial inlay, respectively (). An additional kinematics tracking RP was created coincident with the tibial inlay RP for the LDCS scenario only ().
Figure 1. Exploded view of the components, reference points (RPs), and boundary conditions applied to the LDCS model.

Through these RPs, relative tibiofemoral loads and kinematics from the CAMS-Knee datasets (Taylor et al. Citation2017) were applied as boundary conditions to the FE model in load control (LC), displacement control (DC), and LDCS configurations (): In practice, flexion angles are easily measured with motion capture setups and always applied as a motion in laboratory knee simulators (International Organization for Standardization Citation2009, Citation2014; ASTM International Citation2017). Thus, flexion-extension was prescribed to the femoral RP while its other DoFs remained fixed for all three configurations. Consequently, inlay flexion-extension was also fixed. Measured axial forces and adduction-abduction moments were applied to the tibial inlay RP for all three configurations. These DoFs would be extremely sensitive to even small errors in the measured tibiofemoral kinematics (Fregly et al. Citation2008; Lin et al. Citation2010), prohibiting their application in DC mode. The medio-lateral (ML) direction was left unconstrained because the fluoroscopy kinematics errors of up to 3 mm in out-of-plane direction would be too large to apply measured ML kinematics and ML loads are not commonly applied in test standards (International Organization for Standardization Citation2009, Citation2014) and modelling (Fregly et al. Citation2005b; Reinders et al. Citation2015; Navacchia et al. Citation2016; Shu et al. Citation2021).
Table 1. Summary of applied loads and boundary conditions for the LC, DC, and LDCS boundary condition scenarios (BCS).
The LC, DC, and LDCS models vary only in AP and IE directions, as these DOFs primarily govern CoP location and both loads or kinematics are commonly used to drive them. For the LC scenario, measured AP forces and IE moments were applied to the inlay RP, whereas the measured AP displacement and IE rotation were applied in the DC scenario. For the LDCS scenario (), the same AP forces and IE moments were applied to the inlay RP as in the LC scenario. Additionally, AP displacement and IE rotation were applied to the kinematics-tracking RP, representing the fluoroscopy-based inlay position and orientation. The translational (AP) and rotational (IE) deviation of the inlay RP from the kinematics-tracking RP thus simply represented the inlay’s deviation from the fluoroscopy-based kinematics. Finally, the two reference points were connected by nonlinear translational (AP) and rotational (IE) springs (). Their purpose was to allow the kinematics to deviate only within the bounds of the fluoroscopy measurement error of 1 mm and 1°. For inlay movement within these bounds, the nonlinear springs produced almost zero loads, the resulting kinematics were mainly load-driven, and the applied joint contact loads were closely reproduced. Outside these bounds, however, the springs stiffened and corrected the inlay kinematics towards the measured AP displacement and IE rotation, hence yielding joint contact loads that differed from those measured. Thus, the springs’ effect is to reconcile the in vivo loads and kinematics and obtain the best reproduction of both in the contact mechanics. While their original purpose simply is to simultaneously reduce both the load and kinematics errors, they could be considered a compensation for left out in vivo soft-tissue structures and inertial effects as well as differing geometries, contact, and material properties.
The springs were designed to produce close to zero loads for small kinematics deviations, but large reaction loads for kinematic deviations exceeding the fluoroscopy measurement error. Thus, their stiffnesses were defined as where
was the relative AP or IE displacement of the reference points,
was the aforementioned kinematics boundary (in mm or °), and
was the stiffness at the boundary when
The exponent of 7 was chosen so that
would be close to zero for small
slowly start to rise when approaching the boundary (
) and sharply rise thereafter (
). The stiffness
was estimated based on the absolute maximum values of the CAMS-Knee loads over all subjects, trials, and activities, such that the spring load would have roughly the same magnitude as the applied load at the error bounds. Values of
and
were used in the AP and IE directions, respectively.
The in vivo loads and kinematics data of the CAMS-Knee dataset were smoothed using a fourth-order Butterworth low-pass filter with a cut-off frequency of 6 Hz (Winter Citation2009, p. 65). Then, we ran the LC, DC, and LDCS scenarios for one cycle of each of the six CAMS-Knee subjects’ level walking, downhill walking (data unavailable for one subject), stair descent, sit-to-stand-to-sit, and squat activities, totaling 29 simulations for each of the three scenarios. Afterwards, each of the twelve tibiofemoral load and kinematic components was extracted from the FE results and compared to the in vivo data by calculating the overall RMSE between the simulated and measured time series curves. Additionally, to evaluate the detrimental effect of noise in input loads and kinematics on the contact point location, the jitter in the AP CoP was evaluated by calculating the power spectral density (Persson et al. Citation2005), which is a measure of the roughness of a curve based on its Fourier transform.
Results
The LC models’ kinematics exhibited maximum errors of up to 7 mm and 14° () and overall RMSEs higher than 1° and 1 mm (), while unsurprisingly showing excellent agreement with the measured and applied AP and IE loads. In contrast, DC models showed the largest load errors of up to 29 Nm and 1206 N, exceeding 100% relative error, but with matching kinematics. While the LDCS models also exhibited load errors, they were only roughly a quarter of the DC models’ AP force and half the IE moment RMSE (). Moreover, IE rotation and AP displacement were almost always within the fluoroscopy error bounds (). For this, the corrective springs exhibited loads of 15.8 ± 23.5 N in AP and 1.6 ± 0.9 Nm in IE direction (mean ± SD). In all other DoFs, where boundary conditions were the same between the three scenarios, there were no discernible differences, as expected, with one exception. The extension moment errors were almost twice as large for the DC model compared to the LC and LDCS models.
Figure 2. Range of maximum errors of each load and kinematic component over the motion cycle for all 29 models (six subjects, five activities) for each boundary condition scenario. The grey background areas represent the fluoroscopy and load measurement errors reported by Taylor et al. (Citation2017).

Table 2. Overall RMSE of each load and kinematic component over the motion cycle for the three boundary condition scenarios (BCS) and all 29 simulations.
The AP CoP location varied frequently by up to 20 mm for the DC scenario, as illustrated for one trial of level walking (, top). Importantly, this jittering was not solely due to occasional edge loading but occurred under regular contact conditions at the condylar surface. Confirming the visual inspection, the high frequency components (, bottom) of the DC scenario AP CoP were approximately an order of magnitude larger than for the LDCS scenario. For the LC scenario, the high frequency components were another order of magnitude smaller than for the LDCS scenario.
Discussion
Understanding, simulating, and predicting joint contact mechanics remains difficult due to the inability of existing models to simultaneously reproduce both in vivo loads and kinematics. Here, we introduced a novel method to incorporate both load and displacement boundary conditions (LDCS) in models of the knee. Prediction errors of the LDCS as well as conventional LC and DC setups were evaluated using the CAMS-Knee datasets (Taylor et al. Citation2017) and compared among the three methods.
The conventional LC models were unable to accurately reproduce in vivo kinematics from in vivo loads, shown by a RMSE of 2.5° in IE rotation and 1 mm in AP translation (). The DC models, on the other hand, produced by far the largest contact load RMSE of up to 228 N and 9.3 Nm. The jittery CoP location and flexion moment errors suggest that even filtered single-plane fluoroscopy kinematics alone are insufficiently accurate to reproduce in vivo contact mechanics.
The LDCS method much improved upon these mismatches. The nonlinear springs enabled concurrent application of loads and kinematics and kept AP and IE kinematics inside the specified bounds, in our case the fluoroscopy error. The necessary corrective loads represented soft-tissue, geometry, and inertial effects, but were still significantly smaller than the corresponding joint contact loads measured in vivo. Thus, while the LDCS scenario also showed joint contact load errors, they were nevertheless a factor of two to four smaller than in the DC scenario. The LDCS scenario also much improved the unrealistic jittering of the DC scenario’s AP CoP (). This jittering could be further remedied by introducing e.g. contact damping in computational models.
All three methods showed large RMSE of more than 5 Nm in the extension moment (), plausibly caused by errors in the CoP. This is indicated by the DC models having both the most AP CoP jittering and largest extension moment errors. Some of the extension moment errors may also be due to a different geometry of the ultra-congruent inlay in vivo following plastic deformation of the inlay (Teeter et al. Citation2015).
Few studies evaluated prediction errors of in vivo loads in AP or IE directions. For example, Fitzpatrick and Rullkoetter (Citation2014) predicted tibiofemoral kinematics from the loads and then reapplied the resulting kinematics to determine whether the same original loads would be generated. In this idealized scenario with matching loads and kinematics, they found RMSE of less than 2 Nm and 70 N in IE rotation and AP loads. While the DC models showed higher errors () because of the presence of measurement noise in the kinematics combined with an ultra-congruent implant geometry, the LDCS models successfully achieved very similar values (2.7 Nm and 54 N) to the idealized Fitzpatrick and Rullkoetter study (2014).
Overall, the LDCS method can be characterized as an extension of the pure DC method that yields significantly lower errors in the contact loads. The balance between reproducing in vivo loads or kinematics could be further tuned by modifying the acceptable deviation limit from the in vivo kinematics.
Currently, only the CAMS-Knee (Taylor et al. Citation2017) and Grand Challenge (Fregly et al. Citation2012) datasets contain both in vivo tibiofemoral loads and kinematics. However, these datasets already enable investigations of a wide range of research questions, presenting ample opportunities for the LDCS method to be applied. As such, investigation of knee joint contact mechanics to a level of accuracy not previously achieved becomes possible.
However, the LDCS method is also applicable outside of these datasets. In practice, researchers would be more likely to have access to measured kinematics from fluoroscopy complemented by loads computed from musculoskeletal models. As load-measuring implants are still extremely rare, modelled joint contact loads can be used with the LDCS method instead. Applicable six DoF implant loads have been calculated using freely available (Smith et al. Citation2016, github.com/clnsmith/opensim-jam) and commercial (Kia et al. Citation2014; Frigo and Donno Citation2021) multibody dynamics software. In the LDCS approach, these loads would be enforced less strictly than the kinematics, which will generally not exceed the specified error bounds. The LDCS method is therefore well suited for the described situation where loads are estimated and therefore uncertain but in vivo fluoroscopy kinematics are available.
Furthermore, our LDCS method can also be used to reproduce laboratory knee simulator tests. In such tests, the loads and motion of the machine can be recorded, yielding synchronized input loads and kinematics for a model to replicate. With the LDCS method, the same contact conditions as in the laboratory test can be replicated in a FE model better than with LC and DC methods. This enables further investigations into implant wear and damage, e.g. to evaluate internal stresses or validate computational wear models.
Acknowledgements
The authors thank Zimmer Biomet for providing access to the Innex implant CAD files.
Disclosure statement
No potential conflict of interest was reported by the authors.
Data availability statement
The CAMS-Knee datasets analyzed in this study are available upon request at https://cams-knee.orthoload.com for research purposes.
Additional information
Funding
References
- Acker S, Li R, Murray H, John PS, Banks S, Mu S, Wyss U, Deluzio K. 2011. Accuracy of single-plane fluoroscopy in determining relative position and orientation of total knee replacement components. J Biomech. 44(4):784–787.
- ASTM International. 2017. ASTM F3141-17a. Standard Guide for Total Knee Replacement Loading Profiles. West Conshohocken: ASTM International, [accessed 2021 May 10].
- Banks SA, Hodge WA. 1996. Accurate measurement of three-dimensional knee replacement kinematics using single-plane fluoroscopy. IEEE Trans Biomed Eng. 43(6):638–649.
- Carr BC, Goswami T. 2009. Knee implants: review of models and biomechanics. Mater Design. 30(2):398–413.
- Catani F, Innocenti B, Belvedere C, Labey L, Ensini A, Leardini A. 2010. The Mark Coventry award: articular contact estimation in TKA using in vivo kinematics and finite element analysis. Clin Orthop Relat Res. 468(1):19–28.
- D’Lima DD, Patil S, Steklov N, Colwell CW. 2011. The 2011 ABJS Nicolas Andry Award: ‘Lab’-in-a-knee: in vivo knee forces, kinematics, and contact analysis. Clin Orthop Relat Res. 469(10):2953–2970.
- Dreyer MJ, Trepczynski A, Hosseini Nasab SH, Kutzner I, Schütz P, Weisse B, Dymke J, Postolka B, Moewis P, Bergmann G, et al. 2022. European society of biomechanics S.M. Perren Award 2022: standardized tibio-femoral implant loads and kinematics. J Biomech. 141:111171.
- Fitzpatrick CK, Rullkoetter PJ. 2014. Estimating total knee replacement joint load ratios from kinematics. J Biomech. 47(12):3003–3011.
- Fregly BJ, Banks SA, D'Lima DD, Colwell CW. 2008. Sensitivity of knee replacement contact calculations to kinematic measurement errors. J Orthop Res. 26(9):1173–1179.
- Fregly BJ, Besier TF, Lloyd DG, Delp SL, Banks SA, Pandy MG, D'Lima DD. 2012. Grand challenge competition to predict in vivo knee loads: grand challenge competition. J Orthop Res. 30(4):503–513.
- Fregly BJ, Rahman HA, Banks SA. 2005a. Theoretical accuracy of model-based shape matching for measuring natural knee kinematics with single-plane fluoroscopy. J Biomech Eng. 127(4):692–699.
- Fregly BJ, Sawyer WG, Harman MK, Banks SA. 2005b. Computational wear prediction of a total knee replacement from in vivo kinematics. J Biomech. 38(2):305–314.
- Frigo CA, Donno L. 2021. The effects of external loads and muscle forces on the knee joint ligaments during walking: A Musculoskeletal Model Study. Applied Sciences. 11(5):2356.
- Godest AC, Beaugonin M, Haug E, Taylor M, Gregson PJ. 2002. Simulation of a knee joint replacement during a gait cycle using explicit finite element analysis. J Biomech. 35(2):267–275.
- Gray HA, Guan S, Thomeer LT, Schache AG, de Steiger R, Pandy MG. 2019. Three-dimensional motion of the knee-joint complex during normal walking revealed by mobile biplane x-ray imaging. J Orthop Res. 37(3):615–630.
- Heinlein B, Graichen F, Bender A, Rohlmann A, Bergmann G. 2007. Design, calibration and pre-clinical testing of an instrumented tibial tray. J Biomech. 40: s 4–S10.
- Hoff WA, Komistek RD, Dennis DA, Gabriel SM, Walker SA. 1998. Three-dimensional determination of femoral-tibial contact positions under in vivo conditions using fluoroscopy. Clin Biomech (Bristol, Avon). 13(7):455–472.
- Hosseini Nasab SH, Smith CR, Postolka B, Schütz P, List R, Taylor WR. 2021. In vivo elongation patterns of the collateral ligaments in healthy knees during functional activities. J Bone Joint Surg Am. 103(17):1620–1627.
- Imani Nejad Z, Khalili K, Hosseini Nasab SH, Schütz P, Damm P, Trepczynski A, Taylor WR, Smith CR. 2020. The capacity of generic musculoskeletal simulations to predict knee joint loading using the CAMS-knee datasets. Ann Biomed Eng. 48(4):1430–1440.
- International Organization for Standardization. 2009. ISO 14243-1:2009. Implants for surgery—Wear of total knee-joint prostheses—Part 1: loading and displacement parameters for wear-testing machines with load control and corresponding environmental conditions for test. Geneva: ISO. https://www.iso.org/standard/44262.html.
- International Organization for Standardization. 2014. ISO 14243-3:2014. Implants for surgery—Wear of total knee-joint prostheses—Part 3: loading and displacement parameters for wear-testing machines with displacement control and corresponding environmental conditions for test. Geneva: ISO. https://www.iso.org/standard/56649.html.
- Kia M, Stylianou AP, Guess TM. 2014. Evaluation of a musculoskeletal model with prosthetic knee through six experimental gait trials. Med Eng Phys. 36(3):335–344.
- Kirking B, Krevolin J, Townsend C, Colwell CW, D’Lima DD. 2006. A multiaxial force-sensing implantable tibial prosthesis. J Biomech. 39(9):1744–1751.
- Lin Y-C, Haftka RT, Queipo NV, Fregly BJ. 2010. Surrogate articular contact models for computationally efficient multibody dynamic simulations. Med Eng Phys. 32(6):584–594.
- Mahfouz MR, Hoff WA, Komistek RD, Dennis DA. 2003. A robust method for registration of three-dimensional knee implant models to two-dimensional fluoroscopy images. IEEE Trans Med Imaging. 22(12):1561–1574.
- Navacchia A, Rullkoetter PJ, Schütz P, List RB, Fitzpatrick CK, Shelburne KB. 2016. Subject-specific modeling of muscle force and knee contact in total knee arthroplasty. J Orthop Res. 34(9):1576–1587.
- Persson BNJ, Albohr O, Tartaglino U, Volokitin AI, Tosatti E. 2005. On the nature of surface roughness with application to contact mechanics, sealing, rubber friction and adhesion. J Phys condens Matter. 17(1):R1–R62.
- Pfitzner T, Moewis P, Stein P, Boeth H, Trepczynski A, von Roth P, Duda GN. 2018. Modifications of femoral component design in multi-radius total knee arthroplasty lead to higher lateral posterior femoro-tibial translation. Knee Surg Sports Traumatol Arthrosc. 26(6):1645–1655.
- Postolka B, Schütz P, Fucentese SF, Freeman MAR, Pinskerova V, List R, Taylor WR. 2020. Tibio-femoral kinematics of the healthy knee joint throughout complete cycles of gait activities. J Biomech. 110:109915.
- Prins AH, Kaptein BL, Stoel BC, Nelissen RGHH, Reiber JHC, Valstar ER. 2011. Integrated contour detection and pose estimation for fluoroscopic analysis of knee implants. Proc Inst Mech Eng H. 225(8):753–761.
- Reinders J, Sonntag R, Vot L, Gibney C, Nowack M, Kretzer JP. 2015. Wear testing of moderate activities of daily living using in vivo measured knee joint loading. Zadpoor AA, editor. PLoS One. 10(3):e0123155.
- Schütz P, Postolka B, Gerber H, Ferguson SJ, Taylor WR, List R. 2019. Knee implant kinematics are task-dependent. J R Soc Interface. 16(151):20180678.
- Shu L, Hashimoto S, Sugita N. 2021. Enhanced in-silico polyethylene wear simulation of total knee replacements during daily activities. Ann Biomed Eng. 49(1):322–333.
- Smith CR, Vignos MF, Lenhart RL, Kaiser J, Thelen DG. 2016. The influence of component alignment and ligament properties on tibiofemoral contact forces in total knee replacement. J Biomech Eng. 138(2):021017.
- Taylor WR, Schütz P, Bergmann G, List R, Postolka B, Hitz M, Dymke J, Damm P, Duda G, Gerber H, et al. 2017. A comprehensive assessment of the musculoskeletal system: the CAMS-Knee data set. J Biomech. 65:32–39.
- Teeter MG, Parikh A, Taylor M, Sprague J, Naudie DD. 2015. Wear and creep behavior of total knee implants undergoing wear testing. J Arthroplasty. 30(1):130–134.
- Varadarajan KM, Moynihan AL, D'Lima D, Colwell CW, Li G. 2008. In vivo contact kinematics and contact forces of the knee after total knee arthroplasty during dynamic weight-bearing activities. J Biomech. 41(10):2159–2168.
- Winter DA. 2009. Biomechanics and motor control of human movement. 4th ed. Hoboken, N.J: Wiley.