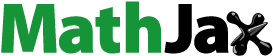
ABSTRACT
The main aim of this paper is to analyse the performance of different intelligent compaction measurement values (ICMVs) and compare them with spot test measurements for asphalt pavement. To accomplish this, a two-layer asphalt testbed was constructed using an instrumented double-drum roller. All ICMVs [compaction meter value (), compaction control value (
), roller-integrated stiffness (
), and vibratory modulus (
)] were calculated using the same accelerometer data collected during the construction of the asphalt testbed. The analysis of ICMV data showed a strong correlation between
and
, while
and
demonstrated a strong correlation only when double jump of the roller drum did not occur. Further, none of the ICMVs recorded during the last roller pass of asphalt compaction showed an acceptable correlation with asphalt densities [measured using a non-nuclear density gauge (NNDG)], mainly due to the influences of the underlying support and asphalt temperature on ICMVs. A correction method to decouple the influence of underlying support on
was developed and validated using existing data from the literature. The applicability of this method for different field scenarios was demonstrated using numerical modelling.
1. Introduction
Asphalt is a mixture of aggregates with bitumen [3% to 7% by mass of aggregates (Department of infrastructure energy and resources Citation2011)] which is used to construct and maintain road pavements, parking lots, airport runways, and playing courts (Australian Asphalt Pavement Association Citation2014). Asphalt layers are constructed by paving hot asphalt material [125°C to 185°C (Halton public works department Citation2021)] on the existing support layers using a paver and immediately compacting the freshly-laid asphalt to the target density using double-drum rollers. The aim of the compaction process is to achieve the desired density while maintaining uniformity throughout the pavement. Previous studies have reported that a minimal rise in the in-situ density of an asphalt pavement can result in a considerable increase in the service life of the pavement. Austroads (Citation1999) reported that a decrease of the asphalt air voids content from 8% to 5% enhances the service life of an asphalt pavement by 50%. As in-situ density provides such benefits, the cost of achieving higher in-situ density is lower than the cost savings from operational and maintenance costs. Laboratory studies of asphalt have concluded that a 1% reduction in air voids increases flexural fatigue life by 8% to 44% and decreases rutting by 7% to 66% (Tran et al. Citation2016).
Road authorities use the density of the compacted asphalt layers as the acceptance criterion for asphalt compaction works (Vicroads Citation2017). The density of the compacted asphalt layers can be determined either in a laboratory by extracting cores from the layers at selected spots or in-situ using a nuclear density gauge (NDG) at selected spots. However, only three spot tests are required for an asphalt layer with an area up to 500 m2 and only six spot tests are required for an asphalt layer with an area between 500 m2 to 5000 m2 (Vicroads Citation2017). Therefore, these test methods fail to provide the compaction quality of the entire area of the asphalt layer.
Intelligent compaction (IC) is an evolving technology which uses various sensors, as shown in .
Figure 1. Schematic diagram of an intelligent compaction (IC) roller (Sivagnanasuntharam et al. Citation2021).

The Global Positioning System (GPS) is used in IC rollers to track the location of the roller. This location information is then used to determine the speed of the roller and the number of times that the roller passes a particular spot (known as ‘pass count’) in real-time. An accelerometer (or a pair of accelerometers) is mounted on the axis of the vibrating drum of the roller to record the vertical acceleration of the drum. The acceleration data are then used to calculate a parameter called the intelligent compaction measurement value (ICMV) along with the frequency and amplitude of the vibration in real-time. During asphalt compaction, infrared (IR) temperature sensors are mounted at the front and back of the roller to measure the surface temperature of the asphalt layer being compacted. All the required real-time manipulations are performed by the control box mounted on the roller and the results (roller speed, pass count, frequency of vibration, amplitude of vibration, ICMV and asphalt surface temperature) are displayed as colour-coded maps on the operator interface so that the operator can adjust the amplitude and frequency of the vibration to increase the efficiency of compaction in real-time in conformity with the specifications. As ICMV requires accelerometer data, it can be used with vibratory rollers only. However, other measurements (roller speed, pass count, and asphalt surface temperature) can be reported for other types, such as static, pneumatic-tyred, and oscillatory rollers (Gallivan et al. Citation2011).
1.1. Intelligent compaction measurement values (ICMVs)
According to U.S. Department of Transportation Federal Highway Administration (Citation2017), ICMV is a collective term representing different values commercially available for asphalt compaction, namely, compaction meter value [ (Chang et al. Citation2014)], compaction control value [
(Chang et al. Citation2014)], vibratory modulus [
(Pistrol et al. Citation2017)], roller integrated stiffness [
(Anderegg and Kaufmann Citation2004)], and density direct (Commuri et al. Citation2008).
is defined as follows (Chang et al. Citation2014):
(1)
(1) where, Ω is the angular speed of the vibration, and
and
are the amplitude of the acceleration of the fundamental and first harmonic components of the vibration frequencies, respectively. The following roller manufacturers use
: Dynapac (Sweden), Hamm (referred to as the Hamm meter value, HMV, Germany) and Caterpillar (America) (Chang et al. Citation2011). Further, IC retrofit kit manufacturers such as Trimble (US) use the parameter
. On the basis of
, Ma et al. (Citation2022) proposed a new quality evaluation index, named Acceleration Intelligent Compaction Value (
), for asphalt compaction taking into account the second harmonic component of the vibration frequencies.
is defined as follows (Ma et al. Citation2022):
(2)
(2) where,
is the amplitude of the acceleration of the second harmonic component of the vibration frequencies and
is a constant.
, which is available with rollers manufactured by Sakai (Japan), is defined as follows (Chang et al. Citation2014):
(3)
(3) where,
,
,
, and
are the amplitude of the acceleration of the second, half, one and a half, and two and a half harmonic components of the vibration frequencies, respectively. Equations 4 and 5 are numerically solved to determine the vibratory modulus,
(Pistrol et al. Citation2017), which is available with BOMAG (German) rollers.
(4)
(4)
(5)
(5) where,
is the displacement of the drum,
is the pavement layer-drum interaction force,
is the length of the drum,
is the contact width of the drum,
is the Poisson’s ratio, and
is the radius of the drum (Chang et al. Citation2014, Oh Citation2014, Hu Citation2018). Roller-integrated stiffness (
), which is available with Ammann (Switzerland) rollers, is defined as follows:
(6)
(6) where,
is the mass of the drum,
is the eccentric mass,
is the eccentricity,
is the amplitude of drum displacement, and
is the phase lag between drum acceleration and excitation force (Anderegg and Kaufmann Citation2004).
1.2. ICMV-asphalt density correlation
Although IC rollers can provide ICMV for the entire asphalt layer being compacted, ICMV cannot be directly used to access the asphalt density unless a strong correlation between ICMV and asphalt density is established. Several field studies have been undertaken to examine the ICMV-asphalt density correlation (Maupin Citation2007, White et al. Citation2010, Savan et al. Citation2015, Chang et al. Citation2018, Hu et al. Citation2019, Foroutan et al. Citation2020), and the reported linear regression correlation coefficients between ICMV and asphalt density are summarised in .
Table 1. Correlation between ICMV and asphalt density [adapted from (Sivagnanasuntharam et al. Citation2021)].
It can be noticed in that 15 field trials (out of 18 filed trials) reported an R2 value less than 0.5, which indicates that a strong correlation between ICMV and asphalt density has not been demonstrated. ICMVs can be influenced by numerous factors including the mass and dimensions of the roller, the amplitude and frequency of vibration, the speed of the roller, asphalt mix proportioning, underlying support condition, and asphalt temperature (U.S. Department of Transportation Federal Highway Administration Citation2017). Therefore, the outcomes of the field studies summarised in cannot be compared to evaluate the performance of different ICMVs. This requires the calculation of different ICMVs using the same accelerometer data for the same compaction process. However, according to the best knowledge of the authors, none of the studies reported in the literature determined different ICMVs for the same compaction process.
1.3. Objectives
The primary objective of this study was to undertake a targeted asphalt compaction experiment in the field to examine different ICMVs calculated from the same accelerometer data and evaluate the performance of each ICMV with respect to asphalt density prediction during compaction. In addition, the following supplementary objectives were incorporated to accomplish the main objective.
Compare in-situ asphalt densities measured during compaction using different methods: core cutting, nuclear density gauge (NDG) – backscatter method (BS), NDG – direct transmission method (DT), and non-nuclear density gauge (NNDG).
Compare in-situ asphalt deflection measured using different methods: falling weight deflectometer (FWD), and lightweight deflectometer (LWD).
Evaluate the in-depth temperature variation in the asphalt layer during compaction and develop a temperature prediction model for ICMV calculations in real-time during asphalt compaction [this work is reported in Sivagnanasuntharam et al. (Citation2022)].
This paper presents the analysis of IC data and spot measurement data collected during the construction of a two-layer asphalt testbed. Details of the material characteristics, roller instrumentation, and laboratory and field experiments are given in Section 2, and analysis of the spot measurement data is presented in Section 3. The analysis of the IC data is discussed in Section 4. A potential method to decouple the influence of underlying support on ICMV () is presented in Section 5. Finally, the conclusions are reported in Section 6.
2. Construction of a two-layer asphalt testbed
A small-scale asphalt testbed (8 m long and 2 m wide) was constructed using an AC14 mix (nominal size of aggregate 14 mm) with C320 bitumen in two layers. The target thickness of each layer was 75 mm after compaction and the initial thickness of the asphalt layer before compaction was 100 mm. The first layer was constructed directly on an existing cement-treated base (CTB) on the first day (layer#1) and the second layer was constructed on the first asphalt layer on the following day (layer#2). illustrates the layout of the testbed and the brushed CTB surface before the first layer of asphalt was placed.
Figure 2. (a) Layout of testbed and (b) brushed CTB surface before placement of first asphalt layer.

2.1. Material properties
shows the particle size distribution of the aggregate obtained using sieve analysis (AS Citation1141.Citation11.Citation1 Citation2009) along with the upper and lower limits recommended for the AC14 mix. presents the physical properties of the aggregate and AC14 asphalt mix according to the respective test standards.
Table 2. Properties of AC14 asphalt aggregate.
2.2. Roller instrumentation
A 4-tonne double drum vibratory roller (Dynapac CC142) was used for asphalt compaction, as shown in . The properties of the vibrating drum of the roller are given in .
Table 3. Properties of vibrating drum of Dynapac CC142 roller.
The following instruments were employed during the field experiment:
A Trimble’s IC kit (CCS900) was retrofitted to the roller. The kit includes GPS (MS972 Smart GNSS and SNR2434 2.4 GHZ machine radio), IR temperature sensors (IS310), accelerometer (CM310 with Firmware Version 2) and control box (CB460) for real-time CMV calculation; and
as Trimble’s IC kit cannot provide raw accelerometer data, an additional accelerometer (Triaxial Delta Tron accelerometer type 4506 B 003) was attached to the roller to obtain raw accelerometer data for ICMV calculations.
2.3. Field experiments
As shown in , a total of eight spots at 1 m spacings (S1 to S8) were selected for spot tests such as NDG, NNDG, LWD, and FWD. The spot test measurements were performed (i) on the existing underlying support before placing the asphalt layer (except NDG and NNDG), (ii) during asphalt compaction (NDG and NNDG only), and (iii) after asphalt compaction when the compacted asphalt layer cooled down. Further details are provided in the following subsections.
2.3.1. Pre-construction experiments
The following tests were carried out on the existing underlying support before the first asphalt layer was placed:
pre-mapping of the underlying support (rolling the instrumented roller with minimum vibration before placing the asphalt layer to record the IC data for the existing support);
levelling survey (device: Bosch GOL 32 D Professional); and
LWD (device: Dynatest 3032) and FWD (device: Dynatest 8000) tests.
2.3.2. Experiments during asphalt compaction
In-situ density measurements were performed during asphalt compaction after each roller pass. Of the eight spots shown in , the asphalt density was measured using (i) NDG (device: Troxler 3440 plus)-DT method at spots S2 and S4, (ii) NDG-BS method at spots S3 and S5, and (iii) NNDG (device: Trans tech PQI 380) at the remaining spots.
2.3.3. Post-construction experiments
After the asphalt compaction process, the following tests were carried out on each asphalt layer when the compacted asphalt layer cooled to ambient temperature:
LWD and FWD () tests;
density measurement using NNDG, NDG-BS method, and NDG-DT method at all spots; and
core extraction: full-depth cores were extracted at all spots (S1 to S8) at the end of the testbed experiments. The extracted cores were used to measure the asphalt layer thickness, and compacted density.
3. Analysis of spot measurement data
3.1. Variation of asphalt density during compaction
shows the variation of asphalt density during compaction for both asphalt layers.
Figure 7. Variation of asphalt density (measured using NDG and NNDG) during compaction: (a) asphalt layer#1; and (b) asphalt layer#2 (S1 to S7 refer to the test spots – refer to ).

According to the NNDG results in , it appears that both the asphalt layers reached the maximum possible density in the first three roller passes at most spots. However, the NDG results show rapid fluctuations in the asphalt density as the number of roller passes increases. This could be due to the fact that the accuracy of gamma-ray detectors in the NDG device is affected by temperatures higher than 70°C (Palilla Citation2014).
3.2. Comparison of different methods of asphalt density measurement
Four different asphalt density measurement methods (NNDG, NDG-BS method, NDG-DT method, and core density measurement) are compared in . Core density measurement was performed in the laboratory after extracting the cores from the asphalt testbed, while all the other measurements were performed onsite at the end of asphalt compaction when the asphalt layer cooled to ambient temperature. According to , NNDG and NDG-BS measurements are in better agreement with the core density values (on average, only 1% difference was noticed between core density values, NNDG and NDG-BS measurements) than the NDG-DT measurements (on average, 3.5% difference was noticed between core density values and NDG-DT measurements, refer to Appendix 1 for details). It appears that NNDG measures asphalt density with acceptable accuracy when the asphalt is dry and has cooled to ambient temperature. However, the accuracy of NNDG for measuring the density of fresh asphalt during compaction (when the asphalt is wet and hot) is limited, because NNDG measurements are often affected by temperature and moisture. Although the NDG – DT method shows a lower density value of 89% of TMD, the range of density values determined using the cores falls between 93% and 95% of TMD.
Figure 8. Comparison of different methods of measurement of asphalt density (asphalt density measurements were performed at the end of compaction process): (a) asphalt layer#1; and (b) asphalt layer#2.

It should be noted that the target density of the asphalt layer was 96% of TMD. As even a 1% increase in air voids can reduce the flexural fatigue life of an asphalt pavement by up to 44% (Tran et al. Citation2016), the density achieved during compaction of the testbed was not satisfactory. Although a total of eight roller passes were used to compact each asphalt layer, no significant change in the asphalt density was observed after the initial three roller passes (based on the NNDG readings taken with each roller pass). This observation is consistent with the asphalt compaction data reported in the literature, i.e. the optimum roller pass count for asphalt compaction ranges between 2–3 (Chang et al. Citation2014, Yoon et al. Citation2015, Polaczyk et al. Citation2021). The main causes of this phenomenon are the reduction of asphalt workability with reducing temperature, and the inability of the roller to provide the additional energy required to compact the asphalt layer further to achieve the desired density.
3.3. Comparison of different in-situ methods of modulus measurement
This section analyses the FWD and LWD measurements taken on the compacted asphalt layers in accordance with Austroads AG:AM/T006 (Citation2011) and ASTM E2583 − Citation07 (Citation2015), respectively. Both FWD and LWD measure the surface deflection of a dynamic load applied on the pavement surface, and the modulus of the pavement layer is determined using the measured deflection. A constant dropping mass of 10 kg and a dropping height of 68.6 cm (27 in.) were used in LWD testing and the exerted dynamic load was measured and used in the analysis (refer to Appendix 2 for further details). During FWD testing, the dropping mass was adjusted in such a way to exert a target dynamic load of 60 ± 6 kN in accordance with Austroads AG:AM/T006 (Citation2011).
In , it can be observed that the FWD and LWD deflection values at spots S1 and S2 for CTB, asphalt layer#1 and asphalt layer#2 are almost two times higher than those of other spots. The reason for this observation is that the CTB material in the vicinities of S1 and S2 is relatively weaker than in the other spots. The FWD deflection at spots S1 to S4 decreases after the construction of asphalt layers over the CTB, as the stiffness of the asphalt layers is higher than that of CTB. However, the FWD deflection at spots S5 and S6 increases after the construction of asphalt layer#1 and then decreases after the construction of asphalt layer#2. This is because the density measured in the laboratory using extracted cores of asphalt layer#1 (ranges from 2,123 kg/m3 to 2,241 kg/m3) is lower than that of asphalt layer#2 (ranges from 2,247 kg/m3 to 2,301 kg/m3), as shown in . As the influence depth of LWD loading [∼1.5–2 times the load plate diameter, which is 300 mm (Narnoli and Suman Citation2020)] is less than the influence depth of FWD loading, the LWD deflection is predominantly governed by the properties of the top layer, while the FWD deflection is governed by the properties of both top and underlying layers. Therefore, the effect of low density in asphalt layer#1 is evident in the LWD deflection at all the spots, as shown in (b).
4. Analysis of intelligent compaction data
The raw accelerometer data were recorded during asphalt compaction using LabView software (National Instruments Corp. Citation2022) at a sampling frequency of 1,000 Hz. Different ICMVs were then calculated by performing a post-analysis of the recorded accelerometer data. illustrates the procedure of ICMV calculation adopted in this study.
4.1. Calculation of 
and 

The calculation of and
requires only the vertical acceleration of the roller drum as the input. As the accelerometer generates an acceleration signal in the time domain, a fast Fourier transform (FFT) was performed to obtain the vertical acceleration signal in the frequency domain. shows a typical vertical acceleration signal in the time domain and frequency domain, respectively.
Figure 11. Vertical acceleration of roller drum during roller pass#4 for asphalt layer#1: (a) in time domain; and (b) in frequency domain.

As shown in , the amplitudes of the fundamental frequency and harmonics are extracted from the FFT result to calculate (Equation 1) and
(Equation 3).
4.2. Calculation of 
and 

In addition to vertical accelerometer data, the calculation of and
requires some additional input parameters, as shown in . Most of these parameters, such as drum width, drum radius, drum mass, eccentric mass, eccentricity, and asphalt Poisson’s ratio, can be pre-defined. However, the phase lag between the eccentric force and ground reaction force [referred to as phase angle (
)] needs to be determined. When an IC roller is manufactured by its original equipment manufacturer (OEM), a sensor is integrated inside the roller drum to measure phase angle (
). Either a proximity sensor such as the Hall effect sensor (Rinehart and Mooney Citation2005) or an encoder is generally used for this purpose. However, retrofitting a conventional roller with such a sensor is not practicable. Accordingly, an estimation is required for the phase angle (
) to determine
and
.
4.2.1. Cross-correlation method
Although the vibratory rollers manufactured in recent times include a pair of identical eccentric masses in such a way to cancel out the horizontal component of the vibration, traditional vibratory rollers include only a single eccentric mass. Therefore, these traditional vibratory rollers also produce horizontal vibration. If the roller drum vibrates freely without any interruption from the ground reaction, the phase difference between the vertical component of the acceleration and the horizontal component of the acceleration is 90°. However, the phase of the vertical acceleration component is altered due to the interruption of the ground reaction. The effect of ground reaction on the horizontal acceleration component can be ignored as the ground reaction is predominantly in the vertical direction. The FFT of a typical horizontal acceleration, as shown in , verifies this assumption [i.e. if the horizontal acceleration is significantly affected by the ground reaction, the horizontal acceleration should also have harmonics similar to the vertical acceleration, as shown in (b)].
Figure 12. Horizontal acceleration of roller drum in frequency domain during roller pass#4 for asphalt layer#1.

The cross-correlation method (Ranasinghe et al., Citation2022), which can be used to determine the phase difference between two time-domain signals (Kostiukov Citation2020), is used in this study to determine the phase difference between horizontal acceleration and vertical acceleration. The required is then obtained by subtracting 90° from the determined phase difference. This
corresponds to
. Similarly, each harmonic has its own phase angle. The amplitudes of the harmonics are significantly less than
as shown in (b). Therefore, it is reasonable to consider only
to perform double integration for the calculation of the vertical displacement of the roller drum. It should be noted that if the entire acceleration signal is used to perform double integration, filtering the raw data is essential to remove or reduce the noise. Once
and vertical displacement are obtained,
can be calculated using Equation 6.
can then be calculated using Equations 4 and 5 by substituting
in place of
in Equation 4.
4.3. Sampling frequency and sample width for FFT analysis
As the calculation of each ICMV requires FFT, the accuracy of the calculated ICMV is highly dependent on the accuracy of FFT. According to the sampling theorem (Lai Citation2003), the sampling frequency should be selected in such a way that the highest frequency in the signal is less than half the sampling frequency. While the highest frequency of interest for ICMV calculation is 3, a frequency component up to 5
can be expected in the signal. Therefore, a sampling frequency of 20
(1 kHz,
is 50 Hz for the roller) was used in the analysis. For a given sampling frequency, the accuracy of FFT can significantly drop if the sample width is insufficient (Reyes and Forgach Citation2016). In order to determine a suitable sample width for the selected sampling frequency, FFT analysis was performed with different sample widths, as shown in .
Figure 13. FFT of vertical acceleration data with different sample widths: (a) 2 s, (b) 1 s, (c) 0.5 s, (d) 0.2 s, (e) 0.1 s, and (f) 0.02 s.

According to (a), when the sample width is 2 s, the acceleration signal has only three frequency components; 50, 100, and 150 Hz. However, when the sample width is reduced to 0.02 s, FFT fails to detect these three frequency components. It detects a single peak instead [(f)]. This is because a sample width of 0.02 s is insufficient for 1 kHz of sampling frequency. Further, it detects multiple peaks of more than three for sample widths between 0.5 s to 0.1 s [(c – e)]. This behaviour can be better explained in conjunction with the speed of the roller. The average speed of the roller during compaction was about 0.5 m/s. Therefore, the distances travelled by the roller in 2, 1, 0.5, 0.2, 0.1, and 0.02 s are 1, 0.5, 0.25, 0.1, 0.05, and 0.01 m, respectively. The FFT performed with a sample width of 2 s represents the average of the signal obtained for 1 m distance, while the FFT performed with a sample width of 0.1 s represents the average of the signal obtained for only 0.05 m distance. Therefore, an optimum sample width should be selected, which is large enough to obtain accurate FFT and small enough to have sufficient spatial resolution in the ICMV calculation. Commercially-available IC rollers report ICMV every 0.3 m (Sivagnanasuntharam et al. Citation2021). Therefore, the calculation of ICMV every 0.1 m is sufficient to capture the local variation in ICMVs. Hence, the sample width of 0.5 s was used in the analysis.
4.4. Correlations among different ICMVs
and show the correlations between different ICMVs for the IC data obtained during the pre-mapping of the CTB layer and the compaction of asphalt layers, respectively (a colour-coded correlation matrix is presented in Appendix 5). summarises the average values of density and ICMVs for each layer in the pavement testbed.
Figure 14. Correlation between ICMVs for IC data obtained during pre-mapping of CTB layer: (a) vs.
, (b)
vs.
, (c)
vs.
, (d)
vs.
, (e)
vs.
, and (f)
vs.
.

Figure 15. Correlation between different ICMVs for IC data obtained during compaction of asphalt layers: (a) vs.
, (b)
vs.
, (c)
vs.
, (d)
vs.
, (e)
vs.
, and (f)
vs.
.

Table 4. Average densities and ICMVs.
The following observations can be made on the basis of the data presented in and .
and
are perfectly correlated, irrespective of the material being compacted. This is because the conversion of stiffness (
) to modulus (
) depends only on the width and radius of the vibrating drum, which are constants for a given roller. This perfect correlation may not be observed if IC data obtained from multiple rollers with different dimensions are pooled for the analysis.
While
and
correlated well for the IC data obtained during the compaction of both asphalt layers, they failed to show an acceptable correlation for the IC data obtained during the pre-mapping of the existing CTB layer. This is because
and
are approximately proportional when the sub-harmonic components are not present in the vertical acceleration of the roller drum. However, the presence of these sub-harmonic components in the vertical acceleration of the drum [which indicates the occurrence of double jump (Sivagnanasuntharam et al. Citation2021)] is evident during pre-mapping, as shown in .
Apart from the
–
correlation and the
–
correlation, none of the other combinations of ICMVs (
–
,
–
,
–
, and
–
) showed an acceptable correlation. The reason may be that
and
are purely empirical equations, whereas
and
are derived using elastic theory.
Figure 16. Presence of sub-harmonic components due to occurrence of double jump during pre-mapping of existing CTB layer.

shows the spatial variation of ICMVs obtained during pre-mapping and the last roller pass of asphalt layer compaction along with asphalt core density (measured at the end of compaction) and the reciprocal of the FWD peak deflection. The FWD test was performed on (i) the existing CTB layer before placing asphalt layer#1, (ii) asphalt layer#1 before placing asphalt layer#2, and (iii) asphalt layer#2 24 h after its construction. In , the above parameters are presented as percentages of their maximum values.
Figure 17. Spatial variation of ICMVs: (a) during pre-mapping, (b) during last pass of asphalt layer#1 compaction, and (c) during last pass of asphalt layer#2 compaction.

The following observations can be made on the basis of the data presented in .
The spatial distribution of ICMVs explains the perfect correlation between
and
for all the IC data and the acceptable correlation between
and
for the IC data obtained during asphalt compaction.
The reciprocal of the FWD peak deflection at each spot shows the same trend for all three cases, with the exception of spot S6 during pre-mapping. This indicates that FWD deflection is influenced by the properties of the underlying support, even after the construction of the asphalt layers above.
The asphalt density values obtained from core samples can also be considered to be consistent across the testbed for both asphalt layers. This suggests that
and
are well correlated with asphalt density. However, the ICMV-density correlation should be analysed using the data obtained in each roller pass to justify this.
4.5. Correlation of different ICMVs with asphalt density measurements
shows the correlation of different ICMVs obtained during each roller pass (eight roller passes in total for each asphalt layer) with the asphalt density measured using NNDG at the end of each respective roller pass.
Figure 18. Correlation of asphalt density measured using NNDG between each roller pass with different ICMVs: (a) , (b)
, (c)
, and (d)
.

According to , none of the ICMVs demonstrate an acceptable correlation with asphalt density. It should be noted that the density and ICMV data obtained for all the spots (S1 to S8) are pooled in the analysis shown in . Nevertheless, the correlation analysis performed using the density and ICMV data separately for each spot also show a poor correlation (R2 < 0.1). The main reasons for these poor correlations could be the influences of the underlying support and asphalt temperature on ICMVs (Sivagnanasuntharam et al. Citation2021).
5. Underlying support correction for 

While and
are considered as Level 1 ICMVs,
and
are treated as Level 3 ICMVs (U.S. Department of Transportation Federal Highway Administration Citation2017). Further,
is independent of roller dimension. Sivagnanasuntharam et al. (Citation2021) suggested that a suitable correction factor is required to decouple the effect of underlying support on
to achieve a better correlation between
and asphalt density.
5.1. Conceptual development of a correction method for effect of underlying support
is an index of the modulus of the pavement up to a certain depth, known as the influence depth of the roller drum, which ranges from 1.4 m to 2.2 m based on the roller’s configuration and dimensions (Fathi et al. Citation2021). If the same material appears throughout the influence depth,
is an index for the modulus of that material. Similarly, if two or more material layers appear within the influence depth,
is an index for the equivalent modulus of all the material layers. During pavement compaction,
is an index for the equivalent modulus of the underlying support and the pavement layer being compacted. Therefore, the system which consists of a roller drum, pavement layer (which is being compacted), and underlying support could be analysed using the analogy of an elastic rigid cylinder resting on an elastic half-space which has a thin coat of paint, as illustrated in .
Figure 19. Thin coat of paint on elastic half-space as an analogy for the pavement layer being compacted on an underlying support.

King (Citation1987) reported the equivalent elastic modulus of a system comprising an elastic rigid indenter, thin coating, and an elastic half-space as follows:
(7)
(7) where,
is the equivalent elastic modulus,
is the elastic modulus of the coating,
is the elastic modulus of the elastic half-space,
is the elastic modulus of the indenter,
is the Poisson’s ratio of the coating,
is the Poisson’s ratio of the elastic half-space,
is the Poisson’s ratio of the indenter,
is the square root of the projected contact area between the indenter and the film coating,
is the thickness of the top layer (paint coat), and
is an indenter shape-dependent constant. This approach works effectively when the ratio between the square root of the projected contact area and the thickness of the coating is in the range of 0–4 (King Citation1987).
The roller drum is made of steel with an elastic modulus of approximately 210 GPa (Chen et al. Citation2016), the elastic modulus of soils ranges between 2 MPa to 320 MPa (Yasir et al. Citation2018), and the elastic modulus of typical asphalt material is around 150 MPa at 49°C (Newcomb et al. Citation2002). The elastic modulus of asphalt during compaction is even lower than 150 MPa, as the asphalt temperature is above 80°C (Timm et al. Citation2001). Therefore, it is reasonable to ignore the third term in Equation 7 which carries the properties of the indenter. As the projected contact area is rectangular for roller compaction, the square root of the projected contact area can be presented as shown in Equation 8:
(8)
(8) One of the benefits of IC is the ability to detect weak spots in underlying layers through pre-mapping so that these weak spots can be strengthened before a new layer is placed (Chang et al. Citation2011). During pre-mapping, the IC roller is rolled with low vibration on the existing layer to record ICMV data and the spots which show a low ICMV are considered to be weak spots. Therefore, if an IC roller which provides
is used for pre-mapping, the
value reported during pre-mapping (
) could be considered as an index for the elastic modulus of the underlying support. Similarly, the
reported during the compaction of the new layer (
) could be considered as an index for the equivalent elastic modulus of the system which comprises the new layer and the underlying support.
An index for the elastic modulus of the top layer () can be derived by replacing
,
,
,
,
, and
with
,
,
,
(Poisson's ratio of the top layer),
(Poisson's ratio of the underlying support material), and
, respectively, and rearranging the terms in Equation 7 as shown below.
(9)
(9) Although the main interest of this paper is decoupling the effect of underlying support on
for asphalt compaction, it is expected that this approach could also be used for other geomaterials.
5.2. Evaluation of proposed underlying support correction method during compaction of crushed rock base
Boundary element modelling (BEM) of a Sakai SV 510D roller was performed by Mooney and Facas (Citation2013) to investigate the influence of the modulus of the underlying support on the stiffness reported by the IC roller, as shown in .
Figure 20. Variation of roller-reported stiffness (k) with base layer (crushed rock) modulus (E1) and underlying support (silty sand) modulus (E2) [adapted from Mooney and Facas (Citation2013)].
![Figure 20. Variation of roller-reported stiffness (k) with base layer (crushed rock) modulus (E1) and underlying support (silty sand) modulus (E2) [adapted from Mooney and Facas (Citation2013)].](/cms/asset/880ee5ac-f05b-4fd7-abc8-502cd4a4550f/gpav_a_2198770_f0020_oc.jpg)
The applicability of the proposed underlying support correction method during the compaction of a crushed rock layer (placed and compacted on silty sand) was evaluated using the data extracted from . As Mooney and Facas (Citation2013) studied only the stiffness reported by the roller and the interest of the current study is , the corresponding
values for the extracted stiffness values were calculated using Equations 4 and 5, as shown in . The parameters used for the calculations are listed in .
Table 5. Parameters used to calculate .
Table 6. Corresponding values for extracted
values.
The value of the shape-dependent constant () was found to be 0.737 by minimising the mean square error using the extracted data (the 3-D numerical simulation described in Appendix 3 explains that
= 0.737 is applicable to any cylindrical roller drum, irrespective of its diameter). A correlation coefficient (R2) of 0.99 was achieved for the correlation between the elastic modulus of the crushed rock base obtained from the simulation by Mooney and Facas (Citation2013) and the elastic modulus was calculated using the proposed underlying support correction method (i.e.
in Equation 9), as shown in .
It should be noted that the thickness of the layer being compacted is required to employ the proposed method during compaction. However, it is difficult to accurately measure and update the layer thickness in real-time during each roller pass. Although the layer thickness can be determined using the elevation data provided by GPS, ± 10% error in the thickness estimation has been reported (Wanninger et al. Citation2022). A sensitivity analysis was undertaken to access the effect of the uncertainty in the layer thickness measurement on prediction. A given layer thickness (30 cm crushed rock) was multiplied by a factor (0.9 and 1.1) and the prediction using the factored layer thickness was compared with the prediction using the actual layer thickness. The results of this sensitivity analysis are given in .
demonstrates that ± 10% error in the layer thickness does not cause a notable error (on average) in the predicted . It can be argued that the uncertainty in the layer thickness measurement does not significantly affect the modulus prediction using the proposed method (i.e.
in Equation 9).
5.3. Influence of asphalt layer thickness on 
and 

In current pavement construction practice, asphalt pavements are compacted in lifts and the lift thickness can range from 20 mm to 200 mm. Therefore, the influence of asphalt layer thickness on and
was investigated in this study by 3-D numerical simulation using ABAQUS CAE (Dassault Systems Citation2020), as described in Appendix 4. shows the variations of
and
with
for different asphalt layer thicknesses, and the modulus of the top layer was kept at 100 MPa during the analysis.
Figure 23. Influence of asphalt layer thickness on: (a) (before underlying support correction) and (b) on
(after underlying support correction).

According to , both and
are sensitive to asphalt layer thickness. However, for a given asphalt layer thickness,
shows a consistent value, irrespective of the modulus of the underlying support (
). As asphalt layers are placed and compacted in lifts on a lot basis [defined as a pavement section constructed in a day using single lift with homogeneous material (Austroads Citation2009)], the thickness of the asphalt layer is constant for a given lift and lot. Therefore,
is an excellent index for the modulus of the asphalt layer in a given lift and lot.
5.4. Application of the proposed correction method for underlying support during asphalt compaction
As is an indenter shape-dependent constant (King Citation1987), the value of
is the same for a selected shape of the roller drum, irrespective of the material being compacted. Therefore, the value of
, which was found to be 0.737 in Section 5.2, is applicable to asphalt compaction since the same roller drum shape is used. Using this
value,
(Equation 9) was calculated for each roller pass for the field experiment carried out in the present study. shows the correlations of the calculated
(after underlying support correction) and
(before underlying support correction) with asphalt density measured in each roller pass using NNDG.
Figure 24. Correlation of asphalt density (measured using NNDG) with: (a) (before underlying support correction) and (b)
(after underlying support correction), and (c) schematic illustration of the underlying support correction.

is almost constant irrespective of the asphalt density variation in asphalt layer#1 and it shows a negative correlation with asphalt density for asphalt layer#2 [ (a)]. After decoupling the effect of underlying support,
shows an increasing trend with asphalt density for both asphalt layers and the correlation coefficient (R2) was improved from 0.01–0.2 for asphalt layer#1 and from 0.2–0.4 for asphalt layer#2, respectively. Further,
(∼155 MPa for asphalt layer#1 and ∼147 MPa for asphalt layer#2) is less than
(∼230 MPa for asphalt layer#1 and ∼220 MPa for asphalt layer#2) for both asphalt layers. This is because the asphalt modulus is less than the underlying support modulus at asphalt compaction temperatures.
An acceptable correlation between asphalt density and was not achieved, even after decoupling the effect of underlying support on
. It should be noted that during the compaction of asphalt layer#2, the asphalt density changed from 1,900 kg/m3 to 2,400 kg/m3, while
changed only from 140 MPa to 170 MPa. This indicates that the change in asphalt density during compaction does not considerably influence
. This is primarily due to the viscous fluid behaviour of the bitumen at higher temperatures (the temperature of asphalt is maintained above 80°C during compaction as per the quality control requirements) and the modulus of the asphalt does not appear to change notably while the density of the asphalt increases during compaction.
6. Conclusion
A small-scale asphalt testbed experiment was undertaken in this study to determine different ICMVs using the same accelerometer data and their correlation with various spot test measurements. While OEM IC rollers have a separate sensor to measure the phase lag between the excitation force and the ground reaction force, a novel method, using the cross-correlation technique, is used in this study to estimate this phase lag without the need of a sensor. Further, a practical method to decouple the effect of underlying support on is presented and analysed in this study. The key findings of this study are summarised below, along with the main recommendations.
NNDG and NDG – BS measurements performed at the end of asphalt compaction were closer to the core density than the NDG – DT measurements. Therefore, either the NNDG or the NDG – BS method can be used to estimate asphalt density non-destructively. Further, the NDG – DT method is not recommended for measuring asphalt density during compaction, as this causes unnecessary delay in the compaction progress.
and
are well correlated for a given roller due to the nature of the equations. This perfect correlation may not be observed if the IC data obtained from multiple rollers with different dimensions are pooled for analysis. Further,
and
were correlated only when double jump did not occur. In the case of a double jump,
will drop. None of the other combinations of ICMVs (such as
–
,
–
,
–
, and
–
) showed an acceptable correlation.
None of the ICMVs calculated using the accelerometer data obtained during the last pass of the roller demonstrated an acceptable correlation with asphalt density measured in the laboratory using extracted cores. This is mainly due to the influences of the underlying support and asphalt temperature on ICMVs collected during each roller pass.
A practical method to decouple the effect of underlying support on
has been developed and validated in this study. This method can be employed in real-time during compaction to determine an index for the modulus of the material layer being compacted.
The ICMVs which were corrected for the effect of the underlying support using the correction method proposed in this study did not show an acceptable correlation with asphalt density measured during asphalt layer compaction owing to the viscous fluid behaviour of the bitumen at compaction temperatures, where the asphalt modulus is less sensitive to the change in asphalt density at high temperatures.
Acknowledgements
This research work is part of a research project (Project number: IH18.05.1) sponsored by the SPARC Hub (https://sparchub.org.au) in the Department of Civil Engineering, Monash University, funded by the Australian Research Council (ARC) Industrial Transformation Research Hub (ITRH) Scheme (Project ID: IH180100010). This research work is also financially supported by the Postgraduate Publications Award granted by the Monash University, Australia. The financial and in-kind support of the Australian Flexible Pavement Association (AfPA), Department of Transport (DoT), Victoria, and Monash University is gratefully acknowledged. The financial support of ARC is also gratefully acknowledged. The in-kind support of Motorway Technologies Pty Ltd (which provided a non-nuclear density gauge), SITECH Construction Systems Pty Ltd (which provided an IC retrofit kit), Trimble Inc. (which provided IC-related technical advice), and the Australian Road Research Board (ARRB), which provided the site is greatly appreciated.
Disclosure statement
No potential conflict of interest was reported by the author(s).
Additional information
Funding
References
- Anderegg, R., and Kaufmann, K, 2004. Intelligent compaction with vibratory rollers: feedback control systems in automatic compaction and compaction control. Transportation Research Record: Journal of the Transportation Research Board, 1868, 124–134.
- AS 1141.5, 2000. Method for sampling and testing aggregates method 5: Particle density and water absorption of fine aggregate.
- AS 1141.6.2, 1996. Methods for sampling and testing aggregates method 6.2: Particle density and water absorption of coarse aggregate - pychnometer method.
- AS 1141.11.1, 2009. Methods for sampling and testing aggregates method 11.1: Particle size distribution-seiving method.
- AS/NZS 2891.3.3, 2013. Methods of sampling and testing asphalt method 3.3: Binder content and aggregate grading-pressure filter method.
- AS/NZS 2891.7.1, 2015. Methods of sampling and testing asphalt method 7.1: Determination of maximum density of asphalt-water displacement method.
- AS/NZS 2891.8, 2014. Methods of sampling and testing asphalt method 8: Voids and volumetric properties of compacted asphalt mixes.
- ASTM E2583 − 07, 2015. Standard test method for measuring deflections with a light weight deflectometer.
- Australian Asphalt Pavement Association, 2014. Administration of asphalt specifications.
- Austroads, 1999. Air voids in asphalt.
- Austroads, 2009. Guide to pavement technology part 8: Pavement construction Sydney.
- Austroads AG:AM/T006, 2011. Pavement deflection measurement with a falling weight deflectometer (fwd). Austroads.
- Chang, G., et al., 2014. A study on intelligent compaction and in-place asphalt density. Austin: The Transtec Group, Inc.
- Chang, G., et al., 2011. Accelerated implementation of intelligent compaction technology for embankment subgrade soils, aggregate base, and asphalt pavement materials. Austin: The Transtec Group, Inc.
- Chang, G. K., et al., 2018. Leveraging intelligent compaction and thermal profiling technologies to improve asphalt pavement construction quality: A case study. Transportation Research Record: Journal of the Transportation Research Board, 2672 (26), 48–56.
- Chen, Z., et al., 2016. Variation and consistency of young's modulus in steel. Journal of Materials Processing Technology, 227, 227–243.
- Cloete, R., 2015. Elastic properties of soils [online]. Available from: https://support.prokon.com/kb/articles/elastic-properties-of-soils [Accessed May 19 2020].
- Commuri, S., Mai, A. T. and Zaman, M., 2008. Neural network-based intelligent compaction analyzer for estimating compaction quality of hot asphalt mixes. In: Myung Jin Chung and Pradeep Misra, eds. 17th World Congress The International Federation of Automatic Control. Seoul, Korea: Elsevier, 2224–2229.
- Dassault Systems, 2020. Abaqus unified fea.
- Department of infrastructure energy and resources, 2011. Road specifications r55 asphalt placement. Tasmania.
- Fathi, A., et al., 2021. Assessing depth of influence of intelligent compaction rollers by integrating laboratory testing and field measurements. Transportation Geotechnics, 28.
- Foroutan, M., Bijay, K. C., and Ghazanfari, E, 2020. Evaluation of correlations between intelligent compaction measurement values and in situ spot measurements. Geo-Congress, 602–611.
- Gallivan, V., et al., 2011. Intelligent compaction for improving roadway construction. ASCE Geotechnical Special Publication, 218, 117–124.
- Halton public works department, 2021. Specifications for asphalt paving, materials, sampling, and testing.
- Hu, W, 2018. Evaluation of intelligent compaction technology in asphalt pavement construction and laboratory compaction. (PhD). University of Tennessee.
- Hu, W., et al., 2019. Investigating key factors of intelligent compaction for asphalt paving: A comparative case study. Construction and Building Materials, 229.
- King, R. B, 1987. Elastic analysis of some punch problems for a layered medium. International Journal of Solids and Structures, 23 (12), 1657–1664.
- Kostiukov, I, 2020. Estimation of dissipation factor by applying cross-correlation method. In: IEEE KhPI week on advanced technology (KhPIWeek). Kharkiv, Ukraine: IEEE.
- Lai, E, 2003. Converting analog to digital signals and vice versa. Practical digital signal processing for engineers and technicians. Singapore: Elsevier Ltd, 14 −49.
- Ma, Y., et al., 2022. Assessment of intelligent compaction quality evaluation index and uniformity. Journal of transportation engineering, Part B: Pavements, 148 (2).
- Maupin, J. G. W., 2007. Preliminary field investigation of intelligent compaction of hot-mix asphalt.: Virginia Transportation Research Council.
- Mooney, M. A., and Facas, N. W, 2013. Extraction of layer properties from intelligent compaction data. Transportation Research Board The National Academies.
- Narnoli, V. K., and Suman, S. K, 2020. Feasibility of lightweight deflectometer measured surface deflection on flexible pavement for quality assessment. International Journal of Civil Engineering, 7 (7), 73–82.
- National Instruments Corp., 2022. What is labview? [online]. Available from: https://www.ni.com/en-au/shop/labview.html [Accessed January 15 2022].
- Newcomb, D., Timm, D., and Mahoney, J., 2002. It's still dirt, rocks and asphalt - right? [online]. Available from: https://www.asphaltpavement.org/uploads/documents/mechdes3.pdf.
- Oh, J, 2014. A review on intelligent compaction techniques in railroad construction. International Journal of Railway, 7 (3), 80–84.
- Palilla, P. 2014. Density gauge testing. ed. NJ Asphalt Paving Conference, Woodbridge, NJ.
- Pistrol, J., et al., 2017. Theoretical and experimental investigation of continuous compaction control (ccc) systems. Geotechnical structures and infrastructures.
- Polaczyk, P., et al., 2021. Improving asphalt pavement intelligent compaction based on differentiated compaction curves. Construction and Building Materials, 301.
- Ranasinghe, R., Sounthararajah, A., and Kodikara, J, 2022. An intelligent compaction analyser: A versatile platform for real-time recording, monitoring and analysing of road material compaction. Research Square. Available from: https://doi.org/10.21203/rs.3.rs-2371914/v1.
- Reyes, J. M. A., and Forgach, C. E. S, 2016. Evaluation of the minimum size of a window for harmonics signals. Journal of Signal and Information Processing, 07 (4), 175–191.
- Rinehart, R. V., and Mooney, M. A., 2005. Instrumentation of a roller compactor to monitor vibration behavior during earthwork compaction. 22nd International Symposium on Automation and Robotics in Construction. Ferrara (Italy).
- Savan, C. M., Weng, N. K., and Ksaibati, K., 2015. Implementation of intelligent compaction technologies for road constructions in wyoming.: U.S. Department of Transportation.
- Sivagnanasuntharam, S., et al., 2022. Prediction of average in-depth temperature of asphalt pavement using surface temperature measured during intelligent compaction. International Journal of Pavement Engineering.
- Sivagnanasuntharam, S., et al., 2021. A state-of-the-art review of compaction control test methods and intelligent compaction technology for asphalt pavements. Road Materials and Pavement Design, 24 (1), 1–30.
- Timm, D. H., et al., 2001. Calcool: A multi-layer asphalt pavement cooling tool for temperature prediction during construction. International Journal of Pavement Engineering, 2 (3), 169–185.
- Tran, N., Turner, P., and Shambley, J, 2016. Enhanced compaction to improve durability and extend pavement service life: A literature review. National Center for Asphalt Technology.
- U.S. Department of Transportation Federal Highway Administration, 2017. Intelligent compaction measurement value (icmv) a road map.
- Vicroads, 2017. Acceptance of field compaction.
- Wanninger, L., Heßelbarth, A., and Frevert, V, 2022. Garmin gpsmap 66sr: assessment of its gnss observations and centimeter-accurate positioning. Sensors, 22, 1964.
- White, D. J., Vennapusa, P., and Gieselman, H, 2010. Iowa dot intelligent compaction research and implementation - phase i. institute for transportation, earthworks engineering research center, Iowa State University.
- Yasir, S. F., Abbas, H. A., and Jani, J. 2018. Estimation of soil young modulus based on the electrical resistivity imaging (eri) by using regression equation. ed. AIP.
- Yoon, S., Hastak, M., and Lee, J, 2015. Intelligent compaction of asphalt pavement implementation. West Lafayette: Purdue University.
Appendices
Appendix 1
Percentage Difference of NNDG, NDG-BS, and NDG-DT measurements from Core Density
Table A1. Percentage Difference of NNDG, NDG-BS, and NDG-DT measurements from Core Density.
Appendix 2
LWD Data Analysis
Figure A1. shows the variation of LWD load and deflection at spot S1 on asphalt layer#1 at 19°C.
As a result of the viscoelastic behaviour of asphalt, a phase lag was noted between LWD deflection and the exerted load. Therefore, peak load and peak deflection were used in the analysis. Each LWD test was repeated at least five times and only the last three test results were used in the analysis. When the last three readings were not consistent, the test was repeated until three consequent readings were consistent.
Appendix 3
3-D Numerical simulation
The system of roller drum and pavement was simulated using ABAQUS CAE (Dassault Systems Citation2020). By utilising the symmetricity of the system, only a quarter of the system was simulated. As the equations for and
were developed based on the linear elastic theory, linear elastic elements were used in the numerical model. Figure A2 shows the model created in this study.
As shown in Figure A2, the entire pavement was modelled as a single part and the layers were separated using partitions. To justify the applicability of = 0.737 for different roller sizes, the roller drum was modelled with three different radius values (0.5, 0.75, and 1 m). In order to study the influence of asphalt layer thickness, the asphalt layer was modelled with three different thickness values (50, 110, and 175 mm). 21,008.5 N/m3 of body force was applied to the drum (i.e. 33 kN of load for the drum with 0.5 m radius). Figure A3 shows the mesh used in this study.
As shown in Figure A3, a fine element size was used in the drum in the region where it interacts with the pavement. As the roller drum is made of steel, the modulus of steel [210 GPa (Chen et al. Citation2016)] was assigned to the drum. Before modelling the asphalt layer, only the granular base and subgrade were modelled to determine the equivalent modulus of the underlying support (), and the results are summarised in .
Table A2. Equivalent modulus () of underlying support (600 mm thick granular base on top of 1000 mm thick subgrade).
Although the values of the granular base modulus used in the model were up to 750 MPa, reached only 202.2 MPa. This is because the low modulus of the subgrade significantly affects
. As this narrow range of
(∼130 MPa to 200 MPa) is not sufficient to demonstrate the impact of the correction of the underlying support, a wide range of
(250, 500, and 750 MPa) was used in the simulations. Figure A4 shows one of the simulation outcomes.
It should be noted that the lowest modulus values were used in the simulation to obtain the results shown in Figure A4 and the results show that the dimensions of the part used in the model (2 m in X-direction, 2 m in Z-direction, and ∼1.6 m in Y-direction) is sufficient for these modulus values and any other modulus values greater than these modulus values.