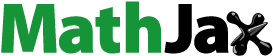
ABSTRACT
In this investigation, the drying of Stevia rebaudiana leaves was carried out in a lab scale convective hot-air dryer at a varying temperature of 30–80°C to analyze the drying behavior, fit mathematical, Artificial Neural Networks (ANNs), and Adaptive Neuro-Fuzzy System (ANFIS) models to predict the drying kinetics of leaves. Further, dried leaf powders were analyzed for color properties, ascorbic acid and total phenol contents, antioxidant activity, water activity (aw), water solubility index (WSI), hygroscopicity (HG), density (bulk, tapped, and particle), bulk porosity, and flowability indices (Hausner ratio (HR), Carr index (CI), and angle of repose (α)). The results showed that ANFIS model with R2 of 0.9998, offers a more accurate forecast of the drying kinetics of leaves dried in a convective hot-air dryer in comparison to mathematical and ANN modeling. The convective drying significantly (p < .05) effected the L*, a*, b*, hue angle and chroma values of dried leaves. Increase in the drying temperature from 30 to 80°C resulted in a decrement of 50.90% in aw, 10.10% in tapped density, while enhancement of 23.26% in WSI, 32.93% in HG, 54% in particle density, and 10.59% in bulk porosity of dried leaf powder. Notably, ascorbic acid and antioxidant activity decreased with rising temperatures, while total phenols enhanced up to 50°C. The bulk density of dried samples remained largely unchanged with increasing temperature, while the flowability of the Stevia powder improved. Thus, these findings provide valuable insights for producers regarding the drying characteristics and properties of Stevia leaf powder.
Introduction
Stevia rebaudiana Bertoni, commonly known as candyleaf, sweetleaf, or sugarleaf, is an Asteraceae (Compositae) family member native to Paraguay.[Citation1] Stevia leaves growing recognition as an appealing alternative for synthetic sweeteners due to their outstanding sweetness level, which exceeds sucrose by a factor of 300, in addition to the benefits of being calorie-free, carbohydrate-free, and free of the risk of causing sudden spikes in blood sugar levels.[Citation2] Stevia leaves are usually ingested in a variety of forms, including fresh or dried and crushed leaves, as well as by extracting its sweet components. Commercially, sweetening agents are manufactured from dried Stevia leaves that range in moisture content from 10% to 13%.[Citation3]
Herbs like Stevia leaves require drying quickly after being collected from the farm in order to avoid microbial activity and retain their natural contents.[Citation4] Furthermore, moisture decrease during drying results in reduced volume, leading to savings in packaging, storage space, and transportation costs.[Citation5] Using natural sunlight to dry agricultural products is a common practice in many tropical countries.[Citation6] However, there are limitations to sun drying in terms of the product’s quality, climate reliance, time, sun exposure, and contamination.[Citation7] As a result, convective hot air drying has evolved as a popular method for drying a wide range of agricultural goods in field, industrial and commercial settings. This choice is due to its ease of use, low cost, and ability to provide consistent and efficient drying rates across a wide temperature range.[Citation8] Convective hot air drying, by extending shelf life, proves to be beneficial in the development of processed foods.[Citation9]
Mathematical modeling is critical in the subject of drying technology, particularly for understanding and forecasting drying processes. Thin-layer equations have been frequently used to explore the drying behavior of diverse goods and to generate generalized drying curves.[Citation10] In the food industry, formulating the drying characteristics of foodstuffs is highly advantageous as it aids in enhancing the design and control of the drying process.[Citation11] While mathematical modeling is a beneficial tool for analyzing material drying processes, choosing the best appropriate complete mathematical model may be challenging and lengthy, especially when numerous factors impact the process of drying.[Citation1] Intelligent modeling tools like Artificial Neural Networks (ANN) and Adaptive Network-based Fuzzy Inference System (ANFIS) have proven to be effective in accurately predicting the drying process in various industrial applications. Recent studies have utilized ANN and ANFIS for convective hot air drying of quince,[Citation12] orange-fleshed sweet potato,[Citation13] garlic and cantaloupe,[Citation14] showcasing the potential of these approaches.
According to the review of literature, it was observed that there was a notable gap in the available knowledge pertaining to the comparative analysis of ANFIS, ANN, and mathematical modeling when applied to the thin-layer drying process of Stevia rebaudiana leaves utilizing a convective hot air dryer.[Citation15–17] Considering the above gap, this paper introduces an innovative research endeavor with a primary focus on intelligent modeling through Artificial Neural Networks (ANNs) and Adaptive Neuro-Fuzzy System (ANFIS) models. Further, this research also encompasses the evaluation of various properties, including color attributes, biochemical properties such as ascorbic acid and total phenol contents, antioxidant activity, and powder characteristics such as water activity (aw), water solubility index (WSI), hygroscopicity (HG), density (bulk, tapped, and particle), bulk porosity, Hausner ratio (HR), Carr index (CI), and angle of repose (α) pertaining to the dried Stevia leaf powder.
Material and methods
Raw material
The Stevia leaf samples used in this study were sourced from a local nursery located in Ludhiana, Punjab, India (30° 56” 15.936”‘N, 75° 44’ 48.2676”” E). The leaves were carefully separated from their stems and selected based on visual criteria, including their harvest date, color, size, and freshness, to ensure a consistent and uniform collection for the study. The methodology stated by AOAC[Citation18] was adopted for estimating the initial moisture content of new leaves.
Procedure of drying
The impact of hot air exposure on Stevia leaves was investigated using a lab-scale 440 V convective dryer (Kilburn laboratory, India). This dryer, with dimensions of 1370 mm × 940 mm × 430 mm, housed stainless steel trays measuring 817 × 410 mm. A 0.25 hp blower fan was situated on the right side, while ventilation holes for inlet and exhaust air were placed on the left and top sides, respectively. The drying system also featured a microcontroller-based temperature controller allowing precise temperature regulation. The desired temperature was set using a digital indicator cum controller, adjusting the power supplied to the heating coil on the left side of the drying chamber. shows the schematic diagram of the convective hot-air dryer. Prior to initiating the drying experiment, the dryer operated without a load for approximately 30 min to attain the desired experimental conditions. A single thin layer of 1200 g of Stevia leaves was spread on a tray and dried at air temperature ranging from 30 to 80°C, maintaining a constant air velocity of 1 m/s and an average relative humidity of 16 ± 2%. Periodic weight measurements of the leaf-laden tray were taken at 10 min intervals throughout the drying process using a weighing scale (DS-252, Essae, India) with each weighing process taking less than 10 s. Drying was continued until a consistent weight loss was achieved. To ensure reliability and accuracy, all experiments were conducted in triplicate.
Drying kinetics and mathematical modeling
The mass obtained for all the samples was used to calculate the moisture loss using equation 1, and the obtained moisture content was converted to moisture ratio (MR) and drying rate (DR) using Equationequation 2(2)
(2) and Equation3
(3)
(3) , respectively.[Citation19]
where is moisture content (g water. g dry matter−1) at time t,
is mass (g) at any time t and
is the mass of the absolutely dried Stevia leaves (g), MR and DR are the moisture ratio (dimensionless) and drying rate (g water/g dry matter·min),
,
, and
are initial moisture content (g water. g dry matter−1), equilibrium moisture content (g water. g dry matter−1) and moisture content (g water. g dry matter−1) at time
, respectively,
is the increment in drying time (min)
The experimental data of moisture ratio (MR) versus drying time were fitted using five commonly used mathematical models to identify the most suitable models for predicting the drying kinetics of Stevia leaves, as presented in . The coefficient of determination (R2), chi-square (χ2), and root mean square error (RMSE) values were utilized as primary criteria for selecting the best equation that accurately describes the experimental data.[Citation2]
Table 1. The five Mathematical models for drying curves.
Artificial neural network (ANN) modeling
In this study, the MATLAB software (R2018a, MathWorks, USA) was utilized to develop and evaluate various Artificial Neural Network (ANN) models. The most well-known ANNs, multi-layer feed-forward with back-propagation learning (FFBP) and Levenberg – Marquardt (LM) training techniques were chosen for this study. These models were designed to predict the moisture ratio during the convective hot air drying process of Stevia leaves. The network design in this study consists of two neurons in the input layer, one neuron in the output layer, and a variable number of neurons in the hidden layer. Too many neurons in the hidden layer can prolong training time and lead to overfitting, while too few can cause underfitting. The ideal neuron configuration is determined through trial and error, selecting the setup with the highest correlation between desired and actual outputs.[Citation22] The number of neurons in the hidden layer varied from 1 to 20, allowing for different configurations and testing of the ANN models. The input layer of ANNs contains two variables: air temperature and drying time, while the MR is the only variable in output layer (). The appropriate number of neurons in the hidden layer is difficult to establish, and it typically relies on the kind and complexity of the job. As a result, it is decided by trial and error.
Before being divided into subgroups for training, validation, and testing, the experimental parameters were initially shuffled. In this process, 70% of the data was used for both predicting the gradient and training the network weights and biases, while 15% was dedicated to network assessment and the remaining 15% was reserved for testing purposes.[Citation2] Throughout the training process, ANNs learn the relationships between neurons with the aid of training data, adjusting these predictions in each training epoch to approach desired outcomes and enhance the model’s efficiency. The determination of composite variables such as the number of hidden layers, neurons, and training epochs was achieved through trial and error.[Citation22] In evaluating the ANN model, the prediction of the proposed parameter (MR) was conducted utilizing two threshold functions: sigmoid activation function (Logsig) and hyperbolic tangent activation function (Tansig) as reported by Abbaspour‐Gilandeh et al.[Citation12] The model’s performance was assessed using three key statistical criteria: chi-square (χ2), root mean square error (RMSE), and coefficient of determination (R2). These metrics were utilized to gauge the accuracy and predictive capacity of the ANN model.
Adaptive neuro-fuzzy inference system (ANFIS) modeling
ANFIS is a hybrid method that combines neural networks and fuzzy inference systems (FIS), enabling the modeling and analysis of input–output relationships based on a provided dataset.[Citation23] In ANFIS, the main problem is determining the type of membership function and their degree of membership, for which there is no specific solution, and by using trial and error, the best structure of the model is determined. ANFIS can be potently employed to find the optimum relationship between a set of input and output data effectively. Fuzzy rules can be generated from the given input-output data by implementing the Takagi – Sugeno fuzzy inference system, thus this system is always interpretable in terms of fuzzy if-then rules in contrast with ANNs where the knowledge gained by training is split in every network weight and is not possible to be interpreted by humans.[Citation22] In the ANFIS modeling process for predicting the moisture ratio (MR), a variety of ANFIS structures were generated using input variables (drying temperature and time) and target data (MR), same as those used in the ANN models. The ANFIS modeling process involved randomly dividing the data into two groups: 70% for training and 30% for model evaluation. The ANFIS toolbox of MATLAB software (R2018a, MathWorks, USA) was utilized for modeling. Different ANFIS structures were generated using the grid partitioning technique. Parameters such as the number of membership functions (MFs) in each input (two or three), the type of input MFs (Gaussian, Sigmoid, or Triangular), the optimization method (Backpropagation or Hybrid), and the type of output MF (Constant and Linear) were altered to create diverse ANFIS structures. provides a visual representation of the ANFIS structure. In this study, criteria such as the coefficient of determination (correlation) (R2), root mean square error (RMSE), and chi-square (χ2) were used to measure the quality of network performance.
Color properties
A colorimeter (Konica Minolta CR-10 color reader, Japan) was used to carry out colorimetric CIE (L*, a*, and b*) measurements of the dried Stevia leaves. The L* values of a color describe its degree of whiteness (100) or blackness (0). The a* values vary from redness (+a) to greenness (−a), while the b* values range from yellowness (+b) to blueness (−b). These values were converted into hue angle, chroma, and browning index,[Citation20,Citation24] using following Equationequations 4(4)
(4) , Equation5
(5)
(5) , Equation6
(6)
(6) , Equation7
(7)
(7) , Equation8
(8)
(8) and Equation9
(9)
(9) .
Ascorbic acid, total phenols, and antioxidant activity
The ascorbic acid content in the Stevia leaves samples was determined using the 2,6-dichloroindophenol titration method as described by Kalsi et al.[Citation2] To prepare the samples, 1 g of Stevia leaves was finely ground using a mortar and pestle and then mixed with 3% metaphosphoric acid. The mixture was subsequently filtered through Whatman No. 1 filter paper. The ascorbic acid solution obtained was titrated against a dye solution containing 2,6-dichloroindophenol, with phenolphthalein serving as the indicator. The titration continued until a faint pink color appeared and persisted for at least 15 s. The results were expressed in mg/100 gm of each stevia leaves sample.
The determination of the total phenolic content (TPC) was carried out spectrophotometrically using a UV-2601 UV/VIS Double Beam spectrophotometer (Rayleigh, China) at a wavelength of 765 nm. The Folin–Ciocalteu method, as described by Zalpouri et al.,[Citation25] was employed for this purpose. To calculate the TPC of the leaf samples, a linear equation was established based on a calibration curve generated using gallic acid as the standard. Gallic acid solutions with concentrations ranging from 5 to 25 µg/ml were used to create the standard curve. The TPC of the Stevia leaves was expressed in terms of gallic acid equivalents per gram (mg GAE/g) of dried leaves.
The antioxidant activity (AA) of the samples was assessed using the 2,2-diphenyl-1-picrylhydrazyl (DPPH) assay, following the procedure outlined by Mouhoubi et al.[Citation21] This assay measures the ability of the samples to donate protons, resulting in a decrease in absorbance, which was measured at a wavelength of 517 nm using a UV-2601 UV/VIS Double Beam spectrophotometer (Rayleigh, China). The inhibition percentage was then calculated using the following formula:
where, A0 and As stands for the absorbance of control (methanol) and sample, respectively.
Water activity, water solubility index (WSI), and hygroscopicity (HG)
Water Activity (aw) of dried stevia powder was measured using a water activity meter (Pawkit, Decagon Devices Inc., USA) at temperature 25.5 ± 1°C. Water solubility index (WSI) was determined according to the method described by Xu et al.[Citation26] with some modification. The procedure involves mixing 0.2 g of superfine powder with 10 mL of distilled water. The mixture was then placed in an 80°C water bath for 30 min. The mixture was then centrifuged for 10 min at 3000 rpm. The resultant supernatant was carefully transferred to a pre-weighed petri dish and dried overnight at 105°C. The Water Solubility Index (WSI) might be calculated using EquationEquation 11(11)
(11)
where Ws is eight of the dried supernatant at 105°C, Wos is weight of the original sample.
Hygroscopicity (HG), the ability of a powder to absorb moisture from a high relative humidity environment, was measured for Stevia leaf powders using the method published by Zalpouri et al.[Citation25] In this experiment, 1 g of Stevia leaf powder was placed inside a desiccator, which was maintained at room temperature and 75% relative humidity. The relative humidity was achieved by utilizing a saturated aqueous NaCl solution. The powders were weighed after 7 days, and the hygroscopicity was represented as HG (%) or grams of absorbed moisture per 100 g of dry solid. The following Equationequation 12(12)
(12) is used to calculate the hygroscopicity:
where Δm represents the weight increase of the powder (g), and w denotes the initial weight of the powder (g).
Bulk density and tapped density
The bulk and tapped density of dried Stevia leaf powder from various drying techniques were measured using the procedure given by Bakshi and Ananthanarayan.[Citation27] To calculate the bulk density, a known mass of material was poured freely into a measuring cylinder under the influence of gravity. On the other hand, the tapped density was determined by suspending the Stevia leaf powder in a 100 mL measuring cylinder and tapping it until a consistent volume was achieved.
Particle density and bulk porosity
The particle (true) density of dried Stevia leaf powder was determined using the liquid displacement method (Padhi & Dwivedi).[Citation28] The process involved measuring the mass of the Stevia leaf powder and then pouring it into a measuring cylinder filled with toluene. The volume of toluene displaced by the powder was recorded, and the particle density is calculated using Equationequation 15(15)
(15) below
The porosity of the sample was calculated using the relationship between the bulk density and true density of the powder, as described by Camacho et al.[Citation29]
where ρB and ρT is bulk and tapped density (g/cm3).
Flowability indexes
The flowability indexes like angle of repose cohesiveness and compressibility of the dried Stevia leaf powder were evaluated using method mentioned by Camacho et al.[Citation29] The powders’ cohesiveness and compressibility were assessed using the Hausner ratio (HR) and Carr index (CI), respectively. The CI and HR values were calculated based on the bulk and tapped density measurements using equations 17 and 18, respectively. To measure the angle of repose, a fixed funnel was employed to pour the powder onto a level surface from a predetermined height, leading to the formation of a conical shape. The angle formed between the side surface of the cone and its base was then measured and documented as the angle of repose, as outlined in Equationequation 19(19)
(19) .
where ρB= Bulk density (g/cm3), ρT=Tapped density (g/cm3), h is the height of the pile, r is the radius at the base of the pile.
Statistical analysis
The non-linear regression analysis was carried out using Origin Pro 8.5 software. The reduced chi-square (χ2), root mean square error (RMSE), and coefficient of determination (R2) were utilized to figure out how effectively the drying curve fits worked. The model that exhibited the highest coefficient of determination (R2) and the lowest root mean square error (RMSE) and chi-square (χ2) values was selected as the most accurate predictor of the moisture ratio.[Citation2] Minitab 19 software was utilized to conduct an analysis of variance (ANOVA) in order to examine the influence of parameters, including drying temperature. The results of the analysis were then used to assess whether there were any significant differences between the various parameters, with a significance level set at p < .05.
Result and discussion
Drying kinetics
Fresh Stevia leaves had an initial moisture content of 80.66% on a wet basis. illustrates the variation in the moisture ratio of Stevia leaves over the drying time during convective hot air drying at different temperatures. Moisture transfer appeared more prominent at the start of the drying stage irrespective of drying temperatures, whereas it was less at the final stage, which might be attributed to plenty of water molecules nearer to the Stevia leaves’ periphery at the start of the drying procedure in a hot air oven dryer.[Citation30] It can be seen that increasing the temperature of air from 30 to 80°C during drying drastically reduced the time period of drying from 530 to 60 min. It took 530, 390, 210, 130, 90 and 60 min for Stevia leaves to achieve their final moisture level (<10% wet basis) at 30, 40, 50, 60, 70, and 80º C, respectively. As the level of temperature of drying air is raised about 2.66 times, the average drying time dropped by about 1/8.83 times. The findings suggest that raising the external temperature leads to an increased rate of moisture transfer from the tissue to the surface of the crop, as well as enhanced evaporation, thereby accelerating the drying process at higher temperatures.[Citation23] Similar observation have been reported in the study on convective drying of bay laurel leaves and noted that drying time reduced from 145 to 75 min as the temperature was increased from 45 to 70°C.[Citation31] Furthermore, in a study by Ben Haj Said et al.[Citation32] on the convective drying of wild edible plant (Allium roseum) leaves, it was noted that elevating the air temperature from 40 to 60°C led to a reduction in the drying time from 28 to 7 h. Similarly, Stępień et al.[Citation33] observed that hiking temperature from 40 to 60°C during convective drying of Pink Rock Rose (Cistus creticus) leaves reduced the drying period by 60%. illustrates the relationship between the drying rate and moisture content at different temperature levels, demonstrating a consistent decrease in drying rate as moisture content decreases. Therefore, a constant drying rate period was not observed in the drying curve of Stevia leaves, indicating that the whole drying take place within the falling rate phase. Furthermore, it was discovered that increasing the temperature of the drying air increased the drying rate. These results align with prior research conducted by Mouhoubi et al.[Citation21] on the convective drying of coriander leaves which indicated that at higher moisture content, the drying rate increased when the temperature was elevated from 40 to 120°C. Similarly, Ali et al.[Citation34] reported that increasing the temperature from 50 to 70°C during convective drying of Rosmarinus officinalis leaves resulted in the escalation of drying rate from 0.0297 to 0.0730 min−1.
Mathematical modeling
Drying data was utilized to describe the convective drying kinetics of Stevia leaves. The experimental data were fitted using nonlinear regression analysis with five frequently used model equations. displays the statistical results for convective drying of Stevia leaves from all models. All models demonstrated a high degree of fit, with R2 values exceeding 0.9461. The analyzed parameters yielded χ2 values ranging from 0.0001 to 0.048 and RMSE values ranging from 0.0044 to 0.0696 for different models. When compared to the statistical parameters obtained for the other investigated models (), the logarithmic model exhibited the best suitability for all experimental data. It achieved a coefficient of determination (R2) greater than or equal to 0.9952, a root mean square error (RMSE) less than or equal to 0.0162, and a chi-square (χ2) value less than or equal to 0.0003. A number of studies have shown that the Logarithmic model is the best fit for describing the drying behavior of a number of agricultural commodities. Kouhila et al.[Citation35] showed that logarithmic model was the best fitting of experimental data with a highest value of correlation coefficient (r), and lowest value of reduced chi-square (χ2) for describing the drying behavior of Mytilus Galloprovincialis dried in a solar dryer operating in forced convection. Similarly, Miraei Ashtiani et al.[Citation36] reported the selection of Logarithmic model as the best model to predict the drying behavior of peppermint leaves during convective drying with R2 varying between 0.99967 and 0.99976. Furthermore, Chen et al.[Citation37] reported logarithmic model as best in forecasting the hot air drying of jujube slices because of lower RMSE values in range of 3.73 × 10−4−0.00235. depicts a comparison of experimental results with a projected logarithmic model. demonstrates that as the temperature of the drying air increased, so did the k value of the Logarithmic model. The drying curve grows steeper as the k value increases, implying quicker drying.[Citation2]
Table 2. Statistical factors of different models fit of moisture ratio for convective hot-air drying of Stevia leaves.
Artificial neural networks (ANN) modeling
During the development of the artificial neural network (ANN), a multi-layer feed-forward topology was employed. Several different topologies were evaluated to identify the most suitable number of hidden neurons for optimal network performance. provides valuable insights into the relationship between the number of hidden neurons and the performance of the artificial neural network. The plots depicting chi-square versus the number of hidden neurons and the coefficient of determination versus the number of hidden neurons demonstrate the impact of varying the number of hidden neurons on the network’s performance. Among the different artificial neural networks evaluated, the most effective network configuration was found to be a three-layered topology with 16 neurons in the hidden layer (2-16-1) and the logarithm sigmoid transfer function.
Figure 5. ANN Performance evaluation based on number of hidden neurons for convective hot-air drying of Stevia leaves.

depicts the best ANN model predictions for the samples’ moisture ratio fluctuation during the drying phase. The close alignment of the data points to a straight line with a slope of one indicates the excellent prediction accuracy of the created ANN technique. The ANN-predicted correlation coefficient (R) for the training, validation, and testing stages was found to be 0.99997, 0.99987, and 0.99978, respectively. These results demonstrate that the ANN model can effectively predict the drying kinetics of Stevia leaves when utilizing a convective hot air dryer. Furthermore, the best ANN model achieved high values of R2 (0.9997), low values of Chi-square (0.00007), and low values of RMSE (0.0042), surpassing the performance of the mathematical models. Similar findings regarding the superior MR prediction accuracy of ANNs compared to mathematical models have been reported in prior studies. Kalsi et al.[Citation2] found out that the most favorable outcomes were obtained by using one hidden layer with 15 neurons for MR prediction in the context of microwave drying of stevia leaves. Similarly, Bakhshipour et al.[Citation1] utilized a neural network with two hidden layers following a 4–17–19–1 topology, which proved to be the most accurate artificial neural network (ANN) for monitoring the moisture ratio of stevia leaves during the process of infrared-assisted continuous hybrid solar drying. It is worth emphasizing that the ANN approach simplifies the construction of a prediction model for the Stevia leaf drying process.
ANFIS modeling
Multiple adjustments were performed to different parameters in order to optimize the ANFIS model for predicting moisture ratio in dried Stevia leaves. This includes experimenting with several input membership functions (Gaussian, Sigmoid, or Triangular) and input number (ranging from 2 to 3). In addition, the output membership functions, which comprised linear and constant functions, were modified. The training algorithm techniques were also improved, with both hybrid and back-propagation approaches applied.
displays the different accurate ANFIS structures used, specifying the number of membership functions for each input, the number of rules, and the types of input and output membership functions employed. The values of R2, chi-square and RMSE indices related to the best network structure for predicting the variation in moisture ratio during drying were 0.9998, 0.00006, and 0.0040, respectively.
Table 3. Structure and performance criteria of the most reliable ANFIS models for predicting MR fluctuations during convective drying of stevia leaves.
The best ANFIS models for predicting the moisture ratio were determined to have the following characteristics: Gaussmf as the type of input membership function, Linear as the type of output membership function, Hybrid as the type of learning algorithm, and three membership functions for each input (3-3-3). The correlation coefficient (R) between the desired output values obtained in the laboratory and the predicted values from this study is depicted in . The high correlation coefficient of 0.9999 demonstrates the excellent agreement between the experimental data and the model outputs, indicating the model’s accuracy and its potential application in industrial operations.
Comparison between mathematical, ANN, and ANFIS modeling
A comparison of statistical characteristics across mathematical, ANN, and ANFIS models indicates that the ANFIS model predicts the MR parameter more accurately during convective drying of Stevia leaves. Based on the data in , , and , it is clear that the R2 value for the anticipated moisture ratio (MR) in the ANFIS model outperformed both the best mathematical model and the ANN models. Furthermore, the ANFIS value in all predicted parameters (chi-square and RMSE) was lower than the both best mathematical and artificial neural networks model. Similar result for ANFIS modeling showing better modeling ability in comparison to ANNs and mathematical models have been reported in a number of investigations. In a research conducted by Abbaspour‐Gilandeh et al.,[Citation12] the utilization of ANFIS modeling employing gaussmf membership function resulted in the most accurate predictions of moisture ratio (MR) during the hot air drying of quince. This approach exhibited the highest R2 value (0.9997) in comparison to mathematical (0.9992) and ANN (0.9993) modeling methods. Similarly, Kaveh et al.[Citation38] discovered that for predicting MR in the context of convective drying of almond kernels, ANFIS modeling achieved the most favorable results with an R2 value of 0.9998. In contrast, the best models from mathematical and ANN approaches exhibited R2 values of 0.9994 and 0.9995, respectively.
Color properties
shows the color characteristics for Stevia leaves dried at various temperatures. The convective drying significantly (p < .05) reduce the values of L*, b*, hue angle and chroma of Stevia leaves in comparison to fresh leaves except for value a* which increased. The L*, a*, b*, hue angle and chroma ranged from 26.59 to 41.30, −11.1 to −6.94, 13.97 to 19.55, 113.35 to 119.60, and 15.63 to 22.48, respectively. The value of L* increased with increasing temperature from 30 to 60°C and then decreased from 70 to 80°C. The drop in L* value at higher drying temperatures might be related to non-enzymatic browning and the production of brown pigment.[Citation39] Lule & Koyuncu[Citation40] reported similar increase and decrease in value of L* when nettle leaves were dried from temperature of 50 to 70°C during convective drying. Opposite trend was shown by value a* which increased from −11.1 ± 0.17 to −8.22 ± 0.03 when temperature was increased from 30 to 50°C and decreased from −6.02 ± 0.12 to −6.94 ± 0.09 when temperature was increased from 60 to 80°C. Ek et al.[Citation41] reported similar fluctuation of a* values during the convective drying of watercress leaves when temperature was hiked from 40 to 70°C. The convective drying of Stevia leaves at temperature of 30–60°C enhanced the value of b* and followed a decrement at high temperature of 70 and 80°C. Youssef and Mokhtar et al.[Citation42] reported similar decreasing trend of b* value from 9.54 to 8.12 followed by increase to a value of 9.39 when Portulaca oleracea L. leaves were subject to convective drying and temperature was increased from 40 to 80°C. This reduction in value of b* might be attributed to degradation of carotenoid pigments.[Citation43] The hue angle is considered a qualitative aspect of color, where specific angles like 0, 90, 180, and 270° correspond to the perception of red, yellow-green, and blue hues, respectively, while chroma is seen as a quantitative attribute, representing the degree of colorfulness in a given color.[Citation44] The hue angle of Stevia leaves decreased after connective drying ranging from 111.39 ± 1.09 to 116.61 ± 0.64. Thus, drying enables sample hues to gradually change to the yellow side than the natural one. The highest chroma value (20.03) was noted for Stevia leaves dried at 40°C. These color changes of the product during drying may be attributed to degradation of chlorophylls and other pigments, changes in carotenoid contents, or enzymatic/non-enzymatic reactions.[Citation45]The browning index increased for all drying temperatures in comparison to fresh leaves and varied within 37.78–47.51. Similar increment of browning index after convective drying has been reported for coriander leaves.[Citation46] The inactivation of polyphenol oxidase and Maillard reactions may be the cause of the increase in the browning index.[Citation8]
Table 4. Variation of color characteristics for the convective hot-air drying of Stevia leaves.
Ascorbic acid, total phenols, and antioxidant activity
The variation in the content of ascorbic acid during convective drying is shown in . The findings of this study indicate a statistically significant (p < .05) reduction in ascorbic acid content in dried Stevia leaves across all drying temperatures when compared to the initial ascorbic acid content in fresh Stevia leaves. The ascorbic acid concentration in dehydrated Stevia leaves decreased from 25.30 ± 0.84 mg/100 g in the fresh leaves to 22.50 ± 0.65, 21.25 ± 0.33, 19.73 ± 0.66, 17.24 ± 0.66, 14.26 ± 0.26, and 11.45 ± 0.28 mg/100 g in the leaves dried at temperatures of 30, 40, 60, 70, and 80°C, respectively. This represents a reduction to approximately 7.5%, 11.07%, 21.9%, 31.9%, 43.6%, and 54.8% of the initial ascorbic acid content for the respective drying temperatures. Hence, the content of ascorbic acid in the dried Stevia leaves decreased with an increase in drying temperature. Similarly, Ek et al.[Citation41] reported that temperature rise from 40 to 70°C resulted in the reduction of ascorbic acid from 344.7.4 to 5.4 mg/100 g d.m. during convective drying of watercress leaves. The degradation of vitamin C during the drying process can be attributed to irreversible oxidative reactions, high-temperature sensitivity, and leaching of this water-soluble vitamin.[Citation47]
Table 5. Variation in biochemical parameters of Stevia leaves dried using convective hot-air drying.
presents the total phenolic content of Stevia leaves dried at various temperatures during convective drying. Total phenolic content increased from 52.51 ± 1.67 to 53.62 ± 0.61 mg GAE/g when the temperature was increased from 30 to 50°C. The observed increase in total phenolic content may be attributed to the formation of phenolic compounds, which is often associated with the availability of precursors of phenolic molecules arising from non-enzymatic inter-conversion between the phenolic molecules.[Citation48] With further increase of temperature from 60 to 70°C, the content of total phenols reduced from 43.46 ± 1.65 to 32.58 ± 0.70 mg GAE/g. This decrease in total phenolic content can be attributed to the binding of polyphenols with other compounds (proteins) or to alterations in the chemical structure of polyphenols, which may render them difficult to extract or determine using available methods.[Citation49] These results align with prior research conducted by Khodja et al.[Citation50] on the convective drying of Laurus nobilis leaves who reported increase of total phenolic content with rise of temperature from 40 to 60°C and further increase in temperature resulted in depletion of total phenolic content.
presents the variations in antioxidant capacity of the hot air-dried Stevia leaves achieved with different levels of temperature. The antioxidant capacity in DPPH reduced by 9.75, 19.31, 36.87, 42.20, 48.12 and 51.69% at 30, 40, 50, 60, 70 and 80°C, respectively. The highest decrease was observed at 80°C. Previous studies have reported that when materials undergo drying and come into contact with hot air, it can lead to the degradation of essential flavors and bioactive compounds.[Citation47] For example, Stępień et al.[Citation33] showed that a hike in the temperature from 40 to 60°C during hot air drying of pink rock rose (Cistus creticus) leaves resulted in a reduction of antioxidant capacity by 10.05%.
Water activity, water solubility index, and hygroscopicity
Water activities (aw) of all the dried samples are shown in . With the rise in drying air temperature from 30 to 80°C, aw values of the dried Stevia leaf powders were reduced significantly (p > .05) from 0.55 to 0.27 which is a 50.90% decrease. A water activity (aw) value of less than 0.6 is typically recognized as a safe threshold for long-term food preservation without the risk of microbial development.[Citation51] As a result, the water activity (aw) values of Stevia leaf powder produced from convective drying were discovered to be below the safe storage level. The aw values decreased with the increase in drying temperature due to enhanced water vapor migration facilitated by higher temperatures.[Citation41] Chua et al.[Citation52] reported decrement of aw from 0.2252 to 0.1081 when the temperature was elevated from 40 to 60°C during hot air drying of Phyla nodiflora leaves.
Table 6. The influence of drying air temperature on powder properties of dried stevia leaf powder.
Water solubility index (WSI) is an important physical property used to assess the ability of a powder to dissolve in water and release-soluble components, resulting in the formation of a homogeneous and stable solution.[Citation53] As demonstrated in , the WSI values of Stevia leaves powders varied from 44.70 ± 0.29 to 55.10 ± 0.59%. In the current study, it was observed that the WSI of the Stevia leaves powder exhibited a noteworthy increase of 23.26% when the drying temperature was raised from 30 to 80°C. The enhancement in solubility with an increase in temperature is likely attributed to the concurrent increase in porosity and the uniformity of pores in the dried samples.[Citation54] González-Jiménez et al.[Citation55] showed that when the air temperature was hiked from 70 to 110°C during drying of Agave angustifolia Haw leaf, the WSI of dried powders increased from 27.64 to 33.36%.
The hygroscopicity (HG) of hot air dried Stevia leaves is shown in . The hygroscopicity of dried Stevia leaf powders exhibited variation ranging from 5.86 ± 0.17 to 7.79 ± 0.20%, depending on the drying temperature employed. The hygroscopicity of powders in increasing order was as follows: 30°C < 40°C < 50°C < 60°C < 70°C < 80°C. The rise in temperature causes a 32.93% enhancement of HG of dried Stevia leaf powder. In the powder classification of hygroscopicity at 75% RH, dried Stevia leaf powders were considered non-hygroscopic powder as the range in present study is below 10%.[Citation56] The hygroscopicity of powders decreased with increase in temperature. González-Jiménez et al.[Citation55] showed that increasing the air temperature from 70 to 90°C during drying of Agave rhodacantha Trel leaf, the hygroscopicity of dried powders increased from 7.60 to 7.76%.
Density (bulk, tapped, and particle) density, and bulk porosity
presents the measurements of the dried Stevia leaf powder’s bulk and tapped density. No significant (p > .05) change in bulk density of dried sample takes place when temperature was raised. This indicates that the bulk density of the sample is not affected by temperature changes, which is also shown by Aprajeeta et al..[Citation57] The dried Stevia leaf powder’s bulk and tapped density exhibited variations within the range of 0.251–0.262 g/cm3 and 0.267–0.297 g/cm3, respectively. A decreasing trend in values of bulk (4.19% decreases) and tapped (10.10% decrease) density was seen as the temperature of drying increased from 30 to 80°C. The decrease in density of the dried powder is attributed to the rapid removal of moisture at higher drying temperatures.[Citation58] Similarly, González-Jiménez et al.[Citation59] showed that when the air temperature was elevated from 70 to 110°C during drying of Agave angustifolia Haw leaf, the bulk and tapped density of dried powders decreased from 0.66 to 0.55 g/cm3 and 0.77 to 0.70 g/cm3, respectively.
The temperature of convective drying had a significant (p < 0.05) effect on the particle density of powder. As the drying temperature increased, the particle density of the powder also increased as shown in . The particle density varied from 1.13 to 1.74 g/cm3 which mean about 54% increase was observed in the values when the temperature of convective drying was raised from 30 to 70°C. However, contradicted findings were observed by by Raja et al.,[Citation59] who found that raising the air temperature from 40 to 120°C during the drying of blanched Carica papaya L. leaf resulted in a decrease of particle porosity of dried powders from 1410 to 1360 kg/m3.The variation in density in porous media is heavily influenced by factors such as porosity (the volume of gas within the sample) and the pattern of shrinkage. In present study, the increase in bulk density of Stevia leaf dried powder may be attributed to the densification of solid components like carbohydrates when water is evaporated.[Citation57]
The bulk porosity of the powder increased as the drying temperature was increased from 30 to 80°C as shown in . A 10.59% increase was observed in values of porosity between 30 and 80°C. This might be due the reason that porosity is inversely proportional to bulk density.[Citation60] Moreover, the porosity of sample increases as the pores containing water is replaced with air.[Citation57] Raja et al.,[Citation59] showed that increasing the air temperature from 40 to 120°C during drying of blanched Carica papaya L. leaf, particle porosity of dried powders showed a variation between 0.536 and 0.621.
Flowability indexes
shows the effect of drying temperature on the Carr index (CI), Hausner ratio (HR), and angle of repose (α) of Stevia leaf powder. As the drying temperature increased, both the Carr index and Hausner ratio decreased. The compressibility of a material is classified as follows based on the Carr index (CI) values: very good when CI is less than 15%, good when CI is between 15% and 20%, fair when CI is between 20% and 35%, bad when CI is between 35% and 45%, and very bad when CI is greater than 45%, while a low cohesiveness is indicated when HR is less than 1.2, an intermediate cohesiveness falls within the range of 1.2 to 1.4, and a high cohesiveness is observed when HR exceeds 1.4. Powders with a repose angle up to 35º are categorized as free-flowing materials. A repose angle between 35º and 45º indicates a fairly cohesive nature. If the repose angle ranges from 45º to 55º, the powder is considered cohesive, while a repose angle exceeding 55º indicates a very cohesive nature.[Citation61,Citation62] In this study, the CI, HR, and α values ranged from 5.73 to 11.92%, 1.061 to 1.135, and 22.62 to 29.05, respectively, as the air temperature increased from 30 to 80°C. Thus, a decrement of 51.92%, 6.52%, and 22.13% was observed in CI, HR, and α values, repectievly, with a rise in drying temperature. It is clear from experimental data that dried Stevia leaf powder possesses excellent flowability characteristics. The investigation also revealed that the flowability of the substance enhanced as the drying temperature was raised. In an another investigation by Dehghannya et al.,[Citation58] it was shown that the Carr index and Hausner ratio exhibited a downward trend, indicating that the flowability of the powder improved with an increase in drying temperature. Öztekin[Citation63] reported the angle of repose (α) in range of 19.96–25.04° when laurel berry was dried in hot air at a temperature from 60°C to 80°C.
Conclusion
Stevia leaves were dried in a hot-air dryer at different temperature levels (30, 40, 50, 60, 70, and 90) to evaluate drying kinetics, color changes followed by quality analysis of dried powder. The study concluded that higher drying air temperatures led to reduced average drying times and increased drying rates. The drying kinetics of stevia leaves were satisfactorily described using Logarithmic model (R2 ≥0.9952). By comparing mathematical, ANN and ANFIS models, the ANFIS outputs were shown to be more accurate with R2 value of 0.9998. The ANFIS has proven to be a feasible alternative for modeling thin layer drying of Stevia leaves, offering satisfactory performance and simplicity. The convective drying process significantly (p < .05) affected the color properties of the Stevia leaves. Moreover, increasing drying temperature from 30 to 80°C decreased the aw, tapped density, CI, HR, and α. Conversely, there was an increase in WSI, HG, particle density, and bulk porosity of the dried Stevia leaf powder, while no significant (p > .05) change in bulk density was observed. Ascorbic acid content and antioxidant activity showed decreasing trend from 30 to 80°C, while the total phenol content increased until 50°C. The findings of this study will provide valuable information to producers regarding the drying behaviors and powder properties of the obtained dried Stevia leaf powder. However, additional research is needed to assess the ability of ANFISs to precisely predict alterations in the nutritional composition of agricultural produce during the drying process.
Acknowledgments
Authors are thankful to AICRP (All India Coordinated Research Project) on Post-Harvest Engineering & Technology and Punjab Agricultural University for supporting research work.
Data availability statement
The authors have chosen not to share data.
Disclosure statement
The authors declare that they have no known competing financial interests or personal relationships that could have appeared to influence the work reported in this paper
Additional information
Funding
References
- Bakhshipour, A.; Zareiforoush, H.; Bagheri, I. Mathematical and Intelligent Modeling of Stevia (Stevia Rebaudiana) Leaves Drying in an Infrared-Assisted Continuous Hybrid Solar Dryer. Food Sci. & Nutrition. 2021, 9(1), 532–543. DOI: 10.1002/FSN3.2022.
- Kalsi, B. S.; Singh, S.; Alam, M. S.; Bhatia, S. Microwave Drying Modelling of Stevia Rebaudiana Leaves Using Artificial Neural Network and Its Effect on Color and Biochemical Attributes. J. Food Qual. 2023, 2023, 1–12. DOI: 10.1155/2023/2811491.
- Castillo Téllez, M.; Pilatowsky Figueroa, I.; Castillo Téllez, B.; López Vidaña, E. C.; López Ortiz, A. Solar Drying of Stevia (Rebaudiana Bertoni) Leaves Using Direct and Indirect Technologies. Solar Energy. 2018, 159, 898–907. DOI: 10.1016/J.SOLENER.2017.11.031.
- Hidar, N.; Ouhammou, M.; Mghazli, S.; Idlimam, A.; Hajjaj, A.; Bouchdoug, M.; Jaouad, A.; Mahrouz, M. The Impact of Solar Convective Drying on Kinetics, Bioactive Compounds and Microstructure of Stevia Leaves. Renewable Energy. 2020, 161, 1176–1183. DOI: 10.1016/J.RENENE.2020.07.124.
- Khodja, Y. K.; Dahmoune, F.; Bey, M. B.; Madani, K.; Khettal, B. Conventional Method and Microwave Drying Kinetics of Laurus Nobilis Leaves: Effects on Phenolic Compounds and Antioxidant Activity. Braz. J. Food Technol. 2020, 23. DOI: 10.1590/1981-6723.21419.
- Hashim, N.; Daniel, O.; Rahaman, E. A Preliminary Study: Kinetic Model of Drying Process of Pumpkins (Cucurbita Moschata) in a Convective Hot Air Dryer. Agric. Agric. Sci. Procedia. 2014, 2, 345–352. DOI: 10.1016/J.AASPRO.2014.11.048.
- Demiray, E.; Tulek, Y. Drying characteristics of garlic (Allium sativum L) slices in a convective hot air dryer. Heat & Mass. Trans. /waerme- Und Stoffuebertragung. 2014, 50(6), 779–786. DOI: 10.1007/s00231-013-1286-9.
- Sahoo, M.; Titikshya, S.; Aradwad, P.; Kumar, V.; Naik, S. N. Study of the Drying Behaviour and Color Kinetics of Convective Drying of Yam (Dioscorea Hispida) Slices. Ind. Crops. Prod. 2022, 176, 176 114258. DOI: 10.1016/j.indcrop.2021.114258.
- Chen, X.; Li, X.; Mao, X.; Huang, H.; Wang, T.; Qu, Z.; Miao, J.; Gao, W. Effects of Drying Processes on Starch-Related Physicochemical Properties, Bioactive Components and Antioxidant Properties of Yam Flours. Food Chem. 2017, 224, 224–232. DOI: 10.1016/J.FOODCHEM.2016.12.028.
- Alara, O. R.; Abdurahman, N. H.; Olalere, O. A. Mathematical Modelling and Morphological Properties of Thin Layer Oven Drying of Vernonia Amygdalina Leaves. J. Saudi Soc. Agric. Sci. 2019, 18(3), 309–315. DOI: 10.1016/j.jssas.2017.09.003.
- Tzempelikos, D. A.; Mitrakos, D.; Vouros, A. P.; Bardakas, A. V.; Filios, A. E.; Margaris, D. P. Numerical Modeling of Heat and Mass Transfer During Convective Drying of Cylindrical Quince Slices. J. Food Eng. 2015, 156, 10–21. DOI: 10.1016/j.jfoodeng.2015.01.017.
- Abbaspour‐Gilandeh, Y.; Jahanbakhshi, A.; Kaveh, M. Prediction Kinetic, Energy and Exergy of Quince Under Hot Air Dryer Using ANNs and ANFIS. Food Sci. & Nutri. 2020, 8(1), 594–611. DOI: 10.1002/fsn3.1347.
- Okonkwo, C. E.; Olaniran, A. F.; Adeyi, A. J.; Adeyi, O.; Ojediran, J. O.; Erinle, O. C.; Mary, I. Y.; Taiwo, A. E. Neural Network and Adaptive Neuro‐Fuzzy Inference System Modeling of the Hot Air‐Drying Process of Orange‐Fleshed Sweet Potato. J. Food Proc. Preserv. 2022, 46(3). DOI: 10.1111/jfpp.16312.
- Kaveh, M.; Rasooli Sharabiani, V.; Amiri Chayjan, R.; Taghinezhad, E.; Abbaspour-Gilandeh, Y.; Golpour, I. ANFIS and ANNs Model for Prediction of Moisture Diffusivity and Specific Energy Consumption Potato, Garlic and Cantaloupe Drying Under Convective Hot Air Dryer. Info. Process. Agric. 2018, 5(3), 372–387. DOI: 10.1016/j.inpa.2018.05.003.
- Lemus-Mondaca, R.; Ah-Hen, K.; Vega-Gálvez, A.; Honores, C.; Moraga, N. O. Stevia Rebaudiana Leaves: Effect of Drying Process Temperature on Bioactive Components, Antioxidant Capacity and Natural Sweeteners. Plant. Foods. Human. Nutr. 2016, 71(1), 49–56. DOI: 10.1007/s11130-015-0524-3.
- Lemus-Mondaca, R.; Vega-Gálvez, A.; Rojas, P.; Stucken, K.; Delporte, C.; Valenzuela-Barra, G.; Jagus, R. J.; Agüero, M. V.; Pasten, A. Antioxidant, Antimicrobial and Anti-Inflammatory Potential of Stevia Rebaudiana Leaves: Effect of Different Drying Methods. J. Appl. Res. Med. Aromat. Plants. 2018, 11, 37–46. DOI: 10.1016/j.jarmap.2018.10.003.
- Lemus-Mondaca, R.; Zura-Bravo, L.; Ah-Hen, K.; Di Scala, K. Effect of Drying Methods on Drying Kinetics, Energy Features, Thermophysical and Microstructural Properties of Stevia Rebaudiana Leaves. J. Sci. Food Agric. 2021, 101(15), 6484–6495. DOI: 10.1002/JSFA.11320.
- AOAC. Official Methods of Analysis of AOAC International -20th Edition, 2016, 20th Ed;AOAC: Gaithersburg, 2016; p. 3172.
- Mahmood, N.; Liu, Y.; Munir, Z.; Zhang, Y.; Niazi, B. M. K. Effects of Hot Air Assisted Radio Frequency Drying on Heating Uniformity, Drying Characteristics and Quality of Paddy. LWT. 2022, 158, 113131. DOI: 10.1016/j.lwt.2022.113131.
- Zhang, P.; Fang, D.; Pei, F.; Wang, C.; Jiang, W.; Hu, Q.; Ma, N. Nanocomposite Packaging Materials Delay the Browning of Agaricus Bisporus by Modulating the Melanin Pathway. Postharvest. Biol. Technol. 2022, 192, 112014. DOI: 10.1016/J.POSTHARVBIO.2022.112014.
- Mouhoubi, K.; Boulekbache-Makhlouf, L.; Mehaba, W.; Himed-Idir, H.; Madani, K. Convective and Microwave Drying of Coriander Leaves: Kinetics Characteristics and Modeling, Phenolic Contents, Antioxidant Activity, and Principal Component Analysis. J. Food Process Eng. 2022, 45(1), e13932. DOI: 10.1111/JFPE.13932.
- Sharabiani, V. R.; Kaveh, M.; Taghinezhad, E.; Abbaszadeh, R.; Khalife, E.; Szymanek, M.; Dziwulska-Hunek, A. Application of Artificial Neural Networks, Support Vector, Adaptive Neuro-Fuzzy Inference Systems for the Moisture Ratio of Parboiled Hulls. Appl. Sci. 2022, 12(4), 1771. DOI: 10.3390/app12041771.
- Kaveh, M.; Abbaspour-Gilandeh, Y.; Chen, G. Drying Kinetic, Quality, Energy and Exergy Performance of Hot Air-Rotary Drum Drying of Green Peas Using Adaptive Neuro-Fuzzy Inference System. Food Bioprod. Process. 2020, 124, 168–183. DOI: 10.1016/j.fbp.2020.08.011.
- Kalsi, B. S.; Singh, S.; Alam, M. S.; Bhatia, S. Application of Thermosonication for Guava Juice Processing: Impacts on Bioactive, Microbial, Enzymatic and Quality Attributes. Ultrason. Sonochem. 2023, 99, 106595. DOI: 10.1016/j.ultsonch.2023.106595.
- Zalpouri, R.; Singh, M.; Kaur, P.; Kaur, A.; Gaikwad, K. K.; Singh, A. Drying Kinetics, Physicochemical and Thermal Analysis of Onion Puree Dried Using a Refractance Window Dryer. Processes. 2023, 11(3), 700. DOI: 10.3390/pr11030700.
- Xu, W.; Sun, H.; Li, H.; Li, Z.; Zheng, S.; Luo, D.; Ning, Y.; Wang, Y.; Shah, B. R. Preparation and Characterization of Tea Oil Powder with High Water Solubility Using Pickering Emulsion Template and Vacuum Freeze-Drying. LWT. 2022, 160, 113330. DOI: 10.1016/j.lwt.2022.113330.
- Bakshi, G.; Ananthanarayan, L. Characterization of Lemon Peel Powder and Its Application as a Source of Pectin Degrading Enzyme in Clarification of Cloudy Apple Juice. J. Food Sci. Technol. 2022, 59(7), 2535–2544. DOI: 10.1007/s13197-021-05270-7.
- Padhi, S.; Dwivedi, M. Physico-Chemical, Structural, Functional and Powder Flow Properties of Unripe Green Banana Flour After the Application of Refractance Window Drying. Fut. Foods. 2022, 5, 100101. DOI: 10.1016/j.fufo.2021.100101.
- Camacho, M. M.; Silva-Espinoza, M. A.; Martínez-Navarrete, N. Flowability, Rehydration Behaviour and Bioactive Compounds of an Orange Powder Product as Affected by Particle Size. Food Bioprocess. Technol. 2022, 15(3), 683–692. DOI: 10.1007/s11947-022-02773-9.
- Luka, B. S.; Vihikwagh, Q. M.; Ngabea, S. A.; Mactony, M. J.; Zakka, R.; Yuguda, T. K.; Adnouni, M. Convective and Microwave Drying Kinetics of White Cabbage (Brassica Oleracae Var Capitata L.): Mathematical Modelling, Thermodynamic Properties, Energy Consumption and Reconstitution Kinetics. J. Agric. Food. Res. 2023, 12, 100605. DOI: 10.1016/j.jafr.2023.100605.
- Ghnimi, T.; Hassini, L.; Bagane, M. Experimental Study of Water Desorption Isotherms and Thin-Layer Convective Drying Kinetics of Bay Laurel Leaves. Heat Mass Transfer. 2016, 52(12), 2649–2659. DOI: 10.1007/s00231-016-1770-0.
- Ben Haj Said, L.; Najjaa, H.; Farhat, A.; Neffati, M.; Bellagha, S. Thin Layer Convective Air Drying of Wild Edible Plant (Allium Roseum) Leaves: Experimental Kinetics, Modeling and Quality. J. Food Sci. Technol. 2015, 52, 3739–3749. DOI: 10.1007/s13197-014-1435-2.
- Stępień, A. E.; Gorzelany, J.; Matłok, N.; Lech, K.; Figiel, A. The Effect of Drying Methods on the Energy Consumption, Bioactive Potential and Colour of Dried Leaves of Pink Rock Rose (Cistus Creticus). J. Food Sci. Technol. 2019, 56(5), 2386–2394. DOI: 10.1007/s13197-019-03656-2.
- Ali, A.; Oon, C. C.; Chua, B. L.; Figiel, A.; Chong, C. H.; Wojdylo, A.; Turkiewicz, I. P.; Szumny, A.; Łyczko, J. Volatile and Polyphenol Composition, Anti-Oxidant, Anti-Diabetic and Anti-Aging Properties, and Drying Kinetics as Affected by Convective and Hybrid Vacuum Microwave Drying of Rosmarinus Officinalis L. Ind. Crops Prod. 2020, 151, 112463. DOI: 10.1016/j.indcrop.2020.112463.
- Kouhila, M.; Moussaoui, H.; Lamsyehe, H.; Tagnamas, Z.; Bahammou, Y.; Idlimam, A.; Lamharrar, A. Drying Characteristics and Kinetics Solar Drying of Mediterranean Mussel (Mytilus Galloprovincilis) Type Under Forced Convection. Renewable Energy. 2020, 147, 833–844. DOI: 10.1016/J.RENENE.2019.09.055.
- Miraei Ashtiani, S.-H.; Salarikia, A.; Golzarian, M. R. Analyzing Drying Characteristics and Modeling of Thin Layers of Peppermint Leaves Under Hot-Air and Infrared Treatments. Information Process. Agric. 2017, 4(2), 128–139. DOI: 10.1016/j.inpa.2017.03.001.
- Chen, Q.; Bi, J.; Wu, X.; Yi, J.; Zhou, L.; Zhou, Y. Drying Kinetics and Quality Attributes of Jujube (Zizyphus Jujuba Miller) Slices Dried by Hot-Air and Short- and Medium-Wave Infrared Radiation. LWT - Food Sci. Technol. 2015, 64(2), 759–766. DOI: 10.1016/J.LWT.2015.06.071.
- Kaveh, M.; Jahanbakhshi, A.; Abbaspour-Gilandeh, Y.; Taghinezhad, E.; Moghimi, M. B. F. The Effect of Ultrasound Pre-Treatment on Quality, Drying, and Thermodynamic Attributes of Almond Kernel Under Convective Dryer Using ANNs and ANFIS Network. J. Food Process Eng. 2018, 41(7), e12868. DOI: 10.1111/jfpe.12868.
- Srikanth, K. S.; Sharanagat, V. S.; Kumar, Y.; Bhadra, R.; Singh, L.; Nema, P. K.; Kumar, V. Convective Drying and Quality Attributes of Elephant Foot Yam (Amorphophallus Paeoniifolius). LWT. 2019, 99, 8–16. DOI: 10.1016/j.lwt.2018.09.049.
- Lule, F.; Koyuncu, T. Convective and Microwave Drying Characteristics, Energy Requirement and Color Retention of Dehydrated Nettle Leaves (Urtica Diocia L.). Legume Res. Intern. J. 2017, 40(4), 649–654. DOI: 10.18805/lr.v0i0.8409.
- Ek, P.; Araujo, A. C.; Oliveira, S. M.; Ramos, I. N.; Brandao, T. R.; Silva, C. L. Assessment of Nutritional Quality and Color Parameters of Convective Dried Watercress (Nasturtium officinale). J. Food Process. Preserv. 2018, 42(2), e13459. DOI: 10.1111/jfpp.13459.
- Youssef, K. M.; Mokhtar, S. M. Effect of Drying Methods on the Antioxidant Capacity, Color and Phytochemicals of Portulaca oleracea L. Leaves. J. Nutr. Food. Sci. 2014, 4(6), 322. DOI: 10.4172/2155-9600.1000322.
- Demiray, E.; Tulek, Y. Color Degradation Kinetics of Carrot (D Aucus Carota L.) Slices During Hot Air Drying. J. Food Process. Preserv. 2015, 39(6), 800–805. DOI: 10.1111/jfpp.12290.
- Kalsi, B. S.; Singh, S.; Alam, M. S. Influence of Ultrasound Processing on the Quality of Guava Juice. J. Food Process Eng. 2022, 46(6), e14163. DOI: 10.1111/JFPE.14163.
- Oliveira, S. M.; Ramos, I. N.; Brandão, T. R. S.; Silva, C. L. M. Effect of Air-Drying Temperature on the Quality and Bioactive Characteristics of Dried Galega Kale (B Rassica Oleracea L. Var. Acephala). J. Food Process. Preserv. 2015, 39(6), 2485–2496. DOI: 10.1111/jfpp.12498.
- Alibaş, İ.; Yılmaz, A. Influence of Basic Drying Techniques on Color, Protein and Mineral Composition of Coriander Leaves. Kahramanmaraş Sütçü İmam Üniversitesi Tarım ve Doğa Dergisi. 2023, 26(5), 1165–1177. DOI: 10.18016/ksutarimdoga.vi.1144982.
- Oliveira, S. M.; Brandão, T. R. S.; Silva, C. L. M. Influence of Drying Processes and Pretreatments on Nutritional and Bioactive Characteristics of Dried Vegetables: A Review. Food Eng. Rev. 2016, 8(2), 134–163. DOI: 10.1007/s12393-015-9124-0.
- Liu, L.; Wang, Y.; Zhao, D.; An, K.; Ding, S.; Wang, Z. Effect of Carbonic Maceration Pre-Treatment on Drying Kinetics of Chilli (Capsicum Annuum L.) Flesh and Quality of Dried Product. Food Bioprocess. Technol. 2014, 7(9), 2516–2527. DOI: 10.1007/s11947-014-1253-6.
- Djendoubi Mrad, N.; Boudhrioua, N.; Kechaou, N.; Courtois, F.; Bonazzi, C. Influence of Air Drying Temperature on Kinetics, Physicochemical Properties, Total Phenolic Content and Ascorbic Acid of Pears. Food Bioprod. Process. 2012, 90(3), 433–441. DOI: 10.1016/j.fbp.2011.11.009.
- Khodja, Y. K.; Dahmoune, F.; Khettal, K.; Madani, B.; Khettal, B. Conventional Method and Microwave Drying Kinetics of Laurus Nobilis Leaves: Effects on Phenolic Compounds and Antioxidant Activity. Braz. J. Food Technol. 2020, 23. DOI: 10.1590/1981-6723.21419.
- İ̇̇lter, I.; Akyıl, S.; Devseren, E.; Okut, D.; Koç, M.; Kaymak Ertekin, F. Microwave and Hot Air Drying of Garlic Puree: Drying Kinetics and Quality Characteristics. Heat Mass Transfer. 2018, 54(7), 2101–2112. DOI: 10.1007/s00231-018-2294-6.
- Chua, L. Y. W.; Chua, B. L.; Figiel, A.; Chong, C. H.; Wojdyło, A.; Szumny, A.; Łyczko, J. Drying of Phyla Nodiflora Leaves: Antioxidant Activity, Volatile and Phytosterol Content, Energy Consumption, and Quality Studies. Processes. 2019, 7(4), 210. DOI: 10.3390/pr7040210.
- Mishra, M.; Kandasamy, P.; Shukla, R. N.; Kumar, A. Convective Hot-Air Drying of Green Mango: Influence of Hot Water Blanching and Chemical Pretreatments on Drying Kinetics and Physicochemical Properties of Dried Product. Int. J. Fruit Sci. 2021, 21(1), 732–757. DOI: 10.1080/15538362.2021.1930626.
- Azizpour, M.; Mohebbi, M.; Khodaparast, M. H. H. Effects of Foam-Mat Drying Temperature on Physico-Chemical and Microstructural Properties of Shrimp Powder. Innovative Food Science Emerging Technologies. 2016, 34, 122–126. DOI: 10.1016/j.ifset.2016.01.002.
- González-Jiménez, F. E. ;, Barojas-Zavaleta, J. E. ;, Vivar-Vera, G. ;, Peredo-Lovillo, A. ;, Morales-Tapia, A. A. ;, Del Ángel-Zumaya, J. A. ;, Erik González-Jiménez, F.; Barojas-Zavaleta, J. E.; Vivar-Vera, G.; Peredo-Lovillo, A.; Morales-Tapia, A. A.; Antonio, J.; Ángel-Zumaya, D.; Reyes-Reyes, M.; Alamilla-Beltrán, L.; Leyva-Daniel, D. E., & Jiménez-Guzmán, J. Effect of Drying Temperature on the Physicochemical, Functional, and Microstructural Properties of Powders from Agave Angustifolia Haw and Agave Rhodacantha Trel. Horticult. 2022. 2022, 8(11), 1070. DOI: 10.3390/HORTICULTURAE8111070.
- Schuck, P.; Dolivet, A.; Jeantet, R. Determination of the Sorption Isotherm, Water Activity and Hygroscopicity of Powders. Anal. Meth. Food & Dairy Powders. 2012, 167–190. DOI: 10.1002/9781118307397.CH11.
- Aprajeeta, J.; Gopirajah, R.; Anandharamakrishnan, C. Shrinkage and Porosity Effects on Heat and Mass Transfer During Potato Drying. J. Food Eng. 2015, 144, 119–128. DOI: 10.1016/j.jfoodeng.2014.08.004.
- Dehghannya, J.; Pourahmad, M.; Ghanbarzadeh, B.; Ghaffari, H. Heat and Mass Transfer Enhancement During Foam-Mat Drying Process of Lime Juice: Impact of Convective Hot Air Temperature. Int. J. Therm. Sci. 2019, 135, 30–43. DOI: 10.1016/j.ijthermalsci.2018.07.023.
- Raja, K. S.; Taip, F. S.; Azmi, M. M. Z.; Shishir, M. R. I. Effect of Pre-Treatment and Different Drying Methods on the Physicochemical Properties of Carica Papaya L. Leaf Powder. J. Saudi Soc. Agric. Sci. 2019, 18(2), 150–156. DOI: 10.1016/j.jssas.2017.04.001.
- Dhurve, P.; Kumar Arora, V. (2022). Investigation of Geometric and Gravimetric Properties of Pumpkin Seeds (Cucurbita Maxima) Under Tray Drying. Materials Today: Proceedings, 59, 437–441. 10.1016/j.matpr.2021.11.452
- Seerangurayar, T.; Manickavasagan, A.; Al-Ismaili, A. M.; Al-Mulla, Y. A. Effect of Carrier Agents on Flowability and Microstructural Properties of Foam-Mat Freeze Dried Date Powder. J. Food Eng. 2017, 215, 33–43. DOI: 10.1016/j.jfoodeng.2017.07.016.
- CARR, R. E. Fundus Flavimaculatus. Arch. Ophtha. 1965, 74(2), 163–168. DOI: 10.1001/archopht.1965.00970040165007.
- Öztekin, S.; Erdem, T.; Karaaslan, S. The Influence of Drying on Some Physical Properties of Laurel Berry. Tarım Makinaları Bilimi Dergisi (Journal of Agricultural Machinery Science). 2010, 6(2), 79–84.