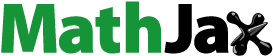
ABSTRACT
For decades researches have been conducted in Ethiopia to improve wheat productivity and quality. This involved extensive selection of breeding lines based on agronomic and laboratory data from numerous trials. However, generating laboratory data for all these services was both time-consuming and expensive. Hence, the study aimed to develop a very rapid and cost-effective testing method for wheat genotypes and varieties using near-infrared spectroscopy. For this purpose, we collected 155 bread wheat samples from high-growing potential areas of Sinana, Kulumsa, and Holeta. The wet chemistry analysis was done in duplicate following the international standard official methods. The same samples were also scanned using TANGO’S Bruker NIRS machine in duplicate to acquire spectral data. A calibration model was then developed by fitting the reference and spectral data using the partial least square (PLS). The calibration models exhibited a high level of strength for all parameters. The performance of the calibration model for protein (R2 = 0.99; RPD = 9.63), moisture (R2 = 0.96; RPD = 5.29), ash (R2 = 0.98; RPD = 6.39), total gluten (R2 = 0.98; RPD = 8.09), dry gluten (R2 = 0.97; RPD = 5.57), gluten index (R2 = 0.96; RPD = 5.23), and falling number (R2 = 0.97 and RPD = 6.23) were deemed suitable for wheat process and quality control purposes. But the models for moisture and gluten index (R2 = 0.96) were found to be suitable for screening and simple quality control purposes only. Therefore, this study clearly demonstrated that the near-infrared spectroscopy model is a highly reliable and robust method for predicting the key quality traits of bread wheat grains, serving the needs of both breeding programs and industry raw material control.
Introduction
Bread wheat (Triticum aestivum L.) is staple food for more than 35% of the world’s population and an annual global production is 772.6 million tons.[Citation1] Globally, China, India, and Russia are the largest wheat producers, while South Africa and Ethiopia are the largest wheat producers in sub-Saharan Africa.[Citation2] Ethiopia’s annual production is about 5.8 million tons with a mean productivity of 3 tons per hectare,[Citation3] which is relatively lower than the attainable yield of the crop, reaching up to 5 t/ha.[Citation4] Wheat accounts for about 17% of the total grain production in Ethiopia, making it the third most important cereal crop after teff and maize.[Citation5] However, wheat production and productivity in Ethiopia is not sufficient enough to meet the consumers and industry needs. Wheat breeding research has been primarily conducted by the Ethiopian Institute of Agricultural Research (EIAR) since the 1960s in order to develop high yielding, drought-tolerant, and disease-resistant wheat varieties with broad adaptation and acceptable end-use qualities. More recently, regional agricultural research institutes and universities have collaborated primarily with the institute and an international agricultural research centers to improve the wheat breading research.[Citation6]
Developing high yielding and stress tolerant new wheat varieties and improving its grain quality is very crucial, because the grain quality of local wheat varieties do not meet the industry quality requirements most of the times. The major wheat grain quality parameters are moisture, protein, ash, and wet gluten contents of the flour and technological parameters such as the sedimentation value, falling number, and rheological properties of wheat flour dough. Because wheat is a raw material for processing of pasta products, breads, cakes, biscuits, and noodles.[Citation7] Wheat provides numerous nutrients, such as carbohydrates, protein, and minerals. However, wheat products are sometimes challenged by the inferior quality parameters which could not fulfill the industry quality criteria. To determine the most important quality parameters laboratory chemical analysis methods have good precision, but most of them are laborious and time-consuming. Consequently, there is an urgent need to develop a rapid, labor-saving, and efficient analytical method for the quality of wheat grain.[Citation8]
Therefore, near-infrared spectroscopy (NIRS) is a rapid, nondestructive, and widely used method to predict the organic compounds of wheat grain materials based on electromagnetic wave interactions.[Citation9] The analysis of organic compounds with NIRs is based on the differences between C-H, O-H, N-H, and S-H functional groups’ structure.[Citation10] NIR is known to be also price effective and robust tools that predict multiple constituents in agricultural products at the same time.[Citation11] While several companies offer different types of NIR machines such as the FOSS-DS2500 flour analyzer, Bruker’s Tango FT-NIR spectrometer and Perten-IM9520) within the last decade, but currently the market has offered also options for portable NIR instruments as well.[Citation12] In our study, we only focused on using the Bruker’s Tango FT-NIR spectrometer as it offers user simplicity and is much more suitable for grain-based analysis.
In this study, it became significant to develop a NIRS calibration model to enable a fast multivariate analysis of protein, moisture, ash, falling number, total gluten, dry gluten and gluten index quality traits in bread wheat grain. Because this quick and reliable multivariate analysis of wheat grain quality helps the pastry industries easily control the raw materials for their regular processing programs to check the required criteria and it also greatly reduces the extensive workloads of line selection in wheat breeding research. Purposively, samples were taken from Holeta, Kulumsa, and Sinana, which are high-potential wheat-growing locations in the Ethiopian highlands, in order to capture the genetic and environmental variability of the wheat grain.
The calibration model was built using wet chemistry and spectral data that were generated by laboratory chemical analysis and NIR machine scanning, respectively. Spectral data can be analyzed under all wave bands and multiple wavelengths qualitatively and quantitatively. The calibration model was developed using the most widely used chemo-metric multivariate statistical analysis, including principal component analysis (PCA, and partial least-squares regression (PLSR).[Citation13] Additionally, the statistical outcomes of the root mean square error (RMSE), relative deviation performance (RPD), and coefficient of determination (R2) were used to evaluate the predictive performances of the models.[Citation9]
Materials and methods
Materials collection and preparation
A total of 155 bread wheat samples were gathered from production-potential locations in the Ethiopian highlands, specifically from Kulumsa, Holeta, and Sinana wheat breading research centers. After being sun-dried, the grain samples were taken from the randomized total harvest of the 2020, 2021, and 2022 seasons. They were manually cleaned to get rid of non-wheat foreign materials and broken seeds. For every wet chemistry analysis, 200 g of cleaned wheat samples were used. The samples were milled using 0.5 mm sieve size sample miller (Perten Instruments, Laboratory Mill 120, and Germany). All wet chemistry laboratory analyses were carried out at Kulumsa Agricultural Research Center’s Food Science and Nutrition Research Laboratory. However, 150 g of the identical wheat grain samples were taken in order to acquire NIR spectral data for each of the samples that were gathered. The NIR spectral data acquisition and development of model calibration were carried out at Holeta Agricultural Research Center’s Food Science and Nutrition Laboratory. The total procedure from sample collection to calibration model development work flow chart is shown as .
Reference sample wet chemistry analysis
To use as reference data, samples were subjected to wet chemistry analyses for every parameter. The laboratory analysis was conducted to determine the moisture content, crude protein, ash content, falling number, total gluten, dry gluten, and gluten index, which are the specific quality traits of interest for bread wheat. All the parameters were analyzed in duplicate for a single sample. The moisture content was assessed by employing the official method 925:10 of the Association of American Cereal Chemists.[Citation14] A quantity of approximately 5 g of dried bread wheat flour sample was carefully weighed and placed into a hot-air electric oven (Model J. 02707 Michel, England) preheated to 105°C for a duration of 3 hours. Once the sample was thoroughly dried in the oven, it was allowed to cool in a desiccator for 30 minutes before being weighed. Then the mathematical calculation of the total moisture content of the sample was computed as follows:
The Kjeldahl method, as outlined in the AOAC standard method 981:10,[Citation14] was employed to determine the crude protein content. Each dried flour sample, weighing 0.5 g was placed in a kjeldhal flask with a volume of 250 ml. For each flask, 10 ml of concentrated H2SO4 and a mixture of catalysts consisting of copper sulfate and potassium sulfate (7 g) were added. The samples were then digested on a digester block at a temperature of 400°C for duration of 3 hours, until a clear solution was appeared. Subsequently, the digested samples were allowed to cool in a fume hood. The clear digested solution was subjected to distillation by the addition of 40% NaOH and distilled water, followed by titration with 0.1 N HCl. The mathematical calculation of crude protein content was computed by
where VHCl = Volume of hydrochloric acid in liter consumed to the endpoint of the titration, N = Normality of hydrochloric acid (HCl), 14 = the molecular weight of nitrogen. Total ash content of the samples was determined using muffle furnace according to AOAC international official standard method[Citation14] method 923:03. About 2 g of dried flour sample was weighed in a crucible and transferred to muffle furnace set at 550°C for 5 hr, after completely changed into ash it was cooled in desiccators for 30 min and weighed, and calculated.
Falling Number (FN) is determined on a 7 g sample of ground wheat by AACC International Method 56–81.04: Determination of Falling Number. A 300 g sample of wheat is ground in a Falling Number Laboratory Mill 3100 according to ICC Standard Method No. 107. FN is used to estimate the alpha-amylase activity resulting from sprout damage in wheat.
The gluten quantity and quality were analyzed by using a perten glutomatic washing instrument (perten, Sweden) according to the standard method 38–12.[Citation15] Where 10 g of flour samples were weighed and placed into the glutomatic washing chamber on top of polyester screen, then every sample of flour was mixed and washed with 2% salt (NaCl) solution for 5 minutes. Then the total wet gluten was removed from the washing chamber placed in the centrifuge holder and centrifuged to 1 minute at 6000 rpm in centrifuge and stop automatically. The passed gluten through the sieve was weighed and the residue retained inside the screen was weighed and then dried in a Glutrok 2020 heater at 150 ◦C for 4 minutes to give dry gluten. The dry gluten was then weighed. The wheat gluten index, total gluten content, and dry gluten content were calculated as follows:
Spectroscopy
Initially, the device’s spectroscopic performance was diagnosed by using the gold standard (1.038) and the light trap standard (0.00065) provided by Bruker, a German company. Subsequently, 155 wheat samples were scanned for spectral data using a near-infrared spectrometer (NIRS, Tango2017, Bruker Optics GmbH, Germany). The spectrometer operated in scan and rotating mode within 16 seconds to generate spectral data. The samples were presented using a rotating accessory with a 600 mL sample cuvette. The spectrometer employed 32 scans within the wave number range of 4000–11000 cm−1, with a wave number band resolution of 16 cm−1. To record the spectral data, the device utilized diffusive reflectance and an integrating sphere. Each sample underwent frequency of 32 scans to obtain a single spectral data point, which fell within the predetermined wavelength range. Duplicated spectral measurements were conducted for each sample.
The raw data obtained from the direct near-infrared scan consisted of additive, multiplicative, bias, and scattering effects. To enhance the reliability of the calibration model, the raw spectral data underwent pre-processing using one of a scatter-corrective method known as Standard Normal Variate (SNV) followed by applying the first derivative of the spectral derivation method with 17 smoothing points, which had been previously utilized by.[Citation16]
A total of 155 data sets were analyzed in this study. Among them, 103 data sets were utilized for developing the calibration model, while 52 data sets were reserved for external validation of the model. The calibration model for near-infrared (NIR) spectroscopy was constructed by correlating the laboratory reference data with duplicated spectral data of the same samples. This was achieved through the application of partial least square (PLS) regression and Principal Component Analysis (PCA) using the OPUS version 7.5.1 software provided by Tango (Bruker, Optics GmbH, Germany). The PLS used up to 10 sensitive selected latent variables as it was commonly used in NIR model development which was later proved by try and error that was best for the model robustness. Simultaneously, an independent validation technique was employed to automatically validate the calibration model using a test set validation method.[Citation9]
The performance of the calibration model was assessed using commonly utilized statistical parameters, namely the Coefficient of Determination (R2), Root Mean Square Error Estimation (RMSEE), Root Mean Square Error Prediction (RMSEP), and Relative Predictive Deviation (RPD). These statistical metrics were employed to describe the linear relationship between the ground-truth (the laboratory method) and the NIR spectra, both for the calibration and validation sets. The statistical parameters R2 (Equation 7), RMSE (Equation 8), and RPD (Equation 9) were calculated using the built-in statistical formula in OPUS, as previously employed by[Citation17] and.[Citation18]
where n is the number of samples; y is the ground-truth values of sample i; ŷi is the model-predicted value of sample i; Ӯ is the mean of the ground-truth values; and SD is the standard deviation of the ground-truth values as used by.[Citation19] After all, the calibration model underwent optimization through the utilization of the OPUS software’s optimization procedure. Various frequency regions and spectral pre-treatments were examined during the optimization process. The resultant optimized calibration model was subsequently stored directly in the device memory to facilitate the execution of multivariate analysis.
Results and discussion
Results
NIRS calibration models were developed for end-use wheat quality assessment based on major quality traits. The same samples analyzed for reference data and spectral data were well summarized in result tables and figures. The raw, preprocessed, calibration, and validation results were also nicely illustrated. The calibration model performance evaluation parameters are shown in . As previously described in the literature, calibration model development highly depends on selecting a representative data set, a good condition of NIR device and using accurate wet chemistry analysis method.
The set of calibration samples used in this study also contained the range of chemical and physical variations for which the calibration model was applied. Accordingly, the calibration experiment was established using a mathematical relationship between the NIR spectrum and chemical properties determined by reference methods. Similarly, comparing the chemical reference data variability and model accuracy is a vital point as reported by.[Citation20] The reference sample data results were summarized as maximum, minimum, mean, and standard deviation (SD) in .
Table 1. Statistical description of reference data summary.
The variations in reference sample values were due to location and cultivar-based sample collection which was very important to develop a strong and well-performed calibration model. Because the genotypes trials were grown at different locations because different agronomic practices, seed rate, fertilizer rate, and genotypes variations are very important model strength to include important representatives. These reference data variations occurred from soil types, variations of season, and other practices that may reduce the outliers during calibration as described by.[Citation11]
shows the raw spectral data recorded between wave number ranges of 3944 to 11,528 cm−1 as directly scanned by a near infrared spectrometer. But, many factors could be interfered while collecting spectral data using NIR machine scanning mode. There must be outlier values, wave length biasedness, and performance of the machine and the personal error of the analyst. The raw spectral feature data acquired direct from scanning was pre-treated before being used in model development. That was the reason why two types of spectral features were observed as shown in the results. The left-hand side was the raw spectral data before treated and the right-hand side was the treated and corrected spectral feature before entered in model development. The spectral data underwent pre-treatment using SNV followed by first derivative, resulting in a complete transformation and improved appearance, as depicted in the figure. This indicates that various biases, scatterings, additions, and other physical errors were effectively eliminated during the pre-processing of the raw spectral data.
The absorbance peaks were primarily observed within specific wave number ranges, namely 9400–7500 cm−1, 7500–6100 cm−1, 6100–5450 cm−1, 5450–4600 cm−1, and 4600–4250 cm−1. These ranges corresponded to the functional group of the chemical structure where the highest absorbance was recorded. The vibration of chemical bonds overtone played a significant role in this absorption process, as the wave energy readily interacted with these regions. Consequently, absorbance served as the sole metric for distinguishing between the traits. The main objective of this discovery was accomplished by examining the interaction between spectrum energy and the chemical structure of the grain composition. By utilizing this spectral data, we were able to successfully develop a multivariate model for each trait type. This was made possible by the presence of molecular bonds absorbed the near infrared light, particularly those involving H atoms attached to N, O, and C atoms. These bonds provided valuable information about the relative proportions of C-H, N-H, O-H and S-H bonds during vibration modes, which occurred as the near infrared energy interacted with the bonds. These bonds were integral components of the organic matrix.[Citation19]
Additionally the calibration model performance was described showing the calibration curve of each trait in , and shown below. The curve provided strong evidence of the strong relationship between true values and fit values, which was further supported by the findings in . Each plot observed along the regression line represented the relationship between the data sets and the correlation between the true value (laboratory result) and the predicted values derived from the spectral data. Consequently, the models demonstrated robustness and accuracy, as indicated by the strong alignment of the regression coefficient vector plots with the regression line. The spectral data underwent preprocessing before being used for model building, resulting in minimal observation of outlier data sets on the calibration curve. Additionally, the reference data was meticulously analyzed and played a significant role in achieving this strong regression calibration curve. The calibration curves for all traits remained unaffected by spectral biasness and inaccurate laboratory reference data, as evidenced by the coefficient determination of calibration models exceeding 0.96, as shown in .
Table 2. Calibration model performance evaluation statistical parameters.
The models were validated and checked for their prediction capacities. The principle was predicting quantitative value using the model from the validation set spectral data and comparing the predicted value against the chemistry data set for validation.[Citation11] The calibration model performance was based the following parameters: coefficient of determination (R2) of the calibration and validation, Root Mean Square Error of Estimation (RMSEE) and Relative Performance Deviation (RPD) as indicated in . The RPD value indicates the suitability of the calibration for the prediction. A calibration model with a higher RPD value was more capable to predict the right-sample trait value as.[Citation21]
A NIR estimation could be considered “robust” as long as the correlation coefficient between the results of NIR readings and the reference method is above 0.75.[Citation22] Also, as shown in all the values of R2 were above 0.96 which was strong model to predict the value hopefully. From this point of view, we can say that all the calibration models were an acceptable to be used for the targeted purpose.
Discussion
The model performance was assessed by the coefficient of determination of the calibration (R2), root mean square error (RMSE) and relative performance deviation (RPD). The RPD value indicates the suitability of the calibration for the prediction. With a higher RPD value, most likely the calibration model be able to predict the right-sample parameters as.[Citation21] According to the previously reported classification based on values of RPD < 1.5 indicates that the calibration is not reliable; a value between 1.5 and 2.0 indicates the capacity of a model to distinguish between high and low values; an RPD value between 2.0 and 2.5 signifies the model’s capacity to “approximate” quantitative prediction; a RPD value between 2.5 and 3.0 suggests “good” quantitative prediction; and a RPD value > 3.0 indicates “excellent” quantitative prediction.[Citation23] Similarly, Williams indicated that R2 = 0.70–0.90 suitable for rough screening, R2 = 0.90–0.97 is suitable for screening or quality control, R2 = 0.97–0.99 is suitable for process control, and larger values are suitable for most applications.[Citation9] Based on the classification, the calibration models could be regarded as a trustworthy method to give the most reliable prediction and that could be used in wheat breeding and production.
As results described in in , based on the performance classification the NIR calibration models for protein (R2 = 0.99; RPD = 9.63), total gluten (R2 = 0.98; RPD = 8.09), falling number (R2 = 0.97 and RPD = 6.23), ash (R2 = 0.98; RPD = 6.39) and dry gluten (R2 = 0.97; RPD = 5.57) were very suitable models to use for process control and more other purposes. But calibration models for moisture (R2 = 0.96; RPD = 5.29) and total gluten index (R2 = 0.96; RPD = 5.23) could be usable for screening and quality control[Citation9] and.[Citation23] Also previous similar studies delivered for protein (R2 = 0.98) and moisture (R2 = 0.95) which was most related findings to our work as reported by Dowell et al. .[Citation24] Unlikely other finding was reported by Kahriman & Egesel for protein (R2 = 0.81 and RPD = 2.17), Moisture (R2 = 0.85 and RPD = 2.43) and Gluten index (R2 = 0.76 and RPD = 1.90) which was almost less robust test method as compared to this findings.[Citation16] In a similar vein, interesting finding was reported by Abeshu yielded (R2 = 0.90) of barley protein content for samples collected from the same environment as our sample collection area.[Citation11] This result was comparable to our reports, although it had a lesser practical application. Conversely, Dowell et al. reported a model (R2 >0.97) for wheat flour protein and moisture,[Citation24] while Mutlu et al. reported (R2 = 0.952 and R2 = 0.920) for both wheat flour protein and moisture content, respectively.[Citation25] Additionally, Jiang et al. reported (R2 = 0.96) model for the fatty acid calibration of stored wheat flour.[Citation26] Furthermore, Cozzolino et al. discovered a protein calibration model with an R2 value of 0.95 and an RPD value of 1.8, as well as a total gluten model with an R2 value of 0.70 and an RPD value of 1.2.[Citation19] However, it is important to note that these models are specifically designed for screening purposes only. In addition, another study successfully developed a robust calibration model for various types of cereals protein, with an R2 ≥0.90. This further confirms the effectiveness of NIR models in accurately predicting protein values, particularly for screening, selection and control purposes as reported by Chadalavada et al.[Citation12]
The previous finding reflected similar predictions in the performance of the prediction model. Some of the models were developed using the same NIR machine and multivariate test method procedures. However, different studies reported varying model performances for NIR calibration models. This could be attributed to the use of different NIR machine performances, different methods of reference sample analysis, variations in the nature of samples used, and personal techniques errors. One study also demonstrated a strong correlation between multi-trait prediction accuracy and genetic correlations between end-product and NIR-predicted data (R2 = 0.69–0.77) (Azizinia et al., 2023). Despite these variations, all findings consistently showed the effectiveness and robustness of NIR-based quality analysis. This implies that NIRS can be utilized for line screening, raw material quality control, and industry process monitoring in our case. The calibration models developed in this study specifically proved highly accurate in predicting wheat grain quality parameters, making them suitable for breeding line selections and industry purposes. Therefore, the finding strongly supports the trustworthiness and reliability of using near infra-red spectroscopy models to predict major quality traits in bread wheat grain.[Citation27]
Conclusion
The incorporation of near-infrared spectroscopy into the examination of agricultural products holds significant importance for breeding programs and industries focused on improving the quality characteristics of wheat. This is due to the fact that traditional chemical laboratory analysis methods are both expensive and time-consuming. Thus, the objective of this study was to develop a calibration using Near Infrared Reflectance Spectroscopy (NIR) that would allow for a rapid assessment of quality traits in bread wheat grain. The study successfully produced a highly promising and practical multivariate test model for various quality parameters of wheat grain, including protein, moisture, falling number, total gluten, dry gluten, and gluten index. The calibration models were thoroughly validated and evaluated to assess their strength and reliability in predicting the desired traits and determining their applicability for specific purposes. The results indicated that the calibration models for moisture and gluten index were particularly suitable for screening and quality control purposes, while the other calibration models were more appropriate for process optimization, quality control, and various other applications. Compared to traditional methods, the utilization of multivariate calibration models greatly simplified wheat grain quality testing. Additionally, it allowed for the simultaneous analysis of multiple parameters without the need for sample preparation, reagents and highly trained personnel. Consequently, these calibration models effectively address the time-consuming nature of chemical-based quality trait analysis, enabling breeders to rapidly select genotypes for research purposes and reducing the efforts required by processing industries during raw material control at receiving points. Therefore, NIRS models offer a noninvasive, fast, and cost-efficient testing method for wheat production and utilization, as they assess the quality criteria necessary for the industry and provide valuable research guidance for breeders.
Disclosure statement
The work of this study was the original findings of the authors. Regarding this manuscript no any potential conflict of interest in case of financial, facilitation and technical participation.
References
- Statista., 2021. Top Wheat-Producing Countries 2020/2021. Accessed August 2021. https://www.statista.com/statistics/237912/global-top-wheat-producing-countries.
- USDA. 2019. USDA World Markets and Trade Report. Accessed on December 15, 2019.
- CSA. Agricultural Sample Survey: Report on Area and Production of Major Crops (Private Peasant Holdings, Meher Season). In Volume I Statistical Bulletins, Vol. 590; Addis Ababa: Ethiopia, 2021.
- Zegeye, F.; Alamirew, B.; Tolossa, D. Analysis of Wheat Yield Gap and Variability in Ethiopia. Int. J. Agric. Econon. 2020, 5(4), 89–98. DOI: 10.11648/j.ijae.20200504.11.
- Nigus, M.; Shimelis, H.; Mathew, I.; Abady, S. Wheat Production in the Highlands of Eastern Ethiopia: Opportunities, Challenges and Coping Strategies of Rust Diseases. Acta Agric. Scandinavica, Section B — Soil & Plant Sci. 2022, 72(1), 563–575. DOI: 10.1080/09064710.2021.2022186.
- Wheat Production and Breeding in Ethiopia: Retrospect and Prospects. Crop Breeding, Genetics and Genomics. 2022. DOI: 10.20900/cbgg20220003.
- Zareef, M.; Arslan, M.; Hassan, M. M.; Ahmad, W.; Ali, S.; Li, H.; Ouyang, Q.; Wu, X.; Hashim, M. M.; Chen, Q. Recent Advances in Assessing Qualitative and Quantitative Aspects of Cereals Using Nondestructive Techniques: A Review. Trends Food Sci. Technol. 2021, 116, 815–828. DOI: 10.1016/j.tifs.2021.08.012.
- Zhang, S.; Liu, S.; Shen, L.; Chen, S.; He, L.; Liu, A. Application of Near-Infrared Spectroscopy for the Nondestructive Analysis of Wheat Flour: A Review. Curr. Res. Food Sci. 2022, 5, 1305–1312. DOI: 10.1016/j.crfs.2022.08.006.
- Williams, P. C.; Norris, K. H. Near-Infrared Technology in the Agricultural and Food Industries, 2nd edn ed.; American Association of Cereal Chemists, St. Paul: MN, 2001.
- Sandorfy, C.; BUCHET, R.; LACHENAL, G. Principles of Molecular Vibrations for Near-Infrared Spectroscopy. In Near-Infrared Spectroscopy in Food Science and Technology; Ozaki, Y., McClure, W. F. Christy, A. A., Eds.; John Wiley and Sons, Inc.: Hoboken, NJ, 2007; pp. 11–46.
- Abeshu, Y. Development of NIRS Re-Calibration Model for Ethiopian Barley (Hordeum Vulgare) Lines Traits to Determine Their Brewing Potential. J. Agric. Food. Res. 2021, 6, 100238. DOI: 10.1016/j.jafr.2021.100238.
- Chadalavada, K.; Anbazhagan, K.; Ndour, A.; Choudhary, S.; Palmer, W.; Flynn, J. R.; Mallayee, S.; Pothu, S.; Prasad, K. V. S. V.; Varijakshapanikar, P., et al. NIR Instruments and Prediction Methods for Rapid Access to Grain Protein Content in Multiple Cereals. Sensors. 2022, 22(10), 3710. DOI: 10.3390/s22103710.
- Walsh, K. B.; Golic, M.; Greensill, C. V. Sorting of Fruit Using Near Infrared Spectroscopy: Application to a Range of Fruit and Vegetables for Soluble and Dry Matter Content. J. Near Infrared Spectrosc. 2004, 12(3), 141–148. DOI: 10.1255/jnirs.419.
- AOAC, G. Official Methods of Analysis of AOAC International; AOAC International: Rockville, MD, 2016.
- AACC. Approved Methods of the American Association of Cereal Chemists 10 Th Edition. The Asso. Paul, Offi. Meth. 08-01. 2000, 38-10, 44-15A, 56-70 and56- 81B.
- KAHRIMAN F, EGESEL CÖ. Development of a calibration model to estimate quality traits in wheat flour using NIR (Near Infrared Reflectance) spectroscopy. Res J Agric Sci. 2011 11;43(3):392-400.
- Williams, P.; Dardenne, P.; Flinn, P. Tutorial: Items to Be Included in a Report on a Near Infrared Spectroscopy Project. J. Near Infrared Spectrosc. 2017, 25(2), 85–90. CrossRef. DOI: 10.1177/0967033517702395.
- Williams, P.; Manley, M.; Antoniczyn, J. Near-InfraRed Technoloy-Getting the Best Out of Light; Sun Press Imprint; Stellenbosch, South Africa, 2019. p. 301.
- Cozzolino, D.; Delucchi, I.; Kholi, M.; Vázquez, D. Use of Near Infrared Reflectance Spectroscopy to Evaluate Quality Characteristics in Whole-Wheat Grain. Agri. Técnica. 2006, 66(4). DOI: 10.4067/S0365-28072006000400005.
- Conzen, J.-P. Multivariate Kalibration. Ein praktischer Leitfaden zur Method enent wicklung in der quantitaven Analytik. 4., neu bearb. Aufl; Bruker: Ettlingen, 2005.
- Duffus, C. M.; Cochrane, M. P. Formation of the Barley Grain - Morphology, Physiology and Biochemistry. In Barley: Chemistry and Technology; MacGregor, A. W. Bhatty, R. S., Eds.; American Association of Cereal Chemists, Inc: St. Paul, Minnesota, USA, 1992; pp. 31–67.
- CWS MANUAL. Sensologic Calibration Workshop Version 2.02; Sensologic Gmbh: Germany, 2003.
- Williams, P. The RPD statistic: A tutorial note. NIR. News. 2014, 25(1), 22–26. DOI: 10.1255/nirn.1419.
- Dowell, F. E.; Maghirang, E. B.; Xie, F.; Lookhart, G. L.; Pierce, R. O.; Seabourn, B. W.; Bean, S. R.; Wilson, J. D.; Chung, O. K. Predicting Wheat Quality Characteristics and Functionality Using Near-Infrared Spectroscopy. Cereal Chem. J. 2006, 83(5), 529–536. DOI: 10.1094/CC-83-0529.
- Mutlu, A. C.; Boyaci, I. H.; Genis, H. E.; Ozturk, R.; Basaran-Akgul, N.; Sanal, T.; Evlice, A. K. Prediction of Wheat Quality Parameters Using Near-Infrared Spectroscopy and Artificial Neural Networks. Eur. Food Res. Technol. 2011, 233(2), 267–274. DOI: 10.1007/s00217-011-1515-8.
- Jiang, H.; Liu, T.; Chen, Q. Quantitative Detection of Fatty Acid Value During Storage of Wheat Flour Based on a Portable Near-Infrared (NIR) Spectroscopy System. Infrared Phys. Technol. 2020, 109, 103423. DOI: 10.1016/j.infrared.2020.103423.
- Azizinia, S.; Mullan, D.; Rattey, A.; Godoy, J.; Robinson, H.; Moody, D.; Forrest, K.; Keeble-Gagnere, G.; Hayden, M. J.; Tibbits, J. F., et al. Improved Multi-Trait Prediction of Wheat End-Product Quality Traits by Integrating NIR-Predicted Phenotypes. Front. Plant Sci. 2023, 14, 1167221. DOI: 10.3389/fpls.2023.1167221.