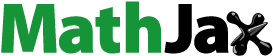
Abstract
Airbag pressure determines the restraint effect during a vehicle crash. The pressure required to restrain the occupant depends on pre-crash detection, collision parameters and the occupant’s mass and position. This work modulated airbag pressure for optimum safety using a novel airbag control valve for cold-gas inflators. This paper evaluates the valve’s stationary and dynamic performances for Helium by 3D flow simulations using a pressure-based solver in ANSYS Fluent® and SAE J2238 laboratory tank tests. The predicted and measured tank pressures for the fully open (stationary) valve were agreed by an average 93.73% with an excellent correlation (correlation coefficient, R = 0.9995). For the first dynamic operation with 10 ms switching time, the results agreed by 92.78% with R = 0.9975. In the second test with 30 ms switching, 83.67% agreement was observed with R = 0.9893. The research concluded that the valve modulates the bag pressure and is implementable in vehicles.
1. Introduction
Airbag energy management and restraint effect optimisation are two extensively researched topics in automotive passive safety with the final goal of mitigating occupant injuries. Combining seat belts and airbag reduces mortality by 67% [Citation1]. However, the current airbags are ‘one-size-fits-all’ systems. Hence, there is a discrepancy in protection for different percentile occupants [Citation2]. If an airbag deploys with high pressure, then the impact force on the occupant increases the probability of face, head or neck injuries [Citation1]. In addition to high pressure, if the occupant is in the bag deployment zone, then the likelihood of injury further increases [Citation2]. Therefore, the airbag’s energy and aggressiveness must be controlled for optimised occupant protection.
Current research mitigates airbag-induced injuries [Citation3–5]. According to US NCAP data, airbag triggers between 17 and 22.5 ms after the crash, based on the vehicle type and without pre-crash detection [Citation6,Citation7]. Radar and lidar technologies have fostered pre-crash detection and time-to-collision (TTC) estimation [Citation6]. In response to TTC and pre-crash sensing, the airbag is deployed in the first stage, and pressure is controlled thereafter based on vehicle sensor signals [Citation8]. If the airbag deploys earlier, then it requires slower inflation and longer standing time, which can be provided by dynamic pressure control.
Further, continuous crash severity prediction has evolved [Citation9]. The bag’s pressure has to be continuously adapted to mitigate the injuries in response to severity prediction. In contrast, the dual-stage airbags offer only improved two-step protection compared to a single-stage baseline inflator. They are better in terms of aggression and protection at high speeds [Citation3]. In addition to severity prediction, the occupant’s position (out-of-position) demands continuous pressure control for optimised protection and mitigated injuries. The occupant hits the vehicle structure if the bag pressure is too low, the problem in rearward seat occupancy. If the pressure is too high, then the probability of head injuries increases due to the airbag’s stiffness, commonly seen in forward seating positions [Citation3,Citation4,Citation10]. Hence, finding the correct pressure for different positions is crucial.
Furthermore, the airbag’s performance depends also on the occupant’s size and position combination. Sitting close to the dashboard, an elderly occupant (mass < 55 kg) requires less airbag pressure and later deployment than a young person (age < 30 years; mass > 80 kg). Hence, Wood’s research suggested occupant’s size- and mass-based pressure adaption [Citation11].
Radu et al. showed that the deployment time directly implicates upper-body injuries, particularly head injuries [Citation5]. Based on a 20 ms trigger time delay, the head injury criterion was increased by about 80% from the baseline test [Citation5]. Impulsive deployment yields higher injuries, too. This problem can be formulated as a delayed pressure rise rate, yielding a pressure control problem. Yang et al. emphasised the requirement for adaptive airbag deployment strategies by detecting the occupant’s pose and location through a computer vision system [Citation12]. The airbag can be partially or fully deployed according to the occupant’s size and position classification, which is a pressure control requirement.
Depowering of the airbag was permitted through Federal Motor Vehicle Safety Standard 208 (FMVSS 208) in 1997 to mitigate the injuries for small occupants and out-of-position situations [Citation2]. In an approach to reduce upper-body injuries, Hault-Dubrulle et al. used depowered airbags that usually consist of helium cold-gas inflators, which are 35% less aggressive than conventional pyrotechnic inflators [Citation2,Citation10,Citation13]. Cold-gas devices inflate with low temperatures and particle emissions. Hault-Dubrulle et al. shifted the paradigm from pyrotechnical towards cold-gas inflators to reduce aggressiveness and power.
In conclusion, controlling the airbag inflation and deflation (pressure) optimises the restraint effect. However, how to control the inflation source still needs to be determined. Wood categorised inflation sources for an airbag into pyrotechnic, cold-gas and hybrid [Citation11]. Pyrotechnic yielded 800 K exit temperature with particle emission and makes it undesirable to control [Citation14,Citation15]. Contrarily, cold-gas inflators with a fast-switching control valve provide output pressure control.
A servo airbag control valve developed by Robert Bosch GmbH modulated the cold-gas inflator pressure for different scenarios [Citation16]. Kästner et al. evaluated the valve’s performance by simulating the computational fluid dynamics (CFD) simulations for Nitrogen and performing tank tests. Controlling the pressure for multiple stages reduced injuries for different scenarios [Citation16]. Contrarily, the valve was operated based on a set of pre-defined activation patterns, which was the system’s downside. However, the system required airbag-occupant contact data to operate in a closed loop. Microsys developed an airbag test bench that consisted of an ultra-fast firing valve with a Helium cold-gas generator. The performance of the valve and pyrotechnic inflator were at par [Citation17].
Since the decision to fire the second stage in dual-stage airbag and cold-gas inflator closed-loop pressure control requires airbag-occupant contact data, Shirur et al. developed a tactile sensor for the airbag. The sensor detected contact and estimated contact time and area. The bag pressure can be controlled based on the contact feedback [Citation18,Citation19].
The critical variables for the restraint effect are pre-crash detection reliability and accuracy, occupant position and mass, airbag pressure and trigger time. These parameters relate to the airbag’s pressure, a dependent variable, while all other parameters are considered independent variables.
Considering the importance and requirement of pressure control, a novel controllable valve for inflating an airbag with cold gas is evaluated in this work. The valve resembles a plug nozzle [Citation20]. The valve’s performance was evaluated in two steps. Firstly, numerical 3D flow simulations were performed to obtain the tank and inlet pressure histories. In the second step, tank pressure histories from the laboratory tank tests were obtained and compared with the flow simulation results. The mass flow rate and temperatures from the simulations were also obtained, which are crucial for occupant safety simulations.
2. Novel control valve for airbags
The control valve comprises a gas inlet, three outlets and an actuator (). The inlet was connected to cool Helium inflators® filled at 660 bar and tailored for the present research application. Outlets 1 and 2 are the main gas outlets to the bag, while Outlet 3 balances the pressure on either side of the plunger. When the electromagnet is actuated, the plunger surface area difference perpendicular to the flow direction at the same pressure displaces the plunger to close the valve ().
Figure 1. Control valve concept: (a) valve’s normally open position allowing gas flow to the airbag or test tank; (b) force balance on the plunger leading to valve’s actuation; (c) actuated valve leading to closed position.

The inflator bursts open on the trigger, and the gas flows through the inlet. The valve is normally in the open position (), allowing the gas to flow towards the airbag through Outlets 1, 2 and 3. The gas exerts a higher force from the right side FR as from the left side FL (FL < FR) and keeps the valve open by displacing the plunger to the left (). A fully open valve yields a conventional airbag without pressure modulation.
However, if the electromagnetic actuator closes Outlet 3, then the pressure on either side of the plunger is balanced. Since the area on the left side, AL, is larger than that on the right side, AR, FL is larger than FR at the same pressure. The force imbalance () moves the plunger and closes the valve (). The valve’s opening and closing are controlled by the diameter d1 of the hole: the larger the hole, the shorter the closing time. The hole diameter determines the valve’s switching time as well.
3. Material and methods
3.1. Mathematical flow model
Helium was expanded from 660 bar to 1 bar in tank tests. A high-pressure gradient resulted in a 3D compressible flow showing real gas effects: it was turbulent and isentropic, i.e. the entropy remained constant as the temperature changed. The flow problem was numerically solved using commercial software (Fluent®, Ansys Inc., Canonsburg, PA, USA) and validated with laboratory tank tests.
At first, continuity, momentum and energy equations were solved, followed by their scalar equations:
Continuity equation: Mass conservation law was applied to a finite volume cell and the continuity equation was derived in the partial differential form [Citation14,Citation21,Citation22] as follows:
Momentum (Navier–Stokes) equation: Following continuity, Newton’s second law was applied to the finite fluid volume in the fluid domain leading to EquationEquation (2)
(2)
(2) . The body (weight of the fluid) and surface (viscous and pressure) forces cause momentum in fluids [Citation14,Citation21,Citation22].
(2)
(2)
Here, is the stress tensor,
the velocity vector and I the identity matrix. μ and λ denote molecular viscosity and second viscosity coefficient, respectively.
Energy equation: Lastly, the energy equation was solved applying the energy balance [Citation14,Citation21,Citation22]
Turbulence model: The Spalart–Allmaras model is a simple, robust and numerically fast one-equation model used to model turbulence. As the filling impacts the performance, the transport equation for the working variable
yields the following equation [Citation23–25]:
(4)
(4)
Pressure equation-of-state (EOS): Redlich–Kwong real-gas equation was applied to calculate the pressure [Citation23,Citation26]:
(5)
(5)
3.2. 3D-CFD simulation
shows a simplified quarter model of the control valve and the inflator mounted in the tank. The inner flow volume from the assembled model was extracted and meshed using Ansys workbench (ANSYS Inc., Canonsburg, PA, USA). It was meshed linearly except for the region near the plunger (). The tetrahedral mesh was used in this work for the plunger and its surrounding parts for easier mesh deformation during plunger movement to actuate the valve. The mesh had approximately 152,000 elements in the quarter model.
Figure 2. Simplified geometry and mesh model: (a) the extracted flow volume of the control valve, inflator and the tank and (b) the meshed model and zoomed view of the control valve region.

All model components were initialised with 1 bar and 291 K; laboratory atmospheric conditions assuming helium as a working gas. The patching method with 660 bar was applied to the inflator pressure to initialise the flow with adiabatic walls under no-slip conditions. The model was simulated for 120 timesteps with 0.5 ms time-step size.
An explicit pressure-based solver in 3D was used to solve the governing equations. Pressure–velocity coupling was obtained with a semi-implicit method for pressure-linked equations (SIMPLE) algorithm [Citation23]. First, pressure and velocity fields were solved, then the scalar properties like temperature and turbulence were derived. First-order discretisation was applied and then the pressure was obtained using EquationEquation (5)(5)
(5) to simplify the problem. Solution control parameters (under relaxation parameters) were eased to answer the high-pressure gradient and obtain convergence. Density relaxation was set to 0.3, keeping the pressure relaxation unchanged (0.3). Modified turbulent viscosity and turbulent viscosity were reduced to 0.5, with energy relaxation equal to 0.6. The mesh was deformed at a given time to move the plunger accordingly, which simulated the valve’s opening and closing.
3.3. Laboratory tank tests
Modified SAE J2238 ballistic tank tests were performed to evaluate the valve’s performance [Citation27]. The airbag control valve (ACV) along with a cold-gas inflator (660 bar) was mounted inside the tank () and two pressure sensors at the inlet and the tank were used to record the pressure. Tank and inlet pressures were measured by expanding helium from 660 to 1 bar.
3.4. Evaluation matrix
Flow simulations and laboratory tank tests were utilised to evaluate the valve’s performance. shows the evaluation matrix. Firstly, a completely open configuration was tested to obtain a pressure history and compare it with the series inflator. Then, two switching times were selected such that the bag has at least 1 bar pressure at 60 ms. Otherwise, the occupant might hit the vehicle structure. This yielded 10 and 30 ms.
Table 1. Control valve performance evaluation matrix.
3.5. Data analysis method
According to the SAE standard, recorded pressure signals were filtered with a channel frequency class 60 filter. Then linear regression was applied to the pressure values obtained by tests and simulations [Citation27]. Correlation between measured and predicted tank pressure was established by estimating Pearson’s correlation coefficient using the following equation [Citation28]:
(6)
(6)
where xi and yi are measured and predicted pressures, respectively, while
and
are the mean values. R = 1 indicates an excellent correlation. It gives the strength of association between the measured and predicted values and determines how the model agrees with the test results.
Further, the relative agreement between the measured and predicted values was calculated using
(7)
(7)
3.6. Hypothesis
It is hypothesised that the fully open control valve with the cold-gas inflator yields an exponential increase in the tank pressure like a series cold-gas inflator. Further, when the valve is closed during the inflation, the tank pressure remains constant and rises exponentially when the valve is opened again.
Therefore, the tank and inlet pressures for tailored research cold-gas inflators were measured with an adapter for pressure measurement. However, the inlet pressure for the series cold-gas inflator was not measured since modification of series cold-gas inflators was not permitted due to safety reasons.
4. Results and discussion
As hypothesised, the tank pressure for the research inflator with the valve in the stationary open position increased exponentially like a series cold-gas inflator. No anomaly was observed in the behaviour. Further, when the valve was closed, the tank pressure was constant and increased exponentially once the valve was opened again ().
Figure 4. Tank pressure comparison for predicted and measured values: (a) series cold-gas inflator; (b) measured and predicted tank pressure for research inflator with fully open valve; (c) measured and predicted tank pressure for research inflator with valve closing for 10 ms; (d) measured and predicted tank pressure for research inflator with valve closing for 30 ms.

4.1. Tank pressure
The tank pressure in laboratory tests increased exponentially and attained 1.13 bar peak pressure at 60 ms for series cold-gas inflators (). For Test 1 (stationary open) also, the tank pressure increased exponentially with 1.41 bar peak pressure at 60 ms, while the CFD model predicted 1.41 bar (). The CFD model showed excellent fidelity for stationary open configuration. The research inflator (660 bar) tailored for this work had 20% higher pressure than series inflators (550 bar), which was observed in the tank pressure as well. The comparison indicates that the control valve in the open position has similar pressure history to a series inflator.
Test 2 in was the first dynamic operation assessment of the valve by closing it between 30 and 40 ms for 10 ms. The gas expanded exponentially, and the pressure remained constant upon closing the valve. On opening the valve again, the pressure increased as expected. The measured peak pressure was 1.37 bar, whereas the model predicted pressure was 1.38 bar. Despite optimised under relaxation parameters in solution control, higher tank pressure in the simulation till 30 ms was observed, after that simulation found good agreement with the measured pressure. The initial deviation (till 30 ms) is attributed to the pressure difference between the tank and the inflator, which resulted in the supersonic flow. A local mesh refinement technique is required to address supersonic flow at the interface between the valve’s exit and the tank. However, this work aimed to obtain tank pressure history, which is required for the safety simulations. In addition, the restraint effect begins after 40 ms when the occupant contacts the bag; until this time, bag or tank pressure deviation does not affect the occupant’s safety, but only deployment behaviour changes.
illustrates tank pressure for Test and Simulation 3, which was the second dynamic assessment of the valve by closing it for longer than in Test 2. The valve was actuated between 20 ms and 50 ms. The measured tank pressure at 60 ms was 1.05 bar whereas the model predicted 1.19 bar. Similar to previous tests, the pressure increased until the valve was closed, showing no deviation. However, when the valve was closed, the simulation showed rising tank pressure indicating leakage from the plunger. The geometry conflict was the limiting factor, which prevented 100% closing of the valve. A small gap had to be maintained to differentiate two model components, otherwise resulting in the single component conflicting with the model integrity. Hence, even during valve closing time, the tank pressure increased continuously, leading to low model fidelity for a longer closing time.
The tank pressure histories for the series inflator and fully open valve obtained in this work are similar to the findings of Slaats et al. [Citation17]. Further, the pressure histories with control valve actuation are identical to the results obtained by Kästner et al. [Citation16]. Their research found exponential filling behaviour followed by constant pressure during valve closing.
Further, linear regression was applied to measured and simulated pressures to obtain the correlation. The predicted tank pressure exhibited excellent correlation (R = 0.9995) to the measured pressure (), indicating the high reliability of the CFD model for the stationary open valve in Test 1 and Simulation 1. Although an excellent correlation (R = 0.9975) was noticed for Test 2 and Simulation 2, it was reduced slightly compared to Test and Simulation 1. The correlation coefficient decreased to R = 0.9893 for Test 3 and Simulation 3, showing low model fidelity for longer closing times. The lower correlation with longer closing times is attributed to the rising tank pressure in the simulations. When the valve was not fully closed, there was a supersonic leakage in the tank through the plunger, which resulted in higher pressure. Decoupling the simulations between valve opening and closing times is one possible solution to overcome the leakage, which will increase the model fidelity.
Figure 5. Correlation between measured and predicted tank pressures for the stationary and dynamic valve: (a) Correlation for Test 1 – Simulation 1; (b) Correlation for Test 2 – Simulation 2; (c) Correlation for Test 3 – Simulation 3.

presents the relative percentage agreement between measured and predicted tank pressures for different time steps calculated from EquationEquation (7)(7)
(7) . The CFD model agreed with the measurements by an average 93.73% for Test 1. For Tests 2 and 3, the predicted pressures agreed with the tests by 92.78% and 83.67%, respectively. In contrast to the agreement from Tests 1 and 2, the agreement in Test 3 dropped due to higher predicted pressures when the valve was closed. For stationary open valve and shorter switching time (Test 1 – Simulation 1 and Test 2 – Simulation 2), the relative agreement was lower till 20 ms and then increased (
), which was attributed to transient developing flow till 20 ms and steady flow after that. However, in test and simulation 3, the flow started with the transient phase with low relative agreement and increased until the valve closed. Contrarily, leakage in the valve after 20 ms (closing time) resulted again in the lower agreement. Since the valve was closed earlier than in Test 2, the inlet pressure was still higher than in Test 2. Hence, leakage was also higher.
4.2. Inlet and tank temperatures
Temperature measurement during the tests was challenging due to the sampling rate limitation of the commercially available temperature sensors and heat transfer between gas and sensor jacket, which records lower gas temperature than the actual gas temperature. Mounting one or two sensors on the tank does not provide accurate average gas temperature; only local point temperature is measured. Hence, the average gas temperature was obtained from the simulation by validating pressure. shows the volume-averaged gas temperature for the inlet and the tank obtained from the CFD simulations. Upon opening the inflator, the temperature at the inlet increased to 462 K due to high velocity (velocity ) throttling and dropped to 150 K in the steady state due to the cooling effect. In all simulations, a similar inlet gas temperature drop was noticed ().
Figure 7. Inlet and tank temperature: (a) volume averaged maximum inlet temperature was 462 K and (b) volume averaged maximum tank temperature was 368 K.

In contrast to the inlet, the tank gas temperature () increased with the pressure rise and reached a maximum of 368 K between 15 and 25 ms from atmospheric 291 K and started to drop after that as a result of cooling effect and heat transfer between gas and the tank surface. The recorded tank gas temperature from the literature for a pyrotechnic inflator was approximately 500 K, which was 36% higher compared to a cold-gas inflator what was noticed in the present research [Citation14]. The lower gas temperature has an advantage in occupant protection. Lowering the temperature reduces the exhaust velocity through the bag pores and the vent holes, which increases the airbag standing time, resulting in prolonged protection time.
4.3. Inlet pressure
In contrast to the exponentially rising tank pressure, the inlet pressure dropped exponentially (). The measured initial inlet peak pressure for Test 1 was 531 bar, while the simulation predicted 559 bar (). Closing the valve in Test 2 (10 ms closed valve) resulted in 536 bar initial peak pressure while the model predicted 560 bar (). Further, in Test 3 (30 ms closed valve), the recorded test bench inlet pressure was 478 bar with the model predicting 560 bar (. In performed tests and simulations, there was a discrepancy in the initial peak pressure. The sensor took 2.5 ms to record the first rise. The delay in pressure rise resulted from the slower response time from the sensor. However, higher initial pressure was predicted in the simulations since the volume averaged pressure is estimated for every time step. In addition, there were pressure fluctuations and peak pressure variation in the measured pressure () until initial 5 ms and during the valve closing. The authors of this work speculate that these fluctuations have resulted from the shock waves arising from the supersonic inflator flow and influenced the sensor. On the other hand, the tank pressure till 20 ms was the same for all measured tests. This finding suggested that the discrepancy in the inlet peak pressures has resulted from the senor measurement but not the physical effect or the leakage.
Figure 8. Inlet pressure comparison. Measured and predicted inlet pressure for research inflator with valve: (a) fully open valve; (b) valve closing for 10 ms; (c) valve closing for 30 ms.

Similar to the tank pressure, a high correlation coefficient for the inlet pressures was noticed (). Test 1 and Simulation 1 exhibit excellent correlation (R = 0.9997). The R values for Test 2 – Simulation 2 and Test 3 – Simulation 3 were 0.9987 and 0.9859, respectively.
Figure 9. Correlation between measured and predicted inlet pressures for the stationary and dynamic valve: (a) correlation for Test 1 – Simulation 1; (b) correlation for Test 2 – Simulation 2; (c) correlation for Test 3 – Simulation 3.

Contrary to the tank pressure, the relative agreement between measured and estimated pressures in the transient phase () for all the tests and simulations was greater than 90% and the average agreement was higher than 92%. The predicted inlet pressure () was found to agree by 95.62% for Test 1. Similarly, 95.49% and 92.30% agreement was observed for Tests 2 and 3, respectively. By comparing the agreement for the tank () and inlet (), a higher agreement was observed between the model and measured values at the inlet than the tank pressures, which suggested that leakage in the tank did not influence the inlet pressure modelling.
4.4. Mass flow rate
illustrates the mass flow rates obtained from the simulations. As soon as the valve was opened, the initial mass flow rate (MFR) was 0.08 kg/s until the full flow development and then reached a peak 0.09 kg/s in steady flow condition. The MFR dropped exponentially with the gas expansion in the tank. All simulations exhibited the same mass flow behaviour until the valve was closed (). The MFR decreased and remained constant when the valve was closed. Interestingly, there was mass flow () even for a closed valve, indicating a leakage in the flow domain. Ideally, there should not be any mass flow when the valve is closed. As explained in Section 4.1, the leakage through the plunger was also observed in the mass flow rate shown in . As expected, the stationary open valve resulted in a continuous drop in the MFR. However, when the valve was opened after closing for 10 ms, the MFR (0.028 kg/s) was higher than Simulation 1 (0.023 kg/s) at the same time (50 ms). Further, longer closing time in Simulation 3 yielded even higher MFR after opening, which was 0.046 kg/s. Due to uncontrolled deployment, the MFR in Simulation 1 leads to faster airbag deflation offering shorter protection time. Simulation 2 MFR is useful to deploy the bag earlier and inflate again when the occupant contacts the bag, while pre-crash information can be used to deploy the bag in the pre-crash phase and control the pressure for a longer standing time. The MFR results obtained in the present research are analogous to the findings of Kästner et al. [Citation16]. In conventional pyrotechnic inflators, the maximum mass flow rate of gas mixtures was between 1.2 and 1.6 kg/s [Citation1]. This work used helium, which is a light gas. Despite lower MFR than pyrotechnic inflators, it offers similar filling characteristics due to its velocity (approximately 1800 m/s), which is higher than for nitrogen (approximately 800 m/s).
4.5. Controlled airbag inflation
All the test tank pressures are compared and presented in showing inflation pressure control. The baseline pressure was set to 1 bar and the time required to reach 1 bar was compared, which is crucial to decide the right bag pressure for optimal occupant protection for different scenarios. When the valve was fully open, the time required was 26 ms, whereas when the valve was fully closed (Test 3), the time required to reach 1 bar was 57 ms.
As mentioned in Section 1, the airbag inflation pressure must be controlled to optimise the restraint effect for different percentile occupants, positions and collision time-frames (pre-crash and in-crash). It is evident from that the control valve developed in this work can control the pressure. Three pressure history curves () can be applied to the following scenarios.
Scenario 1: No pre-crash information available.
Suppose the airbag has to deploy without pre-crash information and optimisation. In this case, the valve can be kept open (Test 1 in ) after the trigger, which is similar to igniting a pyrotechnic inflator. In this configuration, the control valve with a cold-gas inflator offers the same protection with extended time since the deflation is slow. However, the trigger time can be adjusted to control the pressure as per requirements and the interest of implementation.
Scenario 2: Optimisation for in-crash phase.
In-crash pressure modulation is crucial to minimise the occupant rebound after the impact with the airbag. Here, lowering the pressure rise rate is beneficial to reduce the rebound velocity. Thereby, the chance of hitting the headrest can be mitigated. Accordingly, the pressure curve in Test 2 can be applied in real time to mitigate the injuries. From , it can be observed that until 30 ms, the pressure rise is the same, which provides the same unfolding and inflation time. However, after 30 ms, since the pressure rise rate is reduced, momentary softness is created in the bag, which allows larger displacements reducing the deceleration rate. Although 10 ms valve closing resulted in 0.031 bar peak pressure difference, it is beneficial in reducing the bag stiffness during the ride-down phase. Contrarily, if the pressure is decreased beyond the pre-defined threshold, then the airbag will bottom out, and the occupant will hit the vehicle structure. Hence, this application requires careful analysis of the requirements through occupant safety simulations and corresponding validation tests.
Scenario 3: Pre-crash information with out-of-position.
Occupants’ situational awareness reduces due to belief in automation, which leads to out-of-position poses. In such cases, the airbag can be deployed and kept at a lower pressure until the occupant contacts the airbag. The pressure can be increased after contact. The control strategy of Test 3 can be applied to inflate the airbag with a minimum pressure of 0.9 bar at 25 ms. When the occupant impacts at 50 ms, the pressure can be increased again. Airbag-mounted contact sensor can be helpful in this context to identify the occupant contact time and estimate the area. In previous work of this research, a tactile contact sensor was developed that can be integrated with the airbag [Citation18].
The occupant’s rebound velocity increases if the bag is stiff (higher pressure), resulting in an injury probability increase arising from impact with the headrest. The pressure difference at 60 ms for different tests is approximately 320 mbar. For the same scenario, the pressure curve in Test 3 yields fewer injuries than in Tests 1 and 2, considering the airbag will not bottom out.
There was 31.34 ms time difference at 1 bar for different tests. is crucial when TTC and pre-crash information are available. From the baseline, the pressure can be scaled for optimum safety in the time domain by
based on pre-crash and occupant information, which requires crash and occupant safety simulations.
In summary, the standalone CFD model showed good fidelity with test results for the open valve. However, the geometric model needs improvement for closing the valve and avoiding leakage, which can be solved by decoupling the simulations. There was an excellent correlation between the model and the laboratory tests. However, crash and occupant safety simulations are required to determine the valve’s actuation timings, trigger times and inflator pressure.
5. Conclusion
The novel valve design provides an opportunity to control the airbag’s inflation in real-time. The valve can be actuated during the in-crash phase at different times with pre-crash information to regulate the pressure for diverse anthropometries. If pre-crash information is unavailable, then the valve trigger time can be adjusted based on the crash severity estimation. Suppose the deployment time is not a variable. In that case, the airbag still offers better protection due to the slower deflation of the cold gas compared to the faster deflation of the pyrotechnic airbag. If needed, the pressure can then be controlled based on occupant contact with the bag provided by the textile sensor mounted on the bag. In addition, it improves the protection for standard and out-of-position poses for diverse anthropometries with pressure modulation and addresses the limitation of present pyrotechnic inflators. Further, the article also presented inflation strategies for pre-crash detection, out-of-position poses and different sizes of occupants. These findings rely on both comprehensive simulations and experimental verification.
Disclosure statement
All the authors declare no conflict of interest.
Additional information
Funding
References
- Zhou H, Zhong Z, Hu M. Design and occupant-protection performance analysis of a new tubular driver airbag. Engineering. 2018;4(2):291–297. doi: 10.1016/j.eng.2018.03.015.
- Phen RL, Dowdy MW, Ebbeler DH, et al. Advanced airbag technology assessment. Pasadena (CA): JPL Publication; 1998.
- Hollowell WT, Summers LK, Prasad A, et al. Performance evaluation of dual stage passenger air bag systems. In: Proceedings of the 17th international technical conference on the enhanced safety of vehicles, Amsterdam, Netherlands, 2001.
- Yao J, Yang J. Concept design of an a-pillar mounted airbag for pedestrian head protection. In: Proceedings of the 6th European LS-DYNA conference, 2007.
- Radu AI, Cofaru C, Tolea B, et al. Study regarding the influence of airbag deployment time on the occupant injury level during a frontal vehicle collision. MATEC Web Conf. 2018;184:01007. doi: 10.1051/matecconf/201818401007.
- Desrosiers D, Birdsong C, Schuster P. A pre-crash simulator to evaluate vehicle collision prediction algorithms. IFAC Proc Vol. 2007;40(10):531–538. doi: 10.3182/20070820-3-US-2918.00072.
- Martin GY. Airbag triggering in a numerical vehicle fleet [master’s thesis]. Eindhoven: Eindhoven University of Technology; 2004.
- Cuddihy M, Rao MK, Hall I. Method for operating a pre-crash sensing system to deploy airbags using inflation control. Google Patents, 2013.
- Sala DM, Wang JT. Continuously predicting crash severity. Washington DC: National Highway Traffic Safety Administration; 2003.
- Hault-Dubrulle A, Robache F, Drazetic P, et al. Determination of pre-impact occupant postures and analysis of consequences on injury outcome–part II: biomechanical study. Accid Anal Prev. 2011;43(1):75–81. doi: 10.1016/j.aap.2010.07.013.
- Wood R. Characterisation of particulate matter originating from automotive occupant restraints [PhD thesis]. Oxford: Oxford Brookes University; 2013.
- Yang Y, Zao G, Sheng J. Occupant pose and location detect for intelligent airbag system based on computer vision,Fourth International Conference on Natural Computation, Jinan Shandong, China. IEEE Los Alamitos, CA, USA; 2008.
- Virginia Jernigan M, Duma SM. The effects of depowered airbags on severe upper extremity injuries in frontal automobile crashes. In: Annals of advances in automotive medicine/annual scientific conference, Lisbon, Portugal, September 22-24 2003, p. 601–605.
- Cheng C, Zhang X, Wang C, et al. Numerical investigation on cooling performance of filter in a pyrotechnic gas generator. Defence Technol. 2021;17(2):343–351. doi: 10.1016/j.dt.2020.01.004.
- Hsieh WH, Sun LY, Chen JK, et al. Theoretical simulation of combustion processes of airbag inflators. J Autom Eng. 2001;215(1):1–9. doi: 10.1243/0954407011525386.
- Kästner F, Heyer K, Iben U, et al. Gas control valve for enhanced adaptivity of airbag inflators. Pfinzta: Fraunhofer ICT; 2014.
- Slaats PM, Pitonyak AJ, Wipasuramonton P, et al. Alternative airbag evaluation methodology through cold gas inflation system. Warrendale, PA: SAE International; 2008.
- Shirur N, Birkner C, Henze R, et al. Effect of airbag deployment phases on tactile occupant detection sensor, XII International Science-Technical Conference AUTOMOTIVE SAFETY,21-23 Oct. 2020, Kielce, Poland. IEEE, Kielce, Poland; 2020.
- Shirur N, Birkner C, Henze R, et al. Tactile occupant detection sensor for automotive airbag. Energies. 2021;14(17):5288. doi: 10.3390/en14175288.
- Rommel T, Hagemann G, Schley CA, et al. Plug nozzle flowfield analysis. J Propul Power. 1997;13(5):629–634. doi: 10.2514/2.5227.
- John David A. Computational fluid dynamics. McGraw-Hill series in aeronautical and aerospace engineering. McGraw-Hill Book Co, Singapore; 1995.
- Péneau F, Pedro G, Oshkai P, et al. Transient supersonic release of hydrogen from a high pressure vessel: a computational analysis. Int. J. Hydrogen Energy. 2009;34(14):5817–5827. doi: 10.1016/j.ijhydene.2009.05.090.
- ANSYS Fluent Theory Guide. [cited2013 Nov]. Available from: http://www.pmt.usp.br/academic/martoran/notasmodelosgrad/ANSYS%20Fluent%20Theory%20Guide%2015.pdf.
- Kostić C. Review of the Spalart-Allmaras turbulence model and its modifications to three-dimensional supersonic configurations. Sci Tech Rev. 2015;65(1):43–49. doi: 10.5937/STR1501043K.
- Nichols RH. Turbulence models and their application to complex flows. Birmingham (AL): University of Alabama at Birmingham; 2010.
- Melideo D, Baraldi D, Acosta-Iborra B, et al. CFD simulations of filling and emptying of hydrogen tanks. Int J Hydrogen Energy. 2017;42(11):7304–7313. doi: 10.1016/j.ijhydene.2016.05.262.
- Airbag inflator ballistic tank test procedure gas generators used in inflatable restraint systems. Warrendale, PA: SAE International; 2012.
- Asuero AG, Sayago A, González AG. The correlation coefficient: an overview. Crit Rev Analyt Chem. 2006;36(1):41–59. doi: 10.1080/10408340500526766.