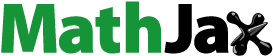
ABSTRACT
Mental disorders threaten the chances of finishing secondary school and can hinder the school-to-work transitions of afflicted youths. Earlier onset depression predicts the chronicity, recurrence, and severity of episodes throughout life. Using rich, objective mental health data and a battery of variables covering personal and family characteristics, we investigate the impact of Norway’s vocational rehabilitation programmes on youths aged 18–23 who are registered as unemployed. Our results indicate that the impact vary with mental health in adolescence, a variable often unavailable in such analyses. Separate analyses for age group 19–21 and 20–23 show that the younger ones with previous diagnosed mental disorders were likely to pursue further education after participating in programmes providing work practice; those with no earlier diagnosed mental disorders were prone to pursue ordinary education after completing a training programme. For the older age cohorts, aged 20–23, vocational rehabilitation programmes seem counterproductive, irrespective of programme type and of whether they were diagnosed with mental health problems in adolescence or not.
Introduction
Mental illness refers to a wide range of mental health conditions – disorders that affect your mood, thinking and behaviour, such as depression, anxiety disorders, schizophrenia, eating disorders and addictive behaviours. Four of the six leading causes of prolonged disability are due to neuropsychiatric disorders (WHO Citation2004). Mental illness incurs huge costs to society, to the individual, the close environment, and the economy. The Organisation for Economic Co-operation and Development (OECD) (OECD Citation2018) estimated the total cost related to mental illness in 2015 to be more than 4% of the GDP – or over EUR 600 billion – across the 28 EU countries. Sobocki et al. (Citation2006) estimate depression to be the costliest mental disorder in Europe, accounting for 33% of the total cost of mental illness, corresponding to 1% of the total economy (GDP) of Europe. Much of the economic costs are derived from workers’ reduced productivity, measured in terms of absenteeism and reduced productivity on the job (Bubonya, Cobb-Clark, and Wooden Citation2017). Even minor levels of depression are associated with loss of productivity (Beck et al. Citation2011). Mental illness also affects the likelihood of getting and keeping a job (Veldman et al. Citation2018), and unemployment is likely to worsen mental health (Browning and Heinesen Citation2012). The long-term effects of mental health deterioration on a population can be dramatic. For example, in the last couple of decades, increased mortality and morbidity related to mental health deterioration among white low-educated Americans has led to a fall in registered life expectancy for the first time ever in the US (Case and Deaton Citation2020).
Mental health problems start very early in life. The typical age at onset of anxiety disorders is 11 years old, with a distribution (25th-75th percentile) of 6 to 21 years. For any mental disorder the equivalent figures is 14 years, with a distribution of 7–24 years (OECD Citation2012). The number of registered patients with mental illness is rising, particularly among young people (Bor et al. Citation2014; Twenge et al. Citation2019). A mental disorder (MD) is one of several mechanisms that can lead to adverse secondary school outcomes and subsequent reduced work capacities. A childhood MD can negatively influence the development of a child’s cognitive abilities, which, in turn, affects the child’s school performance (Guo and Harris Citation2000). The relationship between mental health and educational attainment is complex. It is hard to know whether MDs have a direct adverse effect on educational attainment or if another confounding factor is responsible (Hale, Bevilacqua, and Viner Citation2015). Youth unemployment is associated with psychological symptoms, such as depression and loss of confidence (Paul and Moser Citation2009); hence, early targeted prevention efforts are crucial (Mendelson and Eaton Citation2018; Taylor et al. Citation2017). ‘OECD governments increasingly recognize that policy has a major role to play in improving the employment opportunities for people with mental disorders, including very young people’.Footnote1 There is concern that increasing reliance on digital technology, especially during the COVID-19 pandemic, may exacerbate MDs. Psychological support as an element of job search programmes is seen to positively influence motivation, confidence and other measures of mental health, as well as re-employment probabilities (Vuori and Vinokur Citation2005).
Norway serves as an interesting case. About 30% of young people in Norway between the ages of 15 and 24 report that they suffer from an MD, a share which is unmatched by other OECD countries (OECD Citation2013). Norway’s proportion of young people receiving health-related welfare benefits has increased substantially in recent decades, and this level is now the highest among the OECD countries (OECD Citation2013). The majority of these youths suffer from MDs. Moreover, the Scandinavian countries have experienced the highest increase since the mid-1990s of self-reported MDs, with Norway in the lead (von Simson, Brekke, and Hardoy Citation2022). MDs in adolescence are associated with only a 30–40% likelihood of completing secondary school (von Simson, Brekke, and Hardoy Citation2022).
Using longitudinal register data, we examined the impact of Norway’s vocational rehabilitation programmes (VRPs) targeted at work-impaired unemployed people from the ages of 18 to 23 in the period 2010–2014. We followed the youths for a maximum of six years. One common challenge of many studies on the impact of labour market programmes is the lack of an objective measure of mental health prior to the event under study. Health data is seldom available, and when it is, it is often subjective and associated with the contemporary health situation of the patient (Dean et al. Citation2017). A salient feature of this study is that we have access to information regarding mental health conditions in adolescence. Access to this information has major advantages. First, these data enable us to deal with issues of endogeneity and reverse causality that threaten the identification of causal effects by allowing the isolation of the impact of health on labour market exclusion and subsequent employment probability. By studying the impact of VRPs separately by mental health status in adolescence, we advance our understanding of how VRPs work for different groups depending on their propensity to have MDs. Second, we have data from a stage in life when a significant share of MDs tends to develop. Longitudinal studies show that early onset MDs rarely remit spontaneously and contribute to explaining the burden of MD in adulthood (de Graaf et al. Citation2012; Wittchen et al. Citation2011). Third, most empirical MD studies rely on subjective survey data on mental health and are hence subject to measurement error. Although both the subject and people in the close environment can select/self-select to treatment, register data is less prone to sampling error and selection bias (Thygesen and Ersbøll Citation2014). Another advantage is that we have data on the former diagnoses of MD from adolescence and need not rely on ex-post self-reports by the subjects. Access to adolescent diagnoses permits us to investigate how the impact of VRP varies by type of MD diagnosis, which to our knowledge has not been done before.
To investigate the causal impact of VRP, we applied a multivariate proportional hazard rate model with competing risks. Our sample is comprised of youths aged 18–23 who register as openly unemployed at the local labour market office and are assessed to have a work impairment. High-quality panel data makes it possible to follow the youth from unemployment, through their transitions to and from VRP, and finally out of unemployment, while controlling for a rich set of explanatory variables. Our findings reflect that lack of information on earlier MDs can give a misleading understanding of what works for whom after programme completion, particularly for more vulnerable youths with earlier MD onsets. Specific analyses of the youngest cohort aged 18–21 (and mostly) school dropouts, strengthen these results.
The paper proceeds as follows. First, we provide a review of the literature on VRPs targeted at youth, followed by a brief account of the Norwegian context. Next, we describe our estimation approach and the data at hand. Finally, we present the results, summarise the findings, and conclude.
Previous research
The international literature on the impact of VRP in Europe and the US for the population at large is inconclusive. Below, we review the literature considered rigorous enough to suggest or ascertain whether impacts can be identified. Training seems to have a negative impact in Denmark (Høgelund and Holm Citation2006) and a positive impact in Sweden (Frölich, Heshmati, and Lechner Citation2004). Employment programmes have very mixed effects (Bewley, Dorsett, and Salis Citation2009; Graversen and Jensen Citation2010). Early intervention in the form of mapping, counselling and motivation has mostly positive effects (Høgelund, Holm, and McIntosh Citation2010) and aligns with research results for youth in general (Maibom, Rosholm, and Svarer Citation2017) and the EU recommendations concerning the youth guarantee launched in all member states in 2013.
Few programme evaluations for the population at large have access to health data, and none focusing on young people in particular. One exception is a study by Dean et al. (Citation2017) that found a positive employment effect from work-related programmes in the US, but no impact from classroom courses. Furthermore, supported employment measures, which entail medical support as part of the treatment, seem to have positive employment effects in the US (Drake et al. Citation2009), but less positive employment effects in Europe (Burns et al. Citation2007). However, supported employment measures in the US have been mainly targeted at people with serious MDs, which is not the core target group of VRPs in Europe.
A few rigorous studies have been carried out in Norway, but none differentiate the impact of programmes by diagnosis. The overall picture is that VRPs enhance employability and education, with ordinary education having better results than other qualifying programmes, and that subsidised employment in ordinary firms is more advantageous than employment programmes in sheltered sector firms (Markussen and Røed Citation2014). The only Norwegian comparative study of VRPs focusing on youth (von Simson and Hardoy Citation2020) covers a much broader age group, youth aged 18–29, and has the shortcoming that it does not account for eventual mental health problems in adolescence and hence is not able to differentiate eventual health problems caused by unemployment from those causing unemployment. It concludes that wage subsidies work better for youth than classroom courses, while follow-up initiatives and work practice appear to be used as screening devices and springboards to working life.Footnote2
The Norwegian context
Unemployment and VRPs
Norway’s overall unemployment is low, and so is its youth unemployment. Youth unemployment for the 18–24 age group has fluctuated between 8–10% over the last 15 years. The work impaired unemployed is a subgroup of the registered unemployed, available for and seeking work. There are two streams of work impairment. Those entering work impairment from employment have a general practitioners’ (GP) certificate and have most often been through a period of sick leave and rehabilitation. According to the legislation, however, a GP certificate is not a prerequisite for a work impairment to be registered. The status of work impairment can also be given by a supervisor at the Labour and Welfare Service (NAV) office. This status requires a documented work capacity assessment with no lowest limit on the degree of reduced working capacity. Note that the client’s health history is confidential, and hence the case worker is ignorant of any past mental health diagnosis. The work capacity assessment is the basis for further follow-up and type of labour market measures available to them. Only work-impaired registered as unemployed at the local employment office qualify to VRPs.
Educational system
Primary and secondary education in Norway is free of charge, and the first 10 years is compulsory. Upper secondary school is normally 3 years for those qualifying for tertiary education and normally 3–5 years for those following the vocational track. Most youth (95%) start upper secondary school at the age of 16, However, about 25% of youth do not complete upper secondary school within the first five years. Those who do not are categorised as school dropouts. Most tertiary education is also free of charge, and students are entitled to student loans and grants.
Health care services
Norway’s highly developed universal health system provides publicly funded healthcare services to all people residing in Norway. GPs are often the first point of contact. The GP can also refer children and adolescents with more severe mental health problems to a specialist healthcare service for further treatment and follow-up care, consisting of child and adolescent psychiatric outpatient clinics, child and adolescent psychiatric wards, family units and psychiatric youth teams. All treatment and contact with specialist mental healthcare services are registered in the Norwegian Patient Registry (NPR).
Econometric method
An important issue in all non-experimental treatment evaluation analyses is the potential for selection bias. For example, if programme participants have characteristics unseen by the evaluator and difficult to measure, such as motivation or a drive, which give them an advantage in terms of labour market prospects irrespective of programme participation, a pure selection effect may be wrongly interpreted as a positive programme effect. We use the timing-of-events (ToE) approach formalised by Abbring and Van den Berg (Citation2003) to identify the causal effects of VRPs on subsequent transitions. This framework exploits information on the timing of events to distinguish causal effects from selection effects. For instance, if a VRP participant gets a job immediately after exiting the programme, it is more likely that the participation has had an effect on the employment probability than if it takes the participant a long time after VRP completion to get a job. In addition, assignment to treatment is modelled jointly with the outcome of treatment as a competing risk hazard rate. Selection effects are then explicitly controlled for by allowing the unobserved determinants associated with each hazard rate to be correlated. Lombardi et al. (Citation2021) and Gaure et al. (Citation2007) use Monte Carlo simulations to show that the ToE model is well suited for separating causal treatment effects from sorting effects.
The ToE method has also been shown to perform well relative to other non-experimental methods (Muller, van Der Klaauw, and Heyma Citation2020). Recent applications of the ToE model include Clausen et al. (Citation2009), Heinesen et al. (Citation2013); Kyyrä et al. (Citation2019). Particularly relevant to our context are Holm et al. (Citation2017), who used the ToE model to investigate the impact of labour market programmes for sick-listed workers in Denmark, and von Simson and Hardoy (Citation2020), who studied the impact of VRP among work impaired youth in Norway.
Our econometric approach is a multivariate mixed proportional hazard rate model. The model consists of two equations: (1) the selection equation, which models the transition rate from work impairment into one of three VRPs ‘p’, and (2) the outcome equation, which models the transition rate from work impairment to one of the two outcomes ‘o’.
We jointly estimate six monthly hazard rates: three programme categories (placement, training and follow-up measures) and two outcome states (ordinary employment and ordinary education). All transition rates depend on a set of observed individual-specific covariates x, the quarterly youth unemployment rate c, and the time already spent as work-impaired δ. All covariates are included as flexible as possible, preferably using dummy variables for each value. The effect of programme participation enters the outcome equation. The programme effect, ∆, is divided into two parts: one reflecting the possible transition to one of the outcomes while participating in a programme, and the other reflecting the transition to one of the outcomes after completing the programme. In the empirical specification, the in- and after-programme effects are allowed to vary with the mental health status of the individual.
A fundamental assumption in the ToE-framework is the no-anticipation assumption. This assumption states that knowledge about future treatment should not affect current outcomes. For instance, if individuals know for sure that they are going to participate in a VRP at an exact date in the future, they may choose to reduce/intensify their search for jobs while waiting for the programme to start. This may bias the estimated treatment effect if not controlled for. Noteworthy, the size of the bias will, however, depend on the time span between notification of programme participation and the actual start date; a longer time span allows for more time to act on this information. The supply of VRPs in Norway is constrained, and access to programmes is based on availability, often on short notice, with local variations. Notwithstanding, the no-anticipation does not rule out the possibility that individuals have knowledge of the determinants of programme assignment and act on this information.
Both equations include a set of time-invariant individual unobserved characteristics v. The unobserved characteristics influencing different transitions may be correlated, allowing the same unobserved characteristics to affect both the probability of participating in VRPs as well as the probability of obtaining job offers. If we ignore the correlations between the unobserved heterogeneities (e.g. between employment and programme participation), the estimated treatment effect will be biased. The unobserved characteristics enter the model as random effects and are thus assumed to be uncorrelated with the observed covariates. This is a rather strong assumption to meet. However, Lombardi et al. (Citation2021) show that the ToE-model is relatively robust to correlations between observed and unobserved covariates. Performing extensive Monte Carlo simulations based on actual data, they find that the degree of correlation between observed and unobserved factors does not significantly affect the estimated treatment effect, as long as the distribution of unobserved heterogeneity is flexibly specified, the sample size is large and there is some exogenous variation in the hazard rate. Our modelling approach accommodates all these points. As suggested by Gaure et al. (Citation2007) we let the unobserved heterogeneity follow a discrete distribution with an a priori unknown number of mass points. To avoid over- or under-correction for unobserved heterogeneity, we use the Akaike Information Criterion (AIC) to select the optimal number of mass points, as recommended by Lombardi et al. (Citation2021) We have full population data covering entire cohorts of youth. In addition, the inclusion of time-varying calendar variables induces exogeneity into the hazard rates, further relaxing the random effects assumption as well as the proportional hazards assumption (Brinch Citation2007; Lombardi, van den Berg, and Vikström Citation2021).
The timing-of-events results of Abbring and Van den Berg (Citation2003) ensure that the above-mentioned model is non-parametrically identified. A detailed description of the model and estimation procedure is given in Gaure et al. (Citation2007).
Data
Our sample consists of cohorts of youths aged 18–23 who registered at the local employment office as a work impaired job seeker in the period of 2010–2014. Our longitudinal data from several individual registers covers information on employment, unemployment, income, education, social welfare, demographics, etc., administered and merged by Statistics Norway. Our observation period ends in 2016 so that we have six years of observations for those who turned 18 in 2010 and two years for those who turned 23 in 2014. Importantly, a unique encrypted ID number makes it possible to link this data to the National Patient Register (NPR) to obtain mental health information on these youths from the ages of 15 to 17.Footnote3 The NPR is an administrative database of records reported by all government-owned hospitals and outpatient clinics and by all private health clinics that receive governmental reimbursement. The register contains individual information about all treatments received from the specialist healthcare service. The reporting of encrypted national ID numbers in the NPR began in 2008, allowing us to link it to other national registers.
Spells
We use single spell data, i.e. one spell one person. A spell of work impairment requires that two events occur: (1) that the person is registered as unemployed for at least one month and (2) that the person acquires the status of work impairment. Any two spells of reduced work capacity with durations of less than 60 days in between are merged and the state in between overseen. A spell ends when the youths leave work impairment for another labour market state. We only consider the first VRP in which the youths participate. Transitions to subsequent VRPs are censored. In addition, we censor spells that are still on-going at the end of the observation period. This makes it possible to include all young people who register as work impaired without having to make assumptions regarding spell length.
VRPs and outcome variables
VRPs are grouped into three categories: ‘Training’, ‘Placement’ and ‘Follow-up (FU)’. Training entails classroom courses, while Placement entails on-the-job training in the workplace. Follow-up is of short duration and provides guidance, support and assistance to facilitate the transition to the labour market. Remaining VRP categories are placed in a residual category and censored. We estimate transitions to two possible outcomes: employment and education. Other possible outcomes are put in a residual category and censored. If a person is registered in multiple states at the same time, the above order of priorities applies.
Mental disorders
MDs are primary explanatory variables in the outcome equations, in addition to VRPs. An individual is considered to have an MD if he or she is registered in NPR as having had one or more consultations with a specialist in mental health (psychiatry or psychology) when between the ages of 15–17. Noticeable, some MDs are not diagnosed and not all youth with MD reach out for help. Hence, we capture a lower bound of cases of MD. Depression, anxiety and insomnia are the major internalising disorders, while externalising problems are comprised mainly of ADHD and other conduct disorders. The remaining diagnoses are classified as ‘Other’, and mainly include unspecified MD and disorders related to mental retardation and developmental disorders.
Covariates
The regressions include several individual characteristics defined as non-parametrically as possible, measured at the start of the spell: age (a dummy for each age between 18–23), gender, immigrant status (native-born, born in a western country (Europe, North America, Australia), born in a non-western country (Asia, Africa, South America)), education level (completed upper secondary school), labour income and transfers (measured the calendar year before entering unemployment) and region of youth’s residence at 16 years old. Family background is captured by parents’ education level (compulsory, upper secondary, higher education, unknown), labour income and transfers (measured as an average of the period the youth was aged 7–17), and parent’s civil status when the youth was aged 16. We also include the national quarterly unemployment rate for youths between the ages of 15–24. Lastly, we control for cohort effects by including a dummy for the entry year of unemployment (2010–2014).
Results
Descriptives
shows the descriptive statistics for the youth in our sample. In all, 42% of the work-impaired unemployed youth in our sample were diagnosed with MD in adolescence and among those with MD, about one-third had internalising disorders and 20% had externalising disorders. The sample has an overweight of younger cohorts,Footnote4 half of the sample are girls, and the overwhelming majority are Norwegian born. Only 15% of the youths had completed upper secondary school.Footnote5 Note that even though youth are not officially being categorised as school dropouts until 5 years after starting upper secondary school, the youth in our sample are all registered at local employment office as work-impaired unemployed and hence have de facto dropped out/interrupted upper secondary education. Youth with a history of MD are slightly younger, more likely to be female, Norwegian born, to not have completed secondary school, and to have had lower labour income and higher transfers than those with no MD in adolescence. Differences between parents’ educations and labour incomes for those with and without earlier MD are negligible. However, youth with MD are more likely to have parents who have received public transfers and who have divorced.
Table 1. Descriptive statistics of youth with reduced work capacity aged 18–23, by mental health status (yes/no mental disorders (MD)).
There are surprisingly small differences in VRP participation by whether or not they had MD in adolescence. Some differences, however, are noticeable. Youth with MD are more likely to participate in Training if they have internalising problems, while those with externalising problems tend to participate in Placement. Young women tend to participate more in Training than in Placement, as do non-western born youth and those who have completed upper secondary education. As expected, youth with previous work experience and higher parental labour income and education are more likely to either, not participate in any VRPs, or to participate in Training.
shows durations and proportion of youths in VRPs as well as their transitions to the outcome states. Work impaired youth spend on average nearly two years either as openly unemployed or as a VRP participant, with a two-month difference depending on whether or not they had an earlier MD. However, there is great variation in this statistic, as shown by the large standard deviations. About 60% participated in a VRP irrespective of health conditions in adolescence, of which about half participated in measures providing work experience. While 14% of those with no MD got a job, only 9% of those with MD did. Moreover, many did not experience a transition at all during the period of observation, either because they remained in the initial state or because they started another VRP. These differences underscore the importance of modelling transitions to each VRP as well as the outcomes states separately, with controls for observable individual characteristics, previous mental health conditions, labour market histories and parental human capitals. Furthermore, the observed differences emphasise the need to control for potential selection on unobserved characteristics as well.
Table 2. VRP related descriptives by mental health status in adolescence for youth ages 18–23.
shows that many youths spend long periods being work impaired. There are substantial differences between non-participants and participants in VRPs. While the likelihood of remaining work impaired dropped steadily for non-VRP participants, for VRP participants, it starts dropping only after two years of duration. This likely reflects the known ‘lock-in’ effect of programme participation.
Figure 1. Survival curves by VRP status and mental health in adolescence for youths aged 18–23 years in the period 2010–2014.

shows transition rates into VRPs. Placement is used earlier in the spell of work impairment and stabilises to a lower level after 10 months. Transition rates to Training and FU measures are low and stable throughout the 5-year period of observation. Differences between those with and without previous MD are discernible.
Regression estimates
shows our main results. By exponentiating the estimated value and subtracting 1, we can interpret estimates as percentage changes in the likelihood of a transition occurring over a very short time interval (hazard rate), given that no such transition has yet occurred. For instance, the estimated effect of participating in placement with respect to a transition to work/education while on the programme of −0.929 can be interpreted as a percentage decrease in the hazard rate of 61% ((exp (−0.929) −1) * 100). Worth noting is that the size of the estimates and percentage change should be interpreted in relation to the initial transition probability. For instance, the probability of experiencing a transition to employment during the first month of work-impairment is 0.4. A 61% decrease in this probability due to participation in Placement involves a reduction in the transition probability of 0.24% points (0.61 × 0.4).
Table 3. Effect of VRPs during and after VRP on employment and education for youths aged 18–23.
Estimates indicate that while participating in a VRP (the first three rows of all three models), participants are likely to remain until its completion. These so-called ‘lock-in’ effects are very frequent and expected. After programme completion, there seems to be no impact on employment from any VRP category, irrespective of being diagnosed with a MD in adolescence or not.Footnote6 However, VRPs seem to have an impact on education prospects. Interestingly, participation on Placement has the opposite after-effect for youth with and without an earlier MD diagnosis. Thus, the comparison of after VRP effects by mental health conditions, with the Base model portrays the danger of disregarding mental health when studying the impact of labour market programmes targeted at youth with reduced work capacity.
As shown in , the 18- and 19-year-olds are overrepresented among the work-impaired with a previous MD. Their MD is recent and perhaps still ongoing and may even be the reason for their work-impairment. For the older youth, the selection process into work-impairment may be different.Footnote7 In addition, the efforts regarding the 18- and 19-year-olds may be more oriented towards upper secondary school completion, while for the older youths the main goal may be to enter the labour market or pursue further education. All this may influence the results.
In we leave out the youngest and estimate the model focusing only on the older youth aged 20–23. Let us look at youth with MD first. A comparison of suggests no major changes. The only noticeable change is the after-effect of Training on education, which becomes negative when the youngest are removed. This may signify that they gained the intended competence while on training or that the experience while on training has discouraged them from any further studies.
Table 4. Effect of VRPs during and after VRP on employment and education for youths aged 20–23.
Sensitivity analysis
Due to data availability, we observed the two oldest cohorts for a shorter period of time, a consequence of which is that the impact of VRPs for the younger cohort would drive the results. Because of this we ran separate regressions for the younger cohorts of youth aged 18–21.
shows the well-known lock-in effects while participating in VRPs. Several revealing results emerge when we limit the sample to the younger cohorts. For those with no previous MD, Placement seems to be counterproductive. Training, on the other hand, seems to encourage a transition to further education. Regarding youths with earlier diagnosed MDs, the picture remains stable when the older cohorts are removed.
Table 5. Effect of VRPs during and after VRP on employment and education for youths aged 18–21.
Lastly, following Lombardi et al. (Citation2021), we tested the sensitivity of the estimates to the choice of information criterion used to select the number of mass points in the distribution of unobserved heterogeneity. This study shows that selecting too few or too many mass points for the distribution of unobserved heterogeneity may seriously bias the treatment effects. They compare the performance of the maximum likelihood (ML) criterion to information criteria penalising parameter abundance, such as the AIC and the Bayesian Information Criterion (BIC). Lombardi et al. (Citation2021) conclude that all information criteria perform better than the ML criterion, but no single criterion performs better in all settings, and suggest reporting all as robust checks. They also point out that the risk of over-correcting by including mass points until the likelihood reaches its maximum is larger in smaller samples and suggest the use of AIC.
In , we show Max estimates corresponding to a specification within the maximum mass point (where the likelihood cannot be further improved), and the estimates while applying the AIC and BIC criteria. We reproduce estimates from in Column 2. Few estimated effects bear the test of the all the criteria; however, the earlier results seem quite stable.
Table 6. Impact of VRPs during and after VRP on employment and education performance of estimates with different information criteria for youths aged 18–23.
Summary and concluding remarks
There are rising numbers of youth suffering from anxiety, depression, violence and committing suicide, to name some disabling burdens. The coronavirus pandemic may accentuate these problems, as reflected in the media and clearly stated by the World Health Organization. MD in adolescence is a strong predictor of MDs later in life. Successful policies targeted at preventing, alleviating, and treating youth people with MDs are in high demand. Our analysis highlights the importance of including information on mental health when analysing the impact of VRP on future labour market outcomes. It supports existing evidence indicating that policies need to be targeted to be effective (Crépon and Van Den Berg Citation2016).
Our results reveal that knowledge of diagnosed MD in early adolescence is important to efficiently target VRP for work impaired youth. While lock-in effects and after-effects on employment remain stable, after-effects on education vary with earlier MD. Moreover, even within such as small age window as covered by our analyses (18–23 years of age) results vary with whether the youth are in school age or not. For the older age group, 20–23 years old, the only noticeable impact of VRP after programme completion is the effect of Training on education for youth with earlier MD, which becomes sizeably negative when the youngest are removed. This can be an indication that they gained the intended competence while on Training, thus reducing the need/interest in taking further education after programme completion. But it may also be the case that the VRP discouraged them from taking further education, an outcome which is not desirable since many of these youth have little formal qualifications.
The overwhelming majority of youth 21 years of age or younger registered as unemployed and participating in VRP have not finished secondary education. VRP have worked differently for age group 18–21. Placement stimulated those who had earlier struggled with ill-mental health, and who most likely have not completed secondary education, to go back to school. There is also some indication that counselling, characterised by being of short duration and individually oriented, worked as a ‘nudge by stimulating youth with previous MD who had an employment potential in the short run to succeed in getting a job. For youth with no diagnosed MD, Placement had the opposite effect, i.e. it reduced the likelihood of taking further education and of entering regular employment. In other words, placement seems counterproductive for the youngest cohorts with no MD. Training, on the other hand, stimulated youth with no previous MD to pursue further education. Positive transitions to education after VRP participation are in accordance with the labour market authorities aim to encourage youth to complete secondary school and qualify themselves. Noteworthy, since we investigate the impact on ordinary education and regular employment, our estimates provide a lower bound of social costs related to mental ill-health, disregarding dimensions such as mortality, suicide, well-being, etc.
A few caveats need mentioning. The data has the advantage that the measure of MD is objective and determined by a specialist, but it is unavoidably incomplete; not all youth with MD seek help. Further, there is variation between schools regarding the monitoring of students such that some schools are more likely to offer their pupils a consultation with a specialist than others. Lastly, our analyses focused on short-term effects. Longer time series would have allowed us to follow the youth over longer time intervals, which would have been an advantage since many observations had to be censored without making a transition.
Acknowledgments
We acknowledge financial support from the Norwegian Research Council under Grant nr. 247996; and Grant nr. 280307. We thank the participants in seminars at our respective institutes. Also, the comments we received on this paper from the editor and anonymous referees are highly appreciated.
Disclosure statement
No potential conflict of interest was reported by the author(s).
Data availability statement
Data in Norway is subject to outmost restrictions, particularly health data. It is only available for research purposes under a declaration of confidentiality.
Additional information
Funding
Notes on contributors
Ines Hardoy
Inés Hardoy is a Research Professor at the Institute of Social Research in Oslo, Norway. She holds a PhD in Economics from the University of Oslo. Her research mainly focuses on the labour market adaptation of vulnerable groups such as young people, immigrants, women and people with reduced work capacity with particular emphasis on the impact labour market policies, welfare policies and family policies have on this process. Her expertise is in empirical research, using register data together with state-of-the-art econometric techniques. She has sat in several expert commissions nationally and at the EU level and has published extensively in labour and policy journals.
Kristine von Simson
Kristine von Simson holds a PhD in Economics (University of Oslo) and works as a research analyst at the Norwegian Labour and Welfare Administration. Her research interests relate to empirical labour market economics, particularly issues concerning the transition from school to work for young people, marginalisation of vulnerable groups and the importance of formal and informal skills for labour market attachment. She has solid expertise in econometric techniques for identifying causal effects using register data.
Notes
2. Noticeable, the most popular VRP for young adults aged 25–30 is ordinary education, which the youth in our sample are not entitled to it.
3. We have a 6-years observation window during which no changes in data registration are recorded Moreover, MD is defined using previous registration of contact with a specialist during a 4 years window (in ages 15–17). Given the time span, changes in recording practices are unlikely and smothered by the fact that we use moving average when recording MD.
4. This is due to data accessibility.
5. The share of youth having completed upper secondary school increases with age, from 3% of 18-year-olds to 25% of 20–23-year-olds.
6. A comparison of the effect estimates from a model with no unobserved heterogeneity reveals positive selection into the programmes. Complete estimation results are available upon request.
7. Recall that we only have information on mental health from age 15 to 18, which means that we have no register of recent MD among the older cohorts is not captured in our analyses.
References
- Abbring, J. H., and G. J. Van den Berg. 2003. “The Nonparametric Identification of Treatment Effects in Duration Models.” Econometrica 71 (5): 1491–1517. https://doi.org/10.1111/1468-0262.00456.
- Beck, A., A. L. Crain, L. I. Solberg, J. Unützer, R. E. Glasgow, M. V. Maciosek, and R. Whitebird. 2011. “Severity of Depression and Magnitude of Productivity Loss.” The Annals of Family Medicine 9 (4): 305–311. https://doi.org/10.1370/afm.1260.
- Bewley, H., R. Dorsett, and S. Salis. 2009. The Impact of Pathways to Work on Work, Earnings and Self-Reported Health in the April 2006 Expansion Areas, 68. Vol. 601. DWP research report no. Norwich: Department for Work and Pensions. ISBN 9781847126399.
- Bor, W., A. J. Dean, J. Najman, and R. Hayatbakhsh. 2014. “Are Child and Adolescent Mental Health Problems Increasing in the 21st Century? A Systematic Review.” Australian & New Zealand Journal of Psychiatry 48 (7): 606–616. https://doi.org/10.1177/0004867414533834.
- Brinch, C. N. 2007. “Nonparametric Identification of the Mixed Hazards Model with Time-Varying Covariates.” Econometric Theory 23 (2): 349–354. https://doi.org/10.1017/S0266466607070144.
- Browning, M., and E. Heinesen. 2012. “Effect of Job Loss Due to Plant Closure on Mortality and Hospitalization.” Journal of Health Economics 31 (4): 599–616. https://doi.org/10.1016/j.jhealeco.2012.03.001.
- Bubonya, M., D. A. Cobb-Clark, and M. Wooden. 2017. “Mental Health and Productivity at Work: Does What You Do Matter?” Labour Economics 46:150–165. https://doi.org/10.1016/j.labeco.2017.05.001.
- Burns, T., J. Catty, T. Becker, R. E. Drake, A. Fioritti, M. Knapp, C. Lauber, W. Rössler, T. Tomov, and J. Van Busschbach. 2007. “The Effectiveness of Supported Employment for People with Severe Mental Illness: A Randomised Controlled Trial.” The Lancet 370 (9593): 1146–1152. https://doi.org/10.1016/S0140-6736(07)61516-5.
- Case, A., and A. Deaton. 2020. Deaths of Despair and the Future of Capitalism. Princeton: Princeton University Press. https://doi.org/10.2307/j.ctvpr7rb2.
- Clausen, J., E. Heinesen, H. Hummelgaard, L. Husted, and M. Rosholm. 2009. “The Effect of Integration Policies on the Time Until Regular Employment of Newly Arrived Immigrants: Evidence from Denmark.” Labour Economics 16 (4): 409–417. https://doi.org/10.1016/j.labeco.2008.12.006.
- Crépon, B., and G. J. Van Den Berg. 2016. “Active Labor Market Policies.” Annual Review of Economics 8 (1): 521–546. https://doi.org/10.1146/annurev-economics-080614-115738.
- Dean, D., J. V. Pepper, R. Schmidt, and S. Stern. 2017. “The Effects of Vocational Rehabilitation Services for People with Mental Illness.” Journal of Human Resources 52 (3): 826–858. https://doi.org/10.3368/jhr.52.3.0114-6111R1.
- de Graaf, R., M. Ten Have, C. van Gool, and S. van Dorsselaer. 2012. “Prevalence of Mental Disorders and Trends from 1996 to 2009. Results from the Netherlands Mental Health Survey and Incidence Study-2.” Social Psychiatry and Psychiatric Epidemiology 47 (2): 203–213. https://doi.org/10.1007/s00127-010-0334-8.
- Drake, R. E., J. S. Skinner, G. R. Bond, and H. H. Goldman. 2009. “Social Security and Mental Illness: Reducing Disability with Supported Employment.” Health Affairs 28 (3): 761–770. https://doi.org/10.1377/hlthaff.28.3.761.
- Frölich, M., A. Heshmati, and M. Lechner. 2004. “A Microeconometric Evaluation of Rehabilitation of Long‐Term Sickness in Sweden.” Journal of Applied Econometrics 19 (3): 375–396. https://doi.org/10.1002/jae.757.
- Gaure, S., K. Røed, and T. Zhang. 2007. “Time and Causality: A Monte Carlo Assessment of the Timing-Of-Events Approach.” Journal of Econometrics 141 (2): 1159–1195. https://doi.org/10.1016/j.jeconom.2007.01.015.
- Graversen, B. K., and P. Jensen. 2010. “A Reappraisal of the Virtues of Private Sector Employment Programmes.” Scandinavian Journal of Economics 112 (3): 546–569. https://doi.org/10.1111/j.1467-9442.2010.01611.x.
- Guo, G., and K. M. Harris. 2000. “The Mechanisms Mediating the Effects of Poverty on Children’s Intellectual Development.” Demography 37 (4): 431–447. https://doi.org/10.1353/dem.2000.0005.
- Hale, D. R., L. Bevilacqua, and R. M. Viner. 2015. “Adolescent Health and Adult Education and Employment: A Systematic Review.” Pediatrics 136 (1): 128–140. https://doi.org/10.1542/peds.2014-2105.
- Heinesen, E., L. Husted, and M. Rosholm. 2013. “The Effects of Active Labour Market Policies for Immigrants Receiving Social Assistance in Denmark.” IZA Journal of Migration 2 (1): 1–22. https://doi.org/10.1186/2193-9039-2-15.
- Høgelund, J., and A. Holm. 2006. “Case Management Interviews and the Return to Work of Disabled Employees.” Journal of Health Economics 25 (3): 500–519. https://doi.org/10.1016/j.jhealeco.2005.07.007.
- Høgelund, J., A. Holm, and J. McIntosh. 2010. “Does Graded Return-To-Work Improve Sick-Listed workers’ Chance of Returning to Regular Working Hours?” Journal of Health Economics 29 (1): 158–169. https://doi.org/10.1016/j.jhealeco.2009.11.009.
- Holm, A., J. Høgelund, M. Gørtz, K. S. Rasmussen, and H. S. B. Houlberg. 2017. “Employment Effects of Active Labor Market Programs for Sick-Listed Workers.” Journal of Health Economics 52:33–44. https://doi.org/10.1016/j.jhealeco.2017.01.006.
- Kyyrä, T., J. M. Arranz, and C. García-Serrano. 2019. “Does Subsidized Part-Time Employment Help Unemployed Workers to Find Full-Time Employment?” Labour Economics 56:68–83. https://doi.org/10.1016/j.labeco.2018.12.001.
- Lombardi, S., G. J. van den Berg, and J. Vikström. 2021. “Empirical Monte Carlo Evidence on Estimation of Timing-Of-Events Models.” SSRN Electronic Journal. https://doi.org/10.2139/ssrn.3762885.
- Maibom, J., M. Rosholm, and M. Svarer. 2017. “Experimental Evidence on the Effects of Early Meetings and Activation.” The Scandinavian Journal of Economics 119 (3): 541–570. https://doi.org/10.1111/sjoe.12180.
- Markussen, S., and K. Røed. 2014. “The Impacts of Vocational Rehabilitation.” Labour Economics 31:1–13. https://doi.org/10.1016/j.labeco.2014.08.001.
- Mendelson, T., and W. W. Eaton. 2018. “Recent Advances in the Prevention of Mental Disorders.” Social Psychiatry and Psychiatric Epidemiology 53 (4): 325–339. https://doi.org/10.1007/s00127-018-1501-6.
- Muller, P., B. van Der Klaauw, and A. Heyma. 2020. “Comparing Econometric Methods to Empirically Evaluate Activation Programs for Job Seekers.” Journal of Applied Econometrics 35 (5): 526–547. https://doi.org/10.1002/jae.2763.
- OECD. 2012. Sick on the Job?: Myths and Realities About Mental Health and Work. Paris, France: OECD Publishing. https://doi.org/10.1787/9789264124523-en.
- OECD. (2013). Mental Health and Work: Norway. https://doi.org/10.1787/9789264178984-en
- OECD. 2018. Integrated Mental Health, Skills and Work Policy [OECD Recommendation of the Council]. OECD. https://legalinstruments.oecd.org/public/doc/334/334.en.pdf.
- Paul, K. I., and K. Moser. 2009. “Unemployment Impairs Mental Health: Meta-Analyses.” Journal of Vocational Behavior 74 (3): 264–282. https://doi.org/10.1016/j.jvb.2009.01.001.
- Sobocki, P., B. Jönsson, J. Angst, and C. Rehnberg. 2006. “Cost of Depression in Europe.” The Journal of Mental Health Policy and Economics 9 (2): 87–98.
- Taylor, R. D., E. Oberle, J. A. Durlak, and R. P. Weissberg. 2017. “Promoting Positive Youth Development Through School‐Based Social and Emotional Learning Interventions: A Meta‐Analysis of Follow‐Up Effects.” Child Development 88 (4): 1156–1171. https://doi.org/10.1111/cdev.12864.
- Thygesen, L. C., and A. K. Ersbøll. 2014. “When the Entire Population is the Sample: Strengths and Limitations in Register-Based Epidemiology.” European Journal of Epidemiology 29 (8): 551–558. https://doi.org/10.1007/s10654-013-9873-0.
- Twenge, J. M., A. B. Cooper, T. E. Joiner, M. E. Duffy, and S. G. Binau. 2019. “Age, Period, and Cohort Trends in Mood Disorder Indicators and Suicide-Related Outcomes in a Nationally Representative Dataset, 2005–2017.” Journal of Abnormal Psychology 128 (3): 185. https://doi.org/10.1037/abn0000410.
- Veldman, K., S. Reijneveld, J. Almansa Ortiz, and U. Bultmann. 2018. “Mental Health Trajectories from Childhood to Young Adulthood Affect the Transition into the Labour Market.” European Journal of Public Health 28 (suppl_4): cky213. 891. https://doi.org/10.1093/eurpub/cky212.891.
- von Simson, K., I. Brekke, and I. Hardoy. 2022. “The Impact of Mental Health Problems in Adolescence on Educational Attainment.” Scandinavian Journal of Educational Research 66 (2): 306–320. https://doi.org/10.1080/00313831.2020.1869077.
- von Simson, K., and K. Hardoy. 2020. “Tackling Disabilities in Young Age—Policies That Work.” IZA Journal of Labor Policy 10 (1). https://doi.org/10.2478/izajolp-2020-0013.
- Vuori, J., and A. D. Vinokur. 2005. “Job-Search Preparedness as a Mediator of the Effects of the Työhön Job Search Intervention on Re-Employment and Mental Health.” Journal of Organizational Behavior 26 (3): 275–291. https://doi.org/10.1002/job.308.
- Wealth Health Organization. 2004. Investing in Mental Health. Geneva: World Health Organization.
- Wittchen, H. U., F. Jacobi, J. Rehm, A. Gustavsson, M. Svensson, B. Jönsson, J. Olesen, et al. 2011. “The Size and Burden of Mental Disorders and Other Disorders of the Brain in Europe 2010.” European Neuropsychopharmacology 21 (9): 655–679. https://doi.org/10.1016/j.euroneuro.2011.07.018.