ABSTRACT
Objective
This study compared and explored the neurocognitive profiles of siblings of persons with and without neurodevelopmental conditions (NDCs) and associations between objective test performance and self-reported psychosocial functioning.
Methods
Siblings of persons with and without NDCs (64 NDC and 64 control siblings; mean age 19.88 years, range 11–27 years, 73.44% female, 75.78% White Caucasian) completed self-report questionnaires and self-administered computerized neurocognitive tests of executive functioning (EF). Using Bayesian analyses, we examined cross-sectional associations between self-reported psychosocial functioning and cognitive test performance, and predictors of EF over 15 months.
Results
NDC siblings had poorer working memory, inhibition, attention, and shifting compared to controls, as measured by experimental paradigms on the backward Corsi span, N-Back 2-back task, Stop Signal Task, Sustained Attention to Response Task, and the Wisconsin Card Sorting Test (effect size δ ranging 0.49 to 0.64). Bayesian cross-sectional networks revealed negative emotion reactivity and working memory difficulties were central to the NDC sibling network. Over 15 months, poorer EF (k low test scores) was predicted by negative emotion reactivity, sleep problems, and anxiety, over and above effects of age and subclinical autistic and ADHD traits. Siblings of autistic individuals and persons with fetal alcohol spectrum disorder had higher rates of neurocognitive and psychiatric difficulties than other NDCs and controls (Bayes factors >20).
Conclusions
Neurocognitive difficulties were associated with transdiagnostic vulnerability to poorer wellbeing in NDC siblings. These findings demonstrate the feasibility of remote online cognitive testing and highlight the importance of individualized prevention and intervention for NDC siblings.
HIGHLIGHTS
NDC siblings had poorer attention and executive functioning across five experimental paradigms compared to controls.
NDC siblings had poorer self-reported psychological, emotional and behavioral functioning compared to controls.
Siblings of autistic persons and FASD had diffuse cognitive and self-reported functioning difficulties compared to other NDCs and controls.
Baseline self-reported negative emotion reactivity and sleep problems predicted objective EF difficulties in NDC siblings after 15 months.
Siblings of persons with neurodevelopmental conditions (NDCs) experience increased genetic and environmental vulnerabilities to poorer psychosocial functioning and neurocognitive development compared to controls (Jokiranta-Olkoniemi et al., Citation2016, Citation2019; Shivers et al., Citation2019; Wolff et al., Citation2022).Footnote1 Although a minority of studies report positive outcomes and resiliency (Kovshoff et al., Citation2017), there is robust evidence that subclinical features of NDCs and associated neurocognitive difficulties co-aggregate within NDC families (Rommelse et al., Citation2008) and are associated with poorer mental health and functional outcomes in siblings (Seng et al., Citation2020; Wade et al., Citation2015).
Executive functioning (EF) is a heterogeneous set of neurocognitive skills, with “cold” EFs (cognitive control processes mediated by the dorsolateral prefrontal cortex; Zelazo, Citation2020) encompassing cognitive flexibility, inhibitory control, working memory, and attentional control (Bailey & Jones, Citation2019; Friedman & Miyake, Citation2017). EF difficulties are associated with psychiatric conditions (Abramovitch et al., Citation2021; Yang et al., Citation2022), the ADHD phenotype (Kofler et al., Citation2019), and autistic traits (Demetriou et al., Citation2019).Footnote2 Siblings of persons with other NDCs, including intellectual disability (ID) and fetal alcohol spectrum disorder (FASD), are also susceptible to difficulties with EF, self-regulation, and psychological functioning (Kvigne et al., Citation2009; Marquis et al., Citation2020; Wolff et al., Citation2023), which, in some cases, may reflect missed NDC diagnoses (Fusar-Poli et al., Citation2022).
Executive functioning test performance in NDC siblings
NDC siblings have demonstrated vulnerabilities across the core EFs, including shifting, attention, inhibition, and working memory, although the findings are heterogeneous. Siblings of autistic persons often display minimal difficulties on the Wisconsin Card Sorting Test (Happé et al., Citation2001; Hughes et al., Citation1999; Oerlemans et al., Citation2013; Sumiyoshi et al., Citation2011; Szatmari et al., Citation1993), or other set shifting tasks (e.g., Intra-Extradimensional Task, Trail Making Test B; Brunsdon et al., Citation2015; Delorme et al., Citation2007; Wong et al., Citation2006). Conversely, other studies report significantly more perseverative errors on the WCST when comparing siblings of autistic children (d = 0.57; Hughes et al., Citation1999; Van Eylen et al., Citation2017) or persons with ADHD (d = 0.30; Bidwell et al., Citation2007) to controls.
Sustained attention is often within typical ranges in siblings of autistic children (Rosa et al., Citation2017), although others observe poorer performance on the continuous performance tasks (Chien et al., Citation2017) and other attention tasks (i.e., the NEPSY; Warren et al., Citation2012). In siblings of children with ADHD, deficits are noted on the CCPT, after covarying for ADHD symptoms (Bidwell et al., Citation2007).
Regarding inhibitory control, siblings of children with ADHD display longer and more variable stop signal reaction times, as measured by the Stop Signal Task (SST; Verbruggen et al., Citation2013) than controls, adjusting for ADHD symptoms (Bidwell et al., Citation2007; Rommelse et al., Citation2008; Schachar et al., Citation2005; Slaats-Willemse et al., Citation2003). Siblings of autistic children have response inhibition difficulties on Go/No-Go tasks (Van Eylen et al., Citation2017), while others find minimal group differences on the same (Oerlemans et al., Citation2016) or similar task paradigms (Response Inhibition and Load Task; Luria Hand Game; Brunsdon et al., Citation2015; Wong et al., Citation2006).
Regarding working memory, siblings of autistic children perform worse than controls on forward spatial span tasks in some studies (Mosconi et al., Citation2010; Seng et al., Citation2020). Others report no differences in visual (Wong et al., Citation2006) or verbal working memory (i.e., digit span; Oerlemans et al., Citation2013; Rosa et al., Citation2017), and even superior visuospatial working memory compared to controls (Hughes et al., Citation1999). Siblings of children with ADHD similarly may have poorer spatial span, adjusting for subclinical ADHD traits (Bidwell et al., Citation2007), and verbal working memory than controls (Oerlemans et al., Citation2016; Rommelse et al., Citation2008; Van Lieshout et al., Citation2017), while do not find significant group differences (van Ewijk et al., Citation2014).
Several limitations are evident from the above review. Studies are characterized by inconsistent operationalization of neurocognitive constructs and measures used, and limited exploration of cognitive functioning beyond the infant sibling years (Bidwell et al., Citation2007). To date, there has been little emphasis on self-reported psychological correlates of objective cognitive functioning among siblings. Most sibling studies only report on single EF tasks or domains. Additionally, experimental paradigms in a laboratory setting lack ecological validity (Schmitter-Edgecombe et al., Citation2020), hence, findings may not reflect everyday functional difficulties if examined in isolation without self-reported psychosocial functioning. Finally, few studies include siblings of persons with NDCs other than ADHD and autism, despite known genetic and environmental risks among other NDCs (although see Fombonne et al., Citation1997; Szatmari et al., Citation1993).
Associations between EF and self-reported psychosocial functioning in NDC siblings
Interactions between factors predisposing NDC siblings to suboptimal social, emotional, cognitive, and neuropsychological outcomes are critical for clinical neuropsychologists to consider. Self-regulation, the ability to control behavioral and emotional responses to achieve goals or adjust to social expectations (Duckworth & Kern, Citation2011), overlaps with, and is related to, EF abilities (Diamond, Citation2013). Emotion regulation and reactivity (affective EF skills; Zelazo, Citation2020) are components of self-regulation which, if poorly regulated, are transdiagnostic risk factors for psychopathology and cognitive dysfunction (Gross & Jazaieri, Citation2014; Preece et al., Citation2019), including vulnerable attentional processes, cognitive inflexibility, and poorer affective working memory (Mikels & Reuter-Lorenz, Citation2019; Pe et al., Citation2015). NDC siblings experience greater negative emotion reactivity than controls (Lobato & Kao, Citation2002), which is associated with anxiety, depression, and NDC traits (Wolff et al., Citation2022, Citation2023). Siblings also report elevated sleep difficulties compared to controls (Wolff et al., Citation2022), and these difficulties are associated with poorer self-regulation, and poorer cognitive, psychosocial, and behavioral functioning in adolescence (McArthur et al., Citation2022; Sunderland et al., Citation2021).
Directionality and psychosocial predictors of EF in NDC siblings
NDC sibling atypicalities may, in part, be due to early life circumstances unique to NDC siblings, such as parental stress, reduced parental attention, and modeling of executive functions such as problem-solving behaviors, and limited access to services (Kovshoff et al., Citation2017; Marquis et al., Citation2019) impacting maturation of EF and emotion regulation skills (Snyder et al., Citation2015; Zelazo, Citation2020). Further, psychological difficulties may transactionally impair siblings’ capacity to develop and practice EF skills (e.g., less social engagement, more school absenteeism; Kievit, Citation2020), while maladaptive cognitive and regulatory processes may reduce EF efficiency due to increased load on limited cognitive resources (Levens et al., Citation2009). Further, early life adversity impacts the development of EF and emotion regulation skills (Zelazo, Citation2020), and chronic stress over time experienced by siblings may contribute to vulnerability to psychopathology during important developmental periods (Snyder et al., Citation2015). EF is a transdiagnostic process associated with NDC traits and neuropsychiatric symptoms cross-sectionally (Abramovitch et al., Citation2021; Snyder et al., Citation2015) and longitudinally (Yang et al., Citation2022), considered a cause and consequence of psychopathology (Romer & Pizzagalli, Citation2021). Despite empirical evidence that impairment in multiple psychosocial domains early in childhood may have a developmental cascade effect on cognitive and mental health outcomes (Flouri et al., Citation2019), existing literature is equivocal regarding the neuropsychological characteristics of siblings of persons with NDCs and the nature of their EF difficulties (Bidwell et al., Citation2007; Wong et al., Citation2006). There is also preliminary evidence of directionality, where early emotional and behavioral regulation in childhood predicts EF (working memory and inhibition) development prospectively, while early EF measures often do not predict later psychopathology (Donati et al., Citation2021). Associations between objective EF and self-rated psychosocial functioning, and importantly, earlier predictors of specific EF difficulties, have not been explored in NDC siblings.
Importance to clinical neuropsychology
NDC siblings remain undetected in mental health services throughout childhood, during which interventions may have the greatest impact (Wade et al., Citation2015). A better understanding of NDC siblings’ EF profiles, mental health, and unique psychosocial stressors is required in clinical neuropsychology to improve assessment and timeline allocation of resources. However, this endeavor is limited by sibling studies of small samples and lack of resources for testing (Shivers et al., Citation2019). Self-administered remote assessment (i.e., administration of structured, unsupervised web-based cognitive testing using digital tools; Van Patten, Citation2021) is of high importance to clinical neuropsychologists as it improves access to assessment services and clinical research (i.e., time and financial constraints; Kessels, Citation2019; Marquis et al., Citation2019), provides assessment options for individuals with symptomatic constraints (e.g., social avoidance; Lavigne et al., Citation2022), reduces administration error (Kim et al., Citation2019), allows recruitment of larger and more diverse samples (e.g., rural and remote areas, and has greater ecological validity and generalizability; Bilder, Citation2011). The present study will provide insight into the clinical value, efficiency, and precision of remote assessment, and thus improve the validity of existing NDC sibling literature.
To advance the field, clinical neuropsychologists have a need to tailor methodologies to individual patients and situations. To date, contextual factors are neglected in NDC sibling research, yet consideration of these unique variables is critical for personalized assessment, interpretation of test data (Suchy, Citation2020), and effectively communicating neuropsychological feedback (Gruters et al., Citation2022). Consequently, this study has implications for our understanding of neuropsychological, behavioral, and psychiatric difficulties of NDC siblings which may impact their performance on cognitive measures and indirect testing of subjective wellbeing, hence contributing to a more complete clinical formulation of NDC siblings’ broad functional outcomes.
Study rationale
This study explored the neuropsychological and subjectively reported functioning of NDC siblings within an adapted dynamic bioecological systems framework, emphasizing that neurocognitive and psychological development are shaped by complex interrelations of proximal and distal risk and protective factors (Bronfenbrenner, Citation1995; McElroy et al., Citation2018). We previously found NDC siblings experienced more self-reported everyday EF difficulties (cognitive inflexibility, attention problems, hyperactivity/impulsivity) than controls, which were associated with poorer mental health (Wolff et al., Citation2023); however, there is debate regarding the convergent validity of self-report and objective EF (Howlett et al., Citation2022), and we aim to validate our findings with direct testing.
Further, given the heterogeneity of findings in the existing sibling literature (Shivers et al., Citation2019), and the inability of classical inference to quantify this uncertainty, Bayesian statistics represent a step forward for deriving meaningful predictions regarding the probability of an effect (Wagenmakers et al., Citation2016), and informed variable selection (Heck et al., Citation2022). Bayesian methods thus allow integration of clinical expertise with research findings (Kruschke, Citation2021), hence being of direct relevance to clinical neuropsychologists, and well suited to testing new theories in this under-researched population. To this end, we used Bayesian analyses to compare EF profiles of siblings with and without NDCs, characterize interrelations between EF and self-reported functioning, and identify psychosocial predictors of EF 15 months later. Our aims were to first compare EF test performance and self-reported psychosocial and behavioral functioning between (i.e., NDC to control) and within (i.e., different NDCs) sibling groups. Second, we characterize cross-sectional associations between individual EF domains and between EF and self-reported functioning among NDC siblings. Third, we explore the extent to which baseline (T1) self-reported functioning predicted general and specific EF performance at follow-up (T2) in the NDC group.
We hypothesized first (H1) that NDC siblings would demonstrate relative weaknesses across all EF tasks and self-reported functioning compared to controls, with the largest effect sizes for shifting and working memory, and FASD siblings demonstrating the poorest outcomes. Second (H2), within the NDC group, associations would be observed between poorer self-reported emotion reactivity, sleep problems, greater NDC traits, and poorer EF performance, with attention and working memory exhibiting the strongest conditional associations. Third (H3), T1 self-reported cognitive inflexibility and greater autistic traits would predict more WCST errors, while greater attention difficulties would predict poorer SART, SST, N-Back, and Corsi performance. Sleep problems, anxiety, and negative emotion reactivity would predict poorer general EF across tasks.
Methods
Pre-Registration
This study was pre-registered on OSF registries: https://osf.io/p87k2. In accordance with open science and transparency guidelines (Grant et al., Citation2022), all planned and exploratory analyses are reported herein or available at: https://osf.io/6w7ar/.
Research support and ethics
This research was funded by an Australian National Health and Medical Research Council (NHMRC) grant (1184770) and ethical approval was granted by the Human Ethics Office at The University of Western Australia (2020/ET000049).
Participants and procedure
The objective of the broader Sibling Project was to collect self-report mental health and cognitive test data from siblings using online surveys: a baseline self-report survey open from June to August 2021 (time 1; T1) and a follow-up self-report survey with embedded cognitive tests 15 months later, in October 2022 (time 2; T2; see ). Our Sibling Advisory Group (n = 7, ages 10–24 years) were actively involved in the design and piloting of the baseline (T1) and follow-up (T2) surveys. Participants were recruited through the authors’ Institute and University e-mail, social media, and recruitment pools, and through Qualtrics Panels. Participants were required to be at least 8 years of age, have at least one sibling, and not have a diagnosed NDC. Of the 715 participants from T1 (235 NDC, 480 control, mean age 22.40 years, range 8–65, 76% female, 74% White Caucasian), 351 participants consented to be contacted at T2. They were emailed a Qualtrics survey link 15 months post-T1 and had the option to complete five computerized tests following the T2 survey or finish with the self-report questionnaire only. Cognitive testing mean completion time was 26.2 min (standard deviation; SD; 2.28), and self-report measures 18.6 min (SD 4.07). Participants were offered a $20 online gift card.
Of the 277 T2 participants, 128 completed the cognitive tests (64 NDC, 64 control; 17.9% of T1 respondents). Bayesian independent samples t-tests compared self-reported mental health diagnoses and demographics of participants in the T1 sample to those who completed cognitive testing at T2, indicating absence of effect of group (BFs10 <1, δ 0.02 to 0.11) other than the present sample being younger (BF10 2.98, δ 0.39) and less geographically diverse than the T1 sample.
Test and variable selection
Our self-report variables were selected from empirically and theoretically derived risk and resilience factors (Wolff et al., Citation2022). Cognitive tests were selected based on ambiguity in the sibling literature, as described in the Introduction above (e.g., WCST, SST), and seeking to extend our understanding to task paradigms not yet examined in NDC siblings (e.g., Corsi, SART, N-Back). Tests were obtained from the website PsyToolkit, a free platform which enables embedding of self-directed neuropsychological assessments into online surveys (Stoet, Citation2017). PsyToolkit has been found to have minimal error noise and high-level replicability of results compared to other computerized platforms (Kim et al., Citation2019).
Measures
Cognitive test variables (administered at T2 only)
Backward Corsi block tapping task (Corsi; Kessels et al., Citation2008)
The Corsi backward block tapping task is a spatial working memory task (Corsi, Citation1972; Kessels et al., Citation2008). The Corsi task has been implemented digitally and produces comparable results to the traditional version across a range of ages (Arce & McMullen, Citation2021; Claessen et al., Citation2015). Instructions were presented on the screen, stating: “You will see 9 blocks; some will ‘light’ up (yellow) in a sequence. Once you hear ‘go,’ you need to click the same blocks in the reverse sequence. Each trial, the sequence will increasingly get longer.” There was no practice trial. The participant was required to remember the order in which purple rectangles were highlighted in yellow on the screen (one per second), then click/touch the rectangles in reverse order, from two blocks to a maximum of nine blocks, with two trials per block length, and click “done” when finished. The task discontinued after two consecutive incorrect responses at one length or after 10 s with no response on both trials. Each correct trial was scored one point with a maximum of 18. The mean completion time was 2:30 min. The variable of interest was the longest backward sequence accurately recalled (Corsi).
Wisconsin card sorting task (WCST; Berg, Citation1948)
The WCST is purported to measure cognitive flexibility (Berg, Citation1948) and has been validated as a computerized task (Arán Filippetti et al., Citation2020; Steinke et al., Citation2021). In this adaptation, instructions were displayed on the screen, stating: “In this task, you need to match a card to one of four cards presented at the top of the screen. Following your selection, you will get feedback. If your match was not correct, you need to try a different rule. You will need to find out whether to match according to color, number, or shape. The matching rule changes now and then. You need to carefully monitor the feedback. There are 60 trials.” Four cards were presented on the screen, and the participant identified the classification rule by trial and error to sort the trial card at the bottom of the screen by color, number, or shape. The sorting rule changed after nine cards. After clicking the card, auditory and visual feedback (“good!” or “wrong!”) about the choice was provided. If the participant had not responded within 10 s, the trial was recorded as incorrect. No participants failed to complete the first category. The mean completion time was 4:20 min. We measured perseverative (WCST PE %) and non-perseverative error (WCST NPE %) percentages. We used the Channon (Citation1996) scoring method, defining perseverative errors as perseverative responses that were incorrect.
Stop Signal Task (SST; Verbruggen & Logan, Citation2008)
The Stop Signal Task (SST) was used to measure behavioral (i.e., controlled) inhibition. The SST is a choice reaction time (RT) task, in which participants respond to a set of stimuli and, on a minority of trials, must inhibit a prepared response when a stop-signal is displayed (Verbruggen & Logan, Citation2008). Good task performance and adherence to the horse race model assumption of the SST have been reported in web-based self-administered versions of the SST (Poulton et al., Citation2022). The Stop Signal Task (SST) parameters were guided by Verbruggen et al. (Citation2019). Participants responded to a set of stimuli across 200 trials and, on 50 trials, must inhibit a response when a stop-signal is displayed (Verbruggen & Logan, Citation2008). Instructions were presented on the screen, stating: “In this task, you need to respond as fast as you can to a left or right pointing arrow with the keys B or N; you have only up to half a second, so you must be ready with your full attention! The training block will not end until you can do at least 20 trials without many any mistakes.” Participants must respond within 500 ms, or the words “you should have pressed” were displayed on the screen. The task consisted of “go” and “no go” trials. In go trials, the participant must press the correct key; the green arrow appeared and there was no stop signal. The green arrow was presented for up to 500 ms. In no-go trials, the white circle became red after a short delay (the stop signal delay; SSD), between 100 ms and 450 ms following stimulus onset, in which case the participant should not press a key. SSDs were determined using a staircasing algorithm. The words “wrong key” were presented for an incorrect response. The mean completion time was 11:10 min. We examined the stop signal reaction time (SSRT; calculated using the integration method; Verbruggen et al., Citation2013).
Sustained attention to response task (SART; Robertson et al., Citation1997)
The SART is a measure of sustained attention considered more effective than the classic vigilance continuous performance task in preventing responses becoming automatic rather than controlled (Robertson et al., Citation1997). Instructions were presented on the screen, stating: “In this experiment, you will be presented with the digits 1 to 9 in the center of the screen. Your task is to press the spacebar in response to each digit, except for when the digit is a ‘3.’ Each digit is followed by a circle with a cross, which you can ignore. For example, if you see the digit ‘1,’ press the space bar. If you see a ‘3,’ do not press the space bar. Please give equal importance to both the speed and accuracy of your responses.” The digit was presented for 250 ms followed by the mask (circle surrounding a cross) for 700 ms. Stimuli were presented in white on a black background, with five different font sizes (48, 72, 94, 100, and 120 points). The participant received feedback regarding mistakes only (e.g., if the spacebar was pressed on a “3,” the word “mistake!” appeared), while feedback was not provided for correct responses. There were 18 training trials and 225 test trials (including 25 no go trials). In the training block, each digit ranging from one to nine was used twice, and in the experimental block, each digit was used 25 times. The mean completion time was 5:55 min. Variables of interest were the omission (SARTOm%) and commission (automatic inhibitory control; SARTCom%) error percentages.
N-Back, 2-back task (Kirchner, Citation1958)
The N-Back task is purported to measure working memory, recognition, and updating (Jaeggi et al., Citation2010; Kirchner, Citation1958). It assesses how often an individual can identify whether the current stimulus matches the one two trials back using continuous maintaining and updating of the previous two items viewed (Jaeggi et al., Citation2010). The 2-back condition has previously reliably been associated with working memory across computerized variations (Pelegrina et al., Citation2015). In this iteration, instructions appeared on the screen, “In this task, you will see letters. Each letter is shown for a few seconds. You need to decide if you saw the same letter 2 letters ago. If you did, press the ‘m’ key; if you did it correctly, you will see green colors around the letter. If you press the button when you should not, red will appear around the letter.” Participants were presented with stimuli one at a time and pressed a key if the current stimulus was the same as the one presented two trials previously (i.e., a match). A false alarm occurred when the participant pressed the key incorrectly (i.e., the letter was not the same as the letter two trials previously), and a miss when the participant did not press the key when it was a match (i.e., false negative). The stimulus set contained 15 capitalized alphabet letters (A, B, C, D, E, H, I. K, L, M, O, P, R, S, T), each presented for 500 ms. Participants had 2000 ms to respond. There were 25 trials in each of the three blocks. The mean completion time was 3:30 min. Variables of interest were the percentage of correctly matched items (NBMatch%), and the percentage of false alarms (NBFalsePos%).
Self-reported functioning (completed at T1 and T2)
Cognitive inflexibility (behaviour rating inventory of executive function, 2nd edition, self-report; BRIEF-2-SR; Gioia et al., Citation2015)
The BRIEF-2-SR is a 55-item self-report questionnaire measuring everyday executive functioning. We used the Shift subscale, which consists of eight items assessing the ability to make transitions, tolerate change, problem solve flexibly, and switch attention between tasks. We did not use normative scores from the manual, as our sample included participants over age 18. Raw scores ranged from 3 to 24, with greater scores indicating more difficulties with shifting. The standardized composite reliability (CR) in our sample was 0.90 (NDC) and 0.91 (control).
ADHD traits (youth externalizing behavior screener, YEBS; Arslan, Citation2019)
The YEBS 12-item self-report measure is composed of three subscales; we used the attention (AP) and hyperactivity/impulsivity (HA) problem subscales. Items are scored on a 4-point Likert scale from 0 (almost never) to 3 (almost always), with higher scores representing more behavioral problems. The CRs were 0.82 (HA; 0.82 NDC, 0.83 control) and 0.93 (AP; 0.91 NDC, 0.94 control).
Autistic traits (Autism quotient, AQ, Allison et al., Citation2012)
The AQ-10 is a 10-item measure with three different versions designed to screen for autistic traits in children, adolescents, and adults. Each item has four response options, with the total score ranging from 0 to 10. Higher scores indicate more autistic traits (Allison et al., Citation2012). The AQ-10 has high internal consistency and discriminative validity in autistic and non-autistic participants (Lundin et al., 2019). The CR was 0.79 (0.74 NDC, 0.81 control).
Emotional reactivity (Perth emotional reactivity scale short form, PERS-S; Preece et al., Citation2019)
The PERS-S is an 18-item scale measuring emotional reactivity. Items are scored on a 5-point Likert scale from 1 (very unlike me) to 5 (very like me). Higher scores represent greater emotional reactivity. We used the Positivity (PosER) and Negativity (NegER) subscales, conceptualized as resilience and risk factors, respectively. The CRs were 0.93 (positive; 0.92 NDC, 0.94 control) and 0.92 (negative; 0.91 NDC, 0.92 control).
Sleep
Sleep quality was assessed with a three-question scale adapted from the Adolescent Sleep Wake Scale (Huber et al., Citation2020). Participants were asked over the past three months, please answer the following, on average: 1) I have difficulty falling asleep, 2) I have difficulty staying asleep, and/or frequently waking during the night, 3) I wake early in the morning, and/or I still feel tired when I wake up. A total composite sleep score was derived ranging 0 to 3; higher scores indicated poorer sleep. The CR was 0.92 (0.91 NDC, 0.93 control).
Anxiety (generalised anxiety disorder-7, GAD-7; Spitzer et al., Citation2006)
The GAD-7 (Spitzer et al., Citation2006) consists of seven items measuring worry and anxiety symptoms over the past 2 weeks. Each item is scored on a 4-point Likert scale from 0 (not at all) to 3 (nearly every day). Higher scores indicate greater levels of anxiety. The CR was 0.92 in both groups.
Depression (patient health questionnaire-9, PHQ-9; Kroenke et al., Citation2001)
The PHQ-9 (Kroenke et al., Citation2001) consists of nine items measuring depressive symptoms over the past 2 weeks. Each item has a 4-point Likert response, with each item scored 0 (not at all) to 3 (nearly every day). Higher scores indicate greater depression severity. The CR was 0.91 (0.90 NDC, 0.92 control).
Self-reported psychiatric diagnoses
Self-reported current mental health diagnoses were recorded as present (1) or not present (0).
Data analysis
Code for the analyses is available on the first author’s OSF page: https://osf.io/6w7ar/. All analyses were performed in R software, version 4.2.1 (R Core Team, Citation2022), with packages JAGS (Plummer, Citation2016), jagsUI (Kellner, Citation2021), BMA (Raftery et al., Citation2022), and rstanarm (Goodrich et al., Citation2022). Standards for reporting Bayesian analyses were followed (Kruschke, Citation2021) and explanations on the use of Bayesian analyses are provided further in Appendix A.
Power analyses
Sample size simulations were performed using the BayesFactor package in R with Monte Carlo methods. A study with 100 participants (50 per group) can produce a conclusive Bayes Factor (i.e., outside the range of one-third to three), at d = 0.5, power of 80% and Type II error rate of 0.09. For NDC diagnostic group comparisons, Bayes Factor Design Analysis (Schönbrodt & Wagenmakers, Citation2018) indicated a minimum of 32 observations per group was required for a probability of 0.8, hence smaller effect sizes may not have been detected for individual NDC diagnostic groups. However, Sequential Bayes Factor analyses (i.e., simulations) conducted in rjags did not indicate support for the opposite hypothesis at larger sample sizes, providing confidence in our ability to detect effects.
Preliminary analyses
Order of testing effects were accounted for using counterbalancing (Backx et al., Citation2020), with participants randomly assigned to two conditions (ABCDE or EDBCA; A: SART, B: SST, C: WCST; D: N-Back; E: Corsi). Bayesian independent samples t-tests indicated the absence of order effects (BFs10 <1.3, δ 0.04 to 0.12). There were no missing data for those participants completing both the follow-up questionnaires and cognitive tests. Performance validity indicators on the neurocognitive tests were decided a priori (Weigard et al., Citation2019); no participants required exclusion, all those who commenced cognitive testing completed the five tasks, and there was no indication of a lack of task understanding or noncompliance. No multivariate outliers were identified. Assumption checks indicated tests of non-normality were appropriate.
Main analyses
To test the first hypothesis (H1) regarding group differences on self-rated and objectively measured neurocognitive variables at T2, we ran Bayesian independent samples t-tests using the normalized rank of the dependent variable. We chose a zero-centered Cauchy prior with width 0.707 for the standardized mean difference (effect size δ) and uniform prior for model probabilities. Robustness checks indicated that wide (1.0) or ultrawide priors (√2) did not alter the direction of results; hence, the findings were robust to reasonable changes in prior width given the data (Kruschke, Citation2021).
To understand the nature of the extent of these group differences, we then quantified relative cognitive weaknesses in the NDC siblings by converting raw scores of their T2 cognitive testing to z scores based on control group means (Brunsdon et al., Citation2015). We classified scores as low (>1.5 standard deviations; SD), within acceptable range (−1.5<x < 1.5 SD), or high (>1.5 SD) for each cognitive test score (see Table S3) compared to control group means for each variable. We examined proportions of participants with exactly k low test scores, excluding redundant measures (e.g., four reaction times; Abramovitch et al., Citation2021). We used the control group’s means due to lack of normative data for PsyToolkit. We then classified participants as having atypical EF performance if they had at least five low test scores (i.e., a base rate of <10% in the control group; Brooks & Iverson, Citation2010), to reflect the assumption in neuropsychology that at least one low score within a test battery is common amongst cognitively unimpaired individuals (Binder et al., Citation2009).
We then explored the role of NDC type (i.e., primary diagnosis of autism, ADHD, FASD, ID, or Rett syndrome), using Bayesian ANCOVAs with raw scores of neurocognitive and self-report functioning as dependent variables, age as the covariate, and NDC type as the fixed factor, with a uniform model prior. Multiplicity was accounted for by prior specification and correcting posterior odds by fixing to 0.5 the prior probability that the null hypothesis holds across comparisons (van den Bergh et al., Citation2020).
To test the second hypothesis (H2) regarding cross-sectional associations between EF and self-report functioning, we first examined Bayesian bivariate Kendall’s tau (τb) correlation coefficients for raw scores of all variables at T2 and candidate demographics to test as covariates, using a stretched beta prior width of 1.0. Bayesian methods provided robust estimation of regression parameters to mitigate collinearity; the Kolmogorov–Smirnov test confirmed univariate marginal posteriors converged (Assaf & Tsionas, Citation2021).
We then applied Bayesian network analyses (Marsman et al., Citation2022) to explore the conditional cross-sectional associations between T2 self-report and neurocognitive EF test variables, estimated using BDGraph (Mohammadi & Wit, Citation2019). We specified a uniform prior on the network structure (prior edge inclusion probability of 0.5) and a G-Wishart prior on the precision matrix with three degrees of freedom (Hinne et al., Citation2020). Parameter estimate plots included edges with an inclusion probability larger than 0.5 (Marsman et al., Citation2022; Mohammadi & Wit, Citation2019). Bayesian model averaging (BMA) was then used to obtain parameter precision averaged over all possible networks (Hinne et al., Citation2020). Centrality statistics (i.e., strength, closeness, betweenness, expected influence; Hevey, Citation2018) and 95% highest density intervals (HDIs) assessed the relative importance of each variable given the data, with higher values indicating stronger node connection.
To test the third hypothesis (H3) regarding the prediction of EF over time, we conducted Bayesian linear regressions using BMA (Hinne et al., Citation2020). This employed multi-model inference and is robust to model misspecification (Heck & Bockting, Citation2023). To identify the most probable model given the data, we examined model Bayes Factors (change from prior to posterior odds; BFM) and posterior probabilities of the model given the data (P(M∣data)). To identify predictors, we examined posterior summaries and BF inclusion criteria (BFinc; the change from prior to posterior odds for one predictor, comparing all models with that predictor to all models without it, relative to the data). Null models included T2 age and autistic traits. Raw scores of T1 self-report variables were entered together as potential predictors of k number of low test scores at T2. We chose a Jeffreys-Zellner-Siow (JZS) prior (r scale 0.354; Liang et al., Citation2008), and uniform prior for model probability (Gronau et al., Citation2021).
Bayesian inference criteria
The Bayes Factor, BF10, reflects evidence favoring the alternative hypothesis (i.e., change from prior to posterior odds), while BF01 provides evidence for the null hypothesis. BFs10 >3 were considered evidence for the alternative hypothesis, while inconclusive BFs10 (i.e., one-third to 3) indicated absence of evidence for either hypothesis (Rouder & Morey, Citation2012). Bayesian parameter estimation was then used to examine the uncertainty of estimated effects, with 95% HDIs not including zero considered evidence of an effect (Kruschke, Citation2018). A small, standardized effect size (δ) was defined as 0.2 ≤ δ <0.5 or −0.5 < δ ≤ −0.2 (Cohen, Citation2013).
Results
Demographics
See for T2 characteristics of participants and their NDC siblings. Demographic comparisons demonstrated group equivalence between control and NDC groups (all BFs10 <3) other than higher parental divorce in the NDC group.
Table 1. Characteristics of the participants and their siblings at T2.
Group comparisons between control and NDC siblings at T2
Comparing raw scores at T2, NDC siblings had poorer EF performance compared to controls (), with longer SSRTs (BF10 72.56, δ = −0.63), lower N-Back matches (BF10 69.01, δ = 0.64) and greater false positives (BF10 53.67, δ = −0.59), more SART omissions (BF10 42.65, δ = −0.61) and commissions (BF10 30.36, δ = −0.53), and WCST perseverative errors (BF10 39.17, δ = −0.58). Examining k number of low test scores (based on z scores), although most (70.31%) NDC siblings performed within typical ranges, 19 (29.69%) were classified as atypical (i.e., >4 test scores lower than the 1.5 SD criterion, which had a base rate of <10% in the control group), compared to six controls (9.38%) (Table S3).
Table 2. Descriptive statistics and Bayesian Mann–Whitney independent samples T-Tests at T2.
At T2, 65.63% of the NDC group and 37.50% of the control group reported at least one psychiatric diagnosis (BF10 3.37, δ = −0.41), and poorer self-reported functioning across all measures based on raw score comparisons ().
We then compared NDC subgroups, finding siblings of persons with FASD (n = 14), followed by autism (n = 17), had poorer broad EF (k low scores), individual EF test performance, and worse self-reported functioning than other NDC groups and controls given the data (posterior odds and BFs10 >100; Table S4), although ADHD siblings (n = 23) had the poorest SST performance of all groups.
Cross-sectional T2 interrelations between EF and self-reported functioning
We first examined bivariate Bayesian correlations between raw scores for EF tasks and self-reported subjective functioning cross-sectionally at T2 in the NDC group siblings. We observed cross-task correlations between working memory and attention (Corsi, SART, and N-Back; τb 0.25 to 0.29), while WCST perseverative errors were weakly associated with poorer Corsi span (τb = −0.14) and fewer N-Back matches (τb = −0.19). There were strong associations between self-report and objective measures purported to measure the same construct (i.e., poorer SART with self-rated inattention, τb = 0.39; more WCST perseverative errors with greater self-report poorer shifting; τb = 0.49). Self-reported categorical psychiatric (DSM-5-TR) diagnoses were associated with worse EF across WCST, SART, and N-Back (τb 0.19 to 0.30). In terms of demographics, younger age was associated with poorer general EF; and more severe NDC symptoms in participants’ siblings with NDCs were associated with more participant WCST errors and SART omissions.
We next estimated Bayesian networks of T2 objective testing and self-reported variables for the NDC group siblings. Centrality indices revealed negative emotion reactivity, N-Back matches, and Corsi were the most influential and central to the network given all other variables, although WCST perseverative errors had the highest betweenness (i.e., falling most often on the path between two other nodes; see ; see also Tables S4 and S5; Figures S1-S4).
Figure 2. NDC sibling group Bayesian posterior parameter Plot.
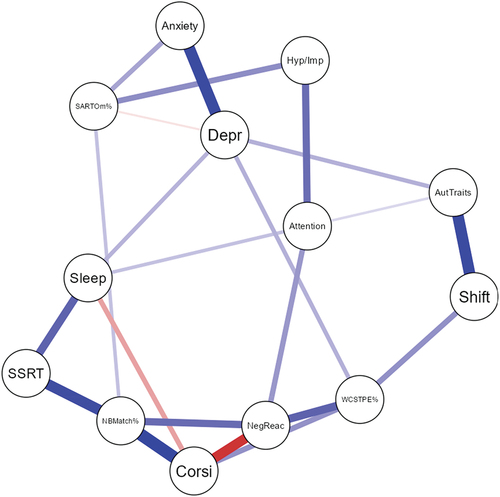
T1 self-reported functioning as predictors of T2 EF performance
Preliminary Bayesian regression analyses revealed low posterior inclusion probabilities with HDIs encompassing the null for demographics and medication status predicting EF performances; as such, we covaried only age and autistic traits.
Within the NDC group, we first modeled k low test scores as the dependent variable, observing the main effects of younger age, greater negative emotion reactivity, and more sleep problems in the most probable model (BFM 5.76, R2 = 0.48; ). The three most likely models have largely similar probabilities, indicating some uncertainty in the data; posterior inclusion probabilities of coefficients and 95% HDIs should thus also be evaluated. We next examined T1 self-reported functioning predicting individual EF test performance in the NDC group (Table S7). For better Corsi span, the most probable model included greater negative emotion reactivity and inattention (BFM 13.65, R2 0.58). More SART omission errors had main effects of higher anxiety, depression, inattention, and younger age (BFM = 6.44, R2 = 0.31). Longer SSRTs were predicted by less hyperactivity/impulsivity and more sleep problems (BFM 6.83, R2 = 0.33). More N-Back matches had main effects of better attention (BFM 11.73, R2 = 0.60). More WCST perseverative errors had effects of greater negative emotion reactivity, BRIEF shift, and sleep problems (BFM 6.77, R2 = 0.48). More SART commissions were predicted by greater negative and positive emotion reactivity and inattention (BFM 10.68; R2 = 0.49).
Table 3. T1 self-reported variables predicting k low test scores at T2 in the NDC group.
Discussion
The present study explored the EF profiles and self-reported functioning of siblings of persons with and without NDCs. There were three key findings. First, as hypothesized (H1), the NDC group demonstrated poorer objectively measured EF and worse self-reported functioning than controls. The poorest self-reported and objective EF functioning was observed for the FASD and, to a lesser extent, autism siblings, compared to other NDCs and controls. Second, as predicted (H2), the NDC group was characterized by interrelated EF difficulties, predominantly attention and working memory, with strong cross-sectional associations with poorer psychosocial functioning. Third, as anticipated (H3), greater T1 self-reported attention problems, sleep difficulties, and negative emotion reactivity consistently predicted poorer T2 EF task performance for NDC siblings.
Group comparisons of EF and self-reported functioning
Compared to controls, NDC siblings had poorer EF test performance and self-reported cognitive and psychosocial functioning. While most had fewer than five low test scores, a minority (29.69%) demonstrated greater difficulties (five to ten low scores). Our results are consistent with previous findings in studies of siblings of persons with ADHD and autism only indicating broad EF difficulties (e.g., Bidwell et al., Citation2007; Hughes et al., Citation1999; Rommelse et al., Citation2008) yet incongruent with other literature suggesting no group differences (McLean et al., Citation2014; Seidman et al., Citation2000). We suggest that these inconsistencies may be attributable to varying ages, smaller samples, and homogeneous NDC groups in previous studies, in addition to task modality (e.g., load versus complexity, verbal or visual; Mukherjee et al., Citation2021) and heterogeneity in the neural basis of these problems (Hoogman et al., Citation2017).
We observed evidence of condition specificity. In contrast to some findings (Rosa et al., Citation2017), siblings of autistic individuals had more attention problems (self-rated inattention, SART omissions, longer SSRTs), sleep problems and anxiety compared to all other groups. FASD siblings displayed diffuse EF difficulties, in keeping with findings of broad neurocognitive dysfunction (Kvigne et al., Citation2009) and self-rated psychosocial disparities in these siblings (Marquis et al., Citation2020; Wolff et al., Citation2023). The more pronounced difficulties among these two groups may be attributable to genetic vulnerability to NDC traits and ongoing environmental and family stressors (Kovshoff et al., Citation2017; Wolff et al., Citation2022) and may, to some extent, reflect missed NDC diagnoses (May et al., Citation2018; Sharma et al., Citation2018). This reinstates the importance of early screening and health practitioner education toward better case formulation. Further, observed high rates of psychological difficulties are also likely maintained and exacerbated poorer neurocognition (Abramovitch et al., Citation2021; Wong et al., Citation2006).
Associations between EF performance and self-reported psychosocial functioning
In characterizing cross-sectional interrelations between neurocognitive and subjective functioning, we found that negative emotion reactivity, working memory, and attention difficulties (poorer N-Back match and Corsi span) were central to the NDC sibling Bayesian network, implicating self-regulation and working memory in the maintenance of functional difficulties and psychopathology.
Examining bivariate correlations, self-reported EF and EF test performance were associated, with medium effect sizes, validating and extending our previous findings (Wolff et al., Citation2023). Strong associations were observed between objective measures of working memory and attention, consistent with complex span and tracking tasks representing both the multicomponent model of working memory (Baddeley, Citation1986) and the executive control component of attention (Peterson & Posner, Citation2012; Treviño et al., Citation2021). Further, the observed interrelations between greater N-Back false positives, SART commission errors, and poorer Corsi suggested that compromised working memory and visual attention at the encoding stage also contribute to poorer inhibition (Friedman & Miyake, Citation2017; Tarle et al., Citation2019).
The WCST was associated with BRIEF shift and autistic traits, demonstrating cross-modality convergent validity (Landry et al., Citation2021). Both types of errors were associated with poorer Corsi and N-Back, suggesting that shifting difficulties observed in NDCs may, in part, underlie selective attention and working memory difficulties, specifically poorer updating and reduced efficiency of feedback utilization (Ashendorf & McCaffrey, Citation2008), in addition to cognitive inflexibility (Demetriou et al., Citation2019). Non-perseverative errors were relatively uncompromised compared to perseverative errors, suggesting less difficulty with trial-and-error problem-solving (Barceló & Knight, Citation2002). Overall, although there was intra- and inter-individual variability in EF, NDC siblings appeared to have most difficulty with attention and working memory. Consequently, understanding possible risk and protective factors over time for these difficulties is critical.
T1 self-reported predictors of T2 EF performance
To extend our T2 cross-sectional examination, we explored whether T1 self-reported risk factors predicted EF task performance in NDC siblings after 15 months. T1 negative emotion reactivity and sleep problems predicted atypical T2 EF (k low test scores) over and above the effects of subclinical NDC traits. Although associations between EF and psychopathology are likely transactional (Abramovitch et al., Citation2021), our findings add to empirical evidence that individuals with higher negative emotionality may be more vulnerable to psychopathology and EF problems (Carver et al., Citation2017), and that earlier sleep disturbances predict later executive dysfunction (e.g., attentional problems, hyperactivity; Nilsson et al., Citation2005). Sleep problems may also drive hypo-arousal associated with poorer EF (Sergeant, Citation2005; Wolff et al., Citation2021), in addition to psychopathology.
Demographics had minimal impact on EF, other than participants’ siblings’ NDC severity (as perceived by participants) being associated with more WCST errors and SART omissions, suggesting chronic stress experienced by siblings of persons with more severe phenotypes may amplify subtle cognitive differences, particularly in problem-solving, shifting, and attention (Constantino, Citation2018; Pilowsky et al., Citation2007), rendering them more vulnerable to negative long-term outcomes. Although older age was a predictor of better EF performance in both groups, reflecting known effects of brain maturation (Diamond, Citation2013), EF difficulties were observed in the NDC group across ages, consistent with evidence that these difficulties are maintained over time in NDC siblings compared to age-related EF improvements in controls (van Lieshout et al., Citation2019). Findings regarding the prediction of EF difficulties are preliminary given the directionality tested. Nonetheless, as this is the first NDC sibling study examining self-reported functioning as a predictor of EF, these findings are clinically important and form the foundation for future research.
Clinical implications
There are three key clinical implications. First, regarding group comparisons, although most NDC siblings did not have objectively atypical EF, overall, they displayed poorer cognitive and self-reported functioning compared to controls. We recommend individualized risk identification and preventative methods to attenuate difficulties, given evidence that the gap between NDC siblings and their same-aged peers can continue to grow throughout young adulthood (van Lieshout et al., Citation2019). These group differences were particularly pronounced in siblings of autistic individuals and those with FASD. Early and targeted support for these subgroups may be beneficial to, at least in part, mitigate more adverse psychosocial outcomes (Haukeland et al., Citation2020; Wolff et al., Citation2022). For instance, affordable support programs, parental psychoeducation, outreach, and school-based prevention efforts may assist in reducing the cumulative impact of other individual-level risk factors (Reid et al., Citation2022). As environmental stress is a transdiagnostic risk factor for psychopathology and EF difficulties (Snyder et al., Citation2015), it is important for practitioners to consider such external variables in individualizing assessment and intervention.
Second, regarding cross-sectional associations, our network analysis revealed greater negative emotionality and anxiety, poorer attention, and reduced working memory mutually reinforced difficulties in other EF domains and self-reported functioning. Critically, working memory is a strong prognostic factor for fluid intelligence (Heitz et al., Citation2006) and competency in emotional processing and social relationships (Groves et al., Citation2022). Working memory training can improve anxiety and emotion dysregulation and may have far transfer effects on psychosocial, academic, occupational, and other cognitive domains (Habib et al., Citation2019), thus being a salient transdiagnostic intervention target (Barkus, Citation2020). Similarly, interventions targeting siblings’ self-regulation and anxiety are also likely to improve everyday EF (Veloso & Ty, Citation2021). Further, mindfulness, cognitive bias modification training, and attentional control interventions may improve executive attention, positive reappraisal, and anxiety in adolescents (Slattery et al., Citation2022). Many of these strategies are available via accessible modalities such as single session interventions (Schleider & Weisz, Citation2018) or free smartphone applications (Jalal et al., Citation2018). Additionally, daily life complexities can impact outcomes in siblings with poorer EF, suggesting that strategies to simplify the unique everyday challenges siblings face may be beneficial to reduce load on EF resources (Suchy et al., Citation2020). As response to psychosocial and pharmacological interventions may be dependent on EF abilities (Snyder et al., Citation2015), pre-treatment EF screening is important for tailoring support.
Third, regarding prediction of EF over time, greater earlier self-reported sleep difficulties and negative emotion reactivity were risk factors for poorer common and specific EF 15 months later, adjusting for age and autistic traits. Given the efficacy of sleep interventions in children with NDCs (Malkani et al., Citation2022), we encourage similar support for siblings, particularly as sleep difficulties co-aggregate in NDC families (Taylor et al., Citation2022). There is also evidence for positive effects of emotion regulation and reactivity interventions in children and adolescents (Eadeh et al., Citation2021; Wade et al., Citation2020) which may improve EF (Pe et al., Citation2015). Clinical neuropsychologists should thus be considering the effects of poorly regulated emotions on cognitive functioning. Additionally, given the fluidity of these emotional states, it is possible that EF is a fluctuating entity (Suchy, Citation2020), reliant on NDC siblings’ intra-individual variability in daily functioning and psychosocial stressor management.
Study limitations and future directions
Our findings are based on two time points, without a measure of EF test performance at T1. Results require replication in larger samples, as most participants were female (73.44%), White Caucasian (75.78%), living in Australia (100%), and middle-high income (78.10%), with small numbers in some NDC subgroups, limiting generalizability. As EF skills are influenced by demographics such as ethnicity and socioeconomic disadvantage (Mooney et al., Citation2022), future research should ensure representation of minority populations within sibling groups, and validation of culturally responsive assessment methods. Direct system-level resource investment may improve accessibility to health literacy, screening, and intervention for lower-income families impacted by NDCs (Hurt et al., Citation2016), capitalizing on the ubiquity of smartphone access (McCool et al., Citation2022).
Self-report variables may be vulnerable to social desirability bias, common method variance, misunderstanding questions, apathy, low self-efficacy, and limited self-awareness common among individuals with psychiatric conditions (Abramovitch et al., Citation2021; Rosenman et al., 2011). We could not independently verify participants’ self-reported psychiatric diagnoses, nor their siblings’ NDCs. Future studies could integrate brief telephone cognitive screening or use of medical records for clinicians to verify diagnoses. Siblings in both groups reported mental health diagnoses greater than current estimates in the general population globally during COVID-19 (i.e., 28% depression, 26.9% anxiety, 50% psychological distress, 27.6% sleep problems; Nochaiwong et al., Citation2021). We may thus be observing, at least to some extent, an artifact of the COVID-19 pandemic, with elevated psychiatric diagnoses (Santomauro et al., Citation2021) and disproportionate negative effects on families with NDCs (Asbury et al., Citation2021). The findings may also reflect better awareness of mental health vulnerabilities.
Methodologically, we acknowledge EF task impurity, and that test-specificity may partially explain observed associations (Friedman & Miyake, Citation2017; Kofler et al., Citation2019), although they correlated with our self-reported measures of behavioral EF, providing some evidence of cross-modal reliability. Heterogeneous operationalization of EF tasks across studies may lead to misinterpretation of deficits (Meule, Citation2017). We did not include a control measure of cognitive performance (e.g., word reading, Corsi forwards, 0-Back) which may have better delineated construct specificity; we also did not assess whether general intelligence levels were comparable between groups. Further, motor control deficits are reported in NDC siblings (Slaats-Willemse et al., Citation2003) which may influence reaction times on computerized tasks (Rommelse et al., Citation2008). Additionally, reaction time variability is consistently associated with attentional difficulties in individuals with ADHD (Wolff et al., Citation2021), which is worthy of further investigation in NDC siblings generally. Finally, we directly measured “cold” EF, and encourage research into “hot” EF (i.e., affective, reward-based processes), given that these functions are differentially related to psychopathology (Yang et al., Citation2022; Zelazo, Citation2020). Future research may seek to investigate the degree to which NDC siblings’ cognitive and psychological symptomatology reflects causal associations between genetic liability to NDC traits and gene–environment interactions underlying both typical and atypical development, given evidence that identical genotypes can result in different behavioral outcomes (Bontinck et al., Citation2018).
Statistically, network centrality indices should be interpreted conservatively, given they assume the absence of latent confounding (Hallquist et al., Citation2021), and Bayesian analyses are relative given the data (Kruschke, Citation2018). However, this study was added to literature in the field (e.g., Crawford et al., Citation2009; Gavett, Citation2015) in emphasizing the utility of Bayes theorem to inform clinical decision-making and translate group-level findings to individual patients (Gelman & Geurts, Citation2017). We also suggest that longitudinal studies optimize ecological momentary assessment, for which norms are less necessary due to the within-subject comparison method, to better understand intra-individual variation in NDC siblings’ cognitive abilities (Schmitter-Edgecombe et al., Citation2020).
Although remote experimental paradigms may improve ecological validity and provide scalable opportunities to reduce healthcare disparities (Hewitt et al., Citation2022), there are limitations, including lack of standardization, absence of psychometric data, and confounding associated with environmental differences and hardware or software variability (Kessels, Citation2019, Parsons et al., Citation2018; Treviño et al., Citation2021). We encourage the development of normative data for remote neuropsychological assessment to advance interpretability of web-based test scores and enable better clinical judgment (Bauer et al., Citation2012; Van Patten, Citation2021). Without normative data, we cannot state whether both groups performed within age-expected ranges (Abramovitch et al., Citation2021). We emphasize that the effect sizes of group comparisons are not indicators of the magnitude of siblings’ difficulties, and that atypical test scores in themselves are not pathognomonic impairment (Gavett, Citation2015; Guilmette et al., Citation2020). Further, we did not use performance validity tests (PVTs), although poor effort is less likely with voluntary participation (Binder et al., Citation2009).
Finally, there is a paucity of research addressing the neuropsychological profile of siblings into older adulthood, despite evidence that persons with NDCs are at increased risk of accelerated age-related neuropathology (Janicki et al., Citation2022). As siblings may share this risk, research into the aging process in NDC siblings with poorer cognitive functioning and mental health concerns and lifespan barriers to assessment and care is critical.
Conclusion
This study provided crucial insights into the multifaceted nature of EF difficulties and subjective functioning of adolescent and young adult NDC siblings, identifying potential transdiagnostic intervention targets as working memory, attention, sleep, and negative emotion reactivity. We suggest that sibling EF difficulties may not reflect core endophenotypes of NDCs but instead may be the product of dynamic interrelations between heterogeneous external and individual-level psychosocial, contextual, and developmental factors. There remain important areas of advancement for the field of clinical neuropsychology, including improved understanding of the mechanisms behind neurocognitive processes among NDC siblings and identification of their unique risk and resilience factors to ensure utility of recommendations. As the clinical neuropsychology landscape rapidly evolves, the incorporation of new methodologies and disruptive technologies into research and practice could enhance clinical decision-making. We encourage adaptation of testing approaches to improve efficiency and accessibility (Bilder & Reise, Citation2019) and to optimize digital neuropsychology’s promise of flexibility, precision, and scalability (Van Patten, Citation2021). These findings highlight the incremental value of cognitive screening in identifying clinically meaningful atypicalities associated with siblings’ subjective functional difficulties. There is a need for not only to attenuate these vulnerabilities with individualized interventions but also adopt a biopsychosocial approach to promote positive environments which enhance protective factors and sibling strengths.
Research support and ethics
This research was funded by an Australian National Health and Medical Research Council (NHMRC) grant (1184770) and ethical approval was granted by the Human Ethics Office at The University of Western Australia (2020/ET000049).
Author contribution statement
EG obtained funding. BW conceptualized the current study, designed the survey, programmed the experimental cognitive paradigms, extracted and analyzed the data, and composed the first manuscript draft. VF advised on and reviewed the Methods and Results. All authors reviewed the earlier drafts, approved the final version, and agreed to be accountable for the contents of this manuscript.
Supplemental Material
Download MS Word (788.8 KB)Acknowledgments
The authors would like to thank the Sibling Advisory Group, part of the larger Sibling Project, for their valuable contribution and for representing siblings of individuals with neurodevelopmental conditions.
Disclosure statement
No potential conflict of interest was reported by the author(s).
Data availability statement
The data that support the findings of this study are available on request from the corresponding author, BW. The data are not publicly available due to information that could compromise the privacy of participants. The analytic code necessary to reproduce the analyses presented in this paper is available on the first author’s OSF page: https://osf.io/u4gaj.
Supplementary material
Supplemental data for this article can be accessed online at https://doi.org/10.1080/13803395.2023.2259042
Additional information
Funding
Notes
1. “Neurodevelopmental disorders” in the Diagnostic and Statistical Manual for Mental Disorders, Fifth Edition, Text-Revision (DSM-5-TR; American Psychiatric Association, Citation2022) are hereafter referred to as neurodevelopmental conditions (NDCs).The term “NDC siblings” refers to unaffected siblings of persons with NDCs, while “sibling with an NDC” refers to their affected proband.
2. Although different groups and individuals have different language choice preferences, this study adopts person-first language. This decision was made after consultation with our Sibling Advisory Group. However, for autism-related discussions, identity-first language will be used (i.e., autistic individuals), reflecting a common consensus amongst the autistic community (Amaral, Citation2023; Taboas et al., Citation2023).
References
- Abramovitch, A., Short, T., & Schweiger, A. (2021). The C factor: Cognitive dysfunction as a transdiagnostic dimension in psychopathology. Clinical Psychology Review, 86, 102007. https://doi.org/10.1016/j.cpr.2021.102007
- Allison, C., Auyeung, B., & Baron-Cohen, S. (2012). Toward brief “red flags” for autism screening: The short autism spectrum quotient and the short quantitative checklist for autism in toddlers in 1,000 cases and 3,000 controls [corrected]. Journal of the American Academy of Child and Adolescent Psychiatry, 51(2), 202–212.e7. https://doi.org/10.1016/j.jaac.2011.11.003
- Amaral, D. G. (2023). Language in Autism Research: Accurate and respectful. Autism Research, 16(1), 7–8. https://doi.org/10.1002/aur.2886
- American Psychiatric Association. (2022). Diagnostic and statistical manual of mental disorders (5th ed., text rev). https://doi.org/10.1176/appi.books.9780890425787
- Arán Filippetti, V., Krumm, G. L., & Raimondi, W. (2020). Computerized versus manual versions of the Wisconsin card sorting test: Implications with typically developing and ADHD children. Applied Neuropsychology. Child, 9(3), 230–245. https://doi.org/10.1080/21622965.2019.1570198
- Arce, T., & McMullen, K. (2021). The Corsi block-tapping test: Evaluating methodological practices with an eye towards modern digital frameworks. Computers in Human Behavior Reports, 4, 100099. https://doi.org/10.1016/j.chbr.2021.100099
- Arslan, G. (2019). Development and validation of the youth externalizing behavior screener: A brief and effective measure of behavioral problems. International Journal of School & Educational Psychology, 7(Suppl 1), 64–74. https://doi.org/10.1080/21683603.2018.1466747
- Asbury, K., Fox, L., Deniz, E., Code, A., & Toseeb, U. (2021). How is COVID-19 affecting the mental health of children with special educational needs and disabilities and their families? Journal of Autism and Developmental Disorders, 51(5), 1772–1780. https://doi.org/10.1007/s10803-020-04577-2
- Ashendorf, L., & McCaffrey, R. J. (2008). Exploring age-related decline on the Wisconsin card Sorting test. The Clinical Neuropsychologist, 22(2), 262–272. https://doi.org/10.1080/13854040701218436
- Assaf, A. G., & Tsionas, M. (2021). Testing for collinearity using Bayesian analysis. Journal of Hospitality & Tourism Research, 45(6), 1131–1141. https://doi.org/10.1177/1096348021990841
- Backx, R., Skirrow, C., Dente, P., Barnett, J. H., & Cormack, F. K. (2020). Comparing web-based and lab-based cognitive assessment using the Cambridge Neuropsychological Test Automated Battery: A within-subjects counterbalanced study. Journal of Medical Internet Research, 22(8), e16792. https://doi.org/10.2196/16792
- Baddeley, A. D. (1986). Working memory. Oxford University Press.
- Bailey, R., & Jones, S. M. (2019). An integrated model of regulation for applied settings. Clinical Child & Family Psychology Review, 22(1), 2–23. https://doi.org/10.1007/s10567-019-00288-y
- Barceló, F., & Knight, R. T. (2002). Both random and perseverative errors underlie WCST deficits in prefrontal patients. Neuropsychologia, 40(3), 349–356. https://doi.org/10.1016/S0028-3932(01)00110-5
- Barkus, E. (2020). Effects of working memory training on emotion regulation: Transdiagnostic review. Psycho Journal, 9(2), 258–279. https://doi.org/10.1002/pchj.353
- Bauer, R. M., Iverson, G. L., Cernich, A. N., Binder, L. M., Ruff, R. M., & Naugle, R. I. (2012). Computerized neuropsychological assessment devices: Joint position paper of the American Academy of Clinical Neuropsychology and the National Academy of Neuropsychology. Archives of Clinical Neuropsychology: The Official Journal of the National Academy of Neuropsychologists, 27(3), 362–373. https://doi.org/10.1093/arclin/acs027
- Berg, E. A. (1948). A simple objective technique for measuring flexibility in thinking. The Journal of General Psychology, 39(1), 15–22. https://doi.org/10.1080/00221309.1948.9918159
- Bidwell, L. C., Willcutt, E. G., Defries, J. C., & Pennington, B. F. (2007). Testing for neuropsychological endophenotypes in siblings discordant for attention-deficit/hyperactivity disorder. Biological Psychiatry, 62(9), 991–998. https://doi.org/10.1016/j.biopsych.2007.04.003
- Bilder, R. M. (2011). Neuropsychology 3.0: Evidence-based science and practice. Journal of the International Neuropsychological Society: JINS, 17(1), 7–13. https://doi.org/10.1017/S1355617710001396
- Bilder, R. M., & Reise, S. P. (2019). Neuropsychological tests of the future: How do we get there from here? The Clinical Neuropsychologist, 33(2), 220–245. https://doi.org/10.1080/13854046.2018.1521993
- Binder, L. M., Iverson, G. L., & Brooks, B. L. (2009). To err is human: “abnormal” neuropsychological scores and variability are common in healthy adults. Archives of Clinical Neuropsychology: The Official Journal of the National Academy of Neuropsychologists, 24(1), 31–46. https://doi.org/10.1093/arclin/acn001
- Bontinck, C., Warreyn, P., Van der Paelt, S., Demurie, E., Roeyers, H., & Salley, B. (2018). The early development of infant siblings of children with autism spectrum disorder: Characteristics of sibling interactions. PloS One, 13(3), e0193367. https://doi.org/10.1371/journal.pone.0193367
- Bronfenbrenner, U. (1995). Developmental ecology through space and time: A future perspective. In P. M. In, G. H. Elder Jr., & K. Lüscher (Eds.), Examining lives in context: Perspectives on the ecology of human development (pp. 619–647). American Psychological Association.
- Brooks, B. L., & Iverson, G. L. (2010). Comparing actual to estimated base rates of “Abnormal” scores on neuropsychological test batteries: Implications for interpretation. Archives of Clinical Neuropsychology, 25(1), 14–21. https://doi.org/10.1093/arclin/acp100
- Brunsdon, V. E., Colvert, E., Ames, C., Garnett, T., Gillan, N., Hallett, V., Lietz, S., Woodhouse, E., Bolton, P., & Happé, F. (2015). Exploring the cognitive features in children with autism spectrum disorder, their co-twins, and typically developing children within a population-based sample. Journal of Child Psychology and Psychiatry, and Allied Disciplines, 56(8), 893–902. https://doi.org/10.1111/jcpp.12362
- Carver, C. S., Johnson, S. L., & Timpano, K. R. (2017). Toward a functional view of the p factor in psychopathology. Clinical Psychological Science, 5(5), 880–889. https://doi.org/10.1177/2167702617710037
- Channon, S. (1996). Executive dysfunction in depression: The Wisconsin card Sorting test. Journal of Affective Disorders, 39(2), 107–114. https://doi.org/10.1016/0165-0327(96)00027-4
- Chien, Y.-L., Chou, M.-C., Chiu, Y.-N., Chou, W.-J., Wu, Y.-Y., Tsai, W.-C., & Gau, S. S.-F. (2017). ADHD-related symptoms and attention profiles in the unaffected siblings of probands with autism spectrum disorder: Focus on the subtypes of autism and Asperger’s disorder. Molecular Autism, 8(1), 37. https://doi.org/10.1186/s13229-017-0153-9
- Claessen, M. H. G., van der Ham, I. J. M., & van Zandvoort, M. J. E. (2015). Computerization of the standard Corsi block-tapping task affects its underlying cognitive concepts: A Pilot study. Applied Neuropsychology. Adult, 22(3), 180–188. https://doi.org/10.1080/23279095.2014.892488
- Cohen, J. (2013). Statistical power analysis for the behavioral sciences. Routledge.
- Constantino, J. N. (2018). Deconstructing autism: From unitary syndrome to contributory developmental endophenotypes. International Review of Psychiatry (Abingdon, England), 30(1), 18–24. https://doi.org/10.1080/09540261.2018.1433133
- Corsi, P. M. (1972). Human memory and the medial temporal region of the brain. Doctoral Thesis at McGill University (Canada). Download from here. (in the search box, ty“e “Co”si”)
- Crawford, J. R., Garthwaite, P. H., & Betkowska, K. (2009). Bayes’ theorem and diagnostic tests in neuropsychology: Interval estimates for post-test probabilities. The Clinical Neuropsychologist, 23(4), 624–644. https://doi.org/10.1080/13854040802524229
- Delorme, R., Goussé, V., Roy, I., Trandafir, A., Mathieu, F., Mouren-Siméoni, M. C., Betancur, C., & Leboyer, M. (2007). Shared executive dysfunctions in unaffected relatives of patients with autism and obsessive-compulsive disorder. European Psychiatry : The Journal of the Association of European Psychiatrists, 22(1), 32–38. https://doi.org/10.1016/j.eurpsy.2006.05.002
- Demetriou, E. A., DeMayo, M. M., & Guastella, A. J. (2019). Executive function in autism spectrum disorder: History, theoretical models, empirical findings, and potential as an Endophenotype. Frontiers in Psychiatry, 10, 10. https://doi.org/10.3389/fpsyt.2019.00753
- Diamond, A. (2013). Executive functions. Annual Review of Psychology, 64(1), 135–168. https://doi.org/10.1146/annurev-psych-113011-143750
- Donati, G., Meaburn, E., & Dumontheil, I. (2021). Internalising and externalising in early adolescence predict later executive function, not the other way around: A cross-lagged panel analysis. Cognition & Emotion, 35(5), 986–998. https://doi.org/10.1080/02699931.2021.1918644
- Duckworth, A. L., & Kern, M. L. (2011). A meta-analysis of the convergent validity of self-control measures. Journal of Research in Personality, 45(3), 259–268. https://doi.org/10.1016/j.jrp.2011.02.004
- Eadeh, H.-M., Breaux, R., & Nikolas, M. A. (2021). A meta-Analytic Review of emotion regulation focused psychosocial interventions for adolescents. Clinical Child and Family Psychology Review, 24(4), 684–706. https://doi.org/10.1007/s10567-021-00362-4
- Flouri, E., Papachristou, E., Midouhas, E., Ploubidis, G. B., Lewis, G., & Joshi, H. (2019). Developmental cascades of internalising symptoms, externalising problems and cognitive ability from early childhood to middle adolescence. European Psychiatry: The Journal of the Association of European Psychiatrists, 57, 61–69. https://doi.org/10.1016/j.eurpsy.2018.12.005
- Fombonne, E., Bolton, P., Prior, J., Jordan, H., & Rutter, M. (1997). A family study of autism: Cognitive patterns and levels in parents and siblings. Journal of Child Psychology and Psychiatry, and Allied Disciplines, 38(6), 667–683. https://doi.org/10.1111/j.1469-7610.1997.tb01694.x
- Friedman, N. P., & Miyake, A. (2017). Unity and diversity of executive functions: Individual differences as a Window on cognitive structure. Cortex, 86, 186–204. https://doi.org/10.1016/j.cortex.2016.04.023
- Fusar-Poli, L., Brondino, N., Politi, P., & Aguglia, E. (2022). Missed diagnoses and misdiagnoses of adults with autism spectrum disorder. European Archives of Psychiatry and Clinical Neuroscience, 272(2), 187–198. https://doi.org/10.1007/s00406-020-01189-w
- Gavett, B. E. (2015). The value of Bayes’ theorem for interpreting Abnormal test scores in cognitively healthy and Clinical samples. Journal of the International Neuropsychological Society, 21(3), 249–257. https://doi.org/10.1017/S1355617715000168
- Gelman, A., & Geurts, H. M. (2017). The statistical crisis in science: How is it relevant to clinical neuropsychology? The Clinical Neuropsychologist, 31(6–7), 1000–1014. https://doi.org/10.1080/13854046.2016.1277557
- Gioia, G. A., Isquith, P. K., Guy, S. C., & Kenworthy, L. (2015). Behavior rating inventory of executive function®, (Second Edition (BRIEF®2). PAR Inc.
- Goodrich, B., Gabry, J., Ali, I., & Brilleman, S. (2022). “Rstanarm: Bayesian applied regression modeling via Stan.” R package version 2.21.3, https://mc-stan.org/rstanarm/.
- Grant, S., Wendt, K. E., Leadbeater, B. J., Supplee, L. H., Mayo-Wilson, E., Gardner, F., & Bradshaw, C. P. (2022). Transparent, open, and reproducible prevention Science. Prevention Science, 23(5), 701–722. https://doi.org/10.1007/s11121-022-01336-w
- Gronau, Q. F., Heck, D. W., Berkhout, S. W., Haaf, J. M., & Wagenmakers, E.-J. (2021). A primer on Bayesian model-averaged meta-analysis. Advances in Methods and Practices in Psychological Science, 4(3). https://doi.org/10.1177/25152459211031256
- Gross, J. J., & Jazaieri, H. (2014). Emotion, emotion regulation, and psychopathology: An affective science perspective. Clinical Psychological Science, 2(4), 387–401. https://doi.org/10.1177/2167702614536164
- Groves, N. B., Wells, E. L., Soto, E. F., Marsh, C. L., Jaisle, E. M., Harvey, T. K., & Kofler, M. J. (2022). Executive functioning and emotion regulation in children with and without ADHD. Research on Child and Adolescent Psychopathology, 50(6), 721–735. https://doi.org/10.1007/s10802-021-00883-0
- Gruters, A. A., Ramakers, I. H., Verhey, F. R., Kessels, R. P., & de Vugt, M. E. (2022). A scoping Review of communicating neuropsychological test results to patients and family members. Neuropsychology Review, 32(2), 294–315. https://doi.org/10.1007/s11065-021-09507-2
- Guilmette, T. J., Sweet, J. J., Hebben, N., Koltai, D., Mahone, E. M., Spiegler, B. J., Stucky, K., & Westerveld, M. (2020). American Academy of Clinical Neuropsychology consensus conference statement on uniform labeling of performance test scores. The Clinical Neuropsychologist, 34(3), 437–453. https://doi.org/10.1080/13854046.2020.1722244
- Habib, A., Harris, L., Pollick, F., Melville, C., & Zhang, J. (2019). A meta-analysis of working memory in individuals with autism spectrum disorders. PloS One, 14(4), e0216198. https://doi.org/10.1371/journal.pone.0216198
- Hallquist, M. N., Wright, A. G. C., & Molenaar, P. C. M. (2021). Problems with centrality measures in psychopathology symptom networks: Why Network Psychometrics Cannot escape psychometric theory. Multivariate Behavioral Research, 56(2), 199–223. https://doi.org/10.1080/00273171.2019.1640103
- Happé, F., Briskman, J., & Frith, U. (2001). Exploring the cognitive phenotype of autism: Weak ‘central coherence’ in parents and siblings of children with autism: I. Experimental tests. Journal of Child Psychology and Psychiatry, 42(3), 299–307. https://doi.org/10.1111/1469-7610.00723
- Haukeland, Y. B., Czajkowski, N. O., Fjermestad, K. W., Silverman, W. K., Mossige, S., & Vatne, T. M. (2020). Evaluation of “SIBS”, an intervention for siblings and parents of children with chronic Disorders. Journal of Child & Family Studies, 29(8), 2201–2217. https://doi.org/10.1007/s10826-020-01737-x
- Heck, D. W., & Bockting, F. (2023). Benefits of Bayesian model averaging for mixed-effects modeling. Computational Brain & Behavior, 6(1), 35–49. https://doi.org/10.1007/s42113-021-00118-x
- Heck, D. W., Boehm, U., Böing-Messing, F., Bürkner, P.-C., Derks, K., Dienes, Z., Fu, Q., Gu, X., Karimova, D., Kiers, H. A. L., Klugkist, I., Kuiper, R. M., Lee, M. D., Leenders, R., Leplaa, H. J., Linde, M., Ly, A., Meijerink-Bosman, M., Moerbeek, M., and Hoijtink, H. (2022). A review of applications of the Bayes factor in psychological research. Psychological Methods. Advance online publication. https://doi.org/10.1037/met0000454
- Heitz, R., Redick, T., Hambrick, D., Kane, M., Conway, A., & Engle, R. (2006). Working memory, executive function, and general fluid intelligence are not the same. Behavioral and Brain Sciences, 29(2), 135–136. https://doi.org/10.1017/S0140525X06319036
- Hevey, D. (2018). Network analysis: A brief overview and tutorial. Health Psychology and Behavioral Medicine, 6(1), 301–328. https://doi.org/10.1080/21642850.2018.1521283
- Hewitt, K. C., Block, C., Bellone, J. A., Dawson, E. L., Garcia, P., Gerstenecker, A., Grabyan, J. M., Howard, C., Kamath, V., LeMonda, B. C., Margolis, S. A., McBride, W. F., Salinas, C. M., Tam, D. M., Walker, K. A., & Del Bene, V. A. (2022). Diverse experiences and approaches to tele neuropsychology: Commentary and reflections over the past year of COVID-19. The Clinical Neuropsychologist, 36(4), 790–805. https://doi.org/10.1080/13854046.2022.2027022
- Hinne, M., Gronau, Q. F., van den Bergh, D., & Wagenmakers, E.-J. (2020). A conceptual Introduction to Bayesian model averaging. Advances in Methods and Practices in Psychological Science, 3(2), 200–215. https://doi.org/10.1177/2515245919898657
- Hoogman, M., Bralten, J., Hibar, D. P., Mennes, M., Zwiers, M. P., Schweren, L. S. J., van Hulzen, K. J. E., Medland, S. E., Shumskaya, E., Jahanshad, N., Zeeuw, P., de Szekely, E., Sudre, G., Wolfers, T., Onnink, A. M. H., Dammers, J. T., Mostert, J. C., & Vives-Gilabert, Y. A, Franke, B. (2017). Subcortical brain volume differences in participants with attention deficit hyperactivity disorder in children and adults: A cross-sectional mega-analysis. The Lancet Psychiatry, 4(4), 310–319. https://doi.org/10.1016/S2215-0366(17)30049-4
- Howlett, C. A., Wewege, M. A., Berryman, C., Oldach, A., Jennings, E., Moore, E., Karran, E. L., Szeto, K., Pronk, L., Miles, S., & Moseley, G. L. (2022). Back to the drawing board—the relationship between self-report and neuropsychological tests of cognitive flexibility in clinical cohorts: A systematic review and meta-analysis. Neuropsychology, 36(5), 347–372. https://doi.org/10.1037/neu0000796
- Huber, N. L., Nicoletta, A., Ellis, J. M., & Everhart, D. E. (2020). Validating the adolescent sleep wake scale for use with young adults. Sleep Medicine, 69, 217–219. https://doi.org/10.1016/j.sleep.2020.01.021
- Hughes, C., Plumet, M. H., & Leboyer, M. (1999). Towards a cognitive phenotype for autism: Increased prevalence of executive dysfunction and superior spatial span amongst siblings of children with autism. Journal of Child Psychology and Psychiatry, and Allied Disciplines, 40(5), 705–718. https://doi.org/10.1111/1469-7610.00487
- Hurt, K., Walker, R. J., Campbell, J. A., & Egede, L. E. (2016). mHealth interventions in low and middle-income countries: A systematic Review. Global Journal of Health Science, 8(9), 54429. https://doi.org/10.5539/gjhs.v8n9p183
- Jaeggi, S. M., Buschkuehl, M., Perrig, W. J., & Meier, B. (2010). The concurrent validity of the N-back task as a working memory measure. Memory (Hove, England), 18(4), 394–412. https://doi.org/10.1080/09658211003702171
- Jalal, B., Brühl, A., O’Callaghan, C., Piercy, T., Cardinal, R. N., Ramachandran, V. S., & Sahakian, B. J. (2018). Novel smartphone interventions improve cognitive flexibility and obsessive-compulsive disorder symptoms in individuals with contamination fears. Scientific Reports, 8(1), 14923. https://doi.org/10.1038/s41598-018-33142-2
- Janicki, M. P., Hendrix, J. A., McCallion, P., & & and the Neuroatypical Conditions Expert Consultative Panel. (2022). Examining older adults with neuroatypical conditions for MCI/dementia: Barriers and recommendations of the neuroatypical conditions expert consultative panel. Alzheimer’s & Dementia, 14(1), e12335. https://doi.org/10.1002/dad2.12335
- Jokiranta-Olkoniemi, E., Cheslack-Postava, K., Joelsson, P., Suominen, A., Brown, A. S., & Sourander, A. (2019). Attention-deficit/hyperactivity disorder and risk for psychiatric and neurodevelopmental disorders in siblings. Psychological Medicine, 49(1), 84–91. https://doi.org/10.1017/S0033291718000521
- Jokiranta-Olkoniemi, E., Cheslack-Postava, K., Sucksdorff, D., Suominen, A., Gyllenberg, D., Chudal, R., Leivonen, S., Gissler, M., Brown, A. S., & Sourander, A. (2016). Risk of psychiatric and neurodevelopmental Disorders among siblings of probands with autism spectrum Disorders. Risk of Psychiatric and Neurodevelopmental Disorders Among Siblings of Probands with Autism Spectrum Disorders JAMA Psychiatry, 73(6), 622–629. https://doi.org/10.1001/jamapsychiatry.2016.0495
- Kellner, K. (2021). jagsUI: A Wrapper around ‘rjags’ to streamline ‘JAGS’ analyses. https://cran.r-project.org/package=jagsUI
- Kessels, R. P. C. (2019). Improving precision in neuropsychological assessment: Bridging the gap between classic paper-and-pencil tests and paradigms from cognitive neuroscience. The Clinical Neuropsychologist, 33(2), 357–368. https://doi.org/10.1080/13854046.2018.1518489
- Kessels, R. P., van den Berg, E., Ruis, C., & Brands, A. M. (2008). The backward span of the Corsi block-Tapping task and its association with the WAIS-III digit span. Assessment, 15(4), 426–434. https://doi.org/10.1177/1073191108315611
- Kievit, R. A. (2020). Sensitive periods in cognitive development: A mutualistic perspective. Current Opinion in Behavioral Sciences, 36, 144–149. https://doi.org/10.1016/j.cobeha.2020.10.007
- Kim, J., Gabriel, U., Gygax, P., & Frank, S. L. (2019). Testing the effectiveness of the Internet-based instrument PsyToolkit: A comparison between web-based (PsyToolkit) and lab-based (E-Prime 3.0) measurements of response choice and response time in a complex psycholinguistic task. PloS One, 14(9), e0221802. https://doi.org/10.1371/journal.pone.0221802
- Kirchner, W. K. (1958). Age differences in short-term retention of rapidly changing information. Journal of Experimental Psychology: General, 55(4), 352–358. https://doi.org/10.1037/h0043688
- Kofler, M. J., Irwin, L. N., Soto, E. F., Groves, N. B., Harmon, S. L., & Sarver, D. E. (2019). Executive functioning heterogeneity in pediatric ADHD. Journal of Abnormal Child Psychology, 47(2), 273–286. https://doi.org/10.1007/s10802-018-0438-2
- Kovshoff, H., Cebula, K., Tsai, H. J., & Hastings, R. P. (2017). Siblings of children with autism: The siblings embedded systems framework. Current Developmental Disorders Reports, 4(2), 37–45. https://doi.org/10.1007/s40474-017-0110-5
- Kroenke, K., Spitzer, R. L., & Williams, J. B. (2001). The PHQ-9: Validity of a brief depression severity measure. Journal of General Internal Medicine, 16(9), 606–613. https://doi.org/10.1046/j.1525-1497.2001.016009606.x
- Kruschke, J. K. (2018). Rejecting or accepting parameter values in Bayesian estimation. Advances in Methods and Practices in Psychological Science, 1(2), 270–280. https://doi.org/10.1177/2515245918771304
- Kruschke, J. K. (2021). Bayesian analysis reporting guidelines. Nature Human Behaviour, 5(10), 1282–1291. https://doi.org/10.1038/s41562-021-01177-7
- Kvigne, V. L., Leonardson, G. R., Borzelleca, J., Neff-Smith, M., & Welty, T. K. (2009). Characteristics of children whose siblings have fetal alcohol syndrome or incomplete fetal alcohol syndrome. Pediatrics, 123(3), e526–e533. https://doi.org/10.1542/peds.2008-2423
- Landry, O., Mitchell, P., & Marno, H. (2021). An examination of perseverative errors and cognitive flexibility in autism. PloS One, 16(1), e0223160. https://doi.org/10.1371/journal.pone.0223160
- Lavigne, K. M., Sauvé, G., Raucher-Chéné, D., Guimond, S., Lecomte, T., Bowie, C. R., Menon, M., Lal, S., Woodward, T. S., Bodnar, M. D., & Lepage, M. (2022). Remote cognitive assessment in severe mental illness: A scoping review. Schizophrenia, 8(1). Article 1. https://doi.org/10.1038/s41537-022-00219-x.
- Levens, S. M., Muhtadie, L., & Gotlib, I. H. (2009). Rumination and impaired resource allocation in depression. Journal of Abnormal Psychology, 118(4), 757–766. https://doi.org/10.1037/a0017206
- Liang, F., Paulo, R., Molina, G., Clyde, M. A., & Berger, J. O. (2008). Mixtures of g priors for Bayesian variable selection. Journal of the American Statistical Association, 103(481), 410–423. https://doi.org/10.1198/016214507000001337
- Lobato, D. J., & Kao, B. T. (2002). Integrated sibling-parent group intervention to improve sibling knowledge and adjustment to chronic illness and disability. Journal of Pediatric Psychology, 27(8), 711–716. https://doi.org/10.1093/jpepsy/27.8.711
- Malkani, M. K., Pestell, C. F., Sheridan, A. M. C., Crichton, A. J., Horsburgh, G. C., & Bucks, R. S. (2022). Behavioral sleep interventions for children with ADHD: A systematic Review and meta-analysis. Journal of Attention Disorders, 26(14), 1805–1821. https://doi.org/10.1177/10870547221106239
- Marquis, S., Hayes, M. V., & McGrail, K. (2019). Factors that may affect the health of siblings of children who have an Intellectual/Developmental disability. Journal of Policy and Practice in Intellectual Disabilities, 16(4), 273–286. https://doi.org/10.1111/jppi.12309
- Marquis, S., McGrail, K., & Hayes, M. V. (2020). Using administrative data to examine variables affecting the mental health of siblings of children who have a developmental disability. Research in Developmental Disabilities, 96, 103516. https://doi.org/10.1016/j.ridd.2019.103516
- Marsman, M., Huth, K., Waldorp, L., & Ntzoufras, I. (2022). Objective Bayesian edge screening and structure selection for networks of binary variables. Psychometrika, 87(1), 47–82. https://doi.org/10.1007/s11336-022-09848-8
- May, P. A., Chambers, C. D., Kalberg, W. O., Zellner, J., Feldman, H., Buckley, D., Kopald, D., Hasken, J. M., Xu, R., Honerkamp-Smith, G., Taras, H., Manning, M. A., Robinson, L. K., Adam, M. P., Abdul-Rahman, O., Vaux, K., Jewett, T., Elliott, A. J. … Hoyme, H. E. (2018). Prevalence of fetal alcohol spectrum Disorders in 4 US communities. JAMA, 319(5), 474–482. https://doi.org/10.1001/jama.2017.21896
- McArthur, G. E., Lee, E., & Laycock, R. (2022). Autism traits and cognitive performance: Mediating roles of sleep disturbance, anxiety and depression. Journal of Autism & Developmental Disorders. https://doi.org/10.1007/s10803-022-05742-5
- McCool, J., Dobson, R., Whittaker, R., & Paton, C. (2022). Mobile health (mHealth) in low- and middle-income countries. Annual Review of Public Health, 43(1), 525–539. https://doi.org/10.1146/annurev-publhealth-052620-093850
- McElroy, E., Belsky, J., Carragher, N., Fearon, P., & Patalay, P. (2018). Developmental stability of general and specific factors of psychopathology from early childhood to adolescence: Dynamic mutualism or p-differentiation? Journal of Child Psychology and Psychiatry, 59(6), 667–675. https://doi.org/10.1111/jcpp.12849
- McLean, R. L., Johnson Harrison, A., Zimak, E., Joseph, R. M., & Morrow, E. M. (2014). Executive function in Probands with autism with average IQ and their unaffected first-degree relatives. Journal of the American Academy of Child & Adolescent Psychiatry, 53(9), 1001–1009. https://doi.org/10.1016/j.jaac.2014.05.019
- Meule, A. (2017). Reporting and interpreting working memory performance in n-back tasks. Frontiers in Psychology, 8, 352.
- Mikels, J. A., & Reuter-Lorenz, P. A. (2019). Affective working memory: An integrative psychological construct. Perspectives on Psychological Science: A Journal of the Association for Psychological Science, 14(4), 543–559. https://doi.org/10.1177/1745691619837597
- Mohammadi, R., & Wit, E. C. (2019). Bdgraph: An R package for Bayesian structure learning in graphical models. Journal of Statistical Software, 89(3), 89. https://doi.org/10.18637/jss.v089.i03
- Mooney, K. E., Pickett, K. E., Shire, K., Allen, R. J., & Waterman, A. H. (2022). Socioeconomic disadvantage and ethnicity are associated with large differences in children’s working memory ability: Analysis of a prospective birth cohort study following 13,500 children. BMC Psychology, 10(1), 67. https://doi.org/10.1186/s40359-022-00773-0
- Mosconi, M. W., Kay, M., D'Cruz, A. M., Guter, S., Kapur, K., Macmillan, C., Stanford, L. D., & Sweeney, J. A. (2010). Neurobehavioral abnormalities in first-degree relatives of individuals with autism. Archives of General Psychiatry, 67(8), 830–840. https://doi.org/10.1001/archgenpsychiatry.2010.87
- Mukherjee, P., Hartanto, T., Iosif, A.-M., Dixon, J. F., Hinshaw, S. P., Pakyurek, M., van den Bos, W., Guyer, A. E., McClure, S. M., Schweitzer, J. B., & Fassbender, C. (2021). Neural basis of working memory in ADHD: Load versus complexity. NeuroImage: Clinical, 30, 102662. https://doi.org/10.1016/j.nicl.2021.102662
- Nilsson, J. P., Söderström, M., Karlsson, A. U., Lekander, M., Åkerstedt, T., Lindroth, N. E., & Axelsson, J. (2005). Less effective executive functioning after one night’s sleep deprivation. Journal of Sleep Research, 14(1), 1–6. https://doi.org/10.1111/j.1365-2869.2005.00442.x
- Nochaiwong, S., Ruengorn, C., Thavorn, K., Hutton, B., Awiphan, R., Phosuya, C., Ruanta, Y., Wongpakaran, N., & Wongpakaran, T. (2021). Global prevalence of mental health issues among the general population during the coronavirus disease-2019 pandemic: A systematic review and meta-analysis. Scientific Reports, 11(1). Article 1. https://doi.org/10.1038/s41598-021-89700-8.
- Oerlemans, A. M., Burmanje, M. J., Franke, B., Buitelaar, J. K., Hartman, C. A., & Rommelse, N. N. (2016). Identifying unique versus shared pre- and perinatal risk factors for ASD and ADHD using a simplex-multiplex stratification. Journal of Abnormal Child Psychology, 44(5), 923–935. https://doi.org/10.1007/s10802-015-0081-0
- Oerlemans, A. M., Droste, K., van Steijn, D. J., de Sonneville, L. M. J., Buitelaar, J. K., & Rommelse, N. N. J. (2013). Co-segregation of social cognition, executive function and local processing style in children with ASD, their siblings and normal controls. Journal of Autism and Developmental Disorders, 43(12), 2764–2778. https://doi.org/10.1007/s10803-013-1807-x
- Parsons, T. D., McMahan, T., & Kane, R. (2018). Practice parameters facilitating adoption of advanced technologies for enhancing neuropsychological assessment paradigms. The Clinical Neuropsychologist, 32(1), 16–41. https://doi.org/10.1080/13854046.2017.1337932
- Pe, M. L., Koval, P., Houben, M., Erbas, Y., Champagne, D., & Kuppens, P. (2015). Updating in working memory predicts greater emotion reactivity to and facilitated recovery from negative emotion-eliciting stimuli. Frontiers in Psychology, 6, 372. https://doi.org/10.3389/fpsyg.2015.00372
- Pelegrina, S., Lechuga, M. T., García-Madruga, J. A., Elosúa, M. R., Macizo, P., Carreiras, M., Fuentes, L. J., & Bajo, M. T. (2015). Normative data on the n-back task for children and young adolescents. Frontiers in Psychology, 6. https://www.frontiersin.org/articles/10.3389/fpsyg.2015.01544
- Petersen, S. E., & Posner, M. I. (2012). The attention system of the human brain: 20 years after. Annual Review of Neuroscience, 35, 73–89. https://doi.org/10.1146/annurev-neuro-062111-150525
- Pilowsky, T., Yirmiya, N., Gross-Tsur, V., & Shalev, R. S. (2007). Neuropsychological functioning of siblings of children with autism, siblings of children with Developmental language delay, and siblings of children with mental retardation of unknown genetic etiology. Journal of Autism and Developmental Disorders, 37(3), 537–552. https://doi.org/10.1007/s10803-006-0185-z
- Plummer, M. (2016). Rjags: Bayesian Graphical Models Using MCMC. R Package, Version 4–6. https://CRAN.R-project.org/package=rjags
- Poulton, A., Chen, L. P. E., Dali, G., Fox, M., & Hester, R. (2022). Web-Based Independent versus Laboratory-Based Stop-Signal Task Performance. Within-Subjects Counterbalanced Comparison Study J Med Internet Res, 24(5), e32922.
- Preece, D., Becerra, R., & Campitelli, G. (2019). Assessing emotional reactivity: Psychometric properties of the Perth emotional reactivity scale and the development of a short form. Journal of Personality Assessment, 101(6), 589–597. https://doi.org/10.1080/00223891.2018.1465430
- Raftery, A., Hoeting, J., Volinsky, C., Painter, I., & Yeung, K. Y. (2022). ‘BMA: Bayesian model averaging’. R package version 3.18.17. https://CRAN.R-project.org/package=BMA
- R Core Team. (2022). R: A language and environment for statistical computing. R Foundation for Statistical Computing. http://www.R-project.org/
- Reid, N., Crawford, A., Petrenko, C., Kable, J., & Olson, H. C. (2022). A family-directed approach for supporting individuals with fetal alcohol spectrum Disorders. Current Developmental Disorders Reports, 9(1), 9–18. https://doi.org/10.1007/s40474-021-00241-1
- Robertson, I. H., Manly, T., Andrade, J., Baddeley, B. T., & Yiend, J. (1997). ‘Oops!’: Performance correlates of everyday attentional failures in traumatic brain injured and normal subjects. Neuropsychologia, 35(6), 747–758. https://doi.org/10.1016/S0028-3932(97)00015-8
- Romer, A. L., & Pizzagalli, D. A. 2021. Is executive dysfunction a risk marker or consequence of psychopathology? A test of executive function as a prospective predictor and outcome of general psychopathology in the adolescent brain cognitive development study®. Developmental cognitive neuroscience, 51. Article 100994. https://doi.org/10.1016/j.dcn.2021.100994
- Rommelse, N. N. J., Altink, M. E., Oosterlaan, J., Buschgens, C. J. M., Buitelaar, J., & Sergeant, J. A. (2008). Support for an independent familial segregation of executive and intelligence endophenotypes in ADHD families. Psychological Medicine, 38(11), 1595–1606. https://doi.org/10.1017/S0033291708002869
- Rosa, M., Puig, O., Lázaro, L., Vallés, V., Lera, S., Sánchez-Gistau, V., & Calvo, R. (2017). Broad cognitive profile in children and adolescents with HF-ASD and in their siblings: Widespread underperformance and its Clinical and adaptive correlates. Journal of Autism and Developmental Disorders, 47(7), 2153–2162. https://doi.org/10.1007/s10803-017-3137-x
- Rouder, J. N., & Morey, R. D. (2012). Default Bayes factors for model selection in regression. Multivariate Behavioral Research, 47(6), 877–903. https://doi.org/10.1080/00273171.2012.734737
- Santomauro, D. F., Herrera, A. M. M., Shadid, J., Zheng, P., Ashbaugh, C., Pigott, D. M., Abbafati, C., Adolph, C., Amlag, J. O., Aravkin, A. Y., Bang-Jensen, B. L., Bertolacci, G. J., Bloom, S. S., Castellano, R., Castro, E., Chakrabarti, S., Chattopadhyay, J., Cogen, R. M., Collins, J. K., and Ferrari, A. J. (2021). Global prevalence and burden of depressive and anxiety disorders in 204 countries and territories in 2020 due to the COVID-19 pandemic. The Lancet, 398(10312), 1700–1712. https://doi.org/10.1016/S0140-6736(21)02143-7
- Schachar, R. J., Crosbie, J., Barr, C. L., Ornstein, T. J., Kennedy, J., Malone, M., Roberts, W., Ickowicz, A., Tannock, R., Chen, S., & Pathare, T. (2005). Inhibition of motor responses in siblings concordant and discordant for attention deficit hyperactivity disorder. American Journal of Psychiatry, 162(6), 1076–1082. https://doi.org/10.1176/appi.ajp.162.6.1076
- Schleider, J., & Weisz, J. (2018). A single-session growth mindset intervention for adolescent anxiety and depression: 9-month outcomes of a randomized trial. Journal of Child Psychology and Psychiatry, and Allied Disciplines, 59(2), 160–170. https://doi.org/10.1111/jcpp.12811
- Schmitter-Edgecombe, M., Sumida, C., & Cook, D. J. (2020). Bridging the gap between performance-based assessment and self-reported everyday functioning: An ecological momentary assessment approach. The Clinical Neuropsychologist, 34(4), 678–699. https://doi.org/10.1080/13854046.2020.1733097
- Schönbrodt, F. D., & Wagenmakers, E.-J. (2018). Bayes factor design analysis: Planning for compelling evidence. Psychonomic Bulletin & Review, 25(1), 128–142. https://doi.org/10.3758/s13423-017-1230-y
- Seidman, L. J., Biederman, J., Monuteaux, M. C., Weber, W., & Faraone, S. V. (2000). Neuropsychological functioning in nonreferred siblings of children with attention deficit/hyperactivity disorder. Journal of Abnormal Psychology, 109(2), 252–265. https://doi.org/10.1037/0021-843X.109.2.252
- Seng, G.-J., Tseng, W.-L., Chiu, Y.-N., Tsai, W.-C., Wu, Y.-Y., & Gau, S. S.-F. (2020). Executive functions in youths with autism spectrum disorder and their unaffected siblings. Psychological Medicine, 51(15), 2571–2580. https://doi.org/10.1017/S0033291720001075
- Sergeant, J. A. (2005). Modeling attention-deficit/hyperactivity disorder: A critical appraisal of the cognitive-energetic model. Biological Psychiatry, 57(11), 1248–1255. https://doi.org/10.1016/j.biopsych.2004.09.010
- Sharma, S. R., Gonda, X., & Tarazi, F. I. (2018). Autism spectrum disorder: Classification, diagnosis and therapy. Pharmacology & Therapeutics, 190, 91–104. https://doi.org/10.1016/j.pharmthera.2018.05.007
- Shivers, C. M., Jackson, J. B., & McGregor, C. M. (2019). Functioning among typically developing siblings of individuals with autism spectrum disorder: A meta-analysis. Clinical Child and Family Psychology Review, 22(2), 172–196. https://doi.org/10.1007/s10567-018-0269-2
- Slaats-Willemse, D., Swaab-Barneveld, H., De Sonneville, L., van der Meulen, E., & Buitelaar, J. (2003). Deficient response inhibition as a cognitive endophenotype of ADHD. Journal of the American Academy of Child & Adolescent Psychiatry, 42(10), 1242–1248. https://doi.org/10.1097/00004583-200310000-00016
- Slattery, E. J., O’Callaghan, E., Ryan, P., Fortune, D. G., & McAvinue, L. P. (2022). Popular interventions to enhance sustained attention in children and adolescents: A critical systematic review. Neuroscience & Biobehavioral Reviews, 137, 104633. https://doi.org/10.1016/j.neubiorev.2022.104633
- Snyder, H. R., Miyake, A., & Hankin, B. L. (2015). Advancing understanding of executive function impairments and psychopathology: Bridging the gap between clinical and cognitive approaches. Frontiers in Psychology, 6, 1–24. https://doi.org/10.3389/fpsyg.2015.00328
- Spitzer, R. L., Kroenke, K., Williams, J. B. W., & Löwe, B. (2006). Generalized Anxiety Disorder 7 (GAD-7) [Database record]
- Steinke, A., Kopp, B., & Lange, F. (2021). The Wisconsin card Sorting test: Split-half reliability estimates for a self-administered computerized variant. Brain Sciences, 11(5), 529. Article 5. https://doi.org/10.3390/brainsci11050529
- Stoet, G. (2017). PsyToolkit: A novel web-based method for running online questionnaires and reaction-time experiments. Teaching of Psychology, 44(1), 24–31. https://doi.org/10.1177/0098628316677643
- Suchy, Y. (2020). Introduction to special issue: Contextually valid assessment of executive functions in the era of personalized medicine. The Clinical Neuropsychologist, 34(4), 613–618. https://doi.org/10.1080/13854046.2020.1748827
- Suchy, Y., Ziemnik, R. E., Niermeyer, M. A., & Brothers, S. L. (2020). Executive functioning interacts with complexity of daily life in predicting daily medication management among older adults. The Clinical Neuropsychologist, 34(4), 797–825. https://doi.org/10.1080/13854046.2019.1694702
- Sumiyoshi, C., Kawakubo, Y., Suga, M., Sumiyoshi, T., & Kasai, K. (2011). Impaired ability to organize information in individuals with autism spectrum disorders and their siblings. Neuroscience Research, 69(3), 252–257. https://doi.org/10.1016/j.neures.2010.11.007
- Sunderland, M., Forbes, M., Mewton, L., Baillie, A., Carragher, N., Lynch, S., and Slade, T. (2021). The structure of psychopathology and association with poor sleep, self-harm, suicidality, risky sexual behavior, and low self-esteem in a population sample of adolescents. Development and Psychopathology, 33(4), 1208–1219. https://doi.org/10.1017/S0954579420000437
- Szatmari, P., Jones, M. B., Tuff, L., Bartolucci, G., Fisman, S., & Mahoney, W. (1993). Lack of cognitive impairment in first-degree relatives of children with pervasive developmental disorders. Journal of the American Academy of Child and Adolescent Psychiatry, 32(6), 1264–1273. https://doi.org/10.1097/00004583-199311000-00022
- Taboas, A., Doepke, K., & Zimmerman, C. (2023). Preferences for identity-first versus person-first language in a US sample of autism stakeholders. Autism: The International Journal of Research and Practice, 27(2), 565–570. https://doi.org/10.1177/13623613221130845
- Tarle, S. J., Alderson, R. M., Patros, C. H., Arrington, E. F., & Roberts, D. K. (2019). Working memory and behavioral inhibition in children with attention-deficit/hyperactivity disorder (ADHD): An examination of varied central executive demands, construct overlap, and task impurity. Child Neuropsychology, 25(5), 664–687. https://doi.org/10.1080/09297049.2018.1519068
- Taylor, M. J., Larsson, H., Lundström, S., Lichtenstein, P., & Butwicka, A. (2022). Etiological links between autism and difficulties in initiating and maintaining sleep: A familial co-aggregation and twin study. Journal of Child Psychology and Psychiatry, 63(3), 315–323. https://doi.org/10.1111/jcpp.13473
- Treviño, M., Zhu, X., Lu, Y. Y., Scheuer, L. S., Passell, E., Huang, G. C., Germine, L. T., & Horowitz, T. S. (2021). How do we measure attention? Using factor analysis to establish construct validity of neuropsychological tests. Cognitive Research: Principles and Implications, 6(1), 51. https://doi.org/10.1186/s41235-021-00313-1
- van den Bergh, D., van Doorn, J., Marsman, M., Draws, T., van Kesteren, E.-J., Derks, K., Dablander, F., Gronau, Q. F., Kucharský, Š., Gupta, A. R. K. N., Sarafoglou, A., Voelkel, J. G., Stefan, A., Ly, A., Hinne, M., Matzke, D., & Wagenmakers, E.-J. (2020). A tutorial on conducting and interpreting a Bayesian ANOVA in JASP. L’Année Psychologique, 120(1), 73–96. https://doi.org/10.3917/anpsy1.201.0073
- van Ewijk, H., Heslenfeld, D. J., Luman, M., Rommelse, N. N., Hartman, C. A., Hoekstra, P., Franke, B., Buitelaar, J. K., & Oosterlaan, J. (2014). Visuospatial working memory in ADHD patients, unaffected siblings, and healthy controls. Journal of Attention Disorders, 18(4), 369–378. https://doi.org/10.1177/1087054713482582
- Van Eylen, L., Boets, B., Cosemans, N., Peeters, H., Steyaert, J., Wagemans, J., & Noens, I. (2017). Executive functioning and local-global visual processing: Candidate endophenotypes for autism spectrum disorder? Journal of Child Psychology and Psychiatry, 58(3), 258–269. https://doi.org/10.1111/jcpp.12637
- van Lieshout, M., Luman, M., Schweren, L., Twisk, J., Faraone, S. V., Heslenfeld, D. J., Hartman, C. A., Hoekstra, P. J., Franke, B., Buitelaar, J. K., Rommelse, N., & Oosterlaan, J. (2019). The course of neurocognitive functioning and prediction of behavioral outcome of ADHD affected and unaffected siblings. Journal of Abnormal Child Psychology, 47(3), 405–419. https://doi.org/10.1007/s10802-018-0449-z
- van Lieshout, M., Luman, M., Twisk, J. W., Faraone, S. V., Heslenfeld, D. J., Hartman, C. A., Hoekstra, P. J., Franke, B., Buitelaar, J. K., Rommelse, N. N., & Oosterlaan, J. (2017). Neurocognitive predictors of ADHD outcome: A 6-year follow-up study. Journal of Abnormal Child Psychology, 45(2), 261–272. https://doi.org/10.1007/s10802-016-0175-3
- Van Patten, R. (2021). Introduction to the special issue - Neuropsychology from a distance: Psychometric properties and clinical utility of remote neurocognitive tests. Journal of Clinical and Experimental Neuropsychology, 43(8), 767–773. https://doi.org/10.1080/13803395.2021.2021645
- Veloso, G. C., & Ty, W. E. G. (2021). The effects of emotional working memory training on trait anxiety. Frontiers in Psychology, 11, 549623. https://doi.org/10.3389/fpsyg.2020.549623
- Verbruggen, F., Aron, A. R., Band, G. P., Beste, C., Bissett, P. G., Brockett, A. T., Brown, J. W., Chamberlain, S. R., Chambers, C. D., Colonius, H., Colzato, L. S., Corneil, B. D., Coxon, J. P., Dupuis, A., Eagle, D. M., Garavan, H., Greenhouse, I., Heathcote, A., Huster, R. J. … Boehler, C. N. (2019). A consensus guide to capturing the ability to inhibit actions and impulsive behaviors in the stop-signal task. ELife, 8, e46323. https://doi.org/10.7554/eLife.46323
- Verbruggen, F., Chambers, C. D., & Logan, G. D. (2013). Fictitious inhibitory differences: How skewness and slowing distort the estimation of stopping latencies. Psychological Science, 24(3), 352–362. https://doi.org/10.1177/0956797612457390
- Verbruggen, F., & Logan, G. D. (2008). Response inhibition in the stop-signal paradigm. Trends in Cognitive Sciences, 12(11), 418–424. https://doi.org/10.1016/j.tics.2008.07.005
- Wade, M., Prime, H., & Madigan, S. (2015). Using sibling designs to understand neurodevelopmental Disorders: From genes and environments to prevention programming. BioMed Research International, 2015, 1–16. https://doi.org/10.1155/2015/672784
- Wade, M., Zeanah, C., Fox, N., & Nelson, C. (2020). Global deficits in executive functioning are transdiagnostic mediators between severe childhood neglect and psychopathology in adolescence. Psychological Medicine, 50(10), 1687–1694. https://doi.org/10.1017/S0033291719001764
- Wagenmakers, E., Morey, R. D., & Lee, M. D. (2016). Bayesian benefits for the pragmatic researcher. Current Directions in Psychological Science, 25(3), 169–176. https://doi.org/10.1177/0963721416643289
- Warren, Z. E., Foss-Feig, J. H., Malesa, E. E., Lee, E. B., Tayor, J. L., Newsom, C. R., Crittendon, J., & Stone, W. L. (2012). Neurocognitive and behavioral outcomes of younger siblings of children with autism spectrum disorder at age five. Journal of Autism and Developmental Disorders, 42(3), 409–418. https://doi.org/10.1007/s10803-011-1263-4
- Weigard, A., Heathcote, A., Matzke, D., & Huang-Pollock, C. (2019). Cognitive modeling suggests that attentional failures drive longer stop-signal reaction time estimates in attention deficit/hyperactivity disorder. Clinical Psychological Science, 7(4), 856–872. https://doi.org/10.1177/2167702619838466
- Wolff, B., Franco, V. R., Magiati, I., Cooper, M. N., Roberts, R., Skoss, R., & Glasson, E. J. (2023). Individual-Level Risk and Resilience Factors Associated with Mental Health in Siblings of Individuals with Neurodevelopmental Conditions: A Network Analysis. Developmental Neuropsychology. 1–23. https://doi.org/10.1080/87565641.2023.2190119
- Wolff, B., Magiati, I., Roberts, R., Pellicano, E., & Glasson, E. J. (2022). Risk and resilience factors impacting the mental health and wellbeing of siblings of individuals with neurodevelopmental conditions: A mixed methods systematic review. Clinical Psychology Review, 98, 102217. https://doi.org/10.1016/j.cpr.2022.102217
- Wolff, B., Magiati, I., Roberts, R., Skoss, R., & Glasson, E. J. (2022). Psychosocial interventions and support groups for siblings of individuals with neurodevelopmental conditions: A mixed Methods systematic Review of sibling self-reported mental health and wellbeing outcomes. Clinical Child and Family Psychology Review, 98, 102217. https://doi.org/10.1007/s10567-022-00413-4
- Wolff, B., Sciberras, E., He, J., Youssef, G., Anderson, V., & Silk, T. J. (2021). The role of sleep in the Relationship between ADHD symptoms and stop signal task performance. Journal of Attention Disorders, 25(13), 1881–1894. https://doi.org/10.1177/1087054720943290
- Wong, D., Maybery, M., Bishop, D. V. M., Maley, A., & Hallmayer, J. (2006). Profiles of executive function in parents and siblings of individuals with autism spectrum disorders. Genes, Brain and Behavior, 5(8), 561–576. https://doi.org/10.1111/j.1601-183X.2005.00199.x
- Yang, Y., Shields, G. S., Zhang, Y., Wu, H., Chen, H., & Romer, A. L. (2022). Child executive function and future externalizing and internalizing problems: A meta-analysis of prospective longitudinal studies. Clinical Psychology Review, 97, 102194. Advance online publication. https://doi.org/10.1016/j.cpr.2022.102194
- Zelazo, P. D. (2020). Executive function and psychopathology: A neurodevelopmental perspective. Annual Review of Clinical Psychology, 16(1), 431–454. https://doi.org/10.1146/annurev-clinpsy-072319-024242