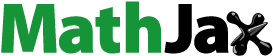
ABSTRACT
A paradigmatic experience of agency is the felt effort associated with the act of making a difficult choice. The challenge of accounting for this experience within a compatibilist framework has been called ‘the agency problem of compatibilism’ (Vierkant, 2022, The Tinkering Mind: Agency, Cognition and the Extended Mind, Oxford University Press, 116). In this paper, I will propose an evolutionarily plausible, actional account of deciding which explains the phenomenology. In summary: The act of making a difficult choice is triggered by a metacognitive decision to intentionally stop deliberating, despite ongoing uncertainty. This decision is the output of a metacognitive cost–benefit computation, which weighs the value of uncertainty reduction against the costs of ongoing deliberation. Strikingly, contemporary theories of effort suggest that this cost–benefit computation is also the source of the feeling of mental effort, which tracks the costs of that decision. If this account is correct, the agency problem of compatibilism has been solved. The act of making a difficult choice and the associated paradigmatic experience of agency, felt effort, both follow from the metacognitive evaluation. Implications are explored.
1. Introduction
1.1. Difficult choices
Some choices are easy,Footnote1 but others are hard. They may be hard to make, because they involve uncertainty about what to do, or hard to stick with, because of an ongoing desire to do otherwise. Phenomenologically, both kinds of hard choice involve the feeling of mental effort, which Massin (Citation2017, 235) categorises as ‘cognitive effort’ and ‘effort of will’ respectively.Footnote2
Although there is plausibly a correlation between choices which require cognitive effort and those that require effort of will, the two should be considered separately as they sit on opposite sides of the moment of intention formation. Cognitive effort is felt in the process of forming intentions, whilst effort of will is felt by an agent trying to act in accordance with a formed intention. In this paper I limit my analysis to the experience of cognitive effort that accompanies the making of difficult choices. I set aside for another day an analysis of effort of will and the associated acts of self-control.
Difficult choices are hard but making them is central to the experience of being an agent. In debates regarding free will, both compatibilists and libertarians agree that a paradigmatic experience of freedom is the felt effort associated with making a difficult choice. As William James describes, ‘those soul-trying moments’ are ‘what give the palpitating reality to our moral life and make it tingle’ (Citation2014, 183).
Despite this agreement, there is dispute over the process by which a difficult choice is made, whether making a difficult choice is an action, and why it feels effortful. My aim in this paper is to provide an evolutionarily plausible, actional account of deciding which explains the phenomenology.
In section 2 I begin by reviewing two arguments for a standard non-actional model of decision making. I then argue that the phenomenology of effort indicates that something is missing from this standard account. What is missing is a consideration of unresolved uncertainty. Making a difficult choice involves resolving indecision without resolving uncertainty. From this foundation I outline a positive proposal, which explains why making a difficult choice is an act, and why it feels effortful.
The act of making a difficult choice is triggered by a decision to intentionally stop deliberation, despite ongoing uncertainty. This decision is the output of a metacognitive cost–benefit computation, which evaluates whether to stop or prolong the cognitive process.
More formally, when making a decision about what to do, an agent performs two evaluations:
Evaluation E (cognitive): An evaluation of whether to perform action A or ¬A.
Evaluation M (metacognitive): An evaluation of whether to stop or prolong E-ing.
Metacognition is the series of processes that monitor, evaluate and control cognitive activity. Evaluation M informs the metacognitive act of stopping (or prolonging) cognition, which is the metacognitive version of the evolutionarily antecedent ability to stop (or prolong) physical activity.
I will suggest that evaluation M compares the value of uncertainty reduction with the opportunity costs of prolonging deliberation. Whatever its precise form, evaluation M has the functional impact of stopping or prolonging the evaluative process E.
Importantly, and perhaps controversially, evaluation M does not include any inputs that are reasons or evidence for action A or its alternatives. The pros and cons of A-ing are irrelevant to evaluation M, other than indirectly via uncertainty. In slogan form one might say that evaluation M compares decision uncertainty with decision urgency, and influences decision timing. In situations of urgency, such as fight-or-flight, or low stake decisions, evaluation M will stop evaluation E very quickly. In scenarios where time is no constraint but making the correct choice is of high importance, evaluation E will be prolonged, possibly for many days.
In section 3 I show that the insights from models of physical stopping behaviour in foraging, as studied within the field of ethology, are directly applicable to the metacognitive stopping behaviour necessary for the making of a difficult choice. I then review evidence that metacognitive monitoring in primates leads to physical stopping behaviour; and finally turn to human metacognitive control of stopping and prolonging study time. As well as supporting my proposed revised model of decision making, this explains its development through a process of what Cisek calls ‘phylogenetic refinement’ (Citation2019, 2265).
In section 4, I review contemporary theories of the feeling of effort. Consistent with these theories is the claim that evaluation M is not only the source of the binary output of whether to stop or prolong cognition, but is also the source of the analogue feeling of effort that tracks the costs of that decision. Making a difficult choice feels effortful because the mind-directed intentional act of stopping the cognitive process has uncertainty-related costs, and those costs are revealed to the agent through affect.
Finally, in section 5, I explore the implications of the proposed account for agency.
The act of making a difficult choice feels effortful. By analysing evaluation M, one can finally explain and understand this paradigmatic experience of agency.
1.2. Clarifications and assumptions
Cognitive effort includes both the temporally extended effort of ongoing deliberation, and the more momentary effort felt when stopping deliberation and making a difficult choice. As Kriegel states, the process of deliberation ‘has a tangible duration to it but the act of deciding, of making up one’s mind, is … instantaneous’ (Citation2015, 85). The account I develop focuses narrowly on what Mele calls ‘momentary mental actions of intention formation’ (Citation2017, 85), when an agent stops deliberating and decides what to do. This is the moment to which I refer when I use the phrase making a (difficult) choice. However, as will be seen, there is a pleasing symmetry between explanations of effortful prolonging and effortful stopping.
I will assume that deliberation captures the full decision-making process, within which are evaluations, mental acts of stopping or prolonging cognition and any further acts of what Hieronymi calls ‘managerial control’ (Citation2009, 138).
Evaluations may be fast and unconscious or may explicitly follow learned propositional rules. Either way, I will assume that an evaluation is involuntary in the sense that the output is a natural function of the inputs and the agent’s cognitive computational process. Despite this, evaluations by different individuals may lead to different outputs, reflecting their differing experiences and values. Some may seem sub-optimal to an external observer.
Finally, I use choice and decision interchangeably and any mention of uncertainty should be understood as shorthand for uncertainty about what to do. I will assume that necessarily, any agent with an unsettled question of what to do, is uncertain about what to do. This implies that it is possible for an agent to be uncertain about what to do even if she knows what she should do.
2. Making a difficult choice
2.1. Uncertainty and decisions
In the philosophical literature, there has been significant debate over whether deciding is, or can sometimes be, an intentional act. Proponents of a non-actional view of deciding argue that decisions are passive events of intention acquisition, and are the automatic culmination of a process of evaluation:
The movement of the natural causality of reason … to its conclusion in choice or decision is lived (by some) as action when it is really just reflex; distinctively rational reflex, to be sure, but not in any case a matter of action. (Strawson Citation2003, 244)
In order to approach the question of actional vs non-actional decisions, I first analyse the interaction between decisions and uncertainty.
The question of what to do arises when an agent is faced with alternative courses of action and there is some uncertainty about what to do. She must decide between the alternatives. If there is no alternative or no uncertainty then no decision is required.
Uncertainty implies a risk of error. To reduce the potential harm associated with error, a decision-making process is engaged. In an ideal world this process would incorporate all relevant evidence and activate all of the agent’s relevant beliefs and desires, enabling her to (consciously or unconsciously) evaluate the pros and cons of an action A vs its alternatives and eliminate the uncertainty. Consistent with this, O’Shaughnessy describes decidings as ‘those comings-to-intend events that resolve a state of uncertainty over what to do’ (Citation1980, 297). In the real world, however, resource constraints, both temporal and cognitive, mean that only a subset of the relevant factors can be considered. Thus, the output of any evaluation will include an error term.Footnote3 Given this, resolving uncertainty should be understood to indicate that uncertainty is brought below some acceptable threshold,Footnote4 not that it is eliminated altogether. I will call this the standard model of decision making as illustrated in .
To summarise the link between uncertainty and the non-actional account: Deliberation begins when uncertainty about what to do arises and ends when that uncertainty is resolved. This is a classic negative feedback system: ‘The action [deliberation] is performed to eliminate the conditions that motivated the action’ (Cisek Citation2019, 2269). Decisions flow naturally and passively once uncertainty is resolved.
The argument that decisions are non-actional is strengthened by the link between actions and intentions: If a decision is an act, then, as Davidson has argued, it must be intentional under some description (Citation1980). Equally, as per Bratman (Citation1999), the content of any intention is an action-plan. The difficult question for proponents of actional decisions is what specific action-plan is contained within the intention to decide? The obvious proposal in the case where an agent decides to A, that the content of the prior intention is the plan to decide to A, seems problematic. An intention to decide to A is at minimum inefficient and, as Kavka’s (Citation1983) famous toxin puzzle illustrates, in normal situations is just an intention to A. Perhaps the reader can imagine some complicated scenarios where an agent is obstructed from forming an intention to A directly, but I will follow Mele in assuming that ‘in normal cases, there is no purpose in non-actionallyFootnote5 acquiring an intention to decide to A that is not more efficiently served by a non-actional acquisition of an intention to A’ (Citation2017, 92).
This implies that for actional decision to exist in normal situations, the content of the prior intention must be something other than < decide to A > . Let us call it the intention to < X > . If making a difficult choice is an act, then it is the act of X-ing. Therefore, in order to answer the question of what triggers the act of making a difficult choice, and to explain its phenomenology, I must first elucidate what X is. It will then be possible to determine the process through which the intention to X is formed, and why X-ing is effortful. I will return to these questions in section 2.3, after a discussion of the importance of phenomenology.
2.2. The phenomenology of effortful choices
Despite the concerns outlined in section 2.1, many philosophers (Holton Citation2006; Mele Citation2017; Pink Citation1996; Shepherd Citation2015) believe that the making of a difficult choice is an intentional mental act. This position is consistent with folk intuitions, which are themselves grounded in phenomenology. Difficult choices are effortful and, as Bayne and Levy state, ‘the experience of effort involves an experience of the self as a source of force’ (Citation2006, 63). The cognitive effort that accompanies the making of a difficult choice makes the agent feel as though the choice is an intentional act. If making a difficult choice is effortful, and effort is a marker of intentional action, then making a difficult choice must be an action.
Holton goes further, arguing that ‘choice is the primary ingredient in the experience of free will’ (Citation2006, 2). This raises a challenge for Holton as he is a compatibilist, engaging with the broader literature regarding the gap between the standard mechanistic or computational accounts of how agency works, and everyday experience of how agency feels. This phenomenological gap explains the intuition that there is something missing from the standard account. As Velleman (Citation1992) explains, a passive weighing of beliefs and desires doesn’t capture what it feels like to be an agent. Difficult choices feel like actions taken by agents, so compatibilists need to be able to place the agent within their model rather than replacing her with a ‘passive vessel’ (Holton Citation2006, 5).
Vierkant has called the challenge of accounting for the experience of effortful choice within a compatibilist framework ‘the agency problem of compatibilism’ (Citation2022, 116). The worry is that if the compatibilist account fails to solve this problem, then that will drive some to libertarianism.
Holton’s solution is to state that difficult choices are made ‘in the absence of judgement’ (Citation2006, 7). Whereas in the non-actional account decisions are assumed to flow naturally from judgements, in situations of indifference, incommensurability or insufficient evidence, uncertainty remains. No decision is able to flow naturally because the agent is as yet unable to form a judgement in which she has a desired level of confidence.Footnote6 Importantly for Holton, a choice in the absence of judgement is not explained by the standard model. Released from these tight constraints, it can be an action.
Having suggested a solution in which a decision can be an action, Holton’s main concern is to deflate worries that decision making in the absence of judgement is just random picking.Footnote7 He relies on a wealth of empirical data, to argue that when making a choice in the absence of consciously available reasons, the individual relies on her subconscious, which can be quite skilful.
Although relevant for the question of why the agent chose what she did, Holton’s analysis of the subconscious doesn’t seem to explain the phenomenology of making an effortful choice. Specifically, why would the shift to a mechanism where choices are influenced by unconscious states make a decision more effortful? The choice would be harder to justify, and may feel more confusing, but, if anything, feelings of effort arising from unconscious processes might be expected to be less forceful than their conscious equivalents. Vierkant worries that Holton’s solution might imply choices ‘have no experiential echo at all’ (Citation2018, 4). At the very least, further work is needed to elucidate this issue.
The central questions highlighted in 2.1 remain. With regards to the intention to X, what it is its precise content and how is it formed? And when the intention to X is executed, why is there a feeling of effort?
Having said that, progress has been made and the importance of uncertainty is becoming apparent. If there is no uncertainty there is no decision to be made. If a decision is made after uncertainty is resolved through a process of deliberation, that is a standard decision. However, if a decision is made whilst uncertainty remains, that is what I have called a difficult choice. Difficult choices are not explained by the standard model, so making a difficult choice can be an action.
In the next section, I outline a positive proposal for what motivates difficult decision making and why it feels effortful. Although I agree with Holton’s insight that the standard model does not account for difficult choices, I suggest that the outstanding questions of section 2.1 can be answered through analysis of unresolved uncertainty, rather than of the subconscious expertise on which Holton focuses. I develop this proposal further, and support it with empirical data, in section 3, where I suggest a revised model of decision making, and section 4, where I incorporate contemporary theories of mental effort.
2.3. Ongoing uncertainty and making difficult choices
In the account set out thus far, uncertainty has played two roles. First, resolved vs unresolved uncertainty is the differentiating factor between standard decisions and difficult choices. Second, in section 2.1 uncertainty was described as an indication of the risk of error and a reason to engage in deliberation. In what follows, I expand on this latter point.
I take it as given that a cognitive infrastructure exists that encodes an agent’s disparate valuesFootnote8 (Levy and Glimcher Citation2012; Padoa-Schioppa Citation2011; Smith et al. Citation2010; Westbrook and Braver Citation2016). These may be innate or learned, intrinsic or extrinsic, subconscious or explicitly endorsed.
A valued end is an end consistent with those values.
An agent’s valued ends can all be considered ends to which she is implicitly or explicitly committed. To be committed implies being motivated, in appropriate circumstances, to act in ways that achieve states of affairs consistent with those ends.
As Cisek says:
At its heart [a behavioural control system] is an evaluation of the animal’s current state in relation to a range of desirable states. Deviations of the …. state outside the desirable range constitute the motivation for actions that improve the state.
The result is a negative feedback system. (Citation2019, 2268–2269)
However, there is also value in appropriately allocating finite cognitive and temporal resources. ‘Sometimes continued deliberation is costly’ (Shepherd Citation2015, 346). Spending too long focusing-on and deliberating-over a single issue has opportunity costs. These relate to the specifics of the situation as well as the general fact that focused attention lowers the ability for quick and flexible reconfiguration to a new task when the environment changes (Kurzban et al. Citation2013; Musslick and Cohen Citation2021). The greater the opportunity costs the greater the urgency to curtail deliberation. ‘It would thus be useful if an agent possessed the ability to terminate deliberation ‘at will’’ (Shepherd Citation2015, 346).
The conflict between decision uncertainty and decision urgency creates an explore-exploit tension (Hills et al. Citation2015) or speed-accuracy trade-off (Bogacz et al. Citation2010) that an agent needs to manage. The agent evaluates these costs and benefits to determine whether to stop or prolong deliberation. This metacognitive evaluation, evaluation M, can culminate in the decision to stop deliberation and make a choice, even if uncertainty remains.
Importantly, the costs and benefits of evaluation M do not include any reasons or evidence for A-ing or ¬A-ing. As Kane states, choices ‘are made intentionally or on purpose not by virtue of a specific prior intention to make the particular choice made but by virtue of the general intention to resolve indecision in one way or another’ (Citation1999, 139). Making a difficult choice resolves indecision without resolving uncertainty.
Note that although the explanandum of this paper is the process by which difficult choices are successfully made, it is possible that the decision-making process ends with a decision between two acts, A and B, left unmade. This can be separated into two scenarios. In the first scenario, the agent actively and effortfully stops the process for good and gives up on making a decision between A and B. This is captured above by the specification that ¬A includes doing nothing. In the second scenario the agent is unable to reach a decision between A and B for now, but (consciously or unconsciously) intends to return to the issue later, in the hope that more evidence or inspiration will lead to a satisfactory resolution at a later time. This might be described as interrupted prolonging and could possibly be effortless if, for example, the need to resolve indecision is not pressing.
Having clarified this nuance, I will follow Kane in suggesting that the searched for X, the content of the intention which is required for a difficult choice to be an action, is resolve indecision now, despite ongoing uncertainty.
Henceforth I will use a shortened version, with the subordinate clause assumed but unstated:
Resolving indecision can be understood as increasing the tolerance for uncertainty.Footnote9 By doing so one can declare a victor in a contest that would otherwise be too close to call. I illustrate with a rather idiosyncratic example, but the idea will be familiar to anyone with an understanding of statistical significance:
In the biennial cross-channel varsity swimming relay between Oxford and Cambridge the rules state that due to problems with accurate timing and the definition of the finish, ‘any difference in times under two minutes is to be declared a dead heat’.Footnote10 In 2014, such a dead heat occurred. If uncertainty had not been taken into consideration, the result would have changed from dead heat to a Cambridge win, despite no change in the measured performance of the athletes ().
This discussion implies that when an agent effortfully makes a difficult choice, she is not asymmetrically biasing the decision towards one outcome or another, nor is she reducing uncertainty. She is merely stopping the deliberative process, and forcing herself to decide now despite ongoing uncertainty. If the agent ends up deciding to A, it is not because she agentially shifts the decision in the A direction, rather it is because she shifts the acceptable level of uncertainty higher. This in turn changes the result from indecision, to decision.
The directionless agential power to resolve indecision may feel a little underwhelming to some readers, but it does have the benefit that it mitigates any Kavkanian concerns. In the proposed model the prior intention is not an intention to decide to A, but merely an intention to resolve indecision. The latter is trivially unproblematic.
I will return to discussions of agential power in section 5 but will now sketch a preliminary answer to the question of why resolving indecision is effortful. Section 4 will provide a more detailed argument based on contemporary theories of cognitive effort. If I can provide a satisfactory answer, then the phenomenological gap with respect to the act of making a difficult choice is closed.
Making a difficult choice involves the mind-directed intentional act of stopping the deliberative process despite ongoing uncertainty. The agent forms an intention to resolve indecision, not because she no longer values reducing uncertainty, but because the benefit of uncertainty reduction is outweighed by the cost of continued deliberation. By valuing certainty, she is motivated to prolong deliberation, but this is outweighed by the motivation to stop. Therefore, whilst executing her intention to resolve indecision, the agent must inhibit her ongoing motivation to prolong deliberation. What makes difficult choices hard ‘are the attempts to inhibit’ (Vierkant Citation2022, 144) the desire to consider the question further.Footnote11
As anyone who has felt temptation knows, inhibiting or resisting an ongoing motive is effortful. This applies to resisting a ‘lust of the mind’, to use Hobbes’ (Citation1997, 44) evocative description of curiosity, just as it does to resisting physical desires.
But there is a possible concern here. In section 1.1 I stated that cognitive effort and effort of will should be considered separately as they sit on opposite sides of the moment of intention formation. Am I now undermining this distinction? The answer is that cognitive effort and effort of will sit either side of the intention to A, but there is now an additional and earlier intention to X. Cognitive effort is prior to the lower-level intention to A but subsequent to the higher-level intention to resolve indecision. With respect to the intention to A the effort of making a difficult decision is cognitive effort, but with respect to the prior, metacognitive, intention to X, it looks (and feels) like effort of will.
Similarly, as the quote from Vierkant indicates, merely deciding to stop deliberation is not enough. For any kind of stopping, once an agent has decided to stop, that is not the end of the matter. She must then try to stop, and trying to do something can be hard. An intention, which is a commitment to a plan of action, is a decision joined to a trying, and ‘trying always mobilizes effort’ (Kriegel Citation2015, 90). In the case of making a difficult choice, effort must be mobilised to inhibit the ongoing motivation to reduce uncertainty.Footnote12
As part of his wider argument for conative phenomenology as an irreducible phenomenological primitive, Kriegel concludes that ‘conative phenomenology is in the first instance a phenomenology of deciding-cum-trying’ (Citation2015, 94). There is much of interest in Kriegel’s detailed analysis but the relevant point here is that the intention to resolve indecision involves deciding-cum-trying, with its associated phenomenology, just as does the more run of the mill intention to A.
This framework also helps to set aside, for the purposes of this paper, a concern about whether it is always possible to resolve indecision: Some readers might believe that in theory there exists a scenario in which there are exactly equally strong reasons for A and ¬A, such that effortfully increasing the tolerance for uncertainty does not resolve the question of what to do. Others might believe that in practice, if the agent is willing and able to tolerate maximum uncertainty, then indecision will always be resolved. This may due to subconscious reasons, internal randomness due to noise in the process, or external randomness, as in the case of a coin flip. However, I can remain neutral with regards this question because it is well understood that effortfully trying does not guarantee success. The important question for this paper is not whether deciding-cum-trying to resolve indecision is always successful, but what is it that motivates this kind of deciding-cum-trying, and why is it effortfulFootnote13?
The arguments of this section suggest that from both a computational and phenomenological perspective, the metacognitive decision to resolve indecision, shares many similarities with an ordinary decision. Both follow from an evaluation of relevant pros and cons, and can be effortful if the cons exert an ongoing motivational pull which must be inhibited. This suggests that making a difficult choice is an action, but also that one might be able to gain insights into metacognitive stopping from studying other stopping behaviour in nature.
In the following section I aim to show that the insights from models of physical stopping behaviour in foraging, as studied within the field of ethology, are directly applicable to the metacognitive stopping behaviour necessary for the making of a difficult choice. As well as supporting my central claim that evaluation M culminates in the act of making a difficult choice, this will also explain its phylogenetic development.Footnote14 As a result of this analysis, I will be able to propose a revised model of decision making.
3. A revised model of decision making
Ethologist, Tinbergen (Citation1963) argued that in studying behaviour, one must address questions in four different areas: mechanism, adaptive significance, phylogenetic development and ontogenetic development. Taken together, section 3 will allow us to approach understanding of those four areas as they relate to a specific kind of human behaviour, the making of a difficult choice.
3.1. Stopping and prolonging the physical activity of foraging
Animals forage to satisfy their energy needs. When their current nutrient state deviates from a desired state, foraging action is initiated. A simplified negative feedback model would suggest that, in the absence of any constraints, animals should continue to forage until their current state of satiation is equal to their desired state. This is represented in the flow diagram in .
Figure 3. Flow chart showing foraging behaviour in the absence of constraints. Rust coloured boxes indicate monitoring and yellow boxes indicate acts of control. Blue boxes indicate evaluations and grey boxes indicate inputs that are temporally or physically ‘external’.

As can be seen, monitoring of the current internal state (rust coloured box) is distinct from control over the state, achieved through stopping or prolonging foraging behaviour (yellow boxes). The evaluation (blue box) is a simple comparison between the observed and desired state. The feedback loop creates an organic circuit (Dewey Citation1896) where control follows monitoring, in the sense that feelings of hunger are the relevant variable input into goal driven behaviour, but also monitoring follows control, in the sense that feelings of hunger indicate the state subsequent to foraging actions.
However, for most animals this model is too simple. By taking into consideration constraints and risks, the picture becomes more complicated and the stopping and prolonging behaviour more sophisticated.
Laland (Citation2017) describes the behaviour of two types of stickleback fish. Nine-spined sticklebacks have small spines and thin lateral plates making them vulnerable to predators. Three-spined sticklebacks, on the other hand, have three large dorsal spines as well as tough lateral plates, which makes them much safer from predators than their cousins. Due to this vulnerability difference, three-spined sticklebacks spend longer exploring the environment and sampling food patches through trial and error, whereas nine-spined sticklebacks are much more often found hiding from potential predators. The modulation of time spent on energy gathering activity, such as foraging, with respect to vulnerability to harm is an obviously adaptive trait.
Even in environments without imminent risk, foraging behaviour is regulated, as first detailed in Charnov’s Marginal Value Theorem (Citation1976). This theorem, which has been shown to be consistent with the behaviour of ‘worms, bees, wasps, spiders, fish, birds, seals, monkeys and human subsistence foragers’ (Gazzaniga, Ivry, and Mangun Citation2014, 525), states that an organism will change foraging location if the rate of change of energy in the current location is lower than the expected rate of return of energy from a competing foraging location. Here stopping foraging in a certain location is not based on predation risk, but on the more sophisticated optimisation of efficient foraging across multiple sites by incorporating opportunity cost computations. Being inefficient is still harmful in the long run, if not necessarily in the short run.
Animals are able to naturally combine these marginal value evaluations with assessments of vulnerability. For example, ‘the bluegill is able to assess changes in both foraging profitability and predation risk’ (Werner et al. Citation1983, 1545).
captures the added complexity. The evaluation now captures the full cost–benefit analysis of prolonging foraging, including the current state of satiation, and the constraints not captured by the simple model: foraging profitability, predation risk and opportunity cost.
Figure 4. Flow chart showing foraging behaviour in a constrained environment where profitability must be incorporated. Colouring as in .

It is uncontroversial to state that foraging involves both the physical act of foraging and the evaluation, innate or learned, conscious or unconscious, of when to stop foraging. I will assume that if E was the physical act of foraging, then readers would accept that for many animals the evaluation of whether to stop or prolong E-ing exists. I am simply arguing that for humans at least, the stopping and prolonging evaluation applies not just to physical acts but also to mental acts such as deliberation. This, I call metacognitive stopping and prolonging.
3.2. Procedural metacognition in animals
Metacognition is higher-order cognition, which includes the series of processes that monitor and control cognitive activity. Whilst metacognitive monitoring refers to the subjective assessment of one's own cognitive processes and knowledge, metacognitive control refers to the processes that regulate cognitive processes and behaviour.
Metacognition can be divided into analytic and procedural (Dokic Citation2012; Proust Citation2013). The former is reliant on meta-representation and involves explicit beliefs about first-order mental states and processes. Procedural metacognition, however, does not require meta-representation. It can be revealed through noetic feelings attached to cognitive processes, such as a feeling of knowing when considering a question. Unlike analytic metacognition, evidence suggests that procedural metacognitive monitoring exists in some non-human animals such as macaques.
Zakrzewski et al. (Citation2014; following Smith, Shields, and Washburn Citation2003) conducted an experiment where participants had to indicate whether a screen presented to them was densely or sparsely populated. Human and macaque participants accumulated food tokens for correct answers but lost all their tokens on an incorrect answer. Alternatively, before making the dense/sparse decision, participants could press a third button that allowed them to cash-out their chips. Inbuilt time delays on cash-out ensured that the optimal behaviour was to cash-out only if the decision was uncertain. Strikingly, macaques behaved even more optimally than humans,Footnote15 becoming more likely to cash-out as the stakes grew higher and more accumulated tokens were at risk. The two macaques averaged 82% accuracy when one coin was at risk, but this increased to 98% when 7 coins were at risk. They cashed-out when stakes were high unless the task was very easy. The authors concluded that macaques ‘monitor their uncertainty about the present trial and determine whether their probability of answering correctly justifies answering to build the reward cache higher without cashing-out’ (2014, 14). Cashing-out is a physical stopping behaviour, driven by the interaction between the metacognitive awareness of uncertainty and the risk of harm, as measured by potential token loss.
This experiment indicates that sophisticated animals such as macaques monitor decision uncertainty and are able to incorporate this monitored uncertainty into evaluations that drive physical actions, such as cashing out. This awareness of uncertainty has been interpreted as ‘the first tender shoots of metacognition’ (Vierkant Citation2022, 150). I will now turn to humans who are able to perform mental as well as physical actions. This allows them to not only monitor and evaluate, but also to intentionally control the cognitive process. Macaques may be able to engage in procedural metacognitive monitoring, but humans are able to exert full blown analytic metacognitive control.
3.3. Human metacognition and study time
There is a significant literature on metacognition, both procedural and analytic, in humans. Relevant to the current analysis is research into impacts of metacognition on allocations of study time.
Since the late 1990s, models of the connection between metacognition and study time in adultsFootnote16 have become increasingly sophisticated. Initial discrepancy reduction models (Dunlosky and Herzog Citation1998) suggested that adults continue to study until a perceived level of learning meets a desired level of learning. This mimics the simplified model of foraging () and the standard model of decision making (). More recently, however, it has been shown that study time is modulated by opportunity costs (Metcalfe and Kornell Citation2005). First, if faced with multiple problems, individuals will allocate time not to the hardest items, but to the easiest as-yet-unlearned items – picking the low hanging fruit. Second, adults use ‘their judgements of the rate of learning’ (Metcalfe and Kornell Citation2005, 465) to decide when to stop. When making decisions, timing is ‘modulated by a context-dependent urgency signal’ where the relevant context is the rate of evidence accumulation (Parés-Pujolràs et al. Citation2021, 8). This additional rate-of-change complexity mirrors that shown for foraging in . Indeed, Metcalfe and Jacobs suggest that study-time theory ‘could benefit from study of the more complex, but also more realistic, persistence equations used in optimal foraging theory’ (Citation2010, 218).
Following Metcalfe and Jacobs’ recommendation, is a suggested flow diagram for the cognitive process of making a decision, inspired by the earlier discussion of foraging. Foraging is replaced by what I have called evaluation E, while the metacognitive evaluation that incorporates urgency is the previously defined evaluation M.
Figure 5. Flow chart of the revised model of decision making. Colouring as in . Cognitive strategies include embodied, extended and social cognition as well as implementation of the norms of reasoning.

I will call this the revised model of decision making, to contrast it with the standard model.
Taken together, the above analysis suggests that stopping and prolonging evaluations are ubiquitous in nature. This is because in a constrained environment it is adaptively beneficial to allocate the time spent on any task appropriately, bearing in mind risk of harm, the available resources and opportunity costs. As Hayden et al. state, ‘deciding when to leave a depleting resource to exploit another is a fundamental problem for all decision makers’ (Citation2011, 933). Given this, it should not be surprising that the same principles are applied to deciding when to stop deliberation.Footnote17
In line with the previous discussion regarding foraging, the cost–benefit analysis of whether to stop or prolong cognition is informed by metacognitive monitoring of the rate of change of uncertainty as well as the level of uncertainty. How long a decision-making process would take in a world without constraints can diverge from how long that process should take when constraints are incorporated. There can be very hard decisions that must be taken quickly or easier decisions that should be made slowly. The guiding principle however, consistent with evolutionary fitness, is the appropriate allocation of a scarce resource.
In the case of simple animals, foraging time is subject to complex stopping and prolonging behaviour, but there is no metacognition. More sophisticated animals such as macaques seem to be not only aware of their uncertainty but able to incorporate this felt uncertainty in decision making. However, this metacognitive awareness is procedural rather than analytic. Finally, humans are able to conceptualise a feeling of uncertainty as such and represent it to themselves or others. This is fully fledged meta-representation. Importantly for this paper, humans are also able to engage in explicit metacognitive control via the intentional mental acts of stopping or prolonging cognition.
Having proposed a revised model of human decision, and explored its ‘phylogenetic refinement’ (Cisek Citation2019, 2265), I will now analyse how this revised model relates to contemporary theories of effort.
4. Cognitive effort and the symmetry argument
Metacognitive stopping can feel effortful, but what makes it so remains unclear. There has been much recent debate in psychology and philosophy of mind on the topic of effort. Discussions often consider effortful actions in general, but here I will retain my focus purely on the effort associated with mental actions.
In recent years, theories of mental effort have shifted from a focus on resource depletion towards a focus on cognitive resource allocation.
Resource depletion models understood will-power as a finite resource that is consumed by intentional mental acts, and mental effort as a measurement of the amount of the resource consumed. Originally an ego depletion model was hypothesised which suggested that mental effort tracked calorific depletion in the brain (Masicampo and Baumeister Citation2008). However, this received sustained criticism, particularly from Kurzban (Citation2010,Citation2016, Kurzban et al. Citation2013) who offered a rival theory, proposing that mental effort is the experiential manifestation of the calculated opportunity costs associated with a mental task (see also Székely and Michael Citation2020; Sripada Citation2021). I will call this the opportunity-cost theory.
A recent paper (André, Audiffren, and Baumeister Citation2019) suggests a similar model which I will call the integrated theory. The authors propose a neural network called the Mechanism of Effort which integrates constraints, perceived costs and benefits, and information relating to the current state of the organism. This mechanism then outputs ‘decisions regarding the intensity and the direction of the engagement in effort in ongoing or future tasks’ (André, Audiffren, and Baumeister Citation2019, 4), and a feeling of effort, that corresponds to the awareness of the perceived costs associated with achieving the goal of the task.
The discussion of the cost–benefit computation, evaluation M, in section 3 focussed on the mind-directed action that follows, but the perceived cost of foregoing alternative actions is an integral part of the computation. Although evaluation M leads to a binary decision, to stop or prolong deliberation, the result of the cost–benefit computation can be anywhere on a continuum from high relative benefit to almost equal cost and benefit. The opportunity-cost or integrated theories, claim that (for prolonging of cognition, at least) rather than being discarded, this more fine-grained information is revealed to the agent in the form of an analogue experience of effort. The same cost–benefit computation, or part thereof, that controls action is parsimoniously employed to modulate the intensity of the phenomenological experience:
According to the opportunity cost view, when systems that can be used for multiple purposes are engaged in a task, the potential benefit of ending the present task in order to perform some other task is computed. This computation is the opportunity cost of persisting in whatever it is that one is doing. (Kurzban Citation2016, 68–69)
The sensation of mental effort is the output of mechanisms designed to measure the opportunity cost of engaging in the current mental task. (Kurzban et al. Citation2013, 665)
If contemporary theories of effort support the existence of evaluation M in the context of effortfully prolonging cognition, they open up the possibility of symmetrically applying the same model to explain the central issue of this paper – what it is that makes stopping cognition effortful.
Metacognitive stopping is an act of cognitive control that follows from evaluation M, just as is the prolonging of cognition in the face of distractions. Cost–benefit computations are neutral with regards the direction of control. As Kurzban says, ‘according to the opportunity cost view … … When this cost is sufficiently great, outweighing the computation of the (potentially long-term) benefits of persisting the task is abandoned’ (Citation2016, 68–69).
A benefit of stopping cognition is an opportunity cost of prolonging cognition, and vice-versa. The same mechanism that outputs a feeling of effort if one action is chosen in the face of opportunity costs, will symmetrically output a feeling of effort if the alternative action is chosen in the face of opportunity costs.
Accounts such as Kurzban et al’s focus on the effort of prolonging cognition. However, the existence of effortful choice suggests a symmetry between metacognitive stopping and prolonging, consistent with that seen in physical stopping and prolonging and in cost–benefit computations more generally. By assuming symmetry in evaluation M one can explain the felt effort associated with making a difficult choice. Without this symmetry, difficult decisions would be unexplained, effortless or impossible.
Assuming symmetry one can state that:
When making a difficult choice, the computation that informs evaluation M leads to two connected outcomes; (i) the decision to stop deliberating and make a choice, and; (ii) the feeling that stopping deliberation is effortful, which is a function of the opportunity cost associated with stopping.
Readers may wonder how something as seemingly cold as the opportunity cost of an evaluation could be linked to feelings of effort. My suggestion is that in order for the idea of a cost–benefit computation to be more than a metaphor, an agent’s cognitive infrastructure must include an action-focused common-currency on which the computation can be performed. And for a decision to be an agent’s decision, that common-currency must be a contextually-relevant manifestation of her own values. When the question of what to do arises, relevant values are activated. Values in support of an action will be benefits, those against the action or in support of an incompatible action will be costs.Footnote18
As discussed in section 2.3, an agent is motivated to achieve a state of affairs consistent with her values. In the case of stopping deliberation, the opportunity costs in evaluation M reveal that the agent is motivated to reduce uncertainty. This motive doesn’t vanish but persists at least until the difficult choice is made.Footnote19 It must be effortfully resisted in trying to execute the intention. What is felt is the conative phenomenology of deciding-cum-trying (Kriegel Citation2015) to resolve indecision now, despite ongoing uncertainty.
Note that the opportunity-cost theory doesn’t only explain why an action is effortful, but also explains the strength of the feeling. The amount of effort felt in making a difficult choice is a function of the value of uncertainty reduction.Footnote20 As uncertainty and the risk of harmful error declines, stopping deliberation becomes less effortful. Equally, if the stakes are low there is less value to uncertainty reduction than in high stakes decisions, so effort is lower. The hardest choices are ones such as Sarte’s case of the young man torn between joining the Resistance and caring for his mother.Footnote21 Here the stakes and uncertainty were so high, that making a decision was seemingly impossible. I return to this case in section 5.
A natural conclusion of the above analysis is that an effortless decision is just one with no (or negligible) opportunity costs. The difference between easy and hard choices is one of degrees. I therefore assume that human cognitive architecture implements the revised model instead of, rather than alongside, the standard model.
In section 3, I proposed a revised model of human decision making that incorporated metacognitive stopping and prolonging. In this section, I have provided further support for this model by showing it makes sense of the relation between metacognitive control and the phenomenology of effort. Contemporary theories of effort suggest that the experience of mental effort originates from the same cost–benefit analysis that grounds metacognitive prolonging. Applying this finding symmetrically to metacognitive stopping explains the phenomenology of an effortful choice.
With these findings in hand, I turn to the implications for agency.
5. Implications for agency
If the account set out in this paper is correct, then the phenomenological gap with respect to the act of making a difficult choice has been closed.
Making a difficult choice is an action because it is preceded by the intention to resolve indecision, despite ongoing uncertainty. This intention is the culmination of a process that evaluates the costs and benefits of stopping cognition, weighing the value of uncertainty reduction against the costs of ongoing deliberation.
Making a difficult choice feels effortful because it involves opportunity costs, which are a function of the value of uncertainty reduction, and overcoming those opportunity-costs is felt. As Wegner described it towards the end of his life, the subjective experience gives us a ‘window on the lovely machinery’ (Citation2018, xvi).
However, although this conclusion satisfies the explanandum of this paper, it may feel unsettling to some readers, as the costs and benefits of the evaluation do not include any reasons or evidence for A-ing or ¬A-ing. When an agent effortfully makes a difficult choice, she is not asymmetrically biasing the decision towards one outcome or another, nor is she resolving uncertainty. She is merely stopping the deliberative process, and forcing herself to decide now, despite ongoing uncertainty. If the agent ends up deciding to A, it is not because she agentially shifts the decision in the A direction, but because she shifts the acceptable level of uncertainty higher.
The experience of effort may be an experience of the self as a source of force, but the agential power revealed by the analysis is perhaps more limited than some were hoping.
However, before resorting to denial, or despair, it is important to recall that the act of making a difficult choice sits between the extended deliberative process and the implementation of the chosen action-plan, and both of these can also involve effortful mental action.
This is why Sartre’s (Citation1946) example is so powerful. The young man faces a choice where all three stages are hard. The process of deliberation is extended and effortful. The stakes are so high that resolving indecision in the face of ongoing uncertainty is borderline impossible. And even if the young man did force himself to make a decision, he would presumably continue to vividly feel the attraction of the unchosen option.
Prolonged cognition can be effortful. This is the feeling that Kurzban seeks to explain. Other mental acts, such as directing attention or selecting and following mathematical or epistemic rules, may also be effortful and may indirectly influence the final decision. Strawson describes this intentional manipulation of deliberation as ‘shepherding’ (Citation2003, 232).
In some mental acts, the agent explicitly treats attitudes as object to be controlled. This requires a sophisticated ability to understand that beliefs can be false and decisions can result in self-harming actions. On the basis of such an understanding an agent can engage in future directed self-control, forming a resolution for example, that can be rehearsed when needed to reduce the likelihood of harm.
All of these mental actions add features to the phenomenological landscape. All require a level of metacognition that is extremely rare in the animal kingdom, and many require analytic meta-representation, which is uniquely human. Effortful mental actions that target cognition represent the zenith of 3.6bn years of phylogenetic refinement.
Additionally, after a difficult choice is made, effort will often be required in either inhibiting a reopening of the question or in executing the decision. The agent may have to resist the ongoing temptation to do otherwise.
The hardest decisions are effortful in advance of, during, and following intention formation, and this full temporally extended phenomenology contributes to the experience of agency throughout the decision-making process.
After having focused so narrowly on the effort felt in stopping deliberation, it is important, and perhaps comforting, to recognise that this is only one element in the rich and diverse experience that surrounds an act of intention formation.
6. Conclusion
A paradigmatic experience of freedom is the felt effort associated with the act of making a difficult choice. Vierkant has called the challenge of accounting for this experience within a compatibilist framework ‘the agency problem of compatibilism’ (Citation2022, 116).
Through analysis of Holton’s (Citation2006) proposal, the core of a solution was uncovered. Difficult decisions can be actions because they are made despite ongoing uncertainty. However, the challenge of providing an account that explained the phenomenology remained.
I argued that difficult choices are made when the agent decides to intentionally stop deliberating, despite ongoing uncertainty. This decision is the output of a metacognitive cost–benefit computation, evaluation M, which weighs the value of uncertainty reduction against the costs of ongoing deliberation. Evaluation M informs the metacognitive act of stopping (or prolonging) cognition, which is the metacognitive version of the evolutionarily antecedent ability to stop (or prolong) physical activity.
Contemporary theories of cognitive effort were reviewed. These, combined with the symmetry of cost–benefit computations, suggest that a single model can explain both the binary mind-directed act of stopping deliberation and the analogue experience of effort. When making a difficult decision, the computation that informs evaluation M leads to two connected outcomes; (i) the decision to stop deliberating and make the choice, and; (ii) the feeling that stopping deliberation is effortful, which is a function of the value of uncertainty reduction.
By analysing the evaluation that culminates in the intention to resolve indecision, one can finally solve the agency problem of compatibilism, and understand a central element of the experience of agency – the effortful act of making a difficult choice.
Acknowledgements
I am very grateful for helpful comments from Tillmann Vierkant, Suilin Lavelle, Graham Doke, and participants of the March 2022 ‘Neurophilosophy of Free Will’ Consortium in Palm Springs.
Disclosure statement
No potential conflict of interest was reported by the author(s).
Additional information
Funding
Notes on contributors
Jonathan J. Hall
Jonathan J. Hall is a PhD candidate in Philosophy of Mind at the University of Edinburgh. His research focuses on metacognition and the phenomenology of agency. He is also an external member of the Bank of England’s Financial Policy Committee.
Notes
1 In the Eddie Izzard sketch, ‘cake or death!’, the protagonist very quickly runs out of cake.
2 I set aside Massin’s third category of ‘muscular effort’.
3 In striking new research, it is proposed that ‘optimistic’ and ‘pessimistic’ neurons map the entire complex distribution rather than just the mean plus an error term (Dabney et al. Citation2020).
4 This idea of a threshold is widely used in cognitive science modelling of decision-making. For example, Parés-Pujolràs et al. describe the decision-making process as a ‘sequential sampling process, where evidence is sampled and accumulated, triggering a decision once a given threshold is reached’ (Citation2021, 1).
5 The assumption that the prior intention is non-actionally acquired is necessary to avoid (potentially infinite) regress.
6 In the language of cognitive science, evidence has been sampled but no decision one way or another has been triggered, because the required threshold has not been met.
7 This is necessary to put space between himself and libertarian Kane, who also believes that ‘exercises of free will by way of self-forming willings typically involve incommensurable alternatives’ (Citation1999, 168).
8 ‘A wealth of results … . indicates that neural representations of value exist in several brain areas and that lesions in some of these areas … specifically impair choice behavior. In essence, the brain actually computes values when subjects make economic choices’ (Padoa-Schioppa Citation2011, 334–335).
9 Research in neuroscience suggests that in drift diffusion models of decision making, the urgency parameter is operationalised via baseline shifts or gain modulation (Parés-Pujolràs et al. Citation2021; Steinemann et al. Citation2018). These reduce the amount of evidence required to reach the decision threshold.
11 Vierkant’s original quote refers to a ‘reopening of the question’ but in correspondence he has said that it applies equally to prolonging deliberation.
12 Kriegel’s terminology is that ‘trying involves the experience of mobilizing force in the face of resistance’. I assume, in line with the principle of least effort (Zipf, Citation1949; see also Massin Citation2017), that the force mobilized in trying to A is equal to the force required to inhibit ¬A-ing.
13 Thanks to an anonymous reviewer for encouraging clarification of this point.
14 ‘Neuroanatomical continuity is taken as a guiding principle’ (Cisek Citation2019, 2267).
15 Suboptimal human behaviour has been explained by overconfidence and/or perceiving that uncertainty responses are signs of weakness.
16 In children, the ability to monitor and report judgements-of-learning develops in advance of the ability to use that information to optimally control studying (Metcalfe and Finn Citation2013). Ontogeny parallels phylogeny.
17 This is supported by neurobiological findings which ‘lead to the compelling conclusion that the same cognitive and neural processes underlie much of human behaviour involving cognitive search – in both external [foraging] and internal [deliberation] environments’ (Hills et al. Citation2015, 47). Even more specifically, recent experiments in neuroscience indicate that both foraging (Hayden, Pearson, and Platt Citation2011; Li et al. Citation2012) and cognitive control (Shenhav, Botvinick, and Cohen Citation2013) are associated with activity in the anterior cingulate cortex.
18 See Berkman et al.’s ‘Self-Control as Value-Based Choice’ (Citation2017) for a similar view.
19 If it persists after the choice is made, the agent will have to resist a reopening of the question.
20 I recommend further research into the link between the rate of change of uncertainty and feelings of effort. My expectation is that a low rate of change increases the likelihood of stopping deliberation, but does not decrease the value of uncertainty reduction, so feelings of effort remain. However, a high rate of change would reduce the opportunity costs of prolonging. This might explain the state of flow where prolonged cognition is effortless or even pleasant.
21 Sartre also seems to endorse a value-based approach: ‘The value of his feeling for his mother was determined precisely by the fact that he was standing by her’ (Citation1946).
References
- André, N., M. Audiffren, and R. Baumeister. 2019. “An Integrative Model of Effortful Control.” Frontiers in Systems Neuroscience 13: 79. doi: 10.3389/fnsys.2019.00079.
- Bayne, T., and N. Levy. 2006. “The Feeling of Doing: Deconstructing the Phenomenology of Agency.” In Disorders of Volition, edited by N. Sebanz and W. Prinz, 49–68. Cambridge, MA: MIT Press.
- Berkman, E. T., C. A. Hutcherson, J. L. Livingston, L. E. Kahn, and M. Inzlicht. 2017. “Self-Control as Value-Based Choice.” Current Directions in Psychological Science 26 (5): 422–428. http://doi.org/10.1177/0963721417704394.
- Bogacz, R., E.-J. Wagenmakers, B. U. Forstmann, and S. Nieuwenhuis. 2010. “The Neural Basis of the Speed–Accuracy Tradeoff.” Trends in Neurosciences 33: 10–16. doi: 10.1016/j.tins.2009.09.002.
- Bratman, M. 1999. Intentions, Plans, and Practical Reason. Stanford, CA: Center for the Study of Language and Information.
- Charnov, E. 1976. “Optimal Foraging, the Marginal Value Theorem.” Theoretical Population Biology 9: 129–136. doi: 10.1016/0040-5809(76)90040-X.
- Cisek, P. 2019. “Resynthesizing Behavior Through Phylogenetic Refinement.” Attention, Perception, & Psychophysics 81: 2265–2287. doi: 10.3758/s13414-019-01760-1.
- Dabney, W., Z. Kurth-Nelson, N. Uchida, C. K. Starkweather, D. Hassabis, R. Munos, and M. Botvinick. 2020. “A Distributional Code for Value in Dopamine-Based Reinforcement Learning.” Nature 577 (7792): 671–675. doi: 10.1038/s41586-019-1924-6.
- Davidson, D. 1980. Essays on Actions and Events. Oxford: Clarendon Press.
- Dewey, J. 1896. “The Reflex arc Concept in Psychology.” Psychological Review 3: 357–370. doi: 10.1037/h0070405.
- Dokic, J. 2012. “Seeds of Self-Knowledge: Noetic Feelings and Metacognition.” In Foundations of Metacognition, edited by M. Beran, J. Brandl, J. Perner, and J. Proust, 302–321. Oxford: Oxford University Press.
- Dunlosky, J., and C. Herzog. 1998. “Training Programs to Improve Learning in Later Adulthood: Helping Older Adults Educate Themselves.” In Metacognition in Educational Theory and Practice, edited by D. J. Hacker, J. Dunlosky, and A. C. Graesser, 249–275. Florence: Routledge.
- Gazzaniga, M., R. Ivry, and G. Mangun. 2014. Cognitive Neuroscience: The Biology of the Mind, 525-526. New York: W.W. Norton and Company.
- Hayden, B., J. Pearson, and M. Platt. 2011. “Neuronal Basis of Sequential Foraging Decisions in a Patchy Environment.” Nature Neuroscience 14: 933–939. doi: 10.1038/nn.2856.
- Hieronymi, P. 2009. “Two Kinds of Agency.” In Mental Actions, edited by L. O'Brien and M. Soteriou, 138–162. Oxford: Oxford University Press.
- Hills, T., P. M. Todd, D. Lazer, A. D. Redish, and I. D. Couzin. 2015. “Exploration Versus Exploitation in Space, Mind, and Society.” Trends in Cognitive Sciences 19: 46–54. doi: 10.1016/j.tics.2014.10.004.
- Hobbes, T. 1997. “Leviathan or the Matter Form and Power of a Commonwealth Ecclesiastical and Civil.” In The English Works of Thomas Hobbes of Malmesbury, Vol. 3, 44, edited by W. Molesworth.
- Holton, R. 2006. “The act of Choice.” Philosophers' Imprint 6: 1–15.
- James, W. 2014. “The Dilemma of Determinism.” In The Will to Believe: And Other Essays in Popular Philosophy, 145–183. Cambridge University Press.
- Kane, R. 1999. “Moral and Prudential Choice.” In The Significance of Free Will, 124–151. New York: Oxford University Press.
- Kavka, G. S. 1983. “The Toxin Puzzle.” Analysis 43 (1): 33–36. doi: 10.1093/analys/43.1.33.
- Kriegel, U. 2015. “Conative Phenomenology.” In The Varieties of Consciousness, 72–96. New York: Oxford University Press.
- Kurzban, R. 2010. “Does the Brain Consume Additional Glucose During Self-Control Tasks?” Evolutionary Psychology 8: 244–259. doi: 10.1177/147470491000800208.
- Kurzban, R. 2016. “The Sense of Effort.” Current Opinion in Psychology 7: 67–70. doi: 10.1016/j.copsyc.2015.08.003.
- Kurzban, R., A. Duckworth, J. Kable, and J. Myers. 2013. “An Opportunity Cost Model of Subjective Effort and Task Performance.” Behavioral and Brain Sciences 36: 661–679. doi: 10.1017/S0140525X12003196.
- Laland, K. 2017. Darwin’s Unfinished Symphony. Princeton, NJ: Princeton University Press.
- Levy, D. J., and P. W. Glimcher. 2012. “The Root of all Value: A Neural Common Currency for Choice.” Current Opinion in Neurobiology 22 (6): 1027–1038. doi: 10.1016/j.conb.2012.06.001.
- Li, F., M. Li, W. Cao, Y. Xu, Y. Luo, X. Zhong, J. Zhang, Ruping, D., Xin-Fu, Z., Zhiyuan, L., Changqi, L. 2012. “Anterior Cingulate Cortical Lesion Attenuates Food Foraging in Rats.” Brain Research Bulletin 88: 602–608. doi: 10.1016/j.brainresbull.2012.05.015.
- Loewenstein, G. 1994. “The Psychology of Curiosity: A Review and Reinterpretation.” Psychological Bulletin 116: 75–98. doi: 10.1037/0033-2909.116.1.75.
- Masicampo, E., and R. Baumeister. 2008. “Toward a Physiology of Dual-Process Reasoning and Judgment: Lemonade, Willpower, and Expensive Rule-Based Analysis.” Psychological Science 19: 255–260. doi: 10.1111/j.1467-9280.2008.02077.x.
- Massin, O. 2017. “Towards a Definition of Efforts.” Motivation Science 3 (3): 230–259. doi: 10.1037/mot0000066.
- Mele, A. (2017). “Deciding to Act.” In Aspects of Agency: Decisions, Abilities, Explanations, and Free Will, 7–26. New York: Oxford University Press.
- Metcalfe, J., and B. Finn. 2013. “Metacognition and Control of Study Choice in Children.” Metacognition and Learning 8: 19–46. doi: 10.1007/s11409-013-9094-7.
- Metcalfe, J., and W. Jacobs. 2010. “People’s Study Time Allocation and its Relation to Animal Foraging.” Behavioural Processes 83: 213–221. doi: 10.1016/j.beproc.2009.12.011.
- Metcalfe, J., and N. Kornell. 2005. “A Region of Proximal Learning Model of Study Time Allocation.” Journal of Memory and Language 52: 463–477. doi: 10.1016/j.jml.2004.12.001.
- Miščević, N. 2020. Curiosity as an Epistemic Virtue. Cham, Switzerland: Palgrave Macmillan.
- Musslick, S., and J. Cohen. 2021. “Rationalizing Constraints on the Capacity for Cognitive Control.” Trends in Cognitive Sciences 25: 757–775. doi: 10.1016/j.tics.2021.06.001.
- O’Shaughnessy, B. 1980. The Will. Vol. 2. Cambridge: Cambridge University Press.
- Padoa-Schioppa, C. 2011. “Neurobiology of Economic Choice: A Good-Based Model.” Annual Review of Neuroscience 34: 333–359. doi: 10.1146/annurev-neuro-061010-113648.
- Parés-Pujolràs, E., E. Travers, Y. Ahmetoglu, and P. Haggard. 2021. “Evidence Accumulation Under Uncertainty - a Neural Marker of Emerging Choice and Urgency.” NeuroImage 232: 117863. doi: 10.1016/j.neuroimage.2021.117863.
- Pink, T. (1996). “In Defence of the Action Model.” In The Psychology of Freedom. Cambridge University Press.
- Proust, J. 2013. “Primate Metacognition.” In The Philosophy of Metacognition: Mental Agency and Self Awareness, 79–109. Oxford: Oxford University Press.
- Sartre, J.-P. 1946. Existentialism Is a Humanism. Reproduced in Existentialism from Dostoyevsky to Sartre (1989). New York: New American Library.
- Shenhav, A., M. Botvinick, and J. Cohen. 2013. “The Expected Value of Control: An Integrative Theory of Anterior Cingulate Cortex Function.” Neuron 79: 217–240. doi: 10.1016/j.neuron.2013.07.007.
- Shepherd, J. 2015. “Deciding as Intentional Action: Control Over Decisions.” Australasian Journal of Philosophy 93: 335–351. doi: 10.1080/00048402.2014.971035.
- Smith, D., B. Y. Hayden, T.-K. Truong, A. W. Song, M. L. Platt, and S. A. Huettel. 2010. “Distinct Value Signals in Anterior and Posterior Ventromedial Prefrontal Cortex.” The Journal of Neuroscience 30: 2490–2495. doi: 10.1523/JNEUROSCI.3319-09.2010.
- Smith, J., W. Shields, and D. Washburn. 2003. “The Comparative Psychology of Uncertainty Monitoring and Metacognition.” Behavioural and Brain Sciences 26: 317–373. doi: 10.1017/S0140525X03000086.
- Sripada, C. 2021. “The Atoms of Self-Control.” Noûs 55 (4): 800–824. http://doi.org/10.1111/nous.v55.4.
- Steinemann, N. A., R. G. O’Connell, and S. P. Kelly. 2018. “Decisions are Expedited through Multiple Neural Adjustments Spanning the Sensorimotor Hierarchy.” Nature Communications 9 (1): Article number: 3627. http://doi.org/10.1038/s41467-018-06117-0.
- Strawson, G. 2003. “Mental Ballistics or the Involuntariness of Spontaneity.” Proceedings of the Aristotelian Society 103 (1): 227–256. doi:10.1111/j.0066-7372.2003.00071.x
- Székely, M., and J. Michael. 2020. “The Sense of Effort: A Cost-Benefit Theory of the Phenomenology of Mental Effort.” Review of Philosophy and Psychology 12 (4): 889–904. doi: 10.1007/s13164-020-00512-7.
- Tinbergen, N. 1963. “On Aims and Methods of Ethology.” Zeitschrift für Tierpsychologie 20: 410–433. doi: 10.1111/j.1439-0310.1963.tb01161.x.
- Velleman, J. D. 1992. “What Happens When Someone Acts?” Mind; A Quarterly Review of Psychology and Philosophy 101: 461–481. doi: 10.1093/mind/101.403.461.
- Vierkant, T. 2018. “Choice in a two Systems World: Picking and Weighing or Managing and Metacognition.” Phenomenology and the Cognitive Sciences 17: 1–13. doi: 10.1007/s11097-016-9493-8.
- Vierkant, T. 2022. The Tinkering Mind: Agency, Cognition and the Extended Mind. Oxford: Oxford University Press.
- Wegner, D. 2018. The Illusion of Conscious Will. New Edition. Cambridge, MA: MIT Press.
- Werner, E., J. Gilliam, D. Hall, and G. Mittelbach. 1983. “An Experimental Test of the Effects of Predation Risk on Habitat Use in Fish.” Ecology 64 (6): 1540–1548. doi: 10.2307/1937508.
- Westbrook, A., and T. S. Braver. 2016. “Dopamine Does Double Duty in Motivating Cognitive Effort.” Neuron 89: 695–710. doi: 10.1016/j.neuron.2015.12.029.
- Zakrzewski, A., Perdue, B. M., Beran, M. J., Church, B. A., and Smith, J. D. 2014. “Cashing Out: The Decisional Flexibility of Uncertainty Responses in Rhesus Macaques (Macaca mulatta) and Humans (Homo sapiens).” Journal of Experimental Psychology: Animal Learning and Cognition 40 (4): 49–501. doi: 10.1037/xan0000041.
- Zipf, G. K. 1949. Human Behavior and the principle of least effort. Addison Wesley Press.