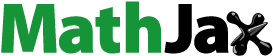
ABSTRACT
A challenge associated with driving vehicles can be navigating to destinations. While driving experience would seem beneficial for improving navigation skill, it remains unclear how driving experience relates to wayfinding ability. Using the mobile video game-based wayfinding task Sea Hero Quest, which is predictive of real-world navigation, we measured wayfinding ability in US-based participants (n = 694, mean age = 26.8 years). We also asked travel-related self-report questions, including those relating to driving experience. A multiple linear regression model found that those who started driving solo below aged 18 had significantly better wayfinding ability than those starting to drive solo aged 18 and above.
1. Introduction
Being able to maintain a sense of direction and location in order to find our way in different environments is a fundamental cognitive function that relies on multiple cognitive and perceptual processes (Newcombe et al., Citation2022; Spiers et al., Citation2023; Wolbers & Hegarty, Citation2010). Human navigation involves a range of processes such as planning routes, reading maps, identifying landmarks and maintaining a sense of direction (Ekstrom et al., Citation2018; Newcombe et al., Citation2022).
Getting lost in everyday environments can lead to distress for patients and family members and in extreme cases death from exposure (Coughlan et al., Citation2018). Additionally, navigation deficits may constitute the earliest signs of Alzheimer’s Disease (Coughlan et al., Citation2018) and be apparent in other disorders such as Traumatic Brain Injury (Seton et al., Citation2023). Moreover, understanding how navigation ability develops in early life and the individual differences that accompany its development are important for furthering the field of spatial cognition at large, and more broadly the factors that contribute to healthy cognitive development. Creating a valid test of navigation that accounts for the wide variation in navigation ability is challenging, given the large sample sizes needed and high levels of environmental manipulation and experimental control required in standard research settings (Newcombe et al., Citation2022). With the recent evolution of widespread touch-screen technology on both tablet and mobile devices and virtual reality (VR), our team developed a series of navigation tests in the form of a mobile video game app Sea Hero Quest (SHQ) (Spiers et al., Citation2023). SHQ has since been used to test the navigation ability of 4 million people globally, has good test-retest reliability (18-month test-retest intraclass correlation coefficient = .50) and is predictive of real-world navigation ability (Coughlan et al., Citation2020; Coutrot et al., Citation2018, Citation2019).
Many studies have shown that spatial cognition is associated with driving ability, where poorer performance on various facets of spatial cognition, including spatial perspective taking, mental rotation and visuospatial processing performance, is associated with worse driving ability (Andrews & Westerman, Citation2012; DiMeco et al., Citation2021; Kunishige et al., Citation2020; Moran et al., Citation2020; Tinella et al., Citation2020, Citation2021a, Citation2021b). Despite these associations between driving ability and spatial cognition, it remains unclear how driving experience per se is associated with spatial cognition. Additionally, no studies have investigated how driving experience relates to wayfinding in particular, a key facet of spatial cognition required for successful spatial navigation where one must actively navigate to a goal from a start location using an internal or external representation of their environment (e.g., in the form of a mental- or cartographic map respectively) (Patai & Spiers, Citation2021). Both poorer driving and wayfinding ability are associated with greater risk of cognitive decline and neurodegenerative disease (Carr et al., Citation2011; Coughlan et al., Citation2019; Duchek et al., Citation2003; Ott et al., Citation2008; Roe et al., Citation2016; Uc et al., Citation2017), where current drivers have significantly slower cognitive decline than former drivers (Choi et al., Citation2013). Examining the association between driving experience and wayfinding ability may therefore provide a better understanding as to why certain individuals are at greater risk of cognitive decline.
Given the many aforementioned studies showing significant positive associations between driving ability and spatial cognition, we hypothesized that those with greater driving experience, defined by greater hours of weekly driving, a younger age at which one learns to drive and a younger age at one starts to drive solo, would have better wayfinding ability. We also included self-reported measures of GPS use, video games experience, and other related variables as covariates in our analysis because of their potential relation to wayfinding ability (see Yavuz et al., Citation2023a, Citation2023b).
2. Methods
2.1. Participants
903 participants living in the US aged 18 and above (395 men, 489 women, mean age = 27 years, SD = 8.0 years, range = 18–66 years, mean number of years of formal education = 16.1 years, 315 currently living in cities, 588 currently living outside cities) were recruited using the Prolific database (www.prolific.co, 2023) and reimbursed for their time. Ethical approval was obtained from the University College London Review Board conforming to Helsinki’s Declaration. All participants provided informed written consent. We first removed 94 participants who reported that they did not currently drive (12% of the sample), and then removed an additional 17 participants who selected “other” for gender given that there were too few participants in this category to run the mediation analysis. We next identified outliers using Mahalanobis’ Distance, a method shown to have high sensitivity and specificity and a minimal change in bias in simulated and real datasets based on questionnaire data when removing outliers compared to other methods (Curran, Citation2016; Zijlstra et al., Citation2011)(Supplementary Materials). We removed 98 participants as outliers. This resulted in a final sample size of 694 participants (291 men, 403 women, mean age = 26.8 years, SD = 7.4 years, range = 18–52 years, mean number of years of formal education = 16.2 years, 232 currently living in cities, 462 currently living outside cities). Demographic information for the final sample is summarized in . Data analysis was completed using R studio (version 1.4.1564) and Python (version 3.9.12).
Table 1. Summary of basic descriptive statistics for the variables included in the model (weighted wayfinding distance, age, weekly hours of video game use on all devices, weekly hours of phone use) for men and women separately, and across gender. Mean and standard deviation (SD) values are shown.
2.2. Statistical power analysis
A power analysis was conducted using G* power (Erdfelder et al., Citation1996). Given that a previous study looking at the association between spatial mental transformation skills and driving performance found medium-to-large effect sizes (Tinella et al., Citation2020), it was determined that 694 participants were sufficient to achieve a medium effect size (Cohen’s f2 = .15) at an alpha threshold of .05 with 95% power (Selya et al., Citation2012).
2.3. Experimental procedure
2.3.1. Self-report questionnaires
To characterize driving-related activities, participants answered a series of driving-related questions including the number of hours spent driving, the age they learnt to drive and the age they started driving solo. We also asked participants a broader series of questions about travel-related activities, including the hours they spent cycling, biking, boating and taking public transport per week (five separate questions), the hours of weekly exercise they engaged in, the hours of weekly exercise they engaged in within the last 6 months and the mode in which they thought about space (thinking in distance, blocks or time)(Supplementary Materials). The number of hours per week spent biking, boating and taking public transport were converted to binary yes/no variables (weekly biking yes/no, weekly boating yes/no, weekly public transport yes/no), given the very small number of participants who engaged in these activities on a weekly basis.
To characterize reliance on GPS, the GPS reliance scale was used (Dahmani & Bohbot, Citation2020). This scale has seven items and assesses the frequency at which people have relied on GPS in different situations within the past month (e.g., “How often do you use GPS to travel new routes to a previously visited destination?”). The average score across questions was calculated for each participant. To characterize video game use, participants were asked to indicate the number of hours per week spent playing video games on all devices and the number of hours of phone use per week. Participants were also asked to report their age, gender and highest education level. Please see the Supplementary Materials for the full set of questionnaires.
2.3.2. Sea hero quest task
Sea Hero Quest (SHQ) is a VR-based video game for mobile and tablet devices which requires participants to navigate through a three-dimensional rendered world in a boat to search for sea creatures in order to photograph them, with the environment consisting of ocean, rivers and lakes (Coutrot et al., Citation2018; Spiers et al., Citation2023) (). We asked participants to play 5 levels – levels 1, 11, 32, 42 and 68 - where level 1 was a tutorial level designed to assess one’s ability to control the boat, whilst the latter 4 levels were wayfinding levels. We selected these specific levels as they showed the greatest effect sizes when investigating the effect of one’s home environment on wayfinding performance in a previous study (Coutrot et al., Citation2022). The wayfinding levels increased in difficulty from level 11 to 68, with the difficulty of a given level based on the number of goals and how far apart they were from each other (see Yesiltepe et al., Citation2023). Participants were required to play all 5 levels, where playing a given level would unlock the next level. For each participant, we quantified the wayfinding distance, defined as the euclidean distance traveled between each sampled location in pixels, for levels 11, 32, 42 and 68 separately. We then divided the wayfinding distance in each level by the wayfinding distance in level 1 to control for the effects of game experience on navigation performance. To control for the difference in wayfinding distance between levels, we then z-scored the distances within each level and averaged these across the levels. This resulted in each participant having a z-score which represented their wayfinding distance across the 4 levels. This z-score was referred to as the weighted wayfinding distance. A shorter weighted wayfinding distance indicated better navigation performance (i.e., a more efficient route to the goal).
(A) At the start of each level presented participants were presented with a map indicating the goals which they had to navigate to in the order indicated, where “1” indicates goal number 1 etc. (the map from level 68 is shown as an example). Level 1 (not shown) provided one goal and a simple river to reach the goal as training. (B) Participants selected to close the map by pressing “close,” at which point the participant had to translate from an allocentric (survey) perspective into a third-person egocentric (route) perspective in order to start navigating to the goals. Third-person view of the environment is shown where the participant tapped left or right of the boat to steer it. (C) Examples of individual player trajectories (level 68) from the start location to the final goal. Trajectories are ordered by performance, with the top left providing the best performance (shortest trajectory length), through to bottom right who has the worst performance (longest trajectory length). Adapted from Coutrot et al. (Citation2018).
3. Data analysis
3.1. Multiple linear regression
We conducted a multiple linear regression model to analyze the effects of the hours of driving per week, the age one started driving solo and the age one started to learn to drive on wayfinding distance. Covariates included age, gender, highest education level, weekly hours of video gaming on all devices, weekly hours of phone use, hours of daily travel, hours of walking per week, engaging in weekly public transport (yes/no), engaging in weekly boating (yes/no) and engaging in weekly cycling (yes/no), average GPS reliance score and one’s mode of thinking about space (whether they usually thought about space in terms of blocks, distance or time). These covariates were chosen to control for variables that may influence the association between driving-related variables and spatial navigation ability (Ben-Elia, Citation2021; Coutrotet al., Citation2022; Jabbari et al., Citation2022; Sahlqvist et al., Citation2012; Yavuz et al., Citation2023a, Citation2023b). Given that the majority of participants had a daily travel time of 0–.5 hours per day, we binned daily travel time into two groups before entering this variable into the model: 1. Those traveling for less than .5 hours per day and 2. Those traveling for .5 hours or greater per day. Additionally, given that our sample was solely based in the US, where most urban environments have a grid-like structure (Coutrotet al., Citation2022), we grouped the mode of thinking about space into two groups before entering this variable into the model: 1. Those thinking about space in terms of “blocks” and 2. Those thinking about space in terms of “distance” or “time.” Finally, hours of weekly exercise over the last 6 months was binned into two groups before entering this variable into the model: 1. Up to 2 hours per week and 2. 2 hours or more per week.
As a check for multicollinearity, we calculated the variance inflation factor (VIF) for each predictor variable in the model (Supplementary Tables S1 and S2). A VIF value of < 5 indicates that multicollinearity is not a concern (Fox & Monette, Citation1992; Kim, Citation2019). Post-hoc t-tests Bonferroni-corrected for multiple comparisons to control for type 1 errors were carried out where main effects and interactions were significant. When running post-hoc tests for the driving-related variables (age one started to drive solo and age one started to learn to drive), we binned the data into two categories: <18 and ≥18 years of age, given that 18 years old is the youngest age at which one can obtain a driving license without any imposed driving restrictions (Wang et al., Citation2020).
3.2. Mediation analysis
After running the multiple linear regression model, we conducted a mediation analysis to see whether the effect of each of the independent predictor variables that showed a significant association with wayfinding distance was mediated by a. age, b. gender, c. hours per week gaming on all devices, d. whether one grew up in a city or not, given previous associations between age, gender, gaming experience and the environment one grew up in and their wayfinding performance on SHQ (see for more: Coutrot et al., Citation2018, Citation2022; Yavuzet al., Citation2023a, Citation2023b). We also included the current environment one lives in at the time of the study (city or not) as a mediator for the effect of weekly biking (yes/no) on wayfinding distance, as we were interested in whether the environment one currently lives in would influence the effect of travel-related activities on navigation. Non-parametric bootstrapping with 5000 simulations was used, with 95% Confidence Intervals reported. Binary logistic regressions and multiple linear regression models were run to determine the significance and direction of association of the paths from the predictor variable to the mediator variable, and from the mediator variable to the outcome variable.
4. Results
4.1. Multiple regression analysis – the age of one started to drive solo was significantly associated with wayfinding distance when controlling for other variables
We conducted a linear multiple regression model to verify these associations when controlling for the associations of other variables with wayfinding distance, with these travel-related variables as key variables of interest, and with age, gender, hours of weekly gaming on all devices and hours of weekly phone use as covariates. Interactions between gender and each of the sleep variables were included in the model (). Before running the regression, as a check for multicollinearity, we calculated the value for each predictor variable in the model. This revealed that all the predictor variables had a
value that was less than 5, indicating that multicollinearity was not a concern (Supplementary Table S1).
Table 2. Model output. P-values for the significant associations are highlighted in bold. ͣ the Cohen’s f2 effect size for daily travel time was calculated for the main effect of the variable as a whole rather than for each category/level of the variable.
The outputs from the multiple linear regression model are as follows:
4.2. Main variables of interest
Age one learnt to drive, age one started driving solo and weekly hours of driving.
There was a significant main effect of the age one started to drive solo on wayfinding distance (β = .11, f2 = .01, p = .015, CI = [.02, .19]) ( and ). Post-hoc t-tests revealed that those who started to learn to drive solo below 18 years old were significantly better navigators than those who started to learn to drive solo aged 18 and above (t = −2.07, p = .039). Neither the age one learnt to drive (β = −.06, f2 = <.01, p = .172, CI = [−.14, .03]) or the weekly hours one spent driving (β = .01, f2 = <.01, p = .793, CI = [−.04, .05]) were significantly associated with wayfinding distance ( and Supplementary Figure S1A-B). The other travel-related variables included in the model did not show a significant association with wayfinding distance (p > 0.05), except from a significant main effect of whether one bikes weekly on wayfinding distance (β = .18, f2 = .02, p = .003, CI = [.06, .29]).
Figure 2. Weighted wayfinding distance in those who started to learn to drive below 18 compared with those who started to drive solo at 18 or above. VR-m = virtual reality meters. Data points represent the mean wayfinding distance across game levels across participants. Bars represent the standard error of the mean corresponding to this wayfinding distance.

4.3. Covariates
Please see Supplementary Figures S2A-M for visualizations of the associations between each of the covariates with wayfinding distance.
4.4. Mediation analysis – the significant association between the age one started to drive solo and wayfinding distance was not significantly mediated by age, gender, gaming experience, home environment or current environment
A mediation analysis was run to determine whether the significant association between the age one started to drive solo and wayfinding distance was mediated by age, gender, weekly hours of video game use on all devices, the type of environment one grew up in (city or not) - home environment -and the type of environment one currently lives in (city or not) - current environment. Although not a main variable of interest, we also ran the same mediation analysis for the association between whether one bikes weekly and wayfinding distance, given that it was significant.
The significant association between the age one started driving solo and wayfinding distance was not significantly mediated by either age, gender, gaming hours per week on all devices, whether one grew up in a city or whether one currently lives in a city, as shown by the non-significant indirect effects of these variables (p > .05) (Supplementary tables S2-S6).
The significant association between whether one bikes weekly and wayfinding distance was significantly mediated by gender, as shown by a significant indirect effect of gender (p = .034)(Supplementary table S8). A binary logistic regression model showed that those who engage in weekly biking are significantly more likely to be female than those not (β = .58, OR = 1.78, p = .019, CI = [.10, 1.06]) (Supplementary table S9), where females were significantly worse navigators than males (β = −.18, f2 = .06, p = .001, CI = [−.28, −.08]) (Table S9). The significant association between weekly biking (yes/no) and wayfinding distance was not significantly mediated by either age, gaming hours per week on all devices, whether one grew up in a city or whether one currently lives in a city, as shown by the non-significant indirect effects of these variables (p > .05) (Supplementary tables S7,S10, S11 and 12).
5. Discussion
Although many studies have shown that specific facets of spatial cognition are associated with driving experience and performance (Andrews & Westerman, Citation2012; DiMeco et al., Citation2021; Kunishige et al., Citation2020; Moran et al., Citation2020; Tinella et al., Citation2020, Citation2021a, Citation2021b), our study was the first to investigate the relationship between self-reported driving experience and measured wayfinding ability. As hypothesized, we found a significant association between the age one started to drive solo and wayfinding distance, where those who started driving solo below 18 were significantly better at navigating than those who started driving solo aged 18 and above. Specifically, when comparing those learning to drive below aged 18 to those doing so at aged 18 and above, we found a Cohen’s d effect size of .2. This effect size is within the range expected for such an effect. Notably, a recent article showed a median effect size of .16 for pre-registered studies (Schäfer & Schwarz, Citation2019). This significant association was not significantly mediated by either age, gender, video game experience, the type of environment one currently lives in or the type of environment one grew up in, suggesting that these factors could not explain why those who started driving solo at a younger age were better navigators. However, we did not find a significant association between hours of weekly driving or the age one started to learn to drive with wayfinding ability.
One potential explanation for the link between learning to drive and navigation ability is that those people who learn earlier have greater spatial perspective taking, mental rotation and visuospatial processing ability which lead to being able to learn to drive faster and learning to navigate better. This is supported by evidence that better performance on different facets of spatial cognition are associated with better driving performance (Andrews & Westerman, Citation2012; DiMeco et al., Citation2021; Kunishige et al., Citation2020; Moran et al., Citation2020; Tinella et al., Citation2020, Citation2021a, Citation2021b) and navigation ability (Garg et al., Citation2023; Wolbers & Hegarty, Citation2010). Indeed, spatial skills can predict driving test success in clinical and non-clinical samples (Barco et al., Citation2014; Barrash et al., Citation2010; Crizzle et al., Citation2012). An alternative explanation is that those learning to drive solo at a younger age may use their new driving skill to spend more time exploring new places over a wider range than the others, giving them greater exposure to navigational challenges and honing navigation skill. This explanation is consistent with research suggesting exposure to different experiences at a young age can shape later life abilities (Everson-Rose, Citation2003; Lee & Schafer, Citation2020). More specifically, this explanation is consistent with our recent research using Sea Hero Quest that found a link between the street network entropy of the environment encountered growing up and later life spatial ability (Coutrot et al.,Citation2022). The greater sample size of those who started driving solo below 18 years old (n = 560) compared with those who started driving solo at aged 18 and above (n = 134) could be explained by the fact that those living in the US are reliant on using cars for transport in most places, making learning to drive early important for one’s independence. This would also align with the notion that exposure to different experiences in youth can shape later life abilities. Finally, it is also possible that the better wayfinding performance in those below the age of 18 was driven by the fact that those who started to drive solo at a younger age did so purely because they wanted to learn to drive or because they were able to due to their personal circumstances, such as the ability to afford lessons or access a vehicle, providing them with greater opportunity to practice their driving skills.
One possibility that we considered was that those who started driving alone at a younger age were participants who had grown up in rural settings, and due to the advantage conferred from rural environments on navigation skill (Coutrot et al., Citation2022), had gained greater spatial skills from the environment rather than the driving. However, the non-significant mediation effect of both the environment one grew up in and currently lives in make this possibility unlikely. If one learns to drive early it would suggest they would likely have longer experience in driving, which in itself could lead to greater spatial skills. Given that we did not ask participants about the number of years of driving experience they had, we were unable to determine whether the effect of starting to drive solo at a younger age was associated with simply having a greater number of years of driving experience. However, the non-significant association between the age one learnt to drive and wayfinding ability suggests that the period of time over which one drives is unlikely to account for the significant association between the age one started to drive solo and wayfinding ability. Rather, it appears that the early exposure to driving or the aptitude to learn to drive are more important in determining navigation ability.
It is important to consider that not all studies have shown that spatial cognition is associated with driving performance. Accordingly, a recent study showed that memory and global cognitive function were significant predictors of driving performance whilst visuospatial processing was not (Ledger et al., Citation2019). Other affect-related measures such as trait anger and behavioral impulsivity have also been associated with poorer self-reported and objective driving performance (Difonzo et al., Citation2022; Stojan & Voelcker-Rehage, Citation2021; Yu et al., Citation2022). Additionally, attitudes toward driving, such as whether one enjoys driving, may have also influenced the association between the age one started to drive solo and wayfinding performance.
5.1. Limitations and future directions
Whilst our study allowed assessment in 694 participants on a virtual navigation task predictive of real-world navigation (Coutrot et al., Citation2019) and good reliability for test-retest assessment (Coughlan et al., Citation2020), there are a number of limitations future studies could consider and address. It would be useful to explore other variables related to driving, such as driving frequency, years of driving experience, the distance covered and the type of environment one usually drives in, using direct driving-related metrics, such as distance traveled daily and diversity of routes taken. Additionally, the participant sample we recruited using prolific may not have been representative of the general population, and so recruiting participants from a wider range of sources would help overcome this issue. Furthermore, we did not ask whether any participants were professional drivers during data collection, which may have biased our findings, and so future studies should discriminate between professional and nonprofessional drivers when understanding how driving may relate to cognitive abilities such as spatial navigation. Additionally, the SHQ wayfinding task used here favors the use of a survey (allocentric) spatial navigation strategy – seeing a top-down view of the environment before navigating, which has previously been used as an explanation for a navigation advantage in men (Munion et al., Citation2019). Finally, SHQ is a video game in itself, potentially resulting in those who game being more motivated to complete the task. Accordingly, future studies would benefit from longitudinal designs, given the reciprocal relationship between driving and cognitive function (Choi et al., Citation2013; Edwards et al., Citation2008), and the use of a broader navigation tests that extend to real-world environments (Brown et al., Citation2010; Burles & Iaria, Citation2020; Coutrot et al., Citation2019; He et al., Citation2021; Hegarty et al., Citation2006; Hill et al., Citation2023; Howard et al., Citation2014; Javadi et al., Citation2019, Patai et al., Citation2019; van der Ham et al., Citation2022). Critically it will be important to examine whether greater spatial cognitive skills predict learning to drive solo, or whether it is the exposure to environment made possible by driving early, or a combination, that predicts later navigation ability.
6. Conclusion
Overall, our findings suggestthat early life experiences, such as driving, may be important in predicting future navigation ability. Accordingly, our findings provide a platform for future research looking into how actively engaging in such activities in early life may improve one’s ability to navigate in complex environments and drive the individual differences in navigation ability, as well as research investigating whether interventions based on training visuospatial abilities can be used to improve driving safety.
Code availability
The code used to produce this data is available at: https://osf.io/cugdw/
Supplemental Material
Download MS Word (2.8 MB)Acknowledgments
We would like to thank all the participants who volunteered to take part in this research. This research is part of the Sea Hero Quest initiative funded and supported by Deutsche Telekom. Alzheimer’s Research UK (ARUK-DT2016-1) funded the analysis; Glitchers designed and produced the game; and Saatchi and Saatchi London managed its creation.
Disclosure statement
No potential conflict of interest was reported by the author(s).
Data availability statement
A dataset containing the preprocessed trajectory lengths and demographic information is available at https://osf.io/cugdw/. We also set up a portal - https://seaheroquest.alzheimersresearchuk.org/ - where researchers can invite a targeted group of participants to play SHQ and generate data about their spatial navigation capabilities. Those invited to play the game will be sent a unique participant key, generated by the SHQ system according to the criteria and requirements of a specific project decided by the experimenter. Access to the portal will be granted for noncommercial purposes. Future publications based on this dataset should add “Sea Hero Quest Project” as a coauthor.
Supplementary material
Supplemental data for this article can be accessed online at https://doi.org/10.1080/13875868.2024.2319735
Additional information
Funding
References
- Andrews, E. C., & Westerman, S. J. (2012). Age differences in simulated driving performance: Compensatory processes. Accident Analysis & Prevention, 45, 660–668. https://doi.org/10.1016/j.aap.2011.09.047
- Barco, P. P., Wallendorf, M. J., Snellgrove, C. A., Ott, B. R., & Carr, D. B. (2014). Predicting road test performance in drivers with stroke. The American Journal of Occupational Therapy, 68(2), 221–229. https://doi.org/10.5014/ajot.2014.008938
- Barrash, J., Stillman, A., Anderson, S. W., Uc, E. Y., Dawson, J. D., & Rizzo, M. (2010). Prediction of driving ability with neuropsychological tests: Demographic adjustments diminish accuracy. Journal of the International Neuropsychological Society, 16(4), 679–686. https://doi.org/10.1017/s1355617710000470
- Ben-Elia, E. (2021). An exploratory real-world wayfinding experiment: A comparison of drivers’ spatial learning with a paper map vs. turn-by-turn audiovisual route guidance. Transportation Research Interdisciplinary Perspectives, 9, 100280. https://doi.org/10.1016/j.trip.2020.100280
- Brown, T. I., Ross, R. S., Keller, J. B., Hasselmo, M. E., & Stern, C. E. (2010). Which way was I going? Contextual retrieval supports the disambiguation of well learned overlapping navigational routes. The Journal of Neuroscience, 30(21), 7414–7422. https://doi.org/10.1523/jneurosci.6021-09.2010
- Burles, F., & Iaria, G. (2020). Behavioural and cognitive mechanisms of developmental topographical disorientation. Scientific Reports, 10(1). https://doi.org/10.1038/s41598-020-77759-8
- Carr, D. B., Barco, P. P., Wallendorf, M. J., Snellgrove, C. A., & Ott, B. R. (2011). Predicting road test performance in drivers with dementia. Journal of the American Geriatrics Society, 59(11), 2112–2117. https://doi.org/10.1111/j.1532-5415.2011.03657.x
- Choi, M., Lohman, M. C., & Mezuk, B. (2013). Trajectories of cognitive decline by driving mobility: Evidence from the health and retirement study. International Journal of Geriatric Psychiatry, 29(5), 447–453. https://doi.org/10.1002/gps.4024
- Coughlan, G., Coutrot, A., Khondoker, M., Minihane, A.-M., Spiers, H., & Hornberger, M. (2019). Toward personalized cognitive diagnostics of at-genetic-risk Alzheimer’s disease. Proceedings of the National Academy of Sciences, 116(19), 9285–9292. https://doi.org/10.1073/pnas.1901600116
- Coughlan, G., Laczó, J., Hort, J., Minihane, A.-M., & Hornberger, M. (2018). Spatial navigation deficits — overlooked cognitive marker for preclinical Alzheimer disease? Nature Reviews Neurology, 14(8), 496–506. https://doi.org/10.1038/s41582-018-0031-x
- Coughlan, G., Puthusseryppady, V., Lowry, E., Gillings, R., Spiers, H., Minihane, A.-M., Hornberger, M., & Ginsberg, S. D. (2020). Test-retest reliability of spatial navigation in adults at-risk of Alzheimer’s disease. Public Library of Science ONE, 15(9), e0239077. https://doi.org/10.1371/journal.pone.0239077
- Coutrot, A., Manley, E., Goodroe, S., Gahnstrom, C., Filomena, G., Yesiltepe, D., Dalton, R. C., Wiener, J. M., Hölscher, C., Hornberger, M., & Spiers, H. J. (2022). Entropy of city street networks linked to future spatial navigation ability. Nature, 604(7904), 104–110. https://doi.org/10.1038/s41586-022-04486-7
- Coutrot, A., Schmidt, S., Coutrot, L., Pittman, J., Hong, L., Wiener, J. M., Hölscher, C., Dalton, R. C., Hornberger, M., Spiers, H. J., & Zamarian, L. (2019). Virtual navigation tested on a mobile app is predictive of real-world wayfinding navigation performance. Public Library of Science ONE, 14(3), e0213272. https://doi.org/10.1371/journal.pone.0213272
- Coutrot, A., Silva, R., Manley, E., de Cothi, W., Sami, S., Bohbot, V. D., Wiener, J. M., Hölscher, C., Dalton, R. C., Hornberger, M., & Spiers, H. J. (2018). Global determinants of navigation ability. Current Biology, 28(17), 2861–2866.e4. https://doi.org/10.1016/j.cub.2018.06.009
- Crizzle, A. M., Classen, S., Bédard, M., Lanford, D., & Winter, S. (2012). MMSE as a predictor of on-road driving performance in community dwelling older drivers. Accident Analysis & Prevention, 49, 287–292. https://doi.org/10.1016/j.aap.2012.02.003
- Curran, P. G. (2016). Methods for the detection of carelessly invalid responses in survey data. Journal of Experimental Social Psychology, 66, 4–19. https://doi.org/10.1016/j.jesp.2015.07.006
- Dahmani, L., & Bohbot, V. D. (2020). Habitual use of GPS negatively impacts spatial memory during self-guided navigation. Scientific Reports, 10(1). https://doi.org/10.1038/s41598-020-62877-0
- Difonzo, T., Martini, M., Zago, S., Gramegna, C., Guarino, M., & Stracciari, A. (2022). Relationship between self-perceived driving ability and neuropsychological performance in neurological and psychiatric patients. Neurological Sciences, 43(6), 3595–3601. https://doi.org/10.1007/s10072-021-05858-z
- DiMeco, A., Bennett, J. M., Batchelor, J., Chekaluk, E., Andrews, E., & Habib, J. (2021). The role of cognition for identifying unsafe young drivers. Safety Science, 138, 105099. https://doi.org/10.1016/j.ssci.2020.105099
- Duchek, J. M., Carr, D. B., Hunt, L., Roe, C. M., Xiong, C., Shah, K., & Morris, J. C. (2003). Longitudinal driving performance in early-stage dementia of the Alzheimer type. Journal of the American Geriatrics Society, 51(10), 1342–1347. https://doi.org/10.1046/j.1532-5415.2003.51481.x
- Edwards, J. D., Ross, L. A., Ackerman, M. L., Small, B. J., Ball, K. K., Bradley, S., & Dodson, J. E. (2008). Longitudinal predictors of driving cessation among older adults from the ACTIVE clinical trial. The Journals of Gerontology: Series B, 63(1), 6–P12. https://doi.org/10.1093/geronb/63.1.P6
- Ekstrom, A. D., Spiers, H. J., Bohbot, V. D., & Rosenbaum, R. S. (2018). Human spatial navigation. Princeton University Press.
- Erdfelder, E., Faul, F., & Buchner, A. (1996). GPOWER: A general power analysis program. Behavior Research Methods, Instruments, & Computers, 28(1), 1–11. https://doi.org/10.3758/bf03203630
- Everson-Rose, S. A. (2003). Early life conditions and cognitive functioning in later life. American Journal of Epidemiology, 158(11), 1083–1089. https://doi.org/10.1093/aje/kwg263
- Fox, J., & Monette, G. (1992). Generalized collinearity diagnostics. Journal of the American Statistical Association, 87(417), 178–183. https://doi.org/10.1080/01621459.1992.10475190
- Garg, T., Velasco, P. F., Patai, Z. E., Malcolm, C. P., Kovalets, V., Bohbot, V. D., Coutrot, A., Hegarty, M., Hornberger, M., & Spiers, H. J. (2023). The relationship between object-based spatial ability and virtual navigation performance. [preprint]. bioRxiv. https://doi.org/10.1101/2023.03.16.532962
- He, Q., Beveridge, E. H., Starnes, J., Goodroe, S. C., & Brown, T. I. (2021). Environmental overlap and individual encoding strategy modulate memory interference in spatial navigation. Cognition, 207, 104508. https://doi.org/10.1016/j.cognition.2020.104508
- Hegarty, M., Montello, D. R., Richardson, A. E., Ishikawa, T., & Lovelace, K. (2006). Spatial abilities at different scales: Individual differences in aptitude-test performance and spatial-layout learning. Intelligence, 34(2), 151–176. https://doi.org/10.1016/j.intell.2005.09.005
- Hill, P. F., McAvan, A. S., Garren, J. D., Grilli, M. D., Barnes, C. A., & Ekstrom, A. D. (2023). Age differences in spatial memory are mitigated during naturalistic navigation. bioRxiv. https://doi.org/10.1101/2023.01.23.525279
- Howard, L. R., Javadi, A., Yu, Y., Mill, R. D., Morrison, L. C., Knight, R., Loftus, M. M., Staskute, L., & Spiers, H. J. (2014). The hippocampus and entorhinal cortex encode the path and euclidean distances to goals during navigation. Current Biology, 24(12), 1331–1340. https://doi.org/10.1016/j.cub.2014.05.001
- Jabbari, Y., Kenney, D. M., von Mohrenschildt, M., & Shedden, J. M. (2022). Testing landmark-specific effects on route navigation in an ecologically valid setting: A simulated driving study. Cognitive Research: Principles and Implications, 7(1). https://doi.org/10.1186/s41235-022-00374-w
- Javadi, A.-H., Patai, E. Z., Marin-Garcia, E., Margolis, A., Tan, H.-R. M., Kumaran, D., Nardini, M., Penny, W., Duzel, E., Dayan, P., & Spiers, H. J. (2019). Prefrontal Dynamics Associated with efficient detours and shortcuts: A combined functional magnetic resonance imaging and magnetoencenphalography study. Journal of Cognitive Neuroscience, 31(8), 1227–1247. https://doi.org/10.1162/jocn_a_01414
- Kim, J. H. (2019). Multicollinearity and misleading statistical results. Korean Journal of Anesthesiology, 72(6), 558–569. https://doi.org/10.4097/kja.19087
- Kunishige, M., Miyaguchi, H., Fukuda, H., Iida, T., Nami, K., & Ishizuki, C. (2020). Spatial navigation ability is associated with the assessment of smoothness of driving during changing lanes in older drivers. Journal of Physiological Anthropology, 39(1). https://doi.org/10.1186/s40101-020-00227-9
- Ledger, S., Bennett, J. M., Chekaluk, E., Batchelor, J., & Meco, A. D. (2019). Cognitive function and driving in middle adulthood: Does age matter? Transportation Research Part F: Traffic Psychology and Behaviour, 66, 471–484. https://doi.org/10.1016/j.trf.2019.10.002
- Lee, H., & Schafer, M. (2020). Are positive childhood experiences linked to Better Cognitive Functioning in later life?: Examining the role of life course pathways. Journal of Aging and Health, 33(3–4), 217–226. https://doi.org/10.1177/0898264320972547
- Moran, C., Bennett, J. M., & Prabhakharan, P. (2020). The relationship between cognitive function and hazard perception in younger drivers. Transportation Research Part F: Traffic Psychology and Behaviour, 74, 104–119. https://doi.org/10.1016/j.trf.2020.08.008
- Munion, A. K., Stefanucci, J. K., Rovira, E., Squire, P., & Hendricks, M. (2019). Gender differences in spatial navigation: Characterizing wayfinding behaviors. Psychonomic Bulletin & Review, 26(6), 1933–1940. https://doi.org/10.3758/s13423-019-01659-w
- Newcombe, N. S., Hegarty, M., & Uttal, D. (2022). Building a cognitive science of human variation: Individual Differences in Spatial Navigation. Topics in Cognitive Science, 15(1), 6–14. https://doi.org/10.1111/tops.12626
- Ott, B. R., Heindel, W. C., Papandonatos, G. D., Festa, E. K., Davis, J. D., Daiello, L. A., & Morris, J. C. (2008). A longitudinal study of drivers with Alzheimer disease. Neurology, 70(14), 1171–1178. https://doi.org/10.1212/01.wnl.0000294469.27156.30
- Patai, E. Z., Javadi, A.-H., Ozubko, J. D., O’Callaghan, A., Ji, S., Robin, J., Grady, C., Winocur, G., Rosenbaum, R. S., Moscovitch, M., & Spiers, H. J. (2019). Hippocampal and retrosplenial goal distance coding after long-term consolidation of a Real-World Environment. Cerebral Cortex, 29(6), 2748–2758. https://doi.org/10.1093/cercor/bhz044
- Patai, E. Z., & Spiers, H. J. (2021). The versatile Wayfinder: Prefrontal contributions to spatial navigation. Trends in Cognitive Sciences, 25(6), 520–533. https://doi.org/10.1016/j.tics.2021.02.010
- Roe, C. M., Babulal, G. M., Head, D., Stout, S. H., Vernon, E. K., Ghoshal, N., Garland, B., Barco, P. P., Williams, M., Johnson, A. L., Fierberg, R., Fague, M. S., Xiong, C., Mormino, E. C., Grant, E., Holtzman, D. M., Benzinger, T. L. S., Fagan, A. M., Ott, B. R., & Carr, D. (2016). Preclinical Alzheimer’s disease and longitudinal driving decline. Alzheimers & Dementia: Translational Research & Clinical Interventions, 3(1), 74–82. https://doi.org/10.1016/j.trci.2016.11.006
- Sahlqvist, S., Song, Y., & Ogilvie, D. (2012). Is active travel associated with greater physical activity? The contribution of commuting and non-commuting active travel to total physical activity in adults. Preventive medicine, 55(3), 206–211. https://doi.org/10.1016/j.ypmed.2012.06.028
- Schäfer, T., & Schwarz, M. A. (2019). The meaningfulness of effect sizes in psychological research: Differences between sub-disciplines and the impact of potential biases. Frontiers in Psychology, [Online], 10, 10. https://doi.org/10.3389/fpsyg.2019.00813
- Selya, A. S., Rose, J. S., Dierker, L. C., Hedeker, D., & Mermelstein, R. J. (2012). A practical Guide to calculating Cohen’s f2, a measure of local effect size, from PROC MIXED. Frontiers in Psychology, 3, 3. https://doi.org/10.3389/fpsyg.2012.00111
- Seton, C., Coutrot, A., Hornberger, M., Spiers, H. J., Knight, R., Whyatt, C., & Cristofori, I. (2023). Wayfinding and path integration deficits detected using a virtual reality mobile app in patients with traumatic brain injury. Public Library of Science ONE, 18(3), e0282255–e0282255. https://doi.org/10.1371/journal.pone.0282255
- Spiers, H. J., Coutrot, A., & Hornberger, M. (2023). Explaining world‐Wide variation in navigation ability from millions of people: Citizen science project sea hero quest. Topics in Cognitive Science, 15(1), 120–138. https://doi.org/10.1111/tops.12590
- Stojan, R., & Voelcker-Rehage, C. (2021). Neurophysiological correlates of age differences in driving behavior during concurrent subtask performance. Neuroimage: Reports, 225, 117492. https://doi.org/10.1016/j.neuroimage.2020.117492
- Tinella, L., Caffò, A. O., Lopez, A., Nardulli, F., Grattagliano, I., & Bosco, A. (2021a). Reassessing fitness-to-drive in drinker drivers: The role of cognition and personality. International Journal of Environmental Research and Public Health, 18(23), 12828–12828. https://doi.org/10.3390/ijerph182312828
- Tinella, L., Lopez, A., Caffò, A. O., Grattagliano, I., & Bosco, A. (2020). Spatial Mental Transformation Skills Discriminate Fitness to drive in young and old adults. Frontiers in Psychology, 11, 11. https://doi.org/10.3389/fpsyg.2020.604762
- Tinella, L., Lopez, A., Caffò, A. O., Nardulli, F., Grattagliano, I., & Bosco, A. (2021b). Cognitive efficiency and fitness-to-drive along the lifespan: The mediation effect of visuospatial transformations. Brain Sciences, 11(8), 1028. https://doi.org/10.3390/brainsci11081028
- Uc, E. Y., Rizzo, M., O’Shea, A. M. J., Anderson, S. W., & Dawson, J. D. (2017). Longitudinal decline of driving safety in parkinson disease. Neurology, 89(19), 1951–1958. https://doi.org/10.1212/wnl.0000000000004629
- van der Ham, I. J. M., Koutzmpi, V., van der Kuil, M. N. A., & van der Hiele, K. (2022). Spatial navigation performance in people with multiple sclerosis-a large-scale online study. Multiple Sclerosis and Related Disorders, 58, 103423. https://doi.org/10.1016/j.msard.2021.103423
- Wang, Y. C., Foss, R. D., Goodwin, A. H., Curry, A. E., & Tefft, B. C. (2020, May 21). The effect of extending graduated Driver licensing to older novice drivers in Indiana. Journal of Safety Research, 74, 103–108. https://doi.org/10.1016/j.jsr.2020.04.001
- Wolbers, T., & Hegarty, M.(2010). What determines our navigational abilities? Trends in Cognitive Sciences, 14(3), 138–146. https://doi.org/10.1016/j.tics.2010.01.001
- Yavuz, E., He, C., Goodroe, S., Ganstrom, C., Coutrot, A., Hornberger, M., Hegarty, M., & Spiers, H. J. (2023a). Video gaming, but not reliance on GPS, is associated with spatial navigation performance. [preprint]. bioRxiv. https://doi.org/10.1101/2023.08.10.552365
- Yavuz, E., Lazar, A., Goodroe, S., Ganstrom, C., Coutrot, A., Hornberger, M., & Spiers, H. J. (2023b). Shorter reported sleep duration in men is associated with worse virtual spatial navigation performance. [preprint]. bioRxiv. https://doi.org/10.1101/2023.09.09.556942
- Yesiltepe, D., Velasco, P., Coutrot, A., Ozbil Torun, A., Wiener, J., Hölscher, C., Hornberger, M., Dalton, R., & Spiers, H. J.(2023). Entropy and a sub-group of geometric measures of paths predict the navigability of an environment. Cognition, 236, 105443–105443. https://doi.org/10.1016/j.cognition.2023.105443
- Yu, Z., Qu, W., & Ge, Y. (2022). Trait anger causes risky driving behavior by influencing executive function and hazard cognition. Accident Analysis & Prevention, 177, 106824. https://doi.org/10.1016/j.aap.2022.106824
- Zijlstra, W. P., van der Ark, L. A., & Sijtsma, K. (2011). Outliers in questionnaire data. Journal of Educational and Behavioral Statistics, 36(2), 186–212. https://doi.org/10.3102/1076998610366263