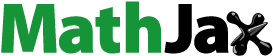
ABSTRACT
We evaluate whether cumulative photosynthesis (An,C) could predict the time of harvest in strawberry better than light (IC) or temperature (Ta,C) as forecast indices. We monitored the daily dynamics of flowering, fruit set, and harvest in over 2,700 fruits across the growing season in a greenhouse in Japan. Photosynthesis was calculated using a biochemical model, and An,C calculated by totalling the cumulative rate from flowering to harvest in each fruit. IC and Ta,C were calculated by totalling daily cumulative light intensity and average temperature. A new index (D) was defined as the normalised variation of An,C, IC, and Ta,C, and a statistical analysis was conducted on the means for each index. The results showed that An,C had the lowest D (0.114), suggesting that it was a better index for predicting the time of harvest. This study suggests the importance of considering the accumulation of photosynthates in individual fruit, which cannot be achieved by IC or Ta,C, these results can be used to improve the forecasting and optimisation of yield. However, further research is required to substantiate these claims.
Introduction
The strawberry (Fragaria × ananassa Duch.) is widely cultivated in temperate, subtropical, and Mediterranean climates (Menzel, Citation2021). Precise data on the number of days from flowering to harvest (HD) are crucial to optimising yield and crop agronomy. Understanding the factors that determine HD is essential for efficient strawberry production, particularly predicting the timing of harvest.
Environmental factors affect strawberry flowering, fruit set, and harvesting (Sønsteby et al., Citation2016; Twitchen et al., Citation2021). Light intensity is especially important (Cervantes et al., Citation2019; Konsin et al., Citation2001; Wang et al., Citation2020). Hidaka et al. (Citation2013) reported that supplemental lighting using a high irradiance light-emitting diode for 12 h increased fruit weight by 8 g and the number of fruits per plant 1.5-fold. Growth is also affected by temperature (Ariza et al., Citation2015; Cui et al., Citation2021; Sønsteby et al., Citation2016). Ledesma et al. (Citation2008) found that high temperatures (30°C/25°C) during fruit growth shortened the time to harvest by 7 days and reduced fruit set by 20%. The responses of growth to the environment have been investigated on a daily to weekly basis using 10 to 60 plants with a 1 to 7-month cultivation period. However, daily longitudinal data on HD are necessary to model the timing of flowering, fruit set, and harvesting.
Cumulative light intensity and temperatures from flowering are conventionally used to model the time of harvest in strawberry (Kawanobu et al., Citation2010; Krüger et al., Citation2012; Opstad et al., Citation2011). Reproductive growth is also related to photosynthesis. Hidaka et al. (Citation2019) suggested a close relationship between fruit enlargement and the translocation of photosynthates. Nakai et al. (Citation2022) reported a role for translocation and cumulative leaf photosynthesis. Photosynthesis is affected by light, temperature, and CO2 concentration, and eco-physiological functions (Farquhar et al., Citation1980). Thus, cumulative photosynthesis from flowering may predict the time of harvest better than cumulative light intensity and temperature.
We tested whether leaf photosynthesis could describe strawberry HD better than conventional indices. We monitored the daily dynamics of flowering, fruit set, and harvesting in 144 plants (>2,700 fruits) over 9 months. The growth dynamics of each inflorescence and fruit position was monitored. We estimated single-leaf photosynthesis using a biochemical model. We statistically compared the use of cumulative leaf photosynthesis, light intensity, and temperature to predict the time of harvest.
Materials and methods
Plant materials and growth conditions
June-bearing strawberry plants (Fragaria × ananassa Duch. cv. Fukuoka S6) were used. The nursery plants were grown in a greenhouse at the National Agriculture and Food Research Organization, Kyushu Okinawa Agricultural Research Center, Japan. On 6 June 2019, nursery plants were planted in plastic pots filled with peat moss, coconut shell, and charcoal [3:5:2]. A nutrient solution (OK F-1; OAT Agrio Co., Ltd., Tokyo, Japan; electrical conductivity, 0.6 dS m−1) was supplied at 300 mL d−1 per plant at 9:00, 11:00, 13:00, 15:00, and 17:00. Nutrient supplementation was stopped between mid-August and mid-September to induce anthesis. Other growth conditions were according to Hidaka et al. (Citation2019). On 3 October 2019, 288 plants were transplanted to beds filled with culture soil (Good Soil; Kaneya Co., Ltd., Aichi Japan) in a greenhouse at the Ito Plant Experiment Fields & Facilities, Faculty of Agriculture, Kyushu University, Fukuoka, Japan (Fig. S1) and spaced at 20 cm intervals with an inter-row spacing of 15 cm. The plants were supplied with a nutrient solution at 200–300 mL d−1 per plant at 1 h intervals between 06:00 and 18:00. The temperature inside the greenhouse was controlled at 8°C to 22°C via an oil heater (HK2027TEV; NEPON Inc., Tokyo, Japan) in winter and a ventilation system with roof and side-wall windows and shade in summer. To prevent excessively high temperature and drying, a fine mist cooling system (mist nozzle diameter, 0.15 mm; dynamic injection pump flow rate, 1.5 L min−1; Green.com Inc., Tokyo, Japan) was operated for 2 min at 15 min intervals from 10:00 to 16:00 on sunny days. The entire cultivation period of the strawberry plants was from 3 October 2019 to 30 June 2020. Flowers were hand-pollinated every morning and the number of leaves and fruit in each inflorescence were managed at about eight and seven, respectively. The inflorescences held by each plant at any one time were limited to one. Damaged or diseased fruit and runners were discarded. In total, 144 of the original 288 plants were used.
Measurement of environmental conditions
Photosynthetic photon flux density (PPFD), air temperature, relative humidity, CO2 concentration, and wind speed inside the greenhouse were measured at a height of 1.2 m. The sensors included a quantum sensor (PAR-02D; Prede Co., Ltd., Tokyo, Japan), a temperature/humidity sensor (HMP60; Vaisala, Helsinki, Finland), an infrared CO2-gas analyser (GMP252; Vaisala, Helsinki, Finland), and an ultrasonic anemometer (model 81000; R.M Young Company, Traverse City, Michigan, USA). Data were measured every 5 seconds and 5 minutes means logged in a programmable data logger (CR-1000; Campbell Scientific Inc., Logan, Utah, USA).
Simulation of the single-leaf photosynthesis
We simulated single-leaf photosynthesis (A) using a biochemical model (Farquhar et al., Citation1980), a stomatal model (Medlyn et al., Citation2011), and an energy balance equation (Appendix A1 and A2).
To estimate A with an ever-changing leaf temperature (Tleaf), we followed Nakai et al. (Citation2022). Firstly, we set the arbitrary stomatal conductance () as the initial value and estimated Tleaf using the ‘tleaf’ in the ‘tealeaves’ package (version 1.0.5; Muir, Citation2019) in R (version 4.0.2; R Development Core Team, Vienna, Austria). This allows users to set leaf and environmental parameters, as well as physical constants. For the environment and leaf parameters, we used the measured environmental condition and a leaf characteristic dimension of 0.064 m (Kimura et al., Citation2020), respectively. We used the default values for the other parameters. Seco
ndly, we obtained the dark respiration rate (Rn; in Eqs. A5, A6) using the night respiration rate at 25°C (Rn,25) and the activation energy for Rn [Ea(Rn) in Eq. A7], and the estimated Tleaf. Thirdly, we estimated A using the ‘Photosyn’ in the ‘plantecophys’ package (version 1.4.6; Duursma, Citation2015) with the estimated Tleaf and Rn values. We determined the maximum carboxylation rate at 25°C (Vcmax,25) and the maximum electron transport rate at 25°C (Jmax,25; in Eqs. A2, A4, A8). Other model parameters related to temperature dependence such as the activation energy for Vcmax [Ea(Vcmax)] and Jmax [Ea(Jmax)], entropy factor for Vcmax [ΔS(Vcmax)] and Jmax [ΔS(Jmax)], and deactivation energy for Vcmax [Hd(Vcmax)] and Jmax [Hd(Jmax)] were obtained from Kimura et al. (Citation2020), who took measurements in real cultivation settings using the same cultivar (Table A.1; in Eq. A8). We used measured PPFD, temperature, relative humidity, CO2 concentration, and wind speed for the microclimate parameters, while default values were used for the remaining parameters. Fourthly, we estimated stomatal conductance () using the stomatal model with the estimated A value, identified the residual stomatal conductance (
) and the empirical constant of stomatal conductance (
; in Eq. A9), and measured environmental conditions. This process was repeated until the difference between
and
was minimal. Finally, the obtained
was used as the next
, and A was continuously estimated throughout the experiment.
Acquisition of reproductive growth data
All the flowers were tagged daily, and the dates of flowering, fruit set, and harvest recorded. The growth stage of the fruit was determined based on specific characteristics (). Flowering was defined when the petals were fully unfolded and the receptacle and all anthers were visible. Fruit set was defined as the falling of petals and the expansion of the achene within the receptacle. Harvest was defined as the fruit surface evenly red. The number of flowerings (FLt), fruit sets (FRt), and harvests (Ht) per day and plant were determined by dividing the daily values by the total number of plants (144). The number of flowers (NFLt), fruit (NFRt), and cumulative harvests (NHt) per day and plant were calculated using the following:
Figure 1. Schematic diagram of the reproductive growth process (flowering, fruit set, and harvesting) in strawberry plants. Flowering – fruit set and fruit set – harvesting were defined as flower and fruit, respectively. The number of days from flowering to harvesting was defined as “HD.

where t is any given day after planting (d).
Statistical analysis
To analyse changes in microclimate parameters and leaf photosynthesis, daily cumulative PPFD (I), daily averaged temperature (Ta), daily averaged CO2 concentration (Ca), and daily cumulative single-leaf photosynthesis (An) were calculated. Average and cumulative values during the fruit growing period for light, temperature, and leaf photosynthesis were calculated using the averaged and cumulative I, Ta, and An from flowering to harvest denoted as IA, Ta,A, An,A and IC, Ta,C, An,C, respectively. Significant differences in the parameters between each fruit position were determined using a Steel – Dwass test in R. The root mean square error (RMSE) was used to evaluate the accuracy of simulating A.
A suitable analysis method was needed to evaluate and compare the appropriateness of each index. Multiple regression analysis is used to compare the influence of each index (Doan & Tanaka, Citation2022), but it was not suitable for this study since the aim was not to investigate the degree of influence. It was better to compare the coefficient of variation that represents variations in IC, Ta,C, and An,C. However, there was only one sample for each index, so statistical comparison was not possible. Therefore, the determining degree (D) was newly defined here as a dimensionless quantity and calculated using the following equation:
where xi is the ith data point, i.e. IC, Ta,C, or An,C of the ith fruit, and is the mean value for each index. The index with the smallest average D has the smallest variance, i.e. the highest appropriateness. Significant differences in D between the indices were determined using the Steel – Dwass test in R.
Results
Microclimate and net photosynthesis
I gradually decreased from 0 to 90 days and then increased to 230 days, after which I decreased because the shade curtains were activated (). Ta changed with I: it gradually decreased from 0 to 50 days as I decreased but was constant at 13°C from 50 to 200 days (). Thereafter, Ta increased as I increased. Ca increased from 0 to 80 days and then decreased ().
Figure 2. Changes in daily cumulative photosynthetic photon flux density I (a), daily average temperature Ta (b), and daily average CO2 concentration (c) measured at a fixed point in the centre of a greenhouse throughout the experimental period (October 3, 2019–June 30, 2020).

Estimated A using the photosynthetic and stomatal characteristics estimated from gas exchange measurements showed reasonable values (; RMSE = 2.00 μmol m−2 s−1). An changed seasonally, with large fluctuations in response to change in I (). On sunny days, An gradually decreased from 0 to 90 days, increased from 90 to 230 days, and then decreased to 200 mmol m−2 d−1.
Figure 3. Relationship between the estimated and measured leaf net photosynthetic rate (a) on May 22 and October 28, 2021 and January 12, 2022. The dashed line denotes a one-to-one relationship. In the model simulation, the mean values of the photosynthetic capacities (Vcmax,25 and Jmax,25; n = 5) and stomatal characteristics ( and
) estimated using the gas exchange measurements were used.

Figure 4. Change in daily cumulative leaf net photosynthesis (An) estimated using biochemical photosynthesis model parameters, including the measured maximum carboxylation rate (Vcmax) and maximum electron transport rate (Jmax), and microclimates inside a greenhouse throughout the experimental period (October 3, 2019–June 30, 2020).

Dynamics of reproductive growth
A FLt wave was followed by a FRt wave and a Ht wave (). These three waves occurred three times during the experiment, and these groups were regarded as the first, second, and third inflorescences, respectively. In the first inflorescence, the respective waves were close to Gaussian and occurred 45 to 101, 52 to 108, and 89 to 150 days after planting. The waves of the second inflorescence were skewed to the left compared with the first and occurred at 108 to 180, 119 to 195, and 157 to 213 days, respectively. The waves of the third inflorescence occurred at 183 to 249, 189 to 255, and 215 to 270 days, respectively, and took the form of Gaussian distributions. The maximum values of these waves were smaller, and the variation increased compared with those of the first inflorescence. Waves of NFLt, NFRt, and NHt were observed periodically (). In the first inflorescence, maximum values for NFLt (2.1), NFRt (6.6), and NHt (6.7) were recorded of the 70, 92, and 150 days, respectively. The maximum NFLt was smaller than the maximum NFRt because the number of days from flowering to fruit set was shorter than that from fruit set to harvest, and fruit set started before all flowers had opened. In the second inflorescence, maximum values for NFLt (1.8), NFRt (6.1), and NHt (6.4) were recorded of the 161, 175, and 213 days. In the third inflorescence, maximum values for NFLt (1.0), NFRt (4.4), and NHt (6.1) were recorded of the 217, 232, and 270 days, respectively.
Figure 5. Daily dynamics of the rate of flowering (FLt; yellow circle), fruit set (FRt; blue circle), and harvesting (Ht; purple circle) (A), and the daily dynamics of the number of flowers (NFLt; yellow circle), fruits (NFRt; blue circle), and harvests (NHt; purple circle) in each inflorescence (B) per plant throughout the cultivation period (October 3, 2019–June 30, 2020). The number of flowers, number of fruits, and cumulative harvest at each date was estimated using Eqs. (1)–(3).

Days to harvesting, light, temperature, and photosynthesis for each inflorescence and fruit position
The mean HD in the first, second, and third inflorescences was 51.75, 34.91, and 23.2 days, respectively Thus, HD shortened with as growth progressed (). The mean HD changed among the primary, secondary, and tertiary fruit positions in the first (48.7, 50.8, and 53.0 days, respectively), second (40.1, 35.7, and 33.3 days, respectively), and third (22.8, 22.9, and 23.5 days, respectively) inflorescence. However, the distribution of HD in the primary and secondary fruit positions in the second inflorescence was wider than that in the other inflorescences. IA increased from 6.2 to 16.4 mol m−2 d−1 as growth progressed (). Although the mean IA changed among the primary, secondary, and tertiary fruit positions in the first (6.3, 6.1, and 6.2 mol m−2 d−1, respectively) and second inflorescence (12.6, 13.0, and 13.4 mol m−2 d−1, respectively), no significant difference was detected in third inflorescence (16.6, 16.6, and 16.2 mol m−2 d−1, respectively). Ta,A of each fruit in the first, second, and third inflorescence increased as IA increased (13.2°C, 16.0°C, and 21.1°C, respectively; ). The mean Ta,A also changed among the fruit positions in the first (13.3°C, 13.1°C, and 13.2°C, respectively), second (15.6°C, 15.9°C, and 16.2°C, respectively), and third (20.2°C, 20.7°C, and 21.5°C, respectively) inflorescence. IC increased in the order of the second, third, and first inflorescence, and the mean values were 458.9, 380.6, and 322.0 mol m−2, respectively (). Although the mean IC changed among the fruit positions in the first (306.1, 311.8, and 331.5 mol m−2, respectively) and second inflorescence (500.1, 462.7, and 447.4 mol m−2, respectively), no difference was detected in the third inflorescence (378.5, 380.2, and 381.4 mol m−2, respectively). The IC distribution in the primary and secondary fruit position in the second inflorescence was wider than that in the other inflorescences. Unlike IC, Ta,C decreased as growth progressed, and the mean values in the first, second, and third inflorescence were 682.8, 557.9, and 488.0°C d, respectively (). The mean Ta,C also changed among the fruit positions in the first (645.5, 665.8, and 701.4°C d, respectively), second (619.9, 564.3, and 540.3°C d, respectively), and third (458.5, 474.4, and 503.8°C d, respectively) inflorescence. Similar to IC, the distribution of Ta,C in the primary and secondary fruit position in the second inflorescence was wider than that in the others.
Figure 6. Days to harvest (HD) in each inflorescence and each fruit position. In the boxplots, the boxes indicate the 25th percentile to the 75th percentile, and the median is shown by a line within the box. The whiskers extend to 1.5 times the interquartile range from the 25th and 75th percentiles. The density curve is shown on the right side of each boxplot. Mean values are represented by white circles. Different letters indicate significant differences (p < 0.01) as determined by a Steel–Dwass test.

Figure 7. Average and cumulative values of daily cumulative PPFD and daily average temperature from flowering to harvesting in each inflorescence and each fruit position (IA, IC, Ta,A, and Ta,C, respectively). In the boxplots, the boxes indicate the 25th percentile to the 75th percentile, and the median is shown by a line within the box. The whiskers extend to 1.5 times the interquartile range from the 25th and 75th percentiles. Mean values are represented by white circles. Mean values are represented by white circles. The density curve is shown on the right side of each boxplot. Different letters indicate significant differences (p < 0.01) as determined by a Steel–Dwass test.

An,A increased from 196.0 to 356.5 mmol m−2 d−1 as growth progressed, and the same trend was detected for IA and Ta,A (). In contrast, mean An,A in the fruit position was similar within the first (198.0, 195.1, and 195.9 mmol m−2 d−1, respectively), second (304.9, 313.1, and 323.4 mmol m−2 d−1, respectively), and third (358.7, 358.6, and 354.7 mmol m−2 d−1, respectively) inflorescence. An,C was stable among different inflorescences and fruit, with means values of 10.14, 11.05, and 8.28 kmol m−2 for the first, second, and third inflorescences respectively (). Similar to IC and Ta,C (), the distribution of An,C in the primary and secondary fruit position in the second inflorescence was wider than that in the others.
Figure 8. Average and cumulative values of the daily integral of leaf photosynthesis from flowering to harvesting per fruit per inflorescence (An,A and An,C, respectively). In the boxplots, the boxes indicate the 25th percentile to the 75th percentile, and the median is shown by a line within the box. The whiskers extend to 1.5 times the interquartile range from the 25th and 75th percentiles. Mean values are represented by white circles. The density curve is shown on the right side of each boxplot. Mean values are represented by white circles. Different letters indicate significant differences (p < 0.01) as determined by a Steel–Dwass test.

Comparison of determining degrees among each index on fruit harvest
The D values of IC, Ta,C, and An,C were 0.149, 0.145, and 0.114, respectively, and the D value of An,C was smaller than that for others (). As shown in the third and fourth rows of panels in and in the second row of panels in , the average values of each index were 386.2 mol m−2, 579.4°C d, and 9.9 kmol m−2, respectively, and the standard deviations were 70.2 mol m−2, 94.8°C d, and 1.5 kmol m−2, respectively.
Figure 9. Determining degree (D) among each predictive index [i.e. cumulative values of daily integral of PPFD, daily average of temperature, and daily integral of leaf photosynthesis (IC, Ta,C, and An,C, respectively)] in all inflorescences. In the boxplots, the boxes indicate the 25th percentile to the 75th percentile, and the median is shown by a line within the box. The whiskers extend to 1.5 times the interquartile range from the 25th and 75th percentiles. Mean values are represented by circles. The density curve is shown on the right side of each boxplot. Different letters indicate significant differences (p < 0.001) as determined by a Steel–Dwass test.
![Figure 9. Determining degree (D) among each predictive index [i.e. cumulative values of daily integral of PPFD, daily average of temperature, and daily integral of leaf photosynthesis (IC, Ta,C, and An,C, respectively)] in all inflorescences. In the boxplots, the boxes indicate the 25th percentile to the 75th percentile, and the median is shown by a line within the box. The whiskers extend to 1.5 times the interquartile range from the 25th and 75th percentiles. Mean values are represented by circles. The density curve is shown on the right side of each boxplot. Different letters indicate significant differences (p < 0.001) as determined by a Steel–Dwass test.](/cms/asset/0fcf02f8-b6fa-483f-9329-cbee4155fd9d/thsb_a_2254759_f0009_oc.jpg)
Discussion
Relationships of light intensity, air temperature, and leaf photosynthesis with strawberry reproductive growth
In this study, all environmental condition inside the greenhouse, photosynthesis, and the dynamics of flowering, fruit set, and harvesting changed markedly throughout the experimental period (). The increases in IA, Ta,A, and An,A with growth progression accelerated fruit harvest timing (). Although several studies have shown that using such relationships to control the environment inside a greenhouse can effectively promote reproductive growth (Hidaka et al., Citation2014, Citation2016, Citation2022; Yoneda et al., Citation2020), some studies have reported that extremely low or high temperatures adversely affect strawberry reproductive growth (Ariza et al., Citation2015; Ledesma & Kawabata, Citation2016; Ledesma et al., Citation2008). For example, Cui et al. (Citation2021) found that short periods of high (35°C) and low temperatures (2°C) decreased strawberry pollen viability and germination rate, increasing the rate of malformed strawberry fruits. Our results are generally consistent with these studies, i.e. the change in air temperature within the appropriate range reduced HD, whereas an extremely low temperature suppressed reproductive growth (100 to 150 days after planting; ). Thus, the distributions of HD, IC, Ta,C, and An,C in the primary and secondary fruit positions in the second inflorescence were likely wider due to differences in reproductive growth activity.
Photosynthesis is a fundamental biochemical process in crop production (Long et al., Citation2006), and its relationship with reproductive growth has been previously reported (Choi et al., Citation2016; Miyoshi et al., Citation2021). For example, Hidaka et al. (Citation2016) found that the use of supplemental lighting increased the photosynthetic rate, number of flowers per inflorescence, and flowering, and these effects were further increased by elevated CO2 concentration and air temperature. Miyoshi et al. (Citation2021) divided strawberry fruit ripeness into different developmental stages and revealed that white-ripening strawberry fruits, the developmental stage during which fruits begin to expand rapidly and increase greatly in size, had the highest translocation rate as well as the highest accumulation of photosynthates. These studies and our findings suggest that photosynthates also affect strawberry reproductive growth. Additionally, the fruit-bearing history after the second inflorescence varied among plants due to different first inflorescence timings in fruit harvesting. Hence, individual fruit’s photosynthate accumulation offers a more accurate description of harvest timing throughout cultivation, compared to light intensity or air temperature as indices.
Implications of leaf photosynthesis as a predictive index of fruit harvest timing on strawberry production
We examined various predictive HD indices in 2,752 strawberry fruits harvests and found that An,C demonstrated superior accuracy while maintaining simplicity of sampling when compared to cumulative light and temperature, conventional indices of days to harvest. Thus, when An,C reaches a specific value, it would be possible to consistently determine whether strawberry fruits can be harvested. Describing the time from flowering to harvesting is essential in forecasting yield dynamics. Therefore, several studies have reported the cumulative values of light intensity or air temperature from flowering as indices of harvest timing (Krüger et al., Citation2012; Opstad et al., Citation2011). Kawanobu et al. (Citation2010) reported that the strawberry fruits were harvested when cumulative solar irradiance and temperature from flowering to harvesting reached about 400 MJ m−2 d and 630°C d, respectively. Although our study is generally consistent with that of Kawanobu et al. (Citation2010), they did not statistically compare and discuss the suitability of indices in terms of the variability of each cumulative value. Doan and Tanaka (Citation2022) showed that mathematical models including cumulative solar radiation and air temperature could accurately predict the reproductive growth of tomato clusters. However, their study differed from ours because solar radiation was used as an index of photosynthesis, and reproductive growth was not directly evaluated using photosynthesis. Hernandez-Santana et al. (Citation2021) found that leaf turgor pressure was more linked to fruit development stage of olive fruits than photosynthesis. They also emphasised that photosynthesis is crucial in early fruit enlargement, while turgor pressure affects fruit ripeness. However, the cumulative photosynthesis, which can be estimated continuously by models, can be a more useful index for predicting the harvest period than turgor pressure, which is continuously difficult to measure. Therefore, although our study does not necessarily support that photosynthesis drives fruit ripeness, we have demonstrated for the first time that An,C can accurately predict the harvest timing of strawberry fruits as well as or even better than IC and Ta,C.
Recent advancements in image analysis technology (Abd-Elrahman et al., Citation2021; Chen et al., Citation2019) allow consistent monitoring of flowers and fruits in large-scale strawberry production, reducing labour and costs. Additionally, a hybrid ANN model (Kaneko et al., Citation2022) accurately estimates photosynthesis based on microclimates, enabling precise prediction without expensive equipment. This progress will optimise fruit harvest timing and greenhouse management, leading to more efficient strawberry production plans based on photosynthesis and individual plant histories. In conclusion, our study takes the first step towards an accurate index for fruit harvest timing based on photosynthesis.
Limitations of this study
Although this study demonstrated that An,C can be used as an accurate index for strawberry harvest timing, this study has some limitations. Firstly, environmental stress such as strong solar radiation and high temperature, which can reduce the photosynthetic rate of strawberry plants (Yokoyama et al., Citation2023), were not considered in this study (). Therefore, the actual An of stressed plants could be lower than the An value estimated in this study, resulting in overestimation of An,C. To improve the accuracy An,C, An must be recalculated considering the environmental stresses (Niinemets & Keenan, Citation2014). Secondly, the reproductive growth dynamics were examined in strawberries grown under normal CO2 concentrations. However, CO2 enrichment can enhance crop growth, flowering, and yield by promoting photosynthesis (Hidaka et al., Citation2016; Miyoshi et al., Citation2017; Nomura et al., Citation2021). In this study, the dynamics of An showed the same tendency as I throughout the experimental period () because the photosynthesis was largely dependent on light intensity; thus, there was little difference in the D values between IC and An,C. Therefore, the usefulness of An,C as a predictive index should be examined also under CO2 enrichment conditions. Despite these limitations, our study represents progress towards realising accurate forecasting and optimising the reproductive growth dynamics required for efficient strawberry production.
Credit author statement
Shintaro Ono: Conceptualisation, Methodology, Investigation, Writing – original draft. Daisuke Yasutake: Supervision, Conceptualisation, Writing – review & editing, Funding acquisition. Kensuke Kimura: Methodology, Investigation, Supervision, Writing – review & editing. Kengo I: Methodology, Investigation. Yoshiya Teruya: Investigation. Kota Hidaka: Supervision, Writing – review & editing. Gaku Yokoyama: Supervision, Writing – review & editing. Tomoyoshi Hirota: Supervision, Writing – review & editing. Masaharu Kitano: Supervision, Conceptualisation, Writing – review & editing, Funding acquisition. Takashi Okayasu: Project administration. Yukio Ozaki: Project administration.
Supplemental Material
Download MS Word (378.7 KB)Supplemental Material
Download MS Word (34.9 KB)Supplemental Material
Download MS Word (30.5 KB)Disclosure statement
No potential conflict of interest was reported by the authors.
Data availability statement
We make all data except for available in the supplementary materials.
Supplementary material
Supplemental data for this article can be accessed online at https://doi.org/10.1080/14620316.2023.2254759.
Additional information
Funding
References
- Abd-Elrahman, A., Wu, F., Agehara, S., & Britt, K. (2021). Improving strawberry yield prediction by integrating ground-based canopy images in modeling approaches. ISPRS International Journal of Geo-Information, 10(4), 1–16. https://doi.org/10.3390/ijgi10040239
- Ariza, M. T., Soria, C., & Martínez-Ferri, E. (2015). Developmental stages of cultivated strawberry flowers in relation to chilling sensitivity. AoB Plants, 7, 419–431. https://doi.org/10.1093/aobpla/plv012
- Cervantes, L., Ariza, M. T., Gómez-Mora, J. A., Miranda, L., Medina, J. J., Soria, C., & Martínez-Ferri, E. (2019). Light exposure affects fruit quality in different strawberry cultivars under field conditions. Scientia horticulturae, 252, 291–297. https://doi.org/10.1016/j.scienta.2019.03.058
- Chen, Y., Lee, W. S., Gan, H., Peres, N., Fraisse, C., Zhang, Y., & He, Y. (2019). Strawberry yield prediction based on a deep neural network using high-resolution aerial orthoimages. Remote Sensing, 11(13), 1–21. https://doi.org/10.3390/rs11131584
- Choi, H. G., Moon, B. Y., & Kang, N. J. (2016). Correlation between strawberry (Fragaria ananassa Duch.) productivity and photosynthesis-related parameters under various growth conditions. Frontiers in Plant Science, 7, 1–13. https://doi.org/10.3389/fpls.2016.01607
- Cui, M., Pham, M. D., Hwang, H., & Chun, C. (2021). Flower development and fruit malformation in strawberries after short-term exposure to high or low temperature. Scientia horticulturae, 288, 110308. https://doi.org/10.1016/j.scienta.2021.110308
- Doan, C. C., & Tanaka, M. (2022). Relationships between tomato cluster growth indices and cumulative environmental factors during greenhouse cultivation. Scientia horticulturae, 295, 110803. https://doi.org/10.1016/j.scienta.2021.110803
- Duursma, R. A. (2015). Plantecophys - an R package for analysing and modelling leaf gas exchange data. PLoS One, 10(11), e0143346. https://doi.org/10.1371/journal.pone.0143346
- Farquhar, G. D., von Caemmerer, S., & Berry, J. A. (1980). A biochemical model of photosynthetic CO2 assimilation in leaves of C3 species. Planta, 149(1), 78–90. https://doi.org/10.1007/BF00386231
- Hernandez-Santana, V., Perez-Arcoiza, A., Gomez-Jimenez, M. C., & Diaz-Espejo, A. (2021). Disentangling the link between leaf photosynthesis and turgor in fruit growth. The Plant Journal: For Cell and Molecular Biology, 107(6), 1788–1801. https://doi.org/10.1111/tpj.15418
- Hidaka, K., Dan, K., Imamura, H., Miyoshi, Y., Takayama, T., Sameshima, K., Kitano, M., & Okimura, M. (2013). Effect of supplemental lighting from different light sources on growth and yield of strawberry. Environment Control in Biology, 51(1), 41–47. https://doi.org/10.2525/ecb.51.41
- Hidaka, K., Dan, K., Miyoshi, Y., Imamura, H., Takayama, T., Kitano, M., Sameshima, K., & Okimura, M. (2016). Twofold increase in strawberry productivity by integration of environmental control and movable beds in a large-scale greenhouse. Environment Control in Biology, 54(2), 79–92. https://doi.org/10.2525/ecb.54.79
- Hidaka, K., Miyoshi, Y., Ishii, S., Suzui, N., Yin, Y. G., Kurita, K., Nagao, K., Araki, T., Yasutake, D., Kitano, M., & Kawachi, N. (2019). Dynamic analysis of photosynthate translocation into strawberry fruits using non-invasive 11C-labeling supported with conventional destructive measurements using 13C-labeling. Frontiers in Plant Science, 9, 1–12. https://doi.org/10.3389/fpls.2018.01946
- Hidaka, K., Nakahara, S., Yasutake, D., Zhang, Y., Okayasu, T., Dan, K., Kitano, M., & Sone, K. (2022). Crop-local CO2 enrichment improves strawberry yield and fuel use efficiency in protected cultivations. Scientia horticulturae, 301, 111104. https://doi.org/10.1016/j.scienta.2022.111104
- Hidaka, K., Okamoto, A., Araki, T., Miyoshi, Y., Dan, K., Imamura, H., Kitano, M., Sameshima, K., & Okimura, M. (2014). Effect of photoperiod of supplemental lighting with light-emitting diodes on growth and yield of strawberry. Environmental Control in Biology, 52(2), 63–71. https://doi.org/10.2525/ecb.52.63
- Kaneko, T., Nomura, K., Yasutake, D., Iwao, T., Okayasu, T., Ozaki, Y., Mori, M., Hirota, T., & Kitano, M. (2022). A canopy photosynthesis model based on a highly generalizable artificial neural network incorporated with a mechanistic understanding of single-leaf photosynthesis. Agricultural and Forest Meteorology, 323, 109036. https://doi.org/10.1016/j.agrformet.2022.109036
- Kawanobu, S., Waiima, T., Zushi, K., Mori, T., & Matsuzoe, N. (2010). Seasonal variations in the maturation period, anthocyanin content, and ascorbic acid content in strawberry fruits. Environment Control in Biology, 48(4), 175–184. https://doi.org/10.2525/ecb.48.175
- Kimura, K., Yasutake, D., Koikawa, K., & Kitano, M. (2020). Spatiotemporal variability of leaf photosynthesis and its linkage with microclimates across an environment-controlled greenhouse. Biosystems Engineering, 195, 97–115. https://doi.org/10.1016/j.biosystemseng.2020.05.003
- Konsin, M., Voipio, I., & Palonen, P. (2001). Influence of photoperiod and duration of short-day treatment on vegetative growth and flowering of strawberry (Fragaria 3 ananassa Duch.). Journal of Horticultural Science and Biotechnology, 76(1), 77–82. https://doi.org/10.1080/14620316.2001.11511330
- Krüger, E., Josuttis, M., Nestby, R., Toldam-Andersen, T. B., Carlen, C., & Mezzetti, B. (2012). Influence of growing conditions at different latitudes of Europe on strawberry growth performance, yield and quality. Journal of Berry Research, 2(3), 143–157. https://doi.org/10.3233/JBR-2012-036
- Ledesma, N. A., & Kawabata, S. (2016). Responses of two strawberry cultivars to severe high temperature stress at different flower development stages. Scientia horticulturae, 211, 319–327. https://doi.org/10.1016/j.scienta.2016.09.007
- Ledesma, N. A., Nakata, M., & Sugiyama, N. (2008). Effect of high temperature stress on the reproductive growth of strawberry cvs. ‘Nyoho’ and ‘Toyonoka’. Scientia horticulturae, 116(2), 186–193. https://doi.org/10.1016/j.scienta.2007.12.010
- Long, S. P., Zhu, X. G., Naidu, S. L., & Ort, D. R. (2006). Can improvement in photosynthesis increase crop yields? Plant, Cell & Environment, 29(3), 315–330. https://doi.org/10.1111/j.1365-3040.2005.01493.x
- Medlyn, B. E., Duursma, R. A., Eamus, D., Ellsworth, D. S., Prentice, I. C., Barton, C. V. M., Crous, K. Y., De Angelis, P., Freeman, M., & Wingate, L. (2011). Reconciling the optimal and empirical approaches to modelling stomatal conductance. Global Change Biology, 17(6), 2134–2144. https://doi.org/10.1111/j.1365-2486.2010.02375.x
- Menzel, C. M. (2021). A review of productivity in strawberries: Marketable yield has a linear, but inconsistent relationship with total yield, and cannot be predicted from total yield. Journal of Horticultural Science and Biotechnology, 96(2), 135–144. https://doi.org/10.1080/14620316.2020.1808086
- Miyoshi, Y., Hidaka, K., Okayasu, T., Yasutake, D., & Kitano, M. (2017). Effects of local CO2 enrichment on strawberry cultivation during the winter season. Environment Control in Biology, 55(4), 165–170. https://doi.org/10.2525/ecb.55.165
- Miyoshi, Y., Hidaka, K., Yin, Y. G., Suzui, N., Kurita, K., & Kawachi, N. (2021). Non-invasive 11C-imaging revealed the spatiotemporal variability in the translocation of photosynthates into strawberry fruits in response to increasing daylight integrals at leaf surface. Frontiers in Plant Science, 12, 688887. https://doi.org/10.3389/fpls.2021.688887
- Muir, C. D. (2019). Tealeaves: An R package for modelling leaf temperature using energy budgets. AoB Plants, 11(6), lz054. https://doi.org/10.1093/aobpla/plz054
- Nakai, H., Yasutake, D., Kimura, K., I, K., Hidaka, K., Eguchi, T., Hirota, T., Okayasu, T., Ozaki, Y., & Kitano, M. (2022). Dynamics of carbon export from leaves as translocation affected by the coordination of carbohydrate availability in field strawberry. Environmental and Experimental Botany, 196, 104806. https://doi.org/10.1016/j.envexpbot.2022.104806
- Niinemets, Ü., & Keenan, T. (2014). Photosynthetic responses to stress in Mediterranean evergreens: mechanisms and models. Environmental and Experimental Botany, 103, 24–41. https://doi.org/10.1016/j.envexpbot.2013.11.008
- Nomura, K., Yasutake, D., Kaneko, T., Takada, A., Okayasu, T., Ozaki, Y., Mori, M., & Kitano, M. (2021). Long-term compound interest effect of CO2 enrichment on the carbon balance and growth of a leafy vegetable canopy. Scientia horticulturae, 283, 110060. https://doi.org/10.1016/j.scienta.2021.110060
- Opstad, N., Sønsteby, A., Myrheim, U., & Heide, O. M. (2011). Seasonal timing of floral initiation in strawberry: Effects of cultivar and geographic location. Scientia horticulturae, 129(1), 127–134. https://doi.org/10.1016/j.scienta.2011.03.022
- Sønsteby, A., Solhaug, K. A., & Heide, O. M. (2016). Functional growth analysis of ‘Sonata’ strawberry plants grown under controlled temperature and daylength conditions. Scientia horticulturae, 211, 26–33. https://doi.org/10.1016/j.scienta.2016.08.003
- Twitchen, C., Else, M. A., & Hadley, P. (2021). The effect of temperature and light intensity on rate of strawberry fruit ripening. Acta horticulturae, 1309(1309), 643–648. https://doi.org/10.17660/ActaHortic.2021.1309.92
- Wang, R., Eguchi, M., Gui, Y., & Iwasaki, Y. (2020). Evaluating the effect of light intensity on flower development uniformity in strawberry (Fragaria ×ananassa) under early induction conditions in forcing culture. HortScience, 55(5), 670–675. https://doi.org/10.21273/HORTSCI14917-20
- Yokoyama, G., Ono, S., Yasutake, D., Hidaka, K., & Hirota, T. (2023). Diurnal changes in the stomatal, mesophyll, and biochemical limitations of photosynthesis in well-watered greenhouse-grown strawberries. Photosynthetica, 61(1), 1–12. https://doi.org/10.32615/ps.2023.001
- Yoneda, A., Yasutake, D., Hidaka, K., Muztahidin, N. I., Miyoshi, Y., Kitano, M., & Okayasu, T. (2020). Effects of supplemental lighting during the period of rapid fruit development on the growth, yield, and energy use efficiency in strawberry plant production. International Agrophysics, 34(2), 233–239. https://doi.org/10.31545/INTAGR/117623