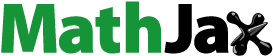
© 2020, Chapman & Hall
The expression ‘Monte Carlo methods’ can be understood in many different ways—correspondingly, there exist different types of Monte Carlo methods. An exhaustive list of the existing books on this topic would arguably be difficult to compile. What is certain is that the present book by Emmanuel Gobet, focused on Monte Carlo methods related to stochastic processes in continuous time, is both a remarkably clear textbook for students (most of the chapters include exercises) and a survey at the edge of current research. A remarkable strength of this book is to be able to cover an impressive amount of topics in only 300 pages, from fundamental results such as the Law of large numbers and the Central Limit Theorem, to the simulation of non-linear processes involving regression (notably Backward Stochastic Differential Equations) and interacting particle systems (related to non-linear diffusions in the sense of McKean). Another important feature of the book is to always keep an eye on computational issues, from the generation of random numbers to the complexity analysis of Monte Carlo methods built on the time discretization of random processes.
This book is organized in three parts. The first one starts with the basics: it is devoted to exact simulation methods for random variables and to the analysis of empirical means of independent and identically distributed samples. Here we find the classical asymptotic results based on the Central Limit Theorem, together with non-asymptotic error estimates based on concentration inequalities. The main variance reduction techniques such as the antithetic sampling, importance sampling and control variates are also described in an elementary setting.
The second part focuses on Monte Carlo methods for Stochastic Differential Equations and the related PDEs. After an introductory part that presents in a concise manner the main tools of stochastic calculus and uniqueness theory for SDEs, a chapter is dedicated to the analysis of the convergence of the Euler scheme. Weak and strong convergence results are given, as well as some extensions of these results to stopped diffusions. The following chapter, arguably one of the most original in this part of the book, focuses on determining the optimal trade-off between the statistical (or Monte Carlo) error and the discretization error. This chapter also presents the MultiLevel Monte Carlo method in the framework of SDEs that allows to recover an asymptotic computational complexity close to , ε being the desired precision (while a Monte Carlo method based on an Euler scheme would typically lead to a complexity of order
).
The third part deals with Monte Carlo methods for non linear stochastic processes. A particular emphasis is put on Backward Stochastic Differential Equations and their approximation. These equations are structurally related to conditional expectations, which therefore need to be approximated within a discretization scheme. The so-called empirical regression is a standard procedure used to approximate these quantities in the simulation of BSDEs. Therefore, an entire chapter is devoted to a description of empirical regression methods and to the choice of the space of approximating functions. Precise theoretical results are provided in the case of the linear regression with local polynomial functions. Finally, a very last chapter contains an introduction to interacting particles systems and how they can be used to approximate non-linear diffusions in the sense of McKean.
As a conclusion, we would suggest this book as a textbook to any instructor in charge of a master-level (or doctoral-level) course on Monte Carlo methods for stochastic processes, to researchers looking for a deep dive into selected research topics, and to practitioners looking for a neat introduction to simulation methods, with a true concern for numerical complexity.
Additional information
Notes on contributors
Aurélien Alfonsi
Aurélien Alfonsi is professor at Ecole des Ponts ParisTech, part-time professor at Ecole Polytechnique, and deputy director of the CERMICS. He is the head of the master of financial mathematics at Ecole des Ponts. He is member of the Inria MathRisk project-team and involved in the chairs “Financial Risks” (Ecole des Ponts, Ecole Polytechnique, Sorbonne Université and Société Générale) and “Future of Quantitative Finance” (Ecole des Ponts, Université Paris Cité and BNP Paribas). His research brings on mathematical finance, insurance and numerical methods in probability.
Stefano De Marco
Stefano De Marco is Associate Professor in Probability and Mathematical Finance at Ecole Polytechnique, Paris. He owns a PhD in applied mathematics from Scuola Normale Superiore di Pisa and Université Paris-Est. He is a member of the steering committee of the Chaire Stress Test (Ecole Polytechnique and BNP Paribas) and he has taken part in the works of the Chaire Risques Financiers, a joint research project with Société Générale. He is the academic director of the 1st year of the Double degree Data and Finance offered jointly by Ecole Polytechnique and HEC Paris. His research focuses on risk management problems for options, volatility modeling and Monte Carlo methods.