1. Introduction
Aging is a significant contributor to various chronic diseases, thus emerging as an increasingly important area of research. Elucidating the mechanisms underlying aging can facilitate the development of dual-purpose therapeutic strategies aimed at slowing aging and preventing the onset of these diseases. Following the identification of the first age-related gene, age-1, in the late 1980s [Citation1], numerous pivotal discoveries have been made in the field of aging research using traditional screening and experimental approaches. However, the advent of the 2020s marked a transformative shift, as artificial intelligence (AI) began to play a significant role in driving innovative and ground-breaking discoveries in aging research (). To date, over 200 geroprotectors that extend lifespan in model organisms have been discovered [Citation2]. For instance, metformin, rapamycin, and lithium have demonstrated anti-aging effects in mice [Citation3,Citation4]. The hallmarks of aging [Citation5] are recognized as a pivotal foundation for investigating the multifaceted process of aging [Citation6]. These hallmarks comprise diverse mechanisms considered as the signatures of aging, including the loss of autophagy, cellular senescence, impaired proteostasis, chronic inflammation and epigenetic changes [Citation5]. Among them, cellular senescence, which is linked to aging and various diseases, has been mitigated through the use of senolytics such as dasatinib, quercetin, and fisetin [Citation7]. Furthermore, metformin and rapamycin have been shown to inhibit the senescence-associated secretory phenotype, thereby preventing cellular senescence [Citation8]. Given that many of these aging processes can also contribute to various diseases [Citation6], targeting them could lead to the discovery of more efficacious dual-purpose interventions, promoting healthy aging and mitigating age-related diseases.
Figure 1. Timeline of aging research and the emergence of dual-purpose aging and disease targets. Key discoveries made in the field of aging research over the past decades are summarized and categorized. PIK3Cs represent PIK3CA, PIK3CB, PIK3CD and PIK3CG which are the human orthologs of age-1 in C. elegans. LLM, large language model.
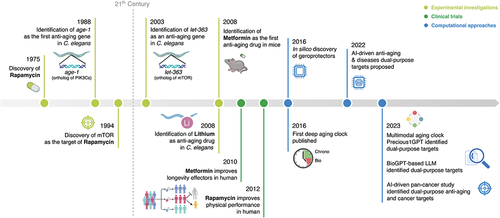
2. Current dual-purpose targets in aging and disease
Aging has been linked to multiple chronic diseases in humans, including cancers, fibrotic, metabolic, cardiovascular, and neurodegenerative diseases [Citation6]. Hence, the molecular mechanisms and features underlying the aging process may also contribute to the onset or progression of such diseases and are worth further investigation. Specifically, aging and cancers share various cellular processes involved in the hallmarks of aging and hallmarks of cancer, such as cellular senescence [Citation9], constituting the common ‘meta-hallmarks’ [Citation10]. These meta-hallmarks are of particular interest in terms of identifying age-related cancer targets. For instance, mTOR (mammalian target of rapamycin) has been proposed as a promising dual-purpose target due to its pivotal role in modulating the aging process and tumorigenesis [Citation11] (). Associated with all established hallmarks of aging [Citation6], mTOR regulates a wide range of biological pathways that contribute to aging, including autophagy, cellular senescence, inflammation, and proteostasis. This involvement offers potential for improving clinical outcomes as aging contributes to the onset and progression of multiple diseases. However, the identification of dual-purpose targets via conventional experimental methods can be time-consuming, requiring the acquisition of multidimensional data that includes omics, clinical, and text-based information. For example, metformin, an agonist of AMPK, was suggested to provide anti-aging effects four years after its FDA approval for treating type II diabetes [Citation12] (). Likewise, lithium targeting GSK3B has been prescribed to psychiatric disorder patients for over 50 years [Citation3], with its anti-aging effects only discovered in the late 2000s [Citation13] (). In addition, the associations between aging and cardiovascular diseases (CVDs) have been well-documented, leading to subsequent in-depth studies on the shared targets and pathways implicated between them. Specifically, mTOR, AMPK, sirtuins, and insulin growth factor-1 (IGF-1) have been suggested to play protective roles in both CVDs and aging [Citation14], further supporting the premise that dual-purpose targeting approaches could deliver benefits across a wide range of diseases.
Table 1. Dual-purpose targets in aging and disease with their approved drugs.
3. AI-powered discovery of potential dual-purpose aging and disease targets
AI is increasingly used in aging research to integrate and analyze multiple omics data types [Citation15] such as genomics, transcriptomics, epigenomics, and proteomics, collected from individuals at different ages. This integration helps explore physiological changes, identify biomarkers, and predict potential anti-aging targets and compounds. Moreover, deep neural networks have been employed to develop deep aging clocks that outperform traditional machine learning algorithms in predicting biological age based on identified biomarkers [Citation16]. These aging clocks hold potential applications in target identification, health trajectory prediction, and patient enrollment in age-adjusted clinical trials [Citation17]. Furthermore, AI-based approaches have shown promise in identifying potential unexplored geroprotectors. For instance, GeroScope, a computational tool that utilizes human gene expression data across different age groups to predict new geroprotectors [Citation18], was used to evaluate the potential side effects of the compounds using AI. Moreover, another study [Citation19] utilized various bioinformatic approaches and deep neural networks to identify natural compounds with high similarity to known anti-aging compounds, metformin and rapamycin, based on their genes and pathways-level signatures. These studies not only showcased the application of AI in aging research but also served as pioneering work in identifying age-related targets that paved the way for the subsequent identification of dual-purpose targets.
AI assistance has led to studies demonstrating substantial reductions in the time and cost required for identifying promising therapeutic targets in aging and age-related diseases [Citation6,Citation20,Citation21]. For example, a study [Citation6] used a combination of omics- and text-based AI models to identify therapeutic targets specific to age-related diseases. Several biological processes, such as inflammation, cellular senescence, and extracellular matrix stiffness, were identified as playing roles in both aging and various age-related diseases. Given the significant increase in disease risk with age, it is not surprising that many AI-prioritized therapeutic targets also show strong associations with diverse hallmarks of aging. These include high-confidence targets such as mTOR, SIRT1, IGF-1, and AKT1, along with the medium to high novelty target TNIK, all of which are associated with multiple aging hallmarks. The study also proposed several dual-purpose targets, namely CXCL12, SPP1, ITGB5, and ADAMTS14, which could hold promise for both anti-aging and disease treatments. In another study, Olsen et al. proposed three potential novel dual-purpose therapeutic targets for glioblastoma multiforme treatment to benefit aged cancer patients by integrating survival data and AI-driven analysis [Citation22]. Furthermore, a recent comprehensive AI-driven analysis of large-scale omic datasets identified several novel dual-purpose target candidates for the treatment of cancer and aging, and validated one of the most promising candidates, KDM1A, a histone demethylase that was shown to be downregulated during aging across multiple tissues while upregulated in various solid cancers, in Caenorhabditis elegans [Citation23]. Urban et al. employed multimodal transformer-based transfer learning and feature importance analysis to analyze DNA methylation and gene expression data. This led to the successful identification of APLNR and IL23R as dual-purpose candidates, implicated in the regulation of both aging and multiple age-related diseases [Citation21]. Recently, Zagirova et al. presented a novel approach for predicting therapeutic targets using a large language model (LLM). This method enabled the identification of two novel dual-purpose targets, CCR5 and PTH, bypassing the need for omics data analysis [Citation24]. Overall, identifying dual-purpose targets can lead to the development of preventive treatments and therapeutic remedies for age-related diseases, ultimately enhancing clinical outcomes for patients. Notably, the use of AI can significantly expedite this process, reducing both the time and cost involved in discovering these dual-purpose targets.
4. Expert opinion
The ultimate goal of aging research is to prolong human lifespan and healthspan. Aging amplifies susceptibility to a wide range of human disorders, often resulting in the development of numerous chronic conditions and disabilities. We believe that the identification of dual-purpose targets could pave the way to more efficient and effective treatments for age-related diseases by targeting the intersecting processes that underpin both aging and disease. Identifying such targets does not only address the specific disease but also yields broader anti-aging effects, which provides additional benefits in preventing various diseases and enhancing clinical outcomes. However, to date, only a limited number of FDA-approved drugs, including dasatinib, lithium, metformin, and rapamycin, exhibit dual-purpose effects for anti-aging and disease treatment. The challenge in discovering additional dual-purpose targets or compounds is largely attributed to the prohibitive costs and durations of in vivo lifespan experiments, along with limited data availability. Besides, the relationship between these diseases and aging is not yet comprehensively understood. Therefore, it is imperative to unmask the associations between diseases and aging, including overlapped signaling pathways, common triggers, and synergistic effects, by developing more effective and efficient approaches for studying aging and age-related diseases.
AI is increasingly harnessed to integrate multiple omics data types and augment our understanding, diagnosis, and treatment of diseases. AI-based approaches have demonstrated considerable potential in discovering therapeutic targets, analyzing complex biological data, screening compounds, evaluating side effects, and predicting novel geroprotectors. Deep learning models, such as generative adversarial networks and deep neural networks, are being employed to identify the patterns underlying aging and diseases. Moreover, the druggability of therapeutic targets can significantly influence the success of drug development. Accessing structural information on therapeutic targets is vital as it can aid in determining their druggability, and in the molecular design and optimization of drugs, which can also be predicted by AI-based tools like AlphaFold [Citation25]. AI-powered prediction models have been used to pinpoint shared biological processes implicated in both aging and diseases. Consequently, dual-purpose targets that modulate these processes could offer a promising path for the development of anti-aging and disease treatments. Moreover, dual-purpose therapeutic strategies could vary depending on the specific disease and desired outcome. First, dual-purpose targets could aim at delaying aging while preventing or combating age-related diseases. Second, they could target the aging components contributing to disease onset or progression in patients to treat the disease or improve clinical outcomes in aged patients.
However, it is also important to recognize some current limitations of AI in dual-purpose target discovery. Firstly, the performance and applicability of AI in aging research are significantly constrained by the availability of accurate longitudinal biological data. Synthetic virtual data may offer a solution to this issue, but this approach is still in its early stages and lacks extensive validation, especially when applied to complex biological processes such as aging. Finally, the use of large language models, typically trained on human-generated text, presents another challenge. These models may lack the ability to accurately assess the quality and relevance of the input data, possibly perpetuating human biases and preconceived notions. Despite these challenges, recent advancements in AI present promising prospects for identifying novel dual-purpose targets and pathways, advancing not only aging research but also multiple disease areas.
Declaration of interest
The authors are affiliated with Insilico Medicine, a commercial company developing and using generative artificial intelligence and other next-generation AI technologies and robotics for drug discovery, drug development, and aging research. Utilizing its generative AI platform and a range of deep aging clocks, Insilico Medicine has developed a portfolio of multiple therapeutic programs targeting fibrotic diseases, cancer, immunological diseases, and a range of age-related diseases.
The authors have no other relevant affiliations or financial involvement with any organization or entity with a financial interest in or financial conflict with the subject matter or materials discussed in the manuscript apart from those disclosed.
Reviewer disclosures
Peer reviewers on this manuscript have no relevant financial or other relationships to disclose.
Additional information
Funding
References
- Friedman DB, Johnson TE. Three mutants that extend both mean and maximum life span of the nematode, Caenorhabditis elegans, define the age-1 gene. J Gerontol. 1988;43(4):B102–9. doi: 10.1093/geronj/43.4.B102
- Moskalev A, Chernyagina E, de Magalhães JP, et al. Geroprotectors.Org: a new, structured and curated database of current therapeutic interventions in aging and age-related disease. Aging. 2015;7(9):616–628. doi: 10.18632/aging.100799
- Araldi E, Jutzeler CR, Ristow M. Lithium treatment extends human lifespan: findings from the UK Biobank. Aging. 2023;15(2):421–440. doi: 10.18632/aging.204476
- Liu JK. Antiaging agents: safe interventions to slow aging and healthy life span extension. Nat Prod Bioprospect. 2022;12(1):18. doi: 10.1007/s13659-022-00339-y
- Lopez-Otin C, Blasco MA, Partridge L, et al. Hallmarks of aging: an expanding universe. Cell. 2023;186(2):243–278. doi: 10.1016/j.cell.2022.11.001
- Pun FW, Leung, G. H. D., Leung, H. W., et al. Hallmarks of aging-based dual-purpose disease and age-associated targets predicted using PandaOmics AI-powered discovery engine. Aging. 2022;14(6):2475–2506. doi: 10.18632/aging.203960
- Zhang L, Pitcher LE, Yousefzadeh MJ, et al. Cellular senescence: a key therapeutic target in aging and diseases. J Clin Invest. 2022;132(15). doi: 10.1172/JCI158450
- Chaib S, Tchkonia T, Kirkland JL. Cellular senescence and senolytics: the path to the clinic. Nat Med. 2022;28(8):1556–1568. doi: 10.1038/s41591-022-01923-y
- Chatsirisupachai K, Palmer D, Ferreira S, et al. A human tissue-specific transcriptomic analysis reveals a complex relationship between aging, cancer, and cellular senescence. Aging Cell. 2019;18(6):e13041. doi: 10.1111/acel.13041
- Lopez-Otin C, Pietrocola F, Roiz-Valle D, et al. Meta-hallmarks of aging and cancer. Cell Metab. 2023;35(1):12–35. doi: 10.1016/j.cmet.2022.11.001
- Johnson SC, Rabinovitch PS, Kaeberlein M. mTOR is a key modulator of ageing and age-related disease. Nature. 2013;493:338–345.
- Anisimov VN, Berstein LM, Egormin PA, et al. Metformin slows down aging and extends life span of female SHR mice. Cell Cycle. 2008;7(17):2769–2773. doi: 10.4161/cc.7.17.6625
- McColl G, Killilea DW, Hubbard AE, et al. Pharmacogenetic analysis of lithium-induced delayed aging in Caenorhabditis elegans. J Biol Chem. 2008;283(1):350–357. doi: 10.1074/jbc.M705028200
- North BJ, Sinclair DA. The intersection between aging and cardiovascular disease. Circ Res. 2012;110(8):1097–1108. doi: 10.1161/CIRCRESAHA.111.246876
- Marino N, Putignano G, Cappilli S, et al. Towards AI-driven longevity research: an overview. Front Aging. 2023;4:1057204. doi: 10.3389/fragi.2023.1057204
- Putin E, Mamoshina, P., Aliper, A., et al. Deep biomarkers of human aging: Application of deep neural networks to biomarker development. Aging. 2016;8(5):1021–1033. doi: 10.18632/aging.100968
- Zhavoronkov A, Mamoshina P. Deep aging clocks: the emergence of AI-Based biomarkers of aging and longevity. Trends Pharmacol Sci. 2019;40(8):546–549. doi: 10.1016/j.tips.2019.05.004
- Aliper A, Belikov AV, Garazha A, et al. In search for geroprotectors: in silico screening and in vitro validation of signalome-level mimetics of young healthy state. Aging. 2016;8(9):2127–2152. doi: 10.18632/aging.101047
- Aliper A, Jellen L, Cortese F, et al. Towards natural mimetics of metformin and rapamycin. Aging. 2017;9(11):2245–2268. doi: 10.18632/aging.101319
- Pun FW, Ozerov IV, Zhavoronkov A. AI-powered therapeutic target discovery. Trends Pharmacol Sci. 2023;44(9):561–572. doi: 10.1016/j.tips.2023.06.010
- Urban A, Sidorenko D, Zagirova D, et al. Precious1GPT: multimodal transformer-based transfer learning for aging clock development and feature importance analysis for aging and age-related disease target discovery. Aging. 2023;15:4649–4666. doi: 10.18632/aging.204788
- Olsen A, Harpaz Z, Ren C, et al. Identification of dual-purpose therapeutic targets implicated in aging and glioblastoma multiforme using PandaOmics - an AI-enabled biological target discovery platform. Aging. 2023;15:2863–2876. doi: 10.18632/aging.204678
- Pun FW, Leung, G. H. D., Leung, H. W., et al. A comprehensive AI-driven analysis of large-scale omic datasets reveals novel dual-purpose targets for the treatment of cancer and aging. Aging Cell. 2023:e14017. doi: 10.1111/acel.14017
- Zagirova D, Pushkov S, Leung GHD, et al. Biomedical generative pre-trained based transformer language model for age-related disease target discovery. Aging. 2023;15(18):9293–9309. doi: 10.18632/aging.205055
- Varadi M, Anyango S, Deshpande M, et al. AlphaFold protein structure database: massively expanding the structural coverage of protein-sequence space with high-accuracy models. Nucleic Acids Res. 2022;50(D1):D439–D444. doi: 10.1093/nar/gkab1061