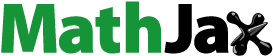
ABSTRACT
Climate change is gradually rising to a level that portends an existential threat to humanity. Nevertheless, environmental modellers, particularly in South Africa, still rely on restrictive environmental quality metrics for policy insights. Here, a comprehensive metric (load capacity factor [LCF]) that captures both the demand and supply sides of environmental qualities was activated. With the dataset (1970–2018), estimates of autoregressive distributive lag and quantile-ARDL underscored the varied effects of the selected factors on LCF. It was unravelled that green-technology significantly improved LCF only at the upper quantile but remained ineffective afterwards. Notably, LCF improved substantially at some quantiles following the transition to clean energy. Resource rents promoted LCF partially at the upper and middle quantiles but ineffective towards the lower quantile of the distributions. Economic growth improved LCF within the upper and middle quantiles, whereas it minimised LCF at the upper quantiles. We have provided relevant policy insights therein.
1. Introduction
Among other African countries, the Republic of South Africa (RSA) has remained committed and consistent in the push towards the evolution of sustainable environments. In 2012, RSA articulated within its national development plan ‘our future make it work’ policy option conducive to mitigating climatic change and its associated environmental pollutions. Interestingly, RSA’s policy template precedes the African Union’s (AU) ‘Africa we want’ agenda 2063. It also precedes the United Nations (UN) Sustainable Development Goals (SDGs). However, it is imperative to underscore that RSA’s development plan aligns consistently with the development policies of both the AU and the UN. Within the national development policy template of RSA, chapter eight (8) specifically highlights the need for the enthronement of an environmental ecosystem that is both sustainable and resilient. The article provides specific policy objectives to ensure resilient environments in RSA. Among the objectives are efficient monitoring and utilisation of natural resources, increased land and ocean protection, and zero-emission building standards by 2030. It also considers absolute reductions in annual waste disposal in landfill areas, as well as the contraction of at least 20 000MW of renewable energy by 2030.Footnote1
As highlighted above, the objective of a sustainable and resilient environment in RSA is in tandem with the UN’s SDGs 7, 11, 12, 13 and 14. Specifically, SDGs 7, 11, 12, 13 and 14 emphasised unrestricted access to modern electricity and increased reliance on renewable energy for all humankind by 2030. To ensure that human settlements and cities are friendly, resilient and sustainable by 2030. The agenda also emphasises efficient management of natural resources through sustainable consumption and production. The UN SDGs also underscored the importance of sustainable waste management, integration of climate change policies into national development plans, and the need to strengthen the adaptive and resilient capacities to hazards associated with climate change. Suffice it to highlight that the UN SDGs set a comprehensive development agenda that rectifies several policy options for global economies, of which the RSA is a committed partner.
Despite RSA’s national development plan and the country’s averred commitment to the UN SDGs framework. However, available facts suggest that several issues need to be ratified to enthrone climate change-resilient ecosystem in the republic. Among such critical issues is that RSA is among the global economies still battling with ecological imbalances. Specifically, based on the evidence available at Global Footprint Network (GFN), RSA has maintained an ecological deficit of 282%. In this case, the country is notable for having an ecological footprint over its biocapacities. This situation highlights a case where the available biocapacity of a nation is under-supplied compared to its population’s ecological footprints. This highlights that SA is engaged in importing biocapacities and liquidating available national ecological assets. The country is also involved in emitting hazardous carbon dioxide (CO2) into the already compromised environments (GFN Citation2023). and are graphical/pictorial illustrations of the peculiarities of RSA pertaining to her prevailing environmental challenges.
illustrates explicitly the imbalance between South Africa's ecological footprint and her biocapacity. On the other hand, demonstrates the prevailing load capacity factor (LCF) in South Africa. In this instance, the graph clearly highlights the dwindling LCF in the republic, which is far fellow the sustainability point (1). A country's LCF is obtained by dividing its biocapacity by its ecological footprint (Adebayo & Samour, Citation2023; Guloglu, Caglar, and Pata Citation2023; Pata et al. Citation2023). LCF, proposed by Siche et al. (Citation2010), represents a comprehensive indicator of environmental quality that, unlike others, considers both the demand and supply sides of environmental trajectories. It highlights simultaneously, the ability of the available natural ecosystem to withstand and sustain the pressures mounted on it through human activities on air, water and soil (Guloglu, Caglar, and Pata Citation2023). Meanwhile, a country’s ecological condition is considered sustainable if its LCF is about ‘1’, whereas it is considered unsustainable when it is less than 1. In the case of RSA, its LCF has been consistently less than one over the years, thereby confirming the highlighted ecological imbalance in the country.
Given the above instances, there is an urgent need to provide comprehensive empirical insights about the relevant factors to abate the prevailing ecological imbalances in the republic. Unfortunately, existing studies failed to consider the specific instance of South Africa in their enquiries. Notwithstanding, few studies (Adebayo and Samour Citation2023; Awosusi et al. Citation2022; Ullah et al. Citation2023) probed the instances of BRICS economies. However, streamlined policy insights peculiar to specific economies are only obtainable when country-specific studies are conducted. Likewise, these studies also failed to factor in the influence of green-innovation into their studies. When an essential environmental quality determinant like green technology is neglected, vital information to aid ecological resilience is concealed. Besides, other studies (Nathaniel, Bekun, and Faizulayev Citation2021; Udeagha and Muchapondwa Citation2022) focusing on RSA relied on sets of uncomprehensive environmental quality indicators like ecological footprint and CO2. It is also imperative to highlight that the assertions of these prior investigations are not without equivocations, which directly undermines policy prescriptions.
The current study seeks to rectify the effects of green-innovation, energy transition, and natural resource utilisations on environmental quality in South Africa. In this case, the current study's novelty lies in its ability to, for the first time, consider LCF as an environmental quality indicator specifically for RSA. Furthermore, unlike prior studies, the present investigation updates policy trajectories by factoring in green-innovation into environmental quality modelling in the case of South Africa. The current study also took advantage of the most updated data in GFN. Nonetheless, the applications of both the traditional autoregressive distributed lag (ARDL) and the quantile autoregressive distributed lag (QARDL) models distinguished the current study from others. Notably, unlike the traditional ARDL procedure, the recently introduced QARDL (Cho, Kim, and Shin Citation2015) is a time-variant econometric toolkit that explores the effects of explanatory variables across the quantile distributions of the explained variable (Hashmi et al. Citation2022; Omoke and Uche Citation2021). Likewise, the QARDL technique probes the potential heterogeneous (asymmetric) relationship both in the short and long term, therefore, facilitating broad-based policy alternatives. These notable steps are lacking in prior investigations particularly for RSA. Hence, due to its broad-based and holistic approaches, unique policy insights capable of invoking ecological balance in the republic are expected. Thus, policymakers in RSA are provided with an additional barometer to verify the progress of the national development plan and the eventual enthronement of living environments that are both sustainable and resilient.
The next section of the study is related literature, followed by the applicable methodologies and data description section. The penultimate section distils the empirical analysis and discussions, whereas the last section provides the summary and policy recommendations.
2. Review of related literature
A Plethora of empirical investigations has attempted to establish the relationship between environmental sustainability and its relevant predictors. In particular, the environmental Kuznets curve hypothesis (EKC [Kuznets Citation2019]) has remained the dominant and most established theoretical foundation for predicting environmental integrity (Alola, Özkan, and Usman Citation2023; Uche, Das, and Bera Citation2023). In light of the present study, the connectedness between the ecological load capacity factor and its determinants is established through the recently proposed load capacity curve (LCC) hypothesis attributable to Dogan and Pata (Citation2022). Like EKC theory, LCC proposes a U-Shaped relationship between economic expansion and load capacity factor. Notably, LCC predicts a dynamic situation whereby increased income improves ecological load capacity in the long term, having deteriorated it in the short run (Pata and Kartal Citation2023). This study probes the validity of the LCC hypothesis by determining the influence of green-technology, energy transition and natural resources utilisation on the ecological load capacity factor for South Africa.
Based on the aforementioned dynamic structure between environmental quality and anthropogenic activities, several studies have lent their opinions towards shaping policy orientations. For clarity, we have partitioned this part of the study into three thematic components to trace the effects of green-technology, energy-transitions, and resource rents on LCF as articulated in recent studies.
2.1. Green-innovations – ecological load capacity factor
As earlier highlighted, prior studies failed to trace the potential effects of green innovations on the ecological load capacity factor for South Africa and other economies. The notable drawbacks of these studies are that they have consistently adopted other environmental quality indicators like ecological footprint and CO2 in their investigations. For instance, Karimi Alavijeh et al. (Citation2023) probed the influence of technological innovation on environmental degradation (CO2) in EU countries. Based on the estimates of the MMQR, the study rectifies the improving effects of the environment through technological innovations. In a related study, Uche, Das, and Bera (Citation2023) confirmed that environmental-control technology warranted only short-term environmental quality-enhancing effects in India. A similar submission was provided in Udeagha and Muchapondwa (Citation2022) in the case of South Africa. Another similar study (Awosusi et al. Citation2022) inferred that technological advancements improved the environmental quality in South Africa. However, Uche et al. (Citation2023) admitted that environmental-control technology dampened environmental quality in BRICS countries.
In the case of Brazil, Liu et al. (Citation2022) aligned with some submissions haven noted that technological innovations enhanced Brazil's ecological load capacity factor. In a supporting submission, Dogan and Pata (Citation2022) illustrated that technological advancements ensure sustainable ecological balance in the case of G7 countries. Ni, Yang, and Razzaq (Citation2022) extended similar assertions. Accordingly, the study informed that technological innovativeness magnifies LCF in highly resource-consuming economies. Likewise, Kihombo et al. (Citation2021) and Yang, Jahanger, and Ali (Citation2021) emphasised technological innovations’ environmental quality soothing effects in West Africa and BICS economies. However, Destek and Manga (Citation2021) argued that technological diffusion increases ecological footprint and reduces CO2 emissions in big emerging markets.
Pata et al. (Citation2023) reemphasized that technological innovations are only effective in reducing CO2 emissions, whereas it minifies the load capacity factor in Germany. More discordant analogies are evident in most previous studies pertaining to the influence of technological innovations on environmental quality. For instance, Adebayo and Kirikkaleli (Citation2021), Su et al. (Citation2021), Usman and Hammar (Citation2021) and Rout, Gupta, and Sahoo (Citation2022) reported that technological innovations failed to improve ecological footprints in Japan, Brazil, Asian Pacifica Economic Cooperation nations and BRICS countries, respectively. In summary, past studies failed to establish a common ground on the innovations – environmental quality nexus. Likewise, only a few considered LCF, whereas South Africa's cases were seldom investigated. Therefore, to further clarify the objectives of the present investigation, we hypothesised that green-innovation is a positive predictor of LCF in South Africa,
2.2. Energy transition – ecological load capacity factor
Previous studies have not been able to quell the controversies bordering on energy transition (low-carbon energy) – load capacity factors interactions. Unfortunately, the discordant analogies still persist even in recent studies. For clarity, Khan et al. (Citation2023), via the estimates of the CS-ARDL procedure, admitted that renewable energy increases LCF in both G7 and E7 countries. Similar submissions prevailed in Iorember et al. (Citation2021) for South Africa, Adebayo and Kirikkaleli (Citation2021) for Spain, Dogan and Pata (Citation2022) for G7 countries, Obiakor, Uche, and Das (Citation2022) in the case of West Africa, Liu et al. (Citation2022) in Brazil, for ASEAN economies, as well as Uche et al. (Citation2022) in the case of E7 countries. Recent studies eulogised the enhancing effects of renewable energy on LCF. Accordingly, Adebayo (2023) informed that renewable energy increases LCF in BRICS nations. Likewise, Adebayo and Samour (Citation2023), Alola, Özkan, and Usman (Citation2023), Guloglu, Caglar, and Pata (Citation2023), Pata et al. (Citation2023), Samour et al. (Citation2023) and Ullah et al. (Citation2023) extended affirmative analogies in the instances of BRICS, India, OECD countries, Germany and BRICS-T economies, respectively.
Notwithstanding the overwhelming submissions of many prior studies on the appealing effects of renewable energy on LCF, there are still some divergent perspectives. In this instance, Pata and Isik (Citation2021) demonstrated through an ARDL analysis that renewable energy improves ELCF in the US, whereas it has no significant effects in the case of Japan. Beyond this, Huilan et al. (Citation2022) applied the fully modified ordinary least squares process. The study admitted that renewable energy minifies LCF in Mexico. Likewise, Pata and Samour (Citation2022) illustrated that renewable energy dampens LCF, particularly in France. This assertion is supported by the submission of Xu et al. (Citation2022), which, based on the estimates of the ARDL toolkit, reported that renewable energy failed to improve LCF in Brazil.
Nonetheless, Pata and Kartal (Citation2023) reaffirmed that renewable energy could not improve LCF in South Korea. Based on the prevailing review, it is imperative to underscore that the perspective of RSA vis-à-vis renewable energy – LCF nexus was neglected. Likewise, there are notable discordant viewpoints in extant studies regarding the nexus. To this end, we hypothesised that energy transition improves LCF in South Africa.
2.3. Natural resource – ecological load capacity factor
The empirical literature revealed that few studies had probed the potential effects of natural resource utilisation on LCF. However, the few studies in this perspective also failed to establish a common ground about the nexus. Accordingly, based on the empirical estimates of the ARDL, Akadiri et al. (Citation2022) inferred that natural resource rent enhances LCF in India. In contrast, Ni, Yang, and Razzaq (Citation2022) relied on the analysis of CS-ARDL to assert that natural resources dampened LCF in highly resource-consuming countries. Interestingly, Guloglu, Caglar, and Pata (Citation2023) remarked a positive influence of natural resource absorption on LCF in OECD countries. Nevertheless, the puzzle became more interesting as Pata and Isik (Citation2021) stressed rather a deleterious effect of resource rents on LCF in China.
Additionally, some extant studies explored the predictive effects of resource rent on other indicators of environmental quality. For instance, Opuala et al. (Citation2023) and Opuala-Charles, Omoke, and Uche (Citation2022) explain that resource rent contributed to CO2 emissions significantly in West Africa. On the flip side, Nathaniel, Bekun, and Faizulayev (Citation2021) maintained that resource rent dampens environmental quality significantly in BRICS economies. Similarly, Khan, Hou, and Le (Citation2021) reported that resource rent moderates the United States’ ecological footprint and CO2 emissions. In contrast, Zhang et al. (Citation2021) maintained that resource rent enhances environmental quality in Pakistan. However, for Zafar et al. (Citation2021) and Ullah et al. (Citation2023), natural resource rent is significantly associated with environmental pollution in Asia and 15 renewable consumption economies, respectively. Byaro, Nkonoki, and Mafwolo (Citation2023) reported that natural resource rent significantly predicts environmental pollution in sub-Saharan African countries. Nathaniel et al. (Citation2021) extended a more puzzling narrative by informing that resource rent hampered environmental quality in the case of ASEAN economies except for Laos PDR and Thailand, where it improves environmental quality significantly. To ascertain the actual effect of resource utilisation on environmental quality, this study hypothesised a negative association between resource rent and LCF in the context of RSA.
2.4. Summary of literature gaps
Notably, previous studies failed to probe the effects of the variables considered in this study on load capacity factor in the context of South Africa. Besides, the accounts of extant literature pertaining to the effects of green innovation on load capacity factor remained unclear. We also noted that prior studies relied on weak econometric tools lacking the capacity to provide reliable empirical estimates. This observed gaps and the need for updated insights compelled the current investigation.
3. Materials and methods
Based on the objectives of this investigation, the purpose of novelty and policy moderation, we have outlined the data descriptions, model specification and relevant estimation techniques within this section.
3.1. Data sources and descriptions
The study relied on secondary sourced annual time-series data spanning 1970–2018 specific to RSA. The choice of the data span is predicted by data availability, particularly data on GFN (Citation2023). The relevant data captured are LCF calculated by the authors following the ratification of Siche et al. (Citation2010). As previously indicated, LCF is obtained by dividing the biocapacity factor by the ecological footprint. Accordingly, the biocapacity factor and ecological footprint data were obtained from GFN. In this respect, green-tech (patent on environmental control technology[GTech]) is obtained from the data repository of the Organization for Economic Cooperation and Development (OECD). Energy transition (low-carbon energy – TWh equivalent[ETran]) is extracted from Our World in Data (OWID). Resource utilisation (% GDP of total natural resources rents[NR]) as well as the control variable (economic growth – Current US$ of GDP per capita[EGrt]) were extracted from World Development Indicator (WDI). The inclusion of economic growth (GDP) is informed by its notable influence on environmental quality as narrated in extant literature (Khan, Hou, and Le Citation2021; Uche and Effiom Citation2021a). Besides, evaluating the validity of the LCC hypothesis is based on its associated short and long-run effects on LCF.
3.2. Model specification
At this stage, the study provides an analytical framework to express the theoretical underpinnings of the ecological load capacity factor and the enlisted predictors. Notably, such a framework is essential in explaining the earlier stated hypotheses. Previous studies have applied many theoretical frameworks to explain the relevant series of environmental quality. Based on insights from Awosusi et al. (Citation2022), Ni, Yang, and Razzaq (Citation2022) and Alola, Özkan, and Usman (Citation2023), we construct a transformed Cobb–Douglas production function that explores the responses of LCF to the changes in the enlisted predictors. The mathematical interactions of the above association are availed in Equation 1.
(1)
(1) where
,
,
,
, and
depict ecological load capacity factor (dependent variable), green-technology, energy transition, natural resources rents, and economic growth. Equation (1) is further transformed into an econometric scheme, as illustrated in Equation 2.
(2)
(2) In Equation (2),
and
depict the time-sets and stochastic error-term, respectively. Meanwhile,
,
,
and
are the coefficients of the explanatory variables,
is the intercept. Following the earlier stated hypotheses, green-technology, as well as energy transitions, are expected to surge ecological load capacity factor, i.e.
,
On the other hand, resources rents is expected to dampen ecological load capacity factor, i.e.
; likewise, GDP is expected to mitigate ecological load capacity factor, i.e.
3.3. Estimation procedures
Besides several preliminary evaluations, including summary statistics, correlation analysis, variance inflation factor analysis, nonlinearity test, stationarity tests and cointegration test, the empirical estimates of this study emerged from two primary techniques. This includes the conventional ARDL procedure and the newly introduced quantile ARDL procedure. Hence, the relevant information on these estimation procedures is provided herewith.
3.3.1. The autoregressive distributed lag (ARDL) procedure
The ARDL econometric technique, akin to Pesaran and Shin (Citation1999), is adopted as the baseline estimation procedure. The option of the ARDL is premised on its several notable attributes, including the ability to avail reliable estimates both in the short and long terms. Besides, the model is dynamic, flexible and permits the evaluation of series with either uniform or mixed-orders of integration (Pesaran and Shin Citation1999). In addition, the ARDL technique adopts the bounds-test cointegration process of Pesaran, Shin, and Smith (Citation2001) to verify potential long-run convergence between the explained variable and the explanatory variables. Several studies, including (Akadiri et al. Citation2022; Opuala-Charles, Omoke, and Uche Citation2022; Uche Citation2022) have relied on this technique. Hence, the typical functional scheme of the ARDL technique is provided in Equation 3.
(3)
(3) In Equation (3) γt depicts the predicted variable, while
represents all the vectors of the explanatory factors,
connotes the difference operator, and εt represents the error correction term or the speed of adjustment.
denotes the short-run outcomes, and
represents the long-term estimates. Furthermore, Equation (3) is transformed into Equation (4) with the inclusions of the enlisted series.
(4)
(4) The long-term convergence of the series can be obtained from Equation (4) following the specification of the null and alternative hypotheses:
;
Hence HO represents the null hypothesis of no long-run convergence, whereas H1 illustrates the alternative hypothesis of long-term convergence among the series. Implicatively, the study will affirm long-term convergence when there is overwhelming evidence not to accept the null hypothesis.
3.3.2. The quantile autoregressive distributed lag (QaRDL) procedure
One factor distinguishing the present study from the rest is the adoption of the QARDL econometrics toolkit to verify the determinants of LCF for RSA. The QARDL procedure extended by Cho, Kim, and Shin (Citation2015) is a unique time-sensitive technique that avails the effects of relevant explanatory variables over the distributions of the predicted series. The model provides reliable empirical evidence over the long and short terms (Cho, Kim, and Shin Citation2015). The QARDL is superior to most estimation techniques since its outputs are characterised by conditional distributional effects rather than average effects (Omoke and Uche Citation2021). The model elicits information about potential short- and long-term distributional asymmetries (Hashmi et al. Citation2022). Besides, the technique is robust to possible serial correlations. On this basis, more policy insights are availed to ensure improvements in A's ecological load capacity factor. Henceforth, the typical functional scheme of the QARDL is depicted in Equation (5).
(5)
(5) In Equation 5,
depicts the quantile index,
is the drift coefficient and n1 – n5 are the respective lag orders determined by the specified lag length criterion.
are short-run coefficients, whereas
depict long-run coefficients. Based on the estimates of equation (5), the study is able to evaluate the potential distributional asymmetric effects of the enlisted series on LCF. Hence, 11 quantiles:
are identified as the basis of this investigation in alignment with (Hashmi et al. Citation2022; Uche and Effiom Citation2021b).
The null hypotheses are provided below:
Short-run parameter constancy test:
Long-run parameter constancy test:
The null hypotheses are provided to critique the eventual parameter constancy effects among the enlisted series. Accordingly, the Wald test, which follows a chi-square distribution asymptotically, is adopted to decide whether to accept or reject the null hypotheses.
4. Data analysis and discussions
As earlier stated, the empirical analysis of this study began with relevant pre-evaluation techniques. Hence, this component is dedicated to revealing the summaries of both the pre-estimations, the primary estimations and the post-estimations tests.
The evidence from indicates that the expected values of LCF, green-technology, energy-transition, resources rents and economic growth in South Africa are 0.418, 9.964, 2.308, 5.491 and 2.308, respectively. Furthermore, the standard deviation indicates a closer spread among series except for GTech that is widely dispersed (3.350). LCF, GTech and NR series have positive tails, whereas ETran and EGrt have negative tails. All the enlisted series have thinner tails except NR, with fatter tail. This made the variable an outlier among other series. The Jarque-Bera statistic also revealed that NR deviates from the normal distribution, while other series are normally distributed. An abnormally distributed series eminently suggests the chances of nonlinearity among the series. In this case, the study probed whether the variables are linear or otherwise. Based on the evidence captured in the BDS test (), it is evident that all the enlisted series are nonlinear. This evidence holds across all the dimensions of the BDS test. Since this is the case, applying rigorous estimation techniques to such datasets is of the essence.
Table 1. The summary statistics and linearity test.
The study also subjected the relevant series to correlation and multicollinearity tests (). These tests are essential to ascertain the correlation strength between the coefficients of the explanatory and explained variables. The tests are also essential to ascertain the chances of multicollinearity among the predicting series. In this case, GTech, and ETran are positively and significantly correlated with LCF, whereas EGrt is negatively and significantly correlated with LCF. On the other hand, NR has a positive but insignificant correlation with LCF. allays the chances of multicollinearity among the explanatory variables since all the VIF coefficients are within the acceptable threshold of 3 (Everitt and Skrondal Citation2010; Uche, Das, and Bera Citation2023).
Table 2. Correlation matrix and variance inflation factors (VIF) test results.
Unarguably, economic series follows a random walk procedure that emanates from economic cycle. Invariably, economic series surfer considerable challenges like structural breaks arising from shocks like the recent COVID-19. On this basis, the enlisted series are subjected to stationarity tests via both linear (ADF) and nonlinear (Zivot-Andrews) unit-root test procedures (). Accordingly, the ADF tests revealed that while some series (GTech, ETran and EGrt) are stationary, others (LCF and NR) are differenced stationary series. However, the nonlinear procedure revealed that while LCF and GTech are level stationary series, ETran, NR, and EGrt are first differenced stationary series. The table also revealed the influence of structural breaks on all the series over different time ranges. Implicatively, the attributes of these series presuppose the application of the ARDL-related techniques, essentially the time-variant (QARDL) version.
Table 3. Stationarity test results.
An essential step in the ARDL estimation procedure is the evaluation of the potential long-term coevolution of all selected datasets. For an ARDL procedure, the bounds test cointegration test remains the most acceptable option. The available evidence () suggests the rejection of the null hypothesis of no long-run cointegration among the series since the F-statistics (4.282) is greater-than the upper-bounds limits (3.49) at 95% confidence interval. This entails that all the enlisted series share a common long-term trend and therefore cointegrated.
Table 4. Bounds test cointegration results.
The outcomes of both the ARDL and the QARDL estimates are summarised in . Accordingly, the upper component elicits the explanatory variables’ effects on LCF in South Africa based on ARDL estimates. In contrast, the lower component elicits the outcomes of the QARDL technique. Accordingly, the traditional ARDL technique estimates demonstrate that green (GTech) failed to stimulate ecological load capacity in South Africa during the period investigated. The result revealed an insignificant long and short-run negative association between GTech and Lcf. This implies that such technology has not effectively improved environmental quality in RSA. The observed unimpressive effects of GTech on Lcf in RSA reflect the embryonic state of such technology in the republic. Such evidence, as explained in prior studies like Rout, Gupta, and Sahoo (Citation2022) and Uche et al. (Citation2023), indicates that South Africa still needs to develop GTech capable of improving its ecological load capacity.
Table 5. ARDL and QARDL estimates.
Accordingly, the ARDL technique revealed that RSA is currently reaping the benefits of energy transition (ETran). The observed long-run significant positive association between ETran and Lcf demonstrates as follows: Specifically, the load capacity factor improves by 0.024%, given a one-percent increase in renewable energy consumption. Such soothing effect, as illustrated in prior investigations (Samour et al. Citation2023; Ullah et al. Citation2023), suggests more commitments from policymakers towards ensuring an unhindered transition to clean energy. Hence this is done, South Africa's LCF would improve and become more sustainable.
Furthermore, the estimates of the ARDL indicate that whereas resource rent is an insignificant positive predictor of LCF, economic growth produced significant mitigating effects in both the long and short run. This outcome indicates that NR utilisation in RSA has failed to improve LCF significantly. Moreover, it indicates a situation where clean environment is traded for economic expansions. By corroborating the submissions of Guloglu, Caglar, and Pata (Citation2023), the outcome indicates that a strategically coordinated use of natural resources has the potential to improve environmental quality in South Africa. However, efforts must be exercised to curtail a potential ecological load capacity factor damage given the notable short-term negative effects of natural resource rent.
Following the approach of Narayan & Narayan (Citation2010) and insights from Pata and Isik (Citation2021), we reject the EKC hypothesis in the case of RSA since the long-term elasticity is more harmful than the short-term elasticity. Consistent with this inference, the load capacity curve (LCC) hypothesis does not hold for RSA. Furthermore, in tandem with the submissions of some extant literature (Byaro, Nkonoki, and Mafwolo, Citation2023), the republic must put lasting control measures pertaining to resource consumption policies if the country is desirious to launch into its expected status by 2030. Most importantly, policymakers must ensure that issues that border on environmental health should be considered alongside economic expansion propagation. Such steps, in line with ‘our future make it work’ agenda, will make the republic more prosperous and greener.
As earlier stated, the study subjected the relevant series to further evaluation via a nonlinear and time-variant technique (QARDL). Unarguably, the characteristics of the enlisted series informed the choice of such a time-variant model. The QARDL technique produced several interesting outcomes relevant to policy considerations. For instance, the estimates illustrated that in the short term, GTech minifies Lcf significantly, particularly at the upper quantiles (q0.05 – q0.1), while it produced insignificant and inconsistent effects thereafter. However, there is evidence of long-term ecological load capacity factor improvement. Specifically, GTech ensured marginal (0.003%) significant improvements in Lcf only at the uppermost quantile (q0.05), whereas it provided largely insignificant positive effects afterwards with the exceptions of (q0.4 – q0.8), where insignificant negative relationships ensued. Based on this outcome, it can be argued that GTech is a positive predictor of load capacity factor in RSA. However, it is imperative to underline that the observed soothing effects are still at an embryonic stage. To this end, it is suggested that environmental technologies must be improved. This will enable the republic reap their full benefits. Therefore, the study rejects the null hypothesis to state that GTech is a positive predictor of environmental vitality in SA.
Consistent with the ARDL, the estimates of the QARDL procedure revealed that the transition to clean energy is a significant positive predictor of Lcf in RSA at all times. In particular, at the upper quantiles (q0.05 - q0.1), Lcf improves given a 1% short-term increase in clean energy adoptions. Beyond these quantiles, its effects remained positive but insignificant except at the lower quantiles (q0.7 – q0.95), where its effects became negative yet insignificant. The positive association between ETran and Lcf is also recorded in the long run, particularly at the middle-quantiles (q0.5 – q0.6). The effect of energy transition remained positive but insignificant at other quantiles except towards the lower quantile (q0.8), where an insignificant negative association was observed.
It is pertinent to highlight that energy-transition improved Lcf much more in the short rather than in the long run. Therefore, in line with the earlier submissions, we affirm that energy-transition is a significant positive predictor of Lcf in RSA. However, more commitments are required to facilitate unhindered transition and all-inclusive adoption of clean energy in the republic. In particular, the ongoing Energy Performance Certificate (EPC) verification that seeks to verify the commitments of property owners towards the zero-emission building standards by 2030 is a step in the right direction. As such, everyone is expected to support the programme to enable the republic realise the objectives of the development plan.
Additionally, available evidence precluded a harmonious and favourable disposition between resources rent and Lcf in RSA. Accordingly, resource rent ensured significant improvements in Lcf, particularly at the upper quantiles and towards the middle quantiles (q0.05–q0.4). NR produced insignificant positive effects thereafter and became unfavourable at the lower quantiles (q0.8–q0.9). The short-term effects also underscored the positive yet insignificant association between NR and Lcf except at some upper quantiles (q0.30–q0.40) and lowest quantile (q0.95), where it produced insignificant adverse effects. Considering this outcome, it is essential to comment that the republic has conducted its natural resource utilisation in such a way that it improved the environment at some quantiles.
However, the observed adverse effects over some quantiles suggest that its effects are only partially favourable. In this case, the republic is expected to provide more robust policy guidelines towards natural resources exploration to avoid its unpleasant effects recorded at the upper quantiles. Beyond the guidelines, efficient monitoring mechanisms must be implemented to ensure maximum compliance at all times. In this context, the null hypothesis is rejected in favour of the alternative. It is pertinent to highlight that the submissions of Caglar et al. (2023) and Guloglu, Caglar, and Pata (Citation2023) in the case of G5 and 26 OECD countries resonate with this finding. Therefore, resource utilisation is a significant positive predictor of Lcf in South Africa.
Unlike the traditional ARDL procedure, the estimates of the QARDL model demonstrated long-term significant Lcf improvements amidst economic growth. In this context, economic growth produced a notable soothing effect across the distributions of Lcf. In particular, its favourable effects were more prominent at the upper quantiles (q0.05 – q0.3), less prominent at the middle quantiles (q0.4 – q0.7) and insignificant at the lower quantiles (q80 – q95). On the flip side, its short-term effects are mainly unfavourable and insignificant across all the distributions of Lcf except at the 90th quantile (q90), where it produced an insignificant favourable effect. This outcome portrays some favourable turn of events that fizzles out over the distributions. For instance, average elasticity of 0.06% and 0.04% were recorded at the upper and middle quantiles, respectively.
Meanwhile, it produced insignificant positive effects at the lower quantiles. This discovery highlights a potentially unsustainable relationship between growth and Lcf in South Africa. This evidence suggests the urgency to ensure that environmental quality is not traded for economic prosperity. Hence such is not adhered to; the republic will invariably bear the negative consequences of a compromised ecosystem.
4.1. Post estimation evaluations
The outcomes of both models, ARDL and QARDL, were subjected to series of post-estimation validity tests. The results of these post-estimation validity tests are summarised in and and .
Table 6. Post-estimation/Parameter constancy tests
The evidence portrayed in the upper panel of , including the normality test, serial correlation test, constant variance test, and specification test, affirmed the validity of the empirical results. Likewise, the stability tests ( and ) demonstrate that the models are stable over time. In the case of parameter constancy tests, the middle and lower panels of the table revealed that the series is homogeneous over time and across the distributions of the explained variable. An exception to this is the presence of heterogeneity in the case of short-run effects of energy transition () on Lcf. Given the potential influence of economic cycles and structural breaks, this entails the desirability of constant policy monitoring and moderation across the distributions. Forthwith, the empirical estimates and the accompanying policy prescriptions are valid for policy moderation that would ensure improved ecological load capacity factor and ecological balance in RSA.
5. Conclusions and policy insights
The RSA is committed to staying on top of everything in the fight against climate change and its associated global warming. In this study, efforts were committed to unravelling the potential influence of green-innovation, energy transition, and resource rent on Lcf in the case of RSA. The load capacity factor is a comprehensive environmental quality indicator that unveils the peculiarities of the demand and supply sides of environmental performance. Unfortunately, such essential information does not exist in the context of an important emerging economy like RSA. There are a handful of studies within the context of developing countries and a few emerging economies. Very few considered the BRICS economics but failed to consider load capacity factor.
To put the records straight and update the literature trajectory, this study explored the dimensions of the green-technology, energy-transition and resource rents towards improving Lcf in South Africa. For this purpose, the study galvanised annual data sets of these variables, spanning 1970–2018 for empirical evaluation.
In addition, unlike existing studies, we adopted linear (ARDL) and nonlinear (QARDL) estimation procedures for empirical evaluation. Likewise, other essential pre and post-estimation tests were activated to give critical expressions to the veracity of the empirical results. Among the pre-evaluation tests, the bounds test conveyed long-term coevolution among the variables. The empirical estimates of the ARDL exposed that GTech failed to improve Lcf in RSA, whereas the QARDL informed that GTech could improve Lcf only at the upper quantile. Likewise, both estimates confirmed the improving effects of energy-transition. However, such a feat was achieved only at the middle quantiles in the case of QARDL.
On the other hand, the ARDL portrayed resource rent as a positive but insignificant predictor of Lcf. In contrast, the QARDL demonstrated that resources rest improved Lcf significantly, mainly at the upper quantiles. However, resource rent produced harmful effects at the lower quantiles of the distributions. The control variable (GDP) remains a concern for environmental sustainability since it minifies Lcf in both the short and long run based on the estimates of the ARDL procedure. However, the estimates of the QARDL revealed that despite its short-term environmental quality-dampening effects, GDP engendered some Lcf soothing effects, particularly at the upper and middle quantiles of the distributions. This suggests some potential and possible positive turn up of events.
What is the policy direction given these varied outcomes? It is pertinent to remark that robust and holistic empirical revelation such as this provides far-reaching policy implications than others. In particular, the unimpressive influence of green-technology can be reversed to an all-time appealing effect since it provided a significant but marginal improving effect at the upper quantile. More investments and commitment are essential to activate the improving effects of this unique technology that has ensured improved environments in some other economies. Admittedly, energy transition offered notable soothing effects, yet it could not offer all-time soothing effects in upper and lower quantiles. In this case, the republic must be more committed to realising a full-scale transition to clean energy. RSA should be more emphatic about the switch to electric vehicles (EVs) like other emerging countries such as China. Moreover, there is a need to review, readjust and realign the prevailing energy and environmental taxes. The ongoing energy performance certification (EPC) programme is commendable and should be pushed with more vigour to achieve the expected results.
The relationship between resource rent also demands further strengthening and adjustments towards the realisation of improved Lcf. This is because resources rent improved environmental quality only at the upper quantiles while it remained insignificant and harmful at some quantiles of the distributions. It would not be out of place to invoke more stringent control measures in the utilisation and explorations of natural resources in the republic. This could entail the imposition of higher tax rates on resources explorations. To this end, more investment should be made on advance technologies to ensure cleaner environments. Most importantly, the republic must ensure that the quest for economic expansions does not overshadow the sustainability of its environmental ecosystem at any time. This is particularly important since it produced some notable unimpressive effects on Lcf in the case of ARDL and QARDL procedures.
At this point, it is expedient to state that all the steps taken in this study are confined within its stated objectives and focus. Indeed, the study may not have identified some other fundamental determinants of environmental performance. Particularly, the study is confined within the peculiarity of South Africa. On this realisation, we encourage the extension of this study by enlisting other determinants to confirm their effects on Lcf. Likewise, other emerging countries should be considered for more empirical insights since only a few were considered in extant studies.
Disclosure statement
No potential conflict of interest was reported by the author(s).
Notes
References
- Adebayo, T.S., and D. Kirikkaleli. 2021. “Impact of Renewable Energy Consumption, Globalisation, and Technological Innovation on Environmental Degradation in Japan: Application of Wavelet Tools.” Environment, Development and Sustainability 23 (11): 16057–16082. https://doi.org/10.1007/s10668-021-01322-2.
- Adebayo, T.S., and A. Samour. 2023. “Renewable Energy, Fiscal Policy and Load Capacity Factor in BRICS Countries: Novel Findings from Panel Nonlinear ARDL Model.” Environment, Development and Sustainability. https://doi.org/10.1007/s10668-022-02888-1.
- Akadiri, S. Saint, T.S. Adebayo, J.S. Riti, A.A. Awosusi, and E.M. Inusa. 2022. “The Effect of Financial Globalisation and Natural Resource Rent on Load Capacity Factor in India: An Analysis Using the Dual Adjustment Approach.” Environmental Science and Pollution Research 29 (59): 89045–89062. https://doi.org/10.1007/s11356-022-22012-0.
- Alola, A.A., O. Özkan, and O. Usman. 2023. “Role of Non-Renewable Energy Efficiency and Renewable Energy in Driving Environmental Sustainability in India: Evidence from the Load Capacity Factor Hypothesis.” Energies 16 (6): 2847. https://doi.org/10.3390/en16062847.
- Awosusi, A.A., K. Kutlay, M. Altuntaş, B. Khodjiev, E.B. Agyekum, M. Shouran, M. Elgbaily, and S. Kamel. 2022. “A Roadmap Toward Achieving Sustainable Environment: Evaluating the Impact of Technological Innovation and Globalization on Load Capacity Factor.” International Journal of Environmental Research and Public Health 19: 3288. https://doi.org/10.3390/ijerph19063288.
- Byaro, M., J. Nkonoki, and G. Mafwolo. 2023. “Exploring the Nexus Between Natural Resource Depletion, Renewable Energy use, and Environmental Degradation in sub-Saharan Africa.” Environmental Science and Pollution Research 30 (8): 19931–19945. https://doi.org/10.1007/s11356-022-23104-7.
- Cho, J.S., T.H. Kim, and Y. Shin. 2015. “Quantile Cointegration in the Autoregressive Distributed-lag Modeling Framework.” Journal of Econometrics 188 (1): 281–300. https://doi.org/10.1016/j.jeconom.2015.05.003.
- Destek, M.A., and M. Manga. 2021. “Technological Innovation, Financialisation, and Ecological Footprint: Evidence from BEM Economies.” Environmental Science and Pollution Research 28 (17): 21991–22001. https://doi.org/10.1007/s11356-020-11845-2.
- Dogan, A., and U.K. Pata. 2022. “The Role of ICT, R&D Spending and Renewable Energy Consumption on Environmental Quality: Testing the LCC Hypothesis for G7 Countries.” Journal of Cleaner Production 380: 135038. https://doi.org/10.1016/j.jclepro.2022.135038.
- Everitt, B.S., and A. Skrondal. 2010. The Cambridge Dictionary of Statistics. Cambridge: Cambridge University Press.
- GFN. 2023. Global Footprint Network. Accessed May 2, 2023. https://www.footprintnetwork.org/our-work/ecological-footprint.
- Guloglu, B., E.A. Caglar, and U.K. Pata. 2023. “Analysing the Determinants of the Load Capacity Factor in OECD Countries: Evidence from Advanced Quantile Panel Data Methods.” Gondwana Research 118: 92–104. https://doi.org/10.1016/j.gr.2023.02.013.
- Hashmi, S.M., B.H. Chang, L. Huang, and E. Uche. 2022. “Revisiting the Relationship Between oil Prices, Exchange Rate, and Stock Prices: An Application of Quantile ARDL Model.” Resources Policy 75: 102543. https://doi.org/10.1016/j.resourpol.2021.102543.
- Huilan, W., S. Saint Akadiri, I. Haouas, A.A. Awosusi, and A.T. Odu. 2022. “Impact of Trade Liberalization and Renewable Energy on Load Capacity Factor: Evidence from Novel Dual Adjustment Approach.” Energy & Environment, https://doi.org/10.1177/0958305X221137559.
- Iorember, P.T., G. Jelilov, O. Usman, A. Işık, and B. Celik. 2021. “The Influence of Renewable Energy use, Human Capital, and Trade on Environmental Quality in South Africa: Multiple Structural Breaks Cointegration Approach.” Environmental Science and Pollution Research 28 (11): 13162–13174. https://doi.org/10.1007/s11356-020-11370-2.
- Karimi Alavijeh, N., M.T. Ahmadi Shadmehri, N. Nazeer, S. Zangoei, and F. Dehdar. 2023. “The Role of Renewable Energy Consumption on Environmental Degradation in EU Countries: Do Institutional Quality, Technological Innovation, and GDP Matter?” Environmental Science and Pollution Research, 30: 44607–44624. https://doi.org/10.1007/s11356-023-25428-4.
- Khan, I., F. Hou, and H.P. Le. 2021. “The Impact of Natural Resources, Energy Consumption, and Population Growth on Environmental Quality: Fresh Evidence from the United States of America.” Science of the Total Environment 754: 142222. https://doi.org/10.1016/j.scitotenv.2020.142222.
- Khan, U., A.M. Khan, M.S. Khan, P. Ahmed, A. Haque, and R.A. Parvin. 2023. “Are the Impacts of Renewable Energy use on Load Capacity Factors Homogeneous for Developed and Developing Nations? Evidence from the G7 and E7 Nations.” Environmental Science and Pollution Research 30 (9): 24629–24640. https://doi.org/10.1007/s11356-022-24002-8.
- Kihombo, S., Z. Ahmed, S. Chen, T.S. Adebayo, and D. Kirikkaleli. 2021. “Linking Financial Development, Economic Growth, and Ecological Footprint: What is the Role of Technological Innovation?” Environmental Science and Pollution Research 28: 61235–61245. https://doi.org/10.1007/s11356-021-14993-1.
- Kuznets, S. 2019. “Economic Growth and Income Inequality.” In In the gap Between Rich and Poor, edited by M.A. Seligson, 25–37. Routledge: Abingdon-on-Thames.
- Liu, X., V.O. Olanrewaju, E.B. Agyekum, M.F. El-Naggar, M.M. Alrashed, and S. Kamel. 2022. “Determinants of Load Capacity Factor in an Emerging Economy: The Role of Green Energy Consumption and Technological Innovation.” Frontiers in Environmental Science 10. https://doi.org/10.3389/fenvs.2022.1028161.
- Narayan, P.K., and S. Narayan. 2010. “Carbon dioxide emissions and economic growth: Panel data evidence from developing countries.” Energy Policy 38 (1): 661–666. https://doi.org/10.1016/j.enpol.2009.09.005
- Nathaniel, S.P., F.V. Bekun, and A. Faizulayev. 2021. “Modelling the Impact of Energy Consumption, Natural Resources, and Urbanisation on Ecological Footprint in South Africa: Assessing the Moderating Role of Human Capital.” International Journal of Energy Economics and Policy 11 (3): 130–139. https://doi.org/10.32479/ijeep.11099.
- Ni, Z., J. Yang, and A. Razzaq. 2022. “How do Natural Resources, Digitalization, and Institutional Governance Contribute to Ecological Sustainability Through Load Capacity Factors in Highly Resource-Consuming Economies?” Resources Policy 79: 103068. https://doi.org/10.1016/j.resourpol.2022.103068.
- Obiakor, R.T., E. Uche, and N. Das. 2022. “Is Structural Innovativeness a Panacea for Healthier Environments? Evidence from Developing Countries.” Technology in Society 70: 102033. https://doi.org/10.1016/j.techsoc.2022.102033.
- Omoke, P.C., and E. Uche. 2021. “How Does Purchasing Power in OPEC Countries Respond to oil Price Periodic Shocks? Fresh Evidence from Quantile ARDL Specification.” OPEC Energy Review 45 (4): 438–461. https://doi.org/10.1111/opec.12216.
- Opuala-Charles, S., P.C. Omoke, and E. Uche. 2022. “The Dynamic Role of Financial Development in Analyzing the N-Shaped Tourism-Led Growth Hypothesis in Mauritius.” Journal of the Knowledge Economy, https://doi.org/10.1007/s13132-022-01048-0.
- Opuala, C.S., P.C. Omoke, and E. Uche. 2023. “Sustainable Environment in West Africa: The Roles of Financial Development, Energy Consumption, Trade Openness, Urbanization and Natural Resource Depletion.” International Journal of Environmental Science and Technology 20: 423–436. https://doi.org/10.1007/s13762-022-04019-9.
- Pata, U.K., and C. Isik. 2021. “Determinants of the Load Capacity Factor in China: A Novel Dynamic ARDL Approach for Ecological Footprint Accounting.” Resources Policy 74: 102313. https://doi.org/10.1016/j.resourpol.2021.102313.
- Pata, U.K., and M.T. Kartal. 2023. “Impact of Nuclear and Renewable Energy Sources on Environment Quality: Testing the EKC and LCC Hypotheses for South Korea.” Nuclear Engineering and Technology 55 (2): 587–594. https://doi.org/10.1016/j.net.2022.10.027.
- Pata, U.K., M.T. Kartal, M.M. Dam, and F. Kaya. 2023. “Navigating the Impact of Renewable Energy, Trade Openness, Income, and Globalization on Load Capacity Factor: The Case of Latin American and Caribbean (LAC) Countries.” International Journal of Energy Research 2023: 1–14. https://doi.org/10.1155/2023/6828781.
- Pata, U.K., and A. Samour. 2022. “Do Renewable and Nuclear Energy Enhance Environmental Quality in France? A new EKC Approach with the Load Capacity Factor.” Progress in Nuclear Energy 149: 104249. https://doi.org/10.1016/j.pnucene.2022.104249.
- Pesaran, M.H., and Y. Shin. 1999. “Autoregressive Distributed Lag Modeling Approach to Cointegration Analysis.” In Econometrics and Economic Theory in the 20th Century: The Ragnar Frisch Centennial Symposium, edited by S. Storm, 371–413. Cambridge: Cambridge University Press. chapter 1.
- Pesaran, M.H., Y. Shin, and R.J. Smith. 2001. “Bounds Testing Approaches to the Analysis of Level Relationships.” Journal of Applied Econometrics 16 (3): 289–326. https://doi.org/10.1002/jae.616.
- Rout, S.K., M. Gupta, and M. Sahoo. 2022. “The Role of Technological Innovation and Diffusion, Energy Consumption and Financial Development in Affecting Ecological Footprint in BRICS: An Empirical Analysis.” Environmental Science and Pollution Research 29 (17): 25318–25335. https://doi.org/10.1007/s11356-021-17734-6.
- Samour, A., T.S. Adebayo, E.B. Agyekum, B. Khan, and S. Kamel. 2023. “Insights from BRICS-T Economies on the Impact of Human Capital and Renewable Electricity Consumption on Environmental Quality.” Scientific Reports 13 (1): 5245. https://doi.org/10.1038/s41598-023-32134-1.
- Siche, R., L. Pereira, F. Agostinho, and E. Ortega. 2010. “Convergence of Ecological Footprint and Emergy Analysis as a Sustainability Indicator of Countries: Peru as Case Study.” Communications in Nonlinear Science and Numerical Simulation 15 (10): 3182–3192. https://doi.org/10.1016/j.cnsns.2009.10.027.
- Su, Z.W., M. Umar, D. Kirikkaleli, and T.S. Adebayo. 2021. “Role of Political Risk to Achieve Carbon Neutrality: Evidence from Brazil.” Journal of Environmental Management 298: 113463. https://doi.org/10.1016/j.jenvman.2021.113463.
- Uche, E. 2022. “Strategic Pathways to Combating Remittance-Induced Carbon Emissions; the Imperatives of Renewable Energy, Structural Transformations, Urbanisation and Human Development.” Energy Sources, Part B: Economics, Planning, and Policy 17 (1), https://doi.org/10.1080/15567249.2022.2141375.
- Uche, E., N. Das, and P. Bera. 2023. “Re-examining the Environmental Kuznets Curve (EKC) for India via the Multiple Threshold NARDL Procedure.” Environmental Science and Pollution Research 30 (5): 11913–11925. https://doi.org/10.1007/s11356-022-22912-1.
- Uche, E., N. Das, P. Bera, and J. Cifuentes-Faura. 2023. “Understanding the Imperativeness of Environmental-Related Technological Innovations in the FDI – Environmental Performance Nexus.” Renewable Energy 206: 285–294. https://doi.org/10.1016/j.renene.2023.02.060.
- Uche, E., N. Das, N.C. Nwaeze, and P. Bera. 2022. “Navigating the Paths to Sustainable Environments via Energy Security, Renewable Energy and Economic Complexity: Evidence from Array of Pollution Metrics.” Energy & Environment. https://doi.org/10.1177/0958305X221140571.
- Uche, E., and L. Effiom. 2021a. “Fighting Capital Flight in Nigeria: Have we Considered Global Uncertainties and Exchange Rate Volatilities? Fresh Insights via Quantile ARDL Model.” SN Business & Economics 1), https://doi.org/10.1007/s43546-021-00082-5.
- Uche, E., and L. Effiom. 2021b. “Financial Development and Environmental Sustainability in Nigeria: Fresh Insights from Multiple Threshold Nonlinear ARDL Model.” Environmental Science and Pollution Research 28 (29): 39524–39539. https://doi.org/10.1007/s11356-021-12843-8.
- Udeagha, M.C., and E. Muchapondwa. 2022. “Investigating the Moderating Role of Economic Policy Uncertainty in Environmental Kuznets Curve for South Africa: Evidence from the Novel Dynamic ARDL Simulations Approach.” Environmental Science and Pollution Research 29 (51): 77199–77237. https://doi.org/10.1007/s11356-022-21107-y.
- Ullah, S., R. Luo, T.S. Adebayo, and M.T. Kartal. 2023. “Paving the Ways Toward Sustainable Development: the Asymmetric Effect of Economic Complexity, Renewable Electricity, and Foreign Direct Investment on the Environmental Sustainability in BRICS-T.” Environment, Development and Sustainability, https://doi.org/10.1007/s10668-023-03085-4.
- Usman, M., and N. Hammar. 2021. “Dynamic Relationship Between Technological Innovations, Financial Development, Renewable Energy, and Ecological Footprint: Fresh Insights Based on the STIRPAT Model for Asia Pacific Economic Cooperation Countries.” Environmental Science and Pollution Research 28 (12): 15519–15536. https://doi.org/10.1007/s11356-020-11640-z.
- Xu, D., S. Salem, A.A. Awosusi, G. Abdurakhmanova, M. Altuntaş, D. Oluwajana, D. Kirikkaleli, and O. Ojekemi. 2022. “Load Capacity Factor and Financial Globalization in Brazil: The Role of Renewable Energy and Urbanization.” Frontiers in Environmental Science 9. https://doi.org/10.3389/fenvs.2021.823185.
- Yang, B., A. Jahanger, and M. Ali. 2021. “Remittance Inflows Affect the Ecological Footprint in BICS Countries: Do Technological Innovation and Financial Development Matter?” Environmental Science and Pollution Research 28 (18): 23482–23500. https://doi.org/10.1007/s11356-021-12400-3.
- Zafar, M.W., A. Saeed, S.A.H. Zaidi, and A. Waheed. 2021. “The Linkages among Natural Resources, Renewable Energy Consumption, and Environmental Quality: A Path Toward Sustainable Development.” Sustainable Development 29 (2): 353–362. https://doi.org/10.1002/sd.2151.
- Zhang, L., D.I. Godil, M. Bibi, M.K. Khan, S. Sarwat, and M.K. Anser. 2021. “Caring for the Environment: How Human Capital, Natural Resources, and Economic Growth Interact with Environmental Degradation in Pakistan? A Dynamic ARDL Approach.” Science of the Total Environment 774: 145553. https://doi.org/10.1016/j.scitotenv.2021.145553.