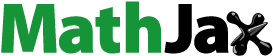
ABSTRACT
In pursuit of net-zero greenhouse gases emissions goals, Nigeria faces challenges like fossil fuel dependence, environmental degradation and energy inefficiency, necessitating a transition to decarbonization. This study employs the Bass model and TOPSIS multi-criteria analysis to investigate the diffusion and ranking of low/zero-carbon technologies for Nigeria. Findings reveal: absence of key mitigation technologies like wind, concentrating solar plant (CSP), geothermal, nuclear, hydrogen, fuel cell, carbon capture utilization and sequestration (CCUS), direct air capture (DAC), and lime soda in Nigeria; that over a fifty-year span from year 2023, existing technologies–solar photovoltaic, bioenergy, natural gas and hydro–in Nigeria indicate slow adoption rates (3.68–106.02MW) based on Nigeria trajectory of technology use, contrasting with significantly accelerated rates (276.06–90,320MW) based on global trajectory; and multi-criteria analysis favors hydro, solar photovoltaic, natural gas, bioenergy, and wind as favorable options, while suggesting further exploration of hydrogen, fuel cell, geothermal, and nuclear technologies within Nigeria's energy landscape.
1. Introduction
The impact of climate change can be viewed as a complex and multifaceted global challenge affecting ecological, environmental, sociopolitical, and socioeconomic domains (Filho et al. Citation2021; Feliciano et al. Citation2022; Abbass et al. Citation2022). Due to its significant effects on the Earth's ecosystems and human societies, global climate change is an urgent topic that has drawn considerable attention. Human activities are primarily responsible for significant shifts in the Earth's climate system. As a result, climate change's consequences are becoming ever more evident and alarming. A key aspect of its characterization is assessing the long-term patterns of temperature and precipitation, along with factors such as atmospheric pressure and humidity (Abbass et al. Citation2022). Climate change has been linked to a number of escalating effects and hazards, such as increased storm activity, depleted ecosystems, and unprecedented heatwaves. Aside from irregular weather patterns, global ice sheets melt which causing sea levels to rise, are globally recognized and domestically observed consequences of climate change (Lipczynska-Kochany Citation2018; Murshed and Dao Citation2022). Although once considered bearable, the rise of global mean surface temperature (GMST) by 2.0°C or more compared to the pre-industrial era is now considered unmanageable and dangerous to both natural and human systems (Hoegh-Guldberg et al. Citation2019). With global climate change posing more challenges than ever before, cutting carbon dioxide emissions is becoming urgent worldwide (Murshed and Dao Citation2022).
Global efforts toward decarbonization have significantly accelerated in response to the growing worries about climate change and its negative consequences on the environment. Decarbonization, which can be describes as the reduction of greenhouse gases (GHGs) and the transition to cleaner and more sustainable energy sources (Virta Citation2023; The Welding Institute Citation2023), has become a paramount objective for governments, organizations, and societies worldwide. The Paris Climate Agreement is a major and the most recent effort to promote international cooperation on climate change. This agreement, which 185 countries have signed or joined, intends to bring nations together voluntarily to take significant steps to reduce climate change. In addition, it highlights how crucial it is to ensure that the essential resources are available for implementation, such as climate finance, as well as the need of developing adaption approaches and strategies (Hoegh-Guldberg et al. Citation2019). According to reports, the energy industry will need to make a large financial investment if global warming is to be limited to 1.5°C. In particular, between 2016 and 2050, it is projected that investments in energy supply measures will range from $1.46 trillion to $3.51 trillion (in US dollars 2010), while investments in energy demand measures will range from $640 billion to $910 billion. The achievement of the goal of net zero greenhouse gas emissions by 2050 depends on these expenditures (Intergovernmental Panel on Climate Change Citation2019). A number of global efforts towards decarbonization have been identified through literature, underscoring the collective commitments of the global community to decarbonization and addressing the challenges of climate change. These initiatives cover a wide range of actions and strategies such as the following (Krishnan et al. Citation2023; International Energy Agency Citation2021; Rissman et al. Citation2020; Cames et al. Citation2021; Chen et al. Citation2022): (i) Encouraging the switch from fossil fuels to electricity with zero emissions and other low emission energy sources, such as hydrogen, in order to change the energy mix (ii) Implementing decarbonization measures in industrial and agricultural processes; (iii) Prioritizing energy efficiency and efficient demand management; (iv) Implementing circular economy principles for sustainable emissions reduction; (v) Consuming fewer emission-intensive goods; (vi) Recognizing the necessity of significant investments in clean energy; in order to achieve net-zero emissions by 2050, the annual global investment in clean energy must triple to approximately $4 trillion by 2030; (vii) Emphasizing rapid deployment of existing technologies and the development of new technologies to align with the net-zero target; (viii) The emergence of a growing coalition of countries, cities, businesses, and other institutions that are steadfast in their commitment to achieving net-zero emissions; (ix) Formulation of national climate action plans that emphasize emission reductions and climate change adaptation; and (x) implementing measures to reduce emissions in the power and transportation sectors, such as boosting the use of renewable energy sources, aiming for zero-emission vehicles by 2030, and pushing for the decarbonization of global shipping.
Technology significantly contributes to decarbonization by offering innovative solutions for reducing greenhouse gas emissions and transitioning to sustainable energy systems (Wang et al. Citation2021). Renewable energy is one important area where technology plays a critical role (Sen and Ganguly Citation2017; Vo et al. Citation2020). Decarbonization has been considerably aided by the development and application of renewable energy technologies like solar, wind, hydro, and geothermal power. With the use of these technologies, power can be produced with little or little emissions, lowering the need for fossil fuels and the energy sector's carbon footprint (Gielen et al. Citation2019; United Nations Citation2023). Energy storage technologies, like batteries, pumped hydro, and thermal storage, are crucial for decarbonization by bridging the gap between renewable energy production and demand, enhancing reliability and stability (Tan et al. Citation2021; Wang et al. Citation2022). Transport technology advancements are crucial for decarbonization in addition to energy-related ones. Alternative fuels such as hydrogen fuel cells, electric vehicles (EVs), and others provide cleaner, more environmentally friendly transportation options that help to reduce the need for fossil fuel-powered cars and the emissions they produce (Sandaka and Kumar Citation2023; Çabukoglu et al. Citation2019; Pustějovská, Janovská, and Jursová Citation2023). Additionally, data analytics and digital technologies are essential for enhancing energy management, maximizing energy systems, and facilitating well-informed decision-making. The efficiency and adaptability of the energy system are improved by the use of smart grids, Internet of Things (IoT) gadgets, and advanced data analytics to monitor energy usage, optimize energy distribution, and enable demand response programs (Li et al. Citation2022; Saleem et al. Citation2023; Sadeeq and Zeebaree Citation2021). Overall, technology acts as a catalyst for decarbonization by offering ways to cut emissions, boost energy efficiency, enable the integration of renewable energy, and push different sectors towards a sustainable, low-carbon future (Rissman et al. Citation2020; Wang et al. Citation2021).
Nigeria's energy landscape is predominantly fueled by fossil sources like oil and natural gas, historically serving power generation, transportation, and industrial needs. While Hydropower remains a primary renewable source, its capacity is limited, whereas solar is rapidly expanding, albeit still constituting a small share of the overall energy mix of the country. In comparison to the rest of the world, Nigeria's adoption of renewable energy has been relatively modest, with fossil fuels maintaining dominance (Olujobi et al. Citation2023). Globally, there is a noticeable shift towards a more diversified energy mix, with renewables (hydro, wind, solar) witnessing rapid growth (Hassan et al. Citation2023). Regional variations are evident with developed nations exhibiting higher renewable shares, while developing countries, like Nigeria, heavily rely on dirty fuels (e.g. fossil and crude biomass). Nigeria's renewable status is characterized as thus: (i) low and slow penetration – contributing less than 16.4% (with over 90% from hydro) to total electricity capacity as at 2022 (Statista Research Department Citation2024); (ii) ambitious policy goals – targeting 30% renewable electricity generation by 2030 (Ajala Citation2024) and net-zero emissions by 2060 (Ogbonna et al. Citation2023); and (iii) associated challenges like infrastructure gaps, financing hurdles, and grid integration issues that could hinder growth. On a global scale, renewables account for about one-third of electricity generation (Our World in Data Citation2024) and is rapidly growing. Nigeria's commitment to transitioning to a low-carbon economy is evident and emphasized in net zero pledge and a number of National climate change/decarbonization documents (Okoh and Okpanachi Citation2023). Abundant resources, a large population, and a rising demand for clean energy underscore the importance for Nigeria to explore its low carbon and clean energy potential. The recent passage of the Electricity Act 2023 marks a pivotal moment, ushering in an era focused on sustainable power sources and technological advancements in the realm of renewable energy (United Nations Conference on Trade and Development Citation2024).
Towards addressing climate change and transitioning to a low-carbon economy, Nigeria has been making efforts to decarbonize. Some key aspects of Nigeria's efforts to decarbonize can be seen in the area of pushing for renewable energy development. Nigeria recognizes the importance of renewable energy sources in decarbonization, such as solar, wind, biomass, and hydropower, and has been actively promoting initiatives like the Nigerian Renewable Energy and Energy Efficiency Project (NREEEP), and the Renewable Energy Master Plan (REMP) aimed at increasing the share of renewable energy in the country's energy mix. The goal of Nigeria's Renewable Energy Master Plan (REMP) is to gradually increase the share of renewable electricity in the country's overall energy mix. The plan establishes goals for renewable electricity generation, increasing from 13% in 2015 to 23% by 2025 and 36% by 2030. For different renewable energy sources, such as small hydro, solar PV, biomass-based power plants, and wind, the REMP also specifies capacity targets. The plan also emphasizes increasing electrification rates, with goals of 60% by 2015 and 75% by 2025, up from 42% in 2005. The REMP also includes a variety of financial and market incentives to assist the adoption of renewable energy (International Energy Agency Citation2023a; International Energy Agency Citation2023b).
The Nigerian government created the National Renewable Energy and Energy Efficiency Policy (NREEEP) to promote the use of renewable energy sources and improve energy efficiency across the country. By encouraging the sustainable use of renewable energy sources and putting energy-efficient practices in place, this strategy was created to address Nigeria's problems with the country's energy supply. The NREEEP seeks to accomplish a number of goals, including enhancing energy security, reducing greenhouse gas emissions, and promoting economic growth. The NREEEP aims to offer a planned and efficient solution to Nigeria's energy demands by increasing access to electricity, lowering energy poverty, and creating job opportunities in the renewable energy sector (Sengupta Citation2023; Federal Ministry of Power Citation2023).
Nigeria has expressly declared its commitment to attain net-zero greenhouse gas emissions by 2060, as expressed in various official documents and initiatives, aligning with President Muhammadu Buhari's explicit pledge at the 2021 Conference of Parties (COP26) in Glasgow (Ogbonna et al. Citation2023; Climate Action Tracker Citation2022). This initiative include the Climate Change Act enacted in 2021 that establishes a legal framework for climate action, articulating the overarching objective of attaining net-zero emissions within the timeframe of 2050–2070. The legislation mandates the formulation of National Climate Change Action Plans in five-year intervals, serving as comprehensive guides for the implementation of emissions reduction strategies (National Council on Climate Change Citation2024). Aside from addressing challenges in implementation, transparency, and financing (Ogbonna et al. Citation2023; Dioha Citation2023), the crucial task of simultaneously ensuring affordable and reliable energy access for all Nigerians while reducing emissions is a significant concern, necessitating careful consideration in the adoption of appropriate technologies.
Nigeria submitted its Nationally Determined Contribution (NDC) in accordance with the Paris Agreement, which specifies the nation's climate change mitigation and adaptation objectives, to demonstrate its efforts toward decarbonization. Nigeria's updated NDC targets to reduce economy-wide emissions conditionally by 47% and 20% unconditionally by 2030 (NDC Partnership Citation2023). It also commits to end flaring by 2030 and reduce fugitive methane emissions from oil and gas operations by 60% by 2031 (Climate Action Tracker Citation2023; International Energy Agency Citation2023c). In addition to mitigation targets, Nigeria's NDC highlights several key points. Firstly, it stresses the promotion of renewable energy sources such as solar, wind, hydro, and biomass to diversify the energy mix and lessen dependency on fossil fuels. Secondly, in recognition of Nigeria's sensitivity to climate change, the NDC also specifies adaptation methods such as enhancement of climate resilience in agriculture, water resource management, and urban infrastructure. Thirdly, it emphasizes the importance of halting deforestation and forest degradation. Lastly, the NDC addresses cross-cutting themes like gender, youth, capacity building, public awareness, and institutional strengthening, which are crucial for effective climate action and sustainable development (Federal Government of Nigeria Citation2023).
The Energy Transition Plan (ETP) is another indication of Nigeria's decarbonization efforts. The Nigeria Energy Transition Plan (ETP) is a strategic roadmap aimed at necessitating substantial emission reductions in five crucial sectors in order to achieve carbon neutrality by 2060. The plan outlines a framework and timeline for implementing emission reduction measures across the Power, Cooking, Oil and Gas, Transport, and Industry sectors, which according to the ETP collectively account for approximately 65% of Nigeria's emissions. The ETP's main goals are to reduce emissions by 65%, alleviate energy poverty, foster investment opportunities, and improve energy efficiency. The strategy offers major investment possibilities in the fields of solar energy, hydrogen, and electric cars, each of which is anticipated to result in the creation of net new jobs (Nigeria Energy Transition Plan Citation2023). Other climate-relevant greenhouse gas (GHG) emissions and energy modelling studies which illustrates Nigeria's efforts to decarbonize include the Long-Term Low Emissions Development Strategy (LT-LEDS), Deep Decarbonization Pathway Project (DDPP), Nigeria Energy Calculator 2050 (NECAL 2050), as well as the Integrated Resource Plan (IRP).
Furthermore, one critical question to be asked is ‘to what extent will technology be involved in the decarbonization effort in Nigeria?’ To address the question, the study aims to conduct technology diffusion and mapping of various low/zero carbon technologies to support Nigeria to achieve its climate change mitigation ambition. The aim of the study is anchored on the objectives to investigate diffusion patterns and adoption rates of low/zero-carbon technologies considering both currently existing and potential technologies in Nigeria, evaluated in terms of both Nigeria and global trajectory of technology use; and to conduct multi-criteria analysis to rank the technologies and offering strategic insight in identifying favorable technology options for Nigeria’s sustainable energy landscape. This research aspires to offer a comprehensive understanding of technology adoption trends thus serving as a foundational resource for policymakers, energy stakeholders, and climate advocates in Nigeria, ultimately accelerating the nation's transition to a low-carbon and sustainable future.
The paper comprises five key sections. First, the introduction sets the stage by reviewing climate change and decarbonization efforts worldwide and in Nigeria. The second section – methodology presents the analytical approach, i.e. utilizing the Bass model for technology diffusion and TOPSIS multi-criteria method for mapping. The third section is the results and discussion section, where the study's findings are presented and discussed in depth. Section four highlights critical findings and their implications. The paper concludes with section five, summarizing key takeaways and insights.
2. Methodology
In this section, the models used for a comprehensive analysis of technology diffusion and evaluation of low/zero carbon technologies are presented. The Bass model, a well-known framework for technology diffusion analysis, was used to analyze the diffusion characteristics of the highlighted technologies (Ratcliff and Doshi Citation2016; Turk and Trkman Citation2012). In addition, we use the Technique for Order Preference by Similarity to Ideal Solution (TOPSIS) method, a multi-criteria analysis approach, to rank the identified low/zero carbon technologies according to different criteria. TOPSIS, with its ability to handle quantitative data and consideration of nuanced criteria, including economic, technical, environmental, and social factors, aligns seamlessly with the complexity and breadth of this study’s dataset. This adaptability makes TOPSIS an ideal choice for robustly assessing and ranking the suitability of diverse low/zero carbon technologies for Nigeria's energy mix (Madanchian and Taherdoost Citation2023; Vavrek and Bečica Citation2022). The selection of the Bass model and TOPSIS method is driven by their proven effectiveness in examining technology diffusion patterns and facilitating decision-making processes through comprehensive multi-criteria evaluation. By combining these two analytical approaches, it provides a robust framework for understanding the diffusion dynamics and ranking of low/zero carbon technologies, thus contributing valuable insights to inform sustainable technology adoption strategies as it relates to Nigeria. The data collection process involved a combination of sources. To establish market potential (m) assumptions, demographic data, global average energy demand per capita and current energy and carbon statistics for Nigeria were gathered from reputable organizations. Information on the installed capacity of various energy generation technologies and carbon capture and removal technologies was sourced from international organizations, including the International Renewable Energy Agency (IRENA), International Atomic Energy Agency (IAEA), International Energy Agency (IEA), and others. The estimation of Bass model parameters (p, q) utilized least square regression to regress collated data, and this analysis was conducted within the integrated environment of Microsoft Excel. All other specific assumptions for the respective analyses are provided in their respective subsections. The flowchart () illustrates the comprehensive methodology employed in this article, outlining the systematic approach to technology diffusion analysis and multi-criteria evaluation for low/zero-carbon technologies in Nigeria's decarbonization process.
2.1 Technology diffusion analysis
In this subsection, the models employed to depict the technology diffusion patterns of the chosen low carbon technologies are presented. The process of technology adoption over time typically exhibits an exponential trend, particularly resembling the S-shaped curve. This distinctive curve is characterized by an initial phase of slow growth or delay, succeeded by a rapid acceleration once a critical point is reached. Subsequently, the growth rate gradually stabilizes, reaching a saturation point known as the carrying capacity. Finally, a sudden surge occurs, representing a significant leap in adoption. This S-curve finds greater relevance in simulating the adoption patterns of low-carbon and/or renewable energy technologies (Schilling and Esmundo Citation2009; Purohit and Kandpal Citation2005). In this study, the Bass diffusion model is adopted to accurately capture and represent this type of growth. The Bass diffusion model holds significance as the pioneering quantitative model for new product diffusion and remains one of the most frequently referenced and extensively analyzed models in its domain (Fibich and Gibori Citation2010).
For simplicity of analysis the following model assumptions were adopted:
The diffusion process is binary
or
, i.e. either adopt
or do not adopt
The adoption of the technology is influenced by two (2) fundamental types of behaviors – ‘innovation’ which is driven by influences such as advertising and ‘imitation’ which is influenced by word of mouth and/or interpersonal relationships;
Maximum number of adopters or market potential is constant over the diffusion process;
All potential adopters eventually purchase or adopt the technology /product;
Repeat and/or replacement purchases are not accounted for, thus one customer per product at each time ‘t’;
There are no supply limitation of the technology/product;
The diffusion process is not interrupted by the decisions and/or diffusion process of other innovations in circulation.
2.1.1 Mathematical formulations of the Bass model
The likelihood of adoption of a product/technology at time given that no prior purchase has yet been made based on the Bass model is described by (Bass Citation2004; Lilien, Rangaswamy, and Bruyn Citation2007; Mahajan, Muller, and Bass Citation1995)
(1)
(1)
(2)
(2) where
represents the probability density function which indicates the rate at which the probability of adoption changes with time
;
is the cumulative distribution function of adoption at time
, i.e. the cumulative probability that the target segment will adopt the technology by time
;
represents the coefficient of innovation or coefficient of external influence; and
is the coefficient of imitation or coefficient of internal influence.
Considering that
(3)
(3)
(4)
(4) Equation
was further manipulated to give:
(5)
(5)
(6)
(6)
(7)
(7) where
is the adoptions at time
;
is the cumulative adoptions at time
;
is the potential market size and/or ceiling; the term
and
in Equation
represents technology adoptions independent of the influence of previous users or buyers of the technology; and technology adoptions influenced by previous users or buyers of the technology, respectively.
Further manipulations of Equation assuming
yields the following S-shaped cumulative adopter distribution function
(8)
(8) For the S-shaped diffusion curve, expressions related to the peak point of adoption are given as:
(9)
(9) where
represents the time of peak adoption.
2.1.1.1 Estimating diffusion model parameters
In this work, a straightforward algebraic estimating approach as shown in this subsection, will be adopted to estimate the diffusion process parameters – coefficient of innovation , coefficient of imitation
and the market potential
, respectively.
First step: Adopt an analogy by aspects strategy – which is an approach to estimating the Bass model parameters, ,
and
from data of historical sales of the product/technology or an analogous products (Ganjeizadeh et al. Citation2017).
Second step: Using least square regression method regress the collated data to obtain quadratic polynomial in the form in Equation
(10)
(10) Third step: Equate Equation (13) to the Bass model relation presented in Equation (7), the parameters can then be resolved as follows:
(11)
(11)
(12)
(12)
(13)
(13)
The market size (m) will be determined in this work for this forecasting process using an external procedure (such as a survey of long-term purchase intentions) to account for terrain peculiarities like population/target market volume, penetration rate, average cost of technology, etc., while the Bass parameters and
will be estimated based on the relationships shown in Equations (11) and (12) in conjunction with
.
2.1.1.2 Determining adopter categories
Adopter of a technology based on the time of adoption can be categorized into the following groups, namely: innovators, early adopters, early majority, late majority and Laggards (see ).
Figure 2. Adopter categories based on Bass diffusion model. Source: Mahajan, Muller, and Srivastava (Citation1990).

Determining the respective adopter categories is dependent on knowing the inflection points and
, which are expressed analytically as follows (Mahajan, Muller, and Srivastava Citation1990):
(14)
(14)
(15)
(15) Furthermore the analytical expressions for the respective adopter categories covered on the Bass adopter distribution are:
Innovators
Time interval – Initiators of diffusion process
Expression for time interval:
(16)
(16) where
means expression for time interval for innovator category of the i-th technology; and
means no expression or value.
Expression for adopter category size:
(17)
(17) where
represents expression for adopter category size for innovator category of the i-th technology
(b) | Early adopters |
Time interval – Up to
Expression for time interval:
(18)
(18) where
represents expression for time interval for early adopters category of the i-th technology
Expression for adopter category size:
(19)
(19) where
represents expression for adopter category size for early adopter category of the i-th technology
(c) | Early majority |
Time interval – to
Expression for time interval:
(20)
(20) where
represents expression for time interval for early majority category of the i-th technology
Expression for adopter category size:
(21)
(21) where
represents expression for adopter category size for early majority category of the i-th technology
(d) | Late majority |
Time interval – to
Expression for time interval:
(22)
(22) where
represents expression for time interval for late majority category of the i-th technology
Expression for adopter category size:
(23)
(23) where
represents expression for adopter category size for late majority category of the i-th technology
(e) | Laggards |
Time interval – and Beyond
Expression for time interval:
(24)
(24) where
means expression for time interval for laggards category of the i-th technology; and
means no expression or value.
Expression for adopter category size:
(25)
(25) where
represents expression for adopter category size for laggards category of the i-th technology
2.2 Multi-criteria analysis
In this section the simple mathematical models employed to conduct the multi-criteria analysis of selected low-carbon technologies to obtain an optimal system ranking of technologies that facilitates the goal of achieving Nigeria’s low carbon energy transition is presented. To accomplish this, a multi-criteria optimization technique called Technique for Order Preference by Similarity to Ideal Solution (TOPSIS) is used. This technique enables the identification of solutions from a finite number of alternatives by simultaneously minimizing the distance from an ideal point and maximizing the distance from a negative ideal point. Employing this methodology aids the synthesis of a ranking system based on attributes such as cost, mitigation potential, technology readiness, and technology diffusion. For simplicity of analysis using the TOPSIS methodology, the following assumptions were adopted:
Each decision-making criterion considers either monotonically increasing or decreasing preference;
The criteria need to be given a set of weights;
Using the appropriate scaling technique, every outcome that is expressed in a non-numerical form should be quantified.
2.2.1 Procedure/mathematical formulations of the TOPSIS methodology
In this subsection, the key steps in carrying out the Technique for Order Preference by Similarity to Ideal Solution (TOPSIS) analysis are presented. The application of TOPSIS will be as follows (Corrente and Tasiou Citation2023; Hwang and Yoon Citation1981; Pavić and Novoselac Citation2013; Tamošaitienė, Zavadskas, and Turskis Citation2013):
Step 1 – Create a decision-making matrix with ‘m’ alternatives and ‘n’ criteria
Consider a ranking problem in which the alternatives of are evaluated on the criteria in
with the intersection of the alternative and criteria given as
to form a matrix
.
(26)
(26) For visibility, the alternatives
, criteria
and weights
are placed in an initial table as presented in .
Table 1. TOPSIS initial table of alternatives and criteria.
Step 2 – Vector normalization
At this stage, each performance rating/value is divided by the norm of the total outcome vector of the criteria under consideration. This normalizing process facilitates easier value comparison by converting dimensioned units of respective attributes into dimensionless units. This is calculated by:
(27)
(27) where
is the normalized ratings of each cell.
Step 3 – Calculating the weighted normalized matrix
The weighted normalized matrix is obtained multiplying the weight of each criteria by the normalized performance value of each cell and is given as:
(28)
(28) where
is the weight.
Step 4 – Determine Positive and Negative Ideal Solutions
For the positive ideal solutions :
(29)
(29)
(30)
(30)
For negative ideal solutions :
(31)
(31)
(32)
(32) where
represent a benefit attribute; and
represents a cost attribute
Step 5 – Calculate the separation measure
Here the separation matrix is obtained by finding the Euclidean distance from the ideal solutions and can be calculated by:
(33)
(33)
(34)
(34) where
and
represents the Euclidean distances from the target alternative
to the positive and negative ideal solutions respectively.
Step 6 – Relative closeness
The relative closeness to the ideal solution of the respective alternatives can be determined by:
(35)
(35) where
is the relative closeness or the overall preference score for each alternative
Step 7 – Ranking of alternatives
This is the final step where the best alternatives are ranked in descending order of relative closeness.
2.2.2 Weight determination for multi-attribute problems
In multi-attribute decision making problems weights are required to convert the normalized matrix into a weighted normalized matrix as presented in §2.2.1 (step 3). A method of obtaining the weights to be used for the criteria weight calculations is based on the Analytic Hierarchical Process (AHP) with the procedure presented as follows (Balasundareshwaran et al. Citation2019; Saaty Citation1987).
Step 1 – Develop the pairwise comparison matrix
Using the Saaty’s Pairwise comparison scale for Analytic Hierarchical Process (AHP) preferences shown in , populate the pair-wise comparison matrix based on the assigned relative importance and
which will be represented as
for every level of the respective criteria
. The pair-wise comparison matrix
is given as:
(36)
(36) i.e.
(37)
(37)
(38)
(38) Step 2 – Generate normalized pairwise comparison matrix
Table 2. Scale of relative importance.
The matrix is normalized by dividing the respective value in each cell by their respective column total, and can be obtained using Equation:
(39)
(39) where
and
represents the respective cell value(s) before and after normalization, respectively
Step 3 – Determine criteria weight
The weights are thus calculated using Equation
which is given as:
(40)
(40)
2.2.2.1 Consistency check in analytical hierarchy process
Step 1 – Obtain weighted sum
Using the pairwise comparison matrix without normalization, the weighted sum value is calculated by:
(41)
(41) where
is the weighted sum value;
represent the criteria weight per column; and
is the respective cell value before normalization.
Step 2 – Calculate the eigenvalue of the pairwise comparison matrix which is obtain by:
(42)
(42) where
is the eigenvalue of the pairwise matrix of the i-th term
Step 3 – Determine the maximum eigenvalue of the pairwise comparison matrix which is computed by:
(43)
(43) Step 4 – Check consistency index
This entails determining the level of inconsistency in the pairwise comparison matrix which is basically the normalized difference between the maximum eigenvalue and the size of the matrix which is obtained by:
(44)
(44) where
is the number of criteria.
Step 5 – Determine consistency ratio
This is obtained by comparing the consistency index with an average random consistency index
, which is given by:
(45)
(45) The average random consistency index
is obtained from a sample of randomly generated matrices using the scale
and is presented in .
Table 3. Random consistency index (RI) (standard values).
Step 6 – Interpretation
2.3 Input data
This section present the relevant data that served as input for the respective analysis carried out in this work and are presented in and , respectively.
Table 4. Pertinent assumptions for market potential.
Table 5. Input data for least square regression analysis.
3. Results and discussion
This section presents the results obtained from the comprehensive analysis of low/zero carbon technology diffusion and mapping, derived from the application of Bass and Technique for Order Preference by Similarity to Ideal Solution (TOPSIS) models used for technology diffusion and multi-criteria analysis, respectively. The mathematical formulations employed to produce these results were coded and computed using Engineering Equation Solver (EES) and the Microsoft Excel Integrated Environment. The outcomes are accompanied by relevant discussions to provide a comprehensive understanding of the findings; and through a meticulous examination of the results and their implications, we aim to shed light on the diffusion patterns, characteristics, and ranking of selected low/zero carbon technologies in the context of Nigeria's decarbonization objectives.
3.1 Results of technology diffusion analysis
In this subsection, the outcomes of a technology diffusion analysis conducted are presented. The analysis employs the Bass Model, and covers results of estimated Bass parameters, cumulative adoptions, time to peak adoptions, and adopter categories. The results shed light on the adoption patterns and characteristics of the low carbon technologies under investigation.
3.1.1 Results of estimated bass parameters
For both Nigeria-specific and global average data, give the estimated regression coefficients and derived Bass parameters based on input data presented in . The evaluation of market potential took into account factors such as the population size
and energy demand
for the energy technologies; as well as the number of power plants
and Nigeria's carbon emission potential
for the carbon mitigation technologies (See ).
Table 6. Estimated Bass parameters based on Nigeria and global-average data for i-th technology.
3.1.2 Results of cumulative adoptions
Cumulative adoption is a key idea in the Bass model and offers important insights on the acceptance and spread of new products or technologies. It helps to forecast market penetration, evaluate market potential, and develop a deeper comprehension of the dynamics of adoption through time which is crucial for making informed decisions and implementing effective strategies to encourage and expedite the adoption process. Understanding cumulative adoption is of utmost importance in successfully introducing and disseminating innovations across diverse markets and industries. presents the cumulative number of adoptions for the energy-generating and carbon capture and removal technologies from 2023 till ten (10) to fifty (50) years into the future based on both global and Nigeria-specific diffusion rates.
Table 7. Cumulative adoption of technologies.
3.1.3 Results of time to peak adoption
The time to peak adoption is another important measure that offers important insights into the timing and intensity of the diffusion process Understanding the mechanics of the time to peak adoption of a technology influences decisions with regard to resource allocation, competitive positioning, and market timing. presents the calculated time to peak adoption for the selected low-carbon technologies, offering crucial information for strategic decision-making.
Table 8. Estimated time to peak adoptions of low carbon technologies.
3.1.4 Results of adopter categories
This subsection presents the categories of adopters/adoption, value, and the corresponding time duration for the respective technologies. A higher
value indicates that the adoption of a technology is significantly influenced by social factors, such as social influence or imitation. In other words, consumers are more likely to accept and adopt a new product or technology based on the suggestions or experiences of others, rather than being solely influenced by marketing initiatives. This underscores the importance of social ties, suggestions, and word-of-mouth in the adoption process. Conversely, for technologies with a lower
value, the coefficient of innovation
has a stronger impact on adoption than imitation
which means consumers’ responses to the technology adoption are more influenced by external factors like advertising campaigns, promotions, or product attributes, rather than the adoption behaviors of others.
and present the value, time duration, and the size of adopter categories for selected low carbon technologies. These tables incorporate global and Nigeria-specific data, considering market potential in terms of population size and energy demand for energy generating technologies, as well as the number of potential power plants and Nigeria's
emission levels for carbon capture and removal technologies.
Table 9. Estimated duration and size of the low-carbon technology adopter category based on global and Nigeria data with market potential based on Nigeria population and projected number of power plants.
Table 10. Estimated duration and size of the low-carbon technology adopter category based on Nigeria and global data with market potential based on Nigeria energy demand and estimated emission levels.
3.2 Results of multi-criteria analysis
It is essential to assess the best technology options that fit Nigeria's energy mix after examining the pace of technological diffusion of each option in relation to the country's population and energy needs. This ranking takes into account a number of factors, including technology readiness, technical, economic, environmental, social, terrain-specific and /or due to primary energy resource potential. This was accomplished through the use of a multi-criteria analysis tool – Technique for Order Preference by Similarity to Ideal Solution (TOPSIS) and the outcomes are presented in this section. Cost (C), Time to Peak Adoption of Technology (TPA), Mitigation Potential (MP), and Technology Readiness Level (TRL) are the four parameters that were taken into consideration for the analysis. The coding for TRL is: ‘1’ represents laboratory research level (TRL 1–3); ‘2’ represents technology development plus small-scale demonstration (TRL 4–6); and ‘3’ represents large-scale operational demonstration and commercialization (TRL 7–9). The results of the multi-criteria analysis are shown in .
Table 11. Decision making matrix.
Table 12. Vector normalization.
Table 13. Initial pairwise comparison matrix (fraction).
Table 14. Check consistency.
Table 15. Consistency index and ratio.
Table 16. Weighted normalized matrix.
Table 17. Separation measures, relative closeness and rank.
3.2.1 Weight determination via analytic hierarchy process
The Analytical Hierarchy Process (AHP) is a commonly employed method for determining weights in multi-criteria analysis. It offers a structured framework that allows decision-makers to evaluate the relative importance of criteria through pairwise comparisons. In this study, the AHP method is employed to determine the criteria weights, and subsequently verify consistency, as presented in . The intermediate processes and results leading to the final criteria weights are outlined in Appendix A (refer to Tables A.1–A.3).
3.2.2 TOPSIS results after weighting and normalization
Having obtained the weights, the results of normalized weighted matrix, positive and negative ideal solutions, separation measures, relative closeness and rank were determined and are presented in and . However, for the results of the positive and negative ideal solutions, please refer to the Appendix (See Tables A.4 and A.5, respectively).
4. Critical findings and discussions
The findings arising from the results of technology diffusion and multi-criteria analysis conducted is presented in this section. The analysis of technology diffusion focused on adoption, considering Nigeria population and estimated required energy demand. However, for the purpose of discussion, the preference of this article is to highlight the technology diffusion characteristics mostly in relation to energy demand; and also draw conclusion and/or proffer recommendations based on the ranking of the multi-criteria analysis. By empirically assessing the diffusion and mapping of low/zero carbon technology diffusion and mapping for Nigeria’s decarbonization, this article provides the following key findings and corresponding discussions.
Several low/zero carbon technologies that offer significant mitigation potential have not found significant adoption in Nigeria. They include wind, concentrating solar plant (CSP), geothermal, nuclear, hydrogen, fuel cell for the energy generating technologies and for carbon capture and removal technology, they are carbon capture utilization and sequestration (CCUS), direct air capture (DAC) and lime soda technology.
For energy generating technologies that are operational in Nigeria, such as hydro, natural gas, bioenergy, and solar photovoltaic, projections based on Nigeria estimated required energy demand with respect to Nigeria data for technology use shows that if the current trajectory of penetration in Nigeria is sustained in the next fifty (50) years, starting from year 2023 cumulative adoptions would amount to 21.12 MW, 0.47MW, 0.11MW and 0.18MW in the short term (circa year 2033); 63.48MW, 2.20MW, 0.82MW and 1.05MW in the medium term (circa year 2053); and 106.02MW, 6.66MW, 3.84MW, and 3.68MW in the long term (circa year 2073), respectively for the technologies which depicts a very slow adoption. Conversely, when applying the average global trajectory of technology use to Nigeria's estimated energy demand, the cumulative adoptions are projected to reach 12,680MW, 36.65MW, 1,132MW, and 4,117MW in the short term (circa year 2033); 43,490MW, 134.89MW, 7,444MW and 75,070MW in the medium term (circa 2053); and 68,860MW, 279.06MW, 25,960MW, and 90,320MW in the long term (circa year 2073), respectively, over the same fifty-year period. This shows that based on current Nigeria trajectory of use, the highest cumulative adoptions was from hydro, followed by natural gas, bioenergy and solar photovoltaic technology. While with respect to the average global trajectory of technology use the highest cumulative adoptions in fifty (50) years was seen to be from solar photovoltaic, followed by hydro, bioenergy, and natural gas.
Furthermore, the findings suggest that for energy-generating technologies currently unavailable in Nigeria, such as wind, concentrating solar power (CSP), geothermal, nuclear, hydrogen, and fuel cell technologies, if Nigeria were to intensify efforts to adopt any of these technologies based on the average global trajectory of technology use in relation to Nigeria's estimated energy demand, the potential electricity generation for Nigeria from these technologies would be 5,159MW, 61.24MW, 136.96MW, 3.85MW, 0.98MW, and 3.11MW in the short term (circa year 2033); 45,110MW, 652.94MW, 771.71MW, 14.07MW, 3.68MW, and 609.36MW in the medium term (circa year 2053); and 83,650MW, 4,627MW, 2,597MW, 28.87MW, 7.81MW, and 50,050MW in the long term (circa year 2073), respectively, for the highlighted technologies.
Overall for all the highlighted energy generating technologies based on average global trajectory of technology use with respect to cumulative adoptions by the Nigeria population, results show that in the next fifty (50) years, solar photovoltaics, wind, hydro, fuel cell and bioenergy technologies are the first five (5) most promising technologies..
The time to peak adoption for existing technologies represented in Nigeria, based on Nigeria-specific data on technology usage in relation to Nigeria's energy demand was too far off into the future with bioenergy (188.8 years) exhibiting the fastest time to adoption, followed by natural gas (266.2 years), solar photovoltaic (231.7 years), and hydro (9092 years) technologies. As for all the highlighted technologies, including those not currently utilized in Nigeria, and based on the average global trajectory of technology use, hydro (22.68 years) demonstrates the fastest time to adoption among the energy generating technologies, followed by solar photovoltaic (22.97 years), wind (29.66 years), fuel cell (49.19 years), bioenergy (62.39 years), concentrating solar power (79.78 years), geothermal (113.70 years), natural gas (324.3 years), nuclear (457 years), and hydrogen (467 years) technologies. Regarding carbon capture and removal technologies, carbon capture utilization and sequestration (CCUS) (4.2 years) exhibit the fastest time to adoption, followed by direct air capture and lime-soda (8.7 years) technologies.
The outcomes of the multi-criteria analysis utilizing the Technique for Order Preference by Similarity to Ideal Solution (TOPSIS) generated a ranking of the most promising low/zero carbon technology options. This ranking took into account factors such as Cost (C), Time to Peak Adoption of Technology (TPA), Mitigation Potential (MP), and Technology Readiness Level (TRL). Based on this analysis, it can be inferred that adoption efforts for Nigeria could be intensified for already matured technology options such as hydro, solar photovoltaic, natural gas, bioenergy, wind, and concentrating solar power. Conversely, for technologies that may be considered novel, not fully matured, or influenced by specific resource constraints in Nigeria, exploratory investigations into options like hydrogen, fuel cell, geothermal, and nuclear can help elucidate the benefits of introducing them to Nigeria's energy mix.
5. Conclusion
An analysis to provide valuable insights into the diffusion and mapping of low/zero carbon technologies for Nigeria’s decarbonization has been presented in this article. Through technology diffusion analysis and multi-criteria analysis, critical findings emerged. Firstly, it is evident that there is a significant potential for mitigation through the adoption of low/zero carbon technologies in Nigeria. However, it is concerning that certain technologies with substantial mitigation potential, such as wind, concentrating solar power, geothermal, nuclear, hydrogen, and carbon capture utilization and sequestration (CCUS), are currently not represented in Nigeria. Furthermore, the projections based on Nigeria's estimated energy demand highlight the slow adoption rate of the technologies currently utilized in the country. If the current trajectory persists, cumulative adoptions in the next fifty years will be relatively low. Comparatively, applying the average global trajectory of technology use to Nigeria's estimated energy demand reveals a more substantial potential for cumulative adoptions. Hydro, natural gas, bioenergy, and solar photovoltaic technologies have shown the highest cumulative adoptions based on Nigeria's current trajectory. However, when considering the average global trajectory, solar photovoltaic technology emerges as the leading option, followed by hydro, bioenergy, and natural gas. Finally, multi-criteria analysis, employing the Technique for Order Preference by Similarity to Ideal Solution (TOPSIS), has provided a ranking of the most promising low/zero carbon technology options. This ranking, considering factors such as cost, time to peak adoption, mitigation potential, and technology readiness level, suggests that efforts should focus on intensifying the adoption of already matured technologies such as hydro, solar photovoltaic, natural gas, bioenergy, wind, and concentrating solar power. However, it is also crucial to explore technologies like hydrogen, fuel cell, geothermal, and nuclear, taking into account their specific constraints and potential benefits within Nigeria's energy mix. It is noteworthy that enhancing the adoption of these technologies requires a focus on targeted policies, incentives, and infrastructure development. Continued research and monitoring of technology trends, along with adaptive policy frameworks, will be crucial for achieving sustained success in Nigeria's decarbonization journey.
The implications of the study's findings extend beyond the immediate context, holding significant relevance for other African and developing economies. One notable contribution lies in the identification of promising low-carbon technologies. The study's systematic ranking of these technologies based on their diffusion potential offers invaluable guidance to countries seeking to embark on their decarbonization journeys. This insight becomes a compass for decision-makers, aiding them in strategically prioritizing technologies for maximum impact. Furthermore, the study equips policymakers with a nuanced understanding of the factors influencing the adoption of low-carbon technologies. This depth of insight is instrumental in the development of effective adoption strategies. Armed with this knowledge, policymakers can design targeted interventions, surmounting barriers, and facilitating the swift integration of these technologies into existing infrastructures. Moreover, the study serves as a catalyst for capacity building in African and developing economies. The findings offer a foundation upon which to raise awareness and enhance understanding of the deployment and operation of low-carbon technologies. This, in turn, empowers local communities and institutions to actively engage in sustainable practices, fostering a collective commitment to a low-carbon future. In summary, the study's broad-ranging implications underscore its pivotal role in shaping the trajectory of environmentally conscious technological adoption across diverse economic landscapes.
Disclosure statement
No potential conflict of interest was reported by the author(s).
Data availability statement
The data that support the findings of this study are available. If further clarification, request or permission for use of specific materials is needed, please contact the corresponding author Chinedum Oscar Nwachukwu at [email protected] upon reasonable request.
References
- Abbass, K., M. Z. Qasim, H. Song, M. Murshed, H. Mahmood, and I. Younis. 2022. “A Review of the Global Climate Change Impacts, Adaptation, and Sustainable Mitigation Measures.” Environ. Sci. Pollut. Res 29 (28): 42539–42559. https://doi.org/10.1007/s11356-022-19718-6.
- Ajala, S. 2024. “BETD2022: Nigeria Commits to 30% Renewable Energy Generation by 2030.” Dataphyte. https://www.dataphyte.com/latest-reports/energy/renewable-energy/betd2022-nigeria-commits-to-30-renewable-energy-generation-by-2030/#:~:text=BETD2022%3A%20Nigeria%20commits%20to%2030%25%20renewable%20energy%20generation%20by%202030,-by%20Samuel%20Ajala&text=The%20Nigerian%20Minister%20of%20Power,energy%20to%20achieve%20Vision%202030 (accessed 11 January 2024).
- Balasundareshwaran, A., S. A. Rahaman, K. Balasubramani, K. Kumaraswamy, and M. Ramkumar. 2019. “Habitat Risk Assessment Along Coastal Tamil Nadu, India—An Integrated Methodology for Mitigating Coastal Hazards.” In Coastal Zone Management, 515–542. City: Elsevier.
- Bass, F. M. 2004. “A New Product Growth for Model Consumer Durables.” Management Science 50 (12_supplement): 1825–1832. https://doi.org/10.1287/mnsc.1040.0264.
- Battelle Memorial Institute. 2016. “Manufacturing Cost Analysis of 100 and 250 kW Fuel Cell Systems for Primary Power and Combined Heat and Power Applications,” U.S. Department of Energy, 2016. [Online]. Available: https://www.energy.gov/eere/fuelcells/articles/manufacturing-cost-analysis-100-and-250-kw-fuel-cell-systems-primary-power.
- Baylin-Stern, A., and N. Berghoit. 2023. “Is carbon capture too expensive?” International Energy Agency. https://www.iea.org/commentaries/is-carbon-capture-too-expensive (accessed 8 July 2023).
- Çabukoglu, E., G. Georges, L. Küng, G. Pareschi, and K. Boulouchos. 2019. “Fuel Cell Electric Vehicles: An Option to Decarbonize Heavy-Duty Transport? Results from a Swiss Case-Study.” Transportation Research Part D: Transport and Environment 70: 35–48. https://doi.org/10.1016/j.trd.2019.03.004.
- Cames, M., H. Böttcher, U. Fuentes Hutfilter, and R. Wilson. 2021. “Options for multilateral initiatives to close the global 2030 climate ambition and action gap. Policy field synthetic e-fuels,” Umweltbundesamt (UBA), 2021. [Online]. Available: https://inis.iaea.org/collection/NCLCollectionStore/_Public/52/076/52076054.pdf?r=1.
- Chen, L., et al. 2022. “Strategies to Achieve a Carbon Neutral Society: A Review.” Environmental Chemistry Letters 20 (4): 2277–2310. https://doi.org/10.1007/s10311-022-01435-8.
- Climate Action Tracker. 2022. “Climate Governance: Assessment of the government’s ability and readiness to transform Nigeria into a zero emissions society,” in “CAT Climate Governance Series,” Abuja, 2022. [Online]. Available: https://climateactiontracker.org/documents/1014/2022_02_CAT_Governance_Report_Nigeria.pdf.
- Climate Action Tracker. 2023. “Nigeria: Target Overview.” https://climateactiontracker.org/countries/nigeria/targets/ (accessed 30 June 2023).
- Corrente, S., and M. Tasiou. 2023. “A Robust TOPSIS Method for Decision Making Problems with Hierarchical and Non-Monotonic Criteria.” Expert Systems with Applications 214: 1–13. https://doi.org/10.1016/j.eswa.2022.119045.
- CSL Stockbrokers. 2023. “The Nigerian Power Sector; Still in Muddy Waters.” Nairametrics. https://nairametrics.com/2021/10/22/the-nigerian-power-sector-still-in-muddy-waters/ (accessed 6 June 2023).
- Dioha, M. 2023. “Making Nigeria’s Energy Transition Plan a Reality.” https://energyforgrowth.org/article/making-nigerias-energy-transition-plan-a-reality/ (accessed 4 January 2023).
- Enerdata. 2023. “Natural Gas Production.” https://yearbook.enerdata.net/natural-gas/world-natural-gas-production-statistics.html (accessed 6 July 2023).
- Federal Government of Nigeria. 2023. “Nigeria's First Nationally Determined Contribution-2021 Update.” https://climatechange.gov.ng/wp-content/uploads/2021/08/NDC_File-Amended-_11222.pdf (accessed 30 June 2023).
- Federal Ministry of Power. 2023. “Nigerian Renewable Energy and Energy Efficiency Policy (NREEEP).” Federal Ministry of Power. https://admin.theiguides.org/Media/Documents/NREEE%20POLICY%202015-%20FEC%20APPROVED%20COPY.pdf (accessed 30 June 2023).
- Feliciano, D., J. Recha, G. Ambaw, K. MacSween, D. Solomon, and E. Wollenberg. 2022. “Assessment of Agricultural Emissions, Climate Change Mitigation and Adaptation Practices in Ethiopia.” Climate Policy 22 (4): 427–444. https://doi.org/10.1080/14693062.2022.2028597.
- Fibich, G., and R. I. Gibori. 2010. “Aggregate Diffusion Dynamics in Agent-Based Models with a Spatial Structure.” Operations Research 58 (5): 1450–1468. https://doi.org/10.1287/opre.1100.0818.
- Filho, L. W., et al. 2021. “The Influence of Ecosystems Services Depletion to Climate Change Adaptation Efforts in Africa.” Science of The Total Environment 779: 1–13. https://doi.org/10.1016/j.scitotenv.2021.146414.
- Ganjeizadeh, F., H. Lei, P. Goraya, and E. Olivar. 2017. “Applying Looks-Like Analysis and Bass Diffusion Model Techniques to Forecast a Neurostimulator Device with no Historical Data.” Procedia Manufacturing 11: 1916–1924. https://doi.org/10.1016/j.promfg.2017.07.334.
- Gielen, D., F. Boshell, D. Saygin, M. D. Bazilian, N. Wagner, and R. Gorini. 2019. “The Role of Renewable Energy in the Global Energy Transformation.” Energy Strategy Reviews 24: 38–50. https://doi.org/10.1016/j.esr.2019.01.006.
- Global Change Data Lab. 2023. “Per Capita Electricity Use: Kilowatt-Hours.” https://ourworldindata.org/grapher/per-capita-electricity-generation?tab=table (accessed 5 July 2023).
- Hassan, Q., S. Algburi, A. Z. Sameen, H. M. Salman, and M. Jaszczur. 2023. “A Review of Hybrid Renewable Energy Systems: Solar and Wind-Powered Solutions: Challenges, Opportunities, and Policy Implications.” Results in Engineering, 1–25. https://doi.org/10.1016/j.rineng.2023.101621.
- Hoegh-Guldberg, O., et al. 2019. “The Human Imperative of Stabilizing Global Climate Change at 1.5 C.” Science 365 (6459): 1–13. https://doi.org/10.1126/science.aaw6974.
- Hwang, C., and K. Yoon. 1981. Multi-Objective Decision Making–Methods and Application. A State-of-the-Art Study. New York: Springer-Verlag.
- Intergovernmental Panel on Climate Change. 2019. “Global Warming of 1.5°C: An IPCC Special Report on the Impacts of Global Warming of 1.5°Cabove Pre-Industrial Levels and Related Global Greenhouse Gas Emission Pathways, in the Context of Strengthening the Global Response to the Threat of Climate Change, Sustainable Development, and Efforts to Eradicate Poverty,” Intergovernmental Panel on Climate Change (IPCC), Cambridge, UK; New York, USA, 2019. [Online]. Available: https://www.ipcc.ch/site/assets/uploads/sites/2/2022/06/SR15_Full_Report_HR.pdf.
- International Atomic Energy Agency. 2023. “Nuclear Power Capacity Trends.” https://pris.iaea.org/PRIS/WorldStatistics/WorldTrendNuclearPowerCapacity.aspx (accessed 6 July 2023).
- International Energy Agency. 2021. “Net Zero by 2050: A Roadmap for Global Energy Sector,” International Energy Agency (IEA), 2021. [Online]. Available: https://iea.blob.core.windows.net/assets/deebef5d-0c34-4539-9d0c-10b13d840027/NetZeroby2050-ARoadmapfortheGlobalEnergySector_CORR.pdf.
- International Energy Agency. 2022. “CO2 Emissions in 2022,” 2022. [Online]. Available: https://iea.blob.core.windows.net/assets/3c8fa115-35c4-4474-b237-1b00424c8844/CO2Emissionsin2022.pdf.
- International Energy Agency. 2023a. “Nigeria Renewable Energy Master Plan (REMP).” https://www.iea.org/policies/4974-nigeria-renewable-energy-master-plan (accessed 27 June 2023).
- International Energy Agency. 2023b. “Renewable Energy Master Plan.” International Energy Agent (IEA), https://www.iea.org/policies/4967-renewable-energy-master-plan (accessed 27 June 2023).
- International Energy Agency. 2023c. “Nationally Determined Contribution (NDC) to the Paris Agreement: Nigeria.” https://www.iea.org/policies/11784-nationally-determined-contribution-ndc-to-the-paris-agreement-nigeria (accessed 30 June 2023).
- International Energy Agency. 2023d. “Hydrogen: Energy System Overview.” https://www.iea.org/reports/hydrogen (accessed 6 July 2023).
- International Energy Agency. 2023e. “Capacity of Large-Scale CO2 Capture Projects, Current and Planned vs. the Net Zero Scenario, 2020–2030.” International Energy Agency, https://www.iea.org/data-and-statistics/charts/capacity-of-large-scale-co2-capture-projects-current-and-planned-vs-the-net-zero-scenario-2020-2030 (accessed 6 July 2023).
- International Energy Agency. 2023f. “Direct Air Capture: Technology Deep Dive.” https://www.iea.org/reports/direct-air-capture (accessed 6 July 2023).
- International Energy Agency. 2023g. “Global Average Levelised Cost of Hydrogen Production by Energy Source and Technology, 2019 and 2050.” International Energy Agency. https://www.iea.org/data-and-statistics/charts/global-average-levelised-cost-of-hydrogen-production-by-energy-source-and-technology-2019-and-2050 (accessed 8 July 2023).
- International Energy Agency. 2023h. “Hydrogen.” International Energy Agency. https://www.iea.org/fuels-and-technologies/hydrogen (accessed 8 July 2023).
- International Energy Agency. 2023i. “Nuclear Power in a Clean Energy System.” https://www.iea.org/reports/nuclear-power-in-a-clean-energy-system (accessed 8 July 2023).
- International Energy Agency. 2023j. “The Role of Gas in Today's Energy Transitions.” international Energy Agency. https://www.iea.org/reports/the-role-of-gas-in-todays-energy-transitions (accessed 8 July 2023).
- International Energy Agency. 2023k. “IEA at COP27: The Role of Hydropower in Achieving Climate Resilience.” International Energy Agency. https://www.iea.org/events/iea-at-cop27-the-role-of-hydropower-in-achieving-climate-resilience (accessed 8 July 2023).
- International Energy Agency. 2023l. “Direct Air Capture 2022: Executive summary.” https://www.iea.org/reports/direct-air-capture-2022/executive-summary (accessed 8 July 2023).
- International Energy Agency. 2023m. “Bioenergy with Carbon Capture and Storage.” https://www.iea.org/reports/bioenergy-with-carbon-capture-and-storage (accessed 8 July 2023).
- International Energy Agency. n.d. “Carbon Capture, Utilisation and Storage.” https://www.iea.org/fuels-and-technologies/carbon-capture-utilisation-and-storage.
- International Renewable Energy Agency. 2023a. “Renewable Capacity Statistics 2023.” https://www.irena.org/Publications/2023/Mar/Renewable-capacity-statistics-2023 (accessed 5 July 2023).
- International Renewable Energy Agency. 2023b. “Renewable Power Generation Cost in 2021.” International Renewable Energy Agency. https://www.apren.pt/contents/publicationsothers/irena-power-generation-costs-2021-pags1-7.pdf (accessed 8 July 2023).
- Krishnan, M., et al. 2023. “The Net-Zero Challenge: Accelerating Decarbonization Worldwide.” McKinsey sustainability. https://www.mckinsey.com/capabilities/sustainability/our-insights/the-net-zero-challenge-accelerating-decarbonization-worldwide (accessed 27 June 2023).
- Li, J., M. S. Herdem, J. Nathwani, and J. Z. Wen. 2022. “Methods and Applications for Artificial Intelligence, Big Data, Internet-of-Things, and Blockchain in Smart Energy Management.” Energy and AI 11: 1–18. https://doi.org/10.1016/j.egyai.2022.100208.
- Lilien, G. L., A. Rangaswamy, and A. D. Bruyn. 2007. “The Bass Model: Marketing Engineering Technical Note.” In Principles of Marketing Engineering (Vol. 1515528067), 2nd ed., 248. DecisionPro Inc.
- Lipczynska-Kochany, E. 2018. “Effect of Climate Change on Humic Substances and Associated Impacts on the Quality of Surface Water and Groundwater: A Review.” Science of the Total Environment 640: 1548–1565. https://doi.org/10.1016/j.scitotenv.2018.05.376.
- Madanchian, M., and H. Taherdoost. 2023. ““A Comprehensive Guide to the TOPSIS Method for Multi-Criteria Decision Making,” A Comprehensive Guide to the TOPSIS Method for Multi-Criteria Decision Making. Sustainable Social Development 1 (1): 1–6. https://doi.org/10.54517/ssd.v1i1.2220.
- Mahajan, V., E. Muller, and F. M. Bass. 1995. “Diffusion of New Products: Empirical Generalizations and Managerial Uses.” Marketing Science 14 (3_supplement): G79–G88. https://doi.org/10.1287/mksc.14.3.g79.
- Mahajan, V., E. Muller, and R. K. Srivastava. 1990. “Determination of Adopter Categories by Using Innovation Diffusion Models.” Journal of Marketing Research 27 (1): 37–50. [Online]. Available: http://www.jstor.org/stable/3172549.
- Murshed, M., and N. T. T. Dao. 2022. “Revisiting the CO2 Emission-Induced EKC Hypothesis in South Asia: The Role of Export Quality Improvement.” GeoJournal 87 (2): 535–563. https://doi.org/10.1007/s10708-020-10270-9.
- Napp, T., T. Hills, S. M. Soltani, J. Bosch, and C. Mazur. 2017. “A Survey of Key Technological Innovations for the Low-Carbon Economy,” Imperial College London, 2017. [Online]. Available: https://www.oecd.org/env/cc/g20-climate/collapsecontents/Imperial-College-London-innovation-for-the-low-carbon-economy.pdf.
- National Council on Climate Change. 2024. “Climate Change Act, 2021.” https://climate-laws.org/documents/nigeria-s-climate-change-act_a2f0?id=nigeria-s-climate-change-act_5ef7 (accessed 4 January 2024).
- NDC Partnership. 2023. “Nigeria's Updated NDC is Ambitious and Inclusive.” NDC Partnership. https://ndcpartnership.org/news/nigeria%E2%80%99s-updated-ndc-ambitious-and-inclusive (accessed 30 June 2023).
- Nigeria Energy Transition Plan. 2023. “Nigeria's Pathway to Achieve Carbon Neutrality by 2060.” https://www.energytransition.gov.ng/#Plan (accessed 1 July 2023).
- Ogbonna, C. G., C. C. Nwachi, I. O. Okeoma, and O. A. Fagbami. 2023. “Understanding Nigeria’s Transition Pathway to Carbon Neutrality Using the Multilevel Perspective.” Carbon Neutrality 2 (1): 1–21. https://doi.org/10.1007/s43979-023-00065-5.
- Okoh, A. S., and E. Okpanachi. 2023. “Transcending Energy Transition Complexities in Building a Carbon-Neutral Economy: The Case of Nigeria.” Cleaner Energy Systems 6: 1–10. https://doi.org/10.1016/j.cles.2023.100069.
- Olujobi, O. J., U. E. Okorie, E. S. Olarinde, and A. D. Aina-Pelemo. 2023. “Legal Responses to Energy Security and Sustainability in Nigeria's Power Sector Amidst Fossil Fuel Disruptions and Low Carbon Energy Transition.” Heliyon 9 (7): 1–24. https://doi.org/10.1016/j.heliyon.2023.e17912.
- OpenInfraMap. n.d. “All 43 power plants in Nigeria.” https://openinframap.org/stats/area/Nigeria/plants.
- Our World in Data. 2024. “Renewable energy.” https://ourworldindata.org/renewable-energy (accessed 11 January 2024).
- Pavić, Z., and V. Novoselac. 2013. “Notes on TOPSIS Method.” International Journal of Research in Engineering and Science 1 (2): 5–12. [Online]. Available: https://www.researchgate.net/publication/285886027_Notes_on_TOPSIS_Method%0Awww.ijres.org.
- PennState. 2023. “Basic economics of power generation, transmission and distribution.” https://www.e-education.psu.edu/eme801/node/530 (accessed 8 July 2023).
- Purohit, P., and T. C. Kandpal. 2005. “Renewable Energy Technologies for Irrigation Water Pumping in India: Projected Levels of Dissemination, Energy Delivery and Investment Requirements Using Available Diffusion Models.” Renewable and Sustainable Energy Reviews 9 (6): 592–607. https://doi.org/10.1016/j.rser.2004.07.002.
- Pustějovská, K., K. Janovská, and S. Jursová. 2023. “Alternative Sources of Energy in Transport: A Review.” Processes 11 (5): 1–14. https://doi.org/10.3390/pr11051517.
- Ratcliff, R., and K. Doshi. 2016. “Using the Bass Model to Analyze the Diffusion of Innovations at the Base of the Pyramid.” Business & Society 55 (2): 271–298. https://doi.org/10.1177/0007650313479529.
- Rissman, J., et al. 2020. “Technologies and Policies to Decarbonize Global Industry: Review and Assessment of Mitigation Drivers Through 2070.” Applied Energy 266: 1–34. https://doi.org/10.1016/j.apenergy.2020.114848.
- Ritchie, H., and M. Roser. 2023. “Nigeria: CO2 country profile.” https://ourworldindata.org/co2/country/nigeria (accessed 5 July 2023).
- Saaty, T. L. 1987. “The Analytic Hierarchy Process-What it is and How it is Used.” Mathematical Modelling 9 (3-5): 161–176. https://doi.org/10.1016/0270-0255(87)90473-8.
- Sadeeq, M. A., and S. Zeebaree. 2021. “Energy Management for Internet of Things via Distributed Systems.” Journal of Applied Science and Technology Trends 2 (02): 59–71. [Online]. Available: 85-Full%20Manuscript-425-1-10-20210416.pdf.
- Saleem, M. U., et al. 2023. “Integrating Smart Energy Management System with Internet of Things and Cloud Computing for Efficient Demand Side Management in Smart Grids.” Energies 16 (12): 1–21. https://doi.org/10.3390/en16124835.
- Sandaka, B. P., and J. Kumar. 2023. “Alternative Vehicular Fuels for Environmental Decarbonization: A Critical Review of Challenges in Using Electricity, Hydrogen, and Biofuels as a Sustainable Vehicular Fuel.” Chemical Engineering Journal Advances, 1–21. https://doi.org/10.1016/j.ceja.2022.100442.
- Schilling, M. A., and M. Esmundo. 2009. “Technology S-Curves in Renewable Energy Alternatives: Analysis and Implications for Industry and Government.” Energy Policy 37 (5): 1767–1781. https://doi.org/10.1016/j.enpol.2009.01.004.
- Sen, S., and S. Ganguly. 2017. “Opportunities, Barriers and Issues with Renewable Energy Development–A Discussion.” Renewable and Sustainable Energy Reviews 69: 1170–1181. https://doi.org/10.1016/j.rser.2016.09.137.
- Sengupta, R. 2023. “Energizing Nigeria: Solar Power Steers the Nation Towards a Sustainable Energy Future.” Solarquarter. https://solarquarter.com/2023/06/01/energizing-nigeria-solar-power-steers-the-nation-towards-a-sustainable-energy-future/ (accessed 30 June 2023).
- Statista Research Department. 2024. “Renewable Energy Share of Electricity Capacity in Nigeria 2011-2022.” https://www.statista.com/statistics/1278245/renewable-energy-share-of-electricity-capacity-in-nigeria/#:~:text=As%20of%202022%2C%20renewable%20energy,total%20electricity%20capacity%20in%20Nigeria. (accessed 11 January 2024).
- Tamošaitienė, J., E. K. Zavadskas, and Z. Turskis. 2013. “Multi-Criteria Risk Assessment of a Construction Project.” Procedia Computer Science 17: 129–133. https://doi.org/10.1016/j.procs.2013.05.018.
- Tan, K. M., T. S. Babu, V. K. Ramachandaramurthy, P. Kasinathan, S. G. Solanki, and S. K. Raveendran. 2021. “Empowering Smart Grid: A Comprehensive Review of Energy Storage Technology and Application with Renewable Energy Integration.” Journal of Energy Storage 39: 102591. https://doi.org/10.1016/j.est.2021.102591.
- Turk, T., and P. Trkman. 2012. “Bass Model Estimates for Broadband Diffusion in European Countries.” Technological Forecasting and Social Change 79 (1): 85–96. https://doi.org/10.1016/j.techfore.2011.06.010.
- United Nations. 2023. “Renewable Energy Sources Cut Carbon Emissions, Efficiently Increase Electricity Output Worldwide, Delegates Say in Second Committee.” https://press.un.org/en/2018/gaef3501.doc.htm (accessed 27 June 2023).
- United Nations Conference on Trade and Development. 2024. “Electricity Act 2023 Liberalizes the Sector and Promotes Renewables.” https://investmentpolicy.unctad.org/investment-policy-monitor/measures/4339/nigeria-electricity-act-2023-liberalizes-the-sector-and-promotes-renewables#:~:text=The%20Act%20encourages%20the%20integration,the%20Nigerian%20Electricity%20Regulatory%20Commission (accessed 11 January 2024).
- Vavrek, R., and J. Bečica. 2022. “Similarity of TOPSIS Results Based on Criterion Variability: Case Study on Public Economic.” PLoS One 17 (8): e0271951. https://doi.org/10.1371/journal.pone.0271951.
- Virta. 2023. “What is Decarbonization, and Why Do We Urgently Need It?” https://www.virta.global/blog/decarbonisation (accessed 26 June 2023).
- Vo, D. H., A. T. Vo, C. M. Ho, and H. M. Nguyen. 2020. “The Role of Renewable Energy, Alternative and Nuclear Energy in Mitigating Carbon Emissions in the CPTPP Countries.” Renewable Energy 161: 278–292. https://doi.org/10.1016/j.renene.2020.07.093.
- Wang, F., et al. 2021. “Technologies and Perspectives for Achieving Carbon Neutrality.” The Innovation 2 (4): 1–23. https://doi.org/10.1016/j.xinn.2021.100180.
- Wang, X., et al. 2022. “Decarbonization of China's Electricity Systems with Hydropower Penetration and Pumped-Hydro Storage: Comparing the Policies with a Techno-Economic Analysis.” Renewable Energy 196: 65–83. https://doi.org/10.1016/j.renene.2022.06.080.
- Weidner, E., R. Ortiz-Cebolla, and J. Davies. 2019. “Global Deployment of Large Capacity Stationary Fuel Cells: Drivers of, and Barriers to, Stationary Fuel Cell Deployment,” Joint Research Centre and European Commission's Science and Knowledge Service, Luxembourg, 2019. [Online]. Available: jrc115923_stationary_fuel_cells_16042019_final_pubsy_online%20(1).pdf.
- The Welding Institute. 2023. “What is Decarbonisation?” https://www.twi-global.com/technical-knowledge/faqs/what-is-decarbonisation (accessed 26 June 2023).
- World Nuclear Association. 2023. “Economics of Nuclear Power.” https://world-nuclear.org/information-library/economic-aspects/economics-of-nuclear-power.aspx (accessed 8 July 2023).
- Worldometers. 2023. “Nigeria Population (LIVE).” https://www.worldometers.info/world-population/nigeria-population/ (accessed 6 June 2023).
Appendix
Table A.1. Initial pairwise comparison matrix (decimals).
Table A.2. Normalized pairwise comparison matrix.
Table A.3. Criteria weights.
Table A.4. Positive ideal solution.
Table A.5. Negative ideal solution.