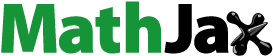
ABSTRACT
Ride-sharing service has rapidly developed around the world in recent years. In this paper, we examine how ride-sharing service affects urban air pollutants based on DIDI trip and air quality data in Shenzhen, China. With the control of weather factors, we apply spatial panel models to analyse the relationship between ride-sharing service and air quality. Our research shows: 1) PM2.5, PM10, O3, NO2, and Air Quality Index (AQI) increased when DIDI order increases, while CO and SO2 are not significantly affected. 2) After analysing the air quality impact of the new policy governing ride-sharing service, the results show that most air pollutants, namely PM2.5, PM10, NO2, CO, and AQI, have significantly decreased. It implies that the ride-sharing service acts more like a substitute of public transportation than a complement as ride-sharing service has increased the number of cars on road and deteriorated the urban air quality.
1. Introduction
With the rapid development of mobile Internet technology and the increasing people’s travel demand, transportation network companies (TNCs), such as DIDI and Uber, have become popular around the world. Reports show that up to 1.05 billion seats were shared by DIDI’s two core carpooling services, Hitch and ExpressPool until 2017 (DIDI Chuxing, Citation2018). Uber, as well, claims that around 15 million uber trips happen each day which accounts for 20% of all trips in cities where it is available (Uber, Citation2020). As an important part of public transport system, ride-sharing service provided by TNCs has attracted much attention. At first, these TNCs are considered beneficial for urban public transportation by improving the utilization of idle private car, reducing traffic congestion and exhausted emissions. As the popularity of ride-sharing service booms, more and more people are attracted from public transit or non-motorized travel into this new service which leads to an increase in traffic and emissions. Meanwhile, the ride sharing service gains increasing attentions around the world. Governments have begun to formulate regulatory policies. China is one of the first countries to legalize ridesharing. In China, the urban public transportation system includes buses, subways, light rails, taxis, ships, etc. In July 2016, the policy announced by the Ministry of Transport affirmed the legal status of ride sharing service, and clearly stated that ride-sharing service belongs to taxi service. In China, the main difference between ride-sharing service and taxi is that ride-sharing service can only take orders through platforms, while taxis can take orders through both the cruise and platform. Therefore, as the legality of ride-sharing service has been recognized, taxis have also joined the ride-sharing service platform to carry passengers and have become part of ride-sharing service. Although the central government encourages the development of the industry, the policies promulgated by the local governments have imposed strict requirements on the vehicles and drivers. Studies focusing on the ride sharing are mainly about pros and cons and whether is beneficial for the industry development (Rauch & Schleicher, Citation2015; Wallsten, Citation2015). Rare has discussed the environmental impacts brought by specific policies. Proost and Van Dender (Citation2012) have mentioned different transport policies around the world and pointed out that more costly vehicle standards are not an effective way to control pollutions in the transportation sector.
Air quality is increasingly recognized as a serious concern in China. A large body of literature has investigated the sources of air pollutants from the perspective of economic development. For instance, Chen, Zhou, Wang, and Li (Citation2018) and Shi, Zhang, Zhou, and Wang (Citation2020) have pointed out that energy consumption, industrial structure and urbanization are the main source of air pollutants. Gan, Liang, Yang, and Liao (Citation2020) further pointed out that urbanization is not only an important source of air pollution, but also an intermediary of serious air pollution caused by economic development. Hao and Liu (Citation2016) explored the factors affecting air quality in China and found that the number of vehicles and the secondary industry have significantly positive influence on PM2.5. Thanks to the rapid development of China’s economy, the number of automobiles in China has grown rapidly in recent years (National Bureau of Statistics of China (NBSC), Citation2014). Meanwhile, according to the Ministry of Ecology and Environment of China (Citation2018), vehicle pollution has become an important source of air pollution in China. Traditionally, scholars mainly divide the influence of vehicle emissions on air quality into three research themes: vehicle ownership, traffic congestion and fuel consumption. Studies have proven that air pollution would badly increase as the private vehicle ownership increases (Button, Citation1990; Lyons, Kenworthy, Moy, & Dos Santos, Citation2003). A report by World Bank also points out that increase in private vehicle ownership leads to severe air pollution and traffic congestion (World Bank Group, Citation2018). In China, when the speed of motor vehicles on the road is lower than a certain value, it is considered as traffic congestion. Currently, due to the rapid increase of vehicles in Chinese cities, traffic congestion has become increasingly serious and a major source of air pollution (Deng, Citation2017). Vehicles emissions derive from fuel consumption. A study by Han, Yong, Wang, and Ouyang (Citation2014) pointed out that private passenger vehicles fuel consumption can be up to 57.7% of the total. Undoubtedly, as a new industry closely related to vehicles, ride-sharing service would cause public environment concerns.
TNCs have propagated that ride-sharing services are environmental sustainability. DIDI assertion that they can practice energy saving and emission reduction through DIDI’s advantage and decreased traffic congestion index over 10% (DIDI Chuxing, Citation2018). Uber claims that they will help save fuel, improve air quality and increase a transportation system’s efficiency by shared trips (Uber, Citation2020). However, the worry of ride-sharing service impacts on environment has never stopped. The private vehicle ownership may reduce as ridesharing service increases vehicle utilization. It’s not the vehicle ownership but the miles vehicle travelled that produce emissions. Bloomberg CityLab reports that approximately 20% of the miles by Uber and Lyft drivers are without passengers in San Francisco and increase the total miles that the car travels (Bliss, Citation2018). As the TNCs provide more convenient travel services, more people may take extra travel which may not happen before, thus induce more emissions (Kerber, Citation2019). However, TNCs have described a beautiful scene that their competition is the private vehicles not the public transportation system. A report by Schaller Consulting in 2018 suggests that the TNCs act more a substitute of public transportation, walking and biking. They find TNCs have added 5.7 million of driving annually in US major cities. The household car ownership growth rate across US major cities exceeds the rate of population growth where the TNCs get popular (Schaller, Citation2018). Furthermore, TNCs may deteriorate the urban air quality by increasing the traffic congestion. San Francisco County Transportation Authority compares traffic citywide between 2010 and 2016, and they conclude that the TNCs have increased the traffic congestion (Castiglione et al., Citation2018). Since the ride-sharing service effects on vehicle ownership, traffic congestion and fuel consumption can be complicated. It’s hard to tell whether the overall effects caused by ride-sharing service is environmentally friendly or not. Therefore, it is essential to explore the relationship between ride-sharing service and air quality.
Literature studies on TNCs’ effects on air quality are not abundant. Kitchel (Citation2017) has questioned whether TNCs are environmentally friendly, and he found that the relationship between Uber operation and automotive fuel consumption is not statistically significant by comparing the data of 2 years before and after Uber into most metropolitan areas of United States. Yu et al. (Citation2017) conducted a questionnaire survey combined with DIDI trip data and studied the environmental benefits of ride-sharing resulted from travel mode shift and attitude change towards car purchase behavior. They calculated travel emissions by data from phone-questionnaire and DIDI, concluding that ride-sharing can largely reduce emissions. Through Beijing taxi rides data, Cai, Wang, Adriaens, and Xu (Citation2019) calculated emission reduction with emission factors by simulating ride sharing and concluded environmental benefits like reducing exhaust emission. Both studies take Beijing as the empirical context and evaluate the environmental impacts by calculating vehicle emissions. Although Yu et al. (Citation2017) and Cai et al. (Citation2019) concluded that emissions can be reduced under their experiments. There is a lack of studies related to air quality to characterize the reduction of air pollution.
More researches focus on effects of ride-sharing service on traffic congestion which provide some insights into its effects on air quality, as traffic congestion and urban air quality is highly correlated (NRC, Citation2002). A study conducted by the Transportation Sustainability Research Center of UC Berkeley shows that ride-sharing can help decrease the will of purchasing a car and reduce the number of cars on the road, which results in the decrease on car congestion (Shaheen & Chan, Citation2015). On the contrary, there are also studies suggest that TNCs, like DIDI and Uber, has added to traffic congestion (Erhardt et al., Citation2019; Schaller, Citation2018). Furthermore, some studies directly indicate that ride-sharing service can promote traffic congestion while weakening public transportation (Li, Hong, & Zhang, Citation2016; Wu, Shen, Xu, Peng, & Ou, Citation2018). Thus, from the perspective of traffic congestion, the relationship between ride-sharing service and air quality remains uncertain.
On the other hand, weather conditions, such as rainfall, temperature, wind, relative humidity and air pressure, are also important factors that can have significant impacts on air quality by influencing the formation, accumulation and dispersion of air pollutants (Kumar, Fennell, & Britter, Citation2008). Urban particulate matter, which is generally measured by PM2.5 and PM10, is significantly influenced by rainfall, temperature, wind, relative humidity and air pressure (Li et al., Citation2014; F. Huang et al., Citation2015). The concentration changes of CO, SO2, NO2, and O3 is found to be connected with relative humidity changes (Zhang et al., Citation2015a); Hu et al., Citation2017). In addition, an increase in O3 concentration is often observed with increase in temperature (EPA, Citation2006). Thus, we control these factors in our analysis.
In the past, many studies used traffic pollutant emission simulation methods to study the pollution of motor vehicles (Guo, Zhang, Shi, & Wang, Citation2007; Liu, Chen, Wang, & Han, Citation2013). However, these studies have some problems. First, the simulated pollutants are relatively one-sided (Kousoulidou, Ntziachristos, Mellios, & Samaras, Citation2008). Moreover, these studies mainly simulate automobile pollutants, which are not exactly the same as air pollutants. Second, subject to simulation conditions, many studies simulate the entire environment through the results of specific experiments (Zhang et al., Citation2015b). These simulations can be very different from the real world. Moreover, these simulations require massive data when applied to the city level, which is difficult to obtain in practice. In a word, there is a big difference between simulation and real world. Therefore, in order to make up for the shortcomings of simulation and to explore whether ride-sharing service is environmentally friendly, it is necessary to use big data to explore the impact of ride-sharing service on the air.
Before being recognized by the government, China’s ride-sharing service market was in a brutal stage of development. The industry does not have explicit requirements for drivers and vehicles, they only need to be approved by platforms. As the industry grew and DIDI became the industry leader, supervision came. In September 2016, the Chinese central government recognized the legal status of ride-sharing service. On 28 December 2016, the Shenzhen Municipal Government promulgated a local ride-sharing service operation policy. The new policy puts forward specific requirements for operating vehicles and drivers. For operating vehicles, requirements for vehicle wheelbase, on-board terminals, displacement, and the length of mandatory retirement of vehicles have been put forward. For drivers, qualification examination, local residence permit, driving experience, and traffic records are all required. The implementation of this policy will screen out some drivers and vehicles to withdraw from the industry. For example, drivers who have been driving for less than 3 years will have to leave the industry. These restrictions place higher demands on both drivers and vehicles, which makes the fast-growing industry briefly enter a period of stagnation.
Based on this, the purpose of this work is to investigate how ride-sharing service influences the air quality and find out whether it is environmentally friendly while providing travel convenience. It’s divided into two parts. First, we use full daily orders of DIDI in Shenzhen to analyse how ridesharing service activity influence various regional timely air quality. We control the weather conditions and the spatial effects in our study. With a detailed analysis, we found that the number of ride-sharing service orders is negatively related with regional air quality. Second, we noticed that there is a local policy released in Shenzhen which has put a strict limitation on drivers and vehicles of ride-sharing service industry. This policy provides us a perfect chance to analyze whether the ride-sharing service is environmentally friendly or not.
In this study, our contributions include three aspects. First, order-level data can describe the relationship between ride-sharing service activity and air quality more accurately. Past research generally used the air quality changes before and after the ride-sharing service entered the city, and most of them used a single air pollutant indicator or the main pollutants of automobile exhaust (Barnes, Guo, & Borgo, Citation2020; Xue et al., Citation2018). Order-level data can describe in more detail the impact of ride-sharing service on air quality. Second, we apply spatial econometric model to measure the spatial characteristics of air pollution. At present, most studies discussing the impact of ride-sharing service consider the impact of pollutants over time, and rarely consider the impact of pollutants on space (Barnes et al., Citation2020; Chen, Chen, & Yin, Citation2020). Third, the overall impact of ride-sharing service on urban air quality has been better studied by policy analysis. The policy on the legalization of ride-sharing service is still not promulgated in many places around the world. Discussing the implementation of the new policy, especially in a large city in a developing country, has a good reference for other countries to formulate similar policies. The rest of this paper is organized as follows: Section 2 reviews the related works on ride-sharing service. Section 3 introduces the data and methodology used in our analysis. Section 4 presents the empirical results. Finally, the detailed discussion and conclusion are presented in Section 5.
2. Literature review
2.1. Ride-sharing service-related researches
As an important form of the sharing economy, ride sharing has many characteristics of the sharing economy. Scholars first focused on supervision and the changes brought about by sharing economy, e.g., Airbnb and Uber (Edelman & Geradin, Citation2015; Fahmy, Citation2020). Afterwards, scholars began to pay attention to environmental effects. Rodier, Alemi, and Smith (Citation2016) explored whether ridesharing had the potential for reduction in vehicle miles travelled (and related GHG emissions) and found that large VMT reduction can be possible with high use of ridesharing. A comparative analysis of online ride-sharing service and taxi shows that online ride-sharing service performs better on saving fuel consumption and reducing emissions from empty cruising (Sui et al., Citation2019).
However, studies of ride-sharing service effects on air quality heavily based on surveys and simulation data. With surveys or scenario analysis to calculate emissions caused by ride-sharing service, Shaheen and Chan (Citation2015); Rayle, Dai, Chan, Cervero, and Shaheen (Citation2016); Chen and Kockelman (Citation2016) suggested that household who participate in ride-sharing activity can get a reduction in transport-related energy use, greenhouse gas (GHG) emissions and personal automobiles ownership. Moreover, similar researches conducted by Shaheen and Chan (Citation2015) and Alonso-Mora, Samaranayake, Wallar, Frazzoli, and Rus (Citation2017) indicate that ride-sharing service like Uber can reduce traffic congestion and reduce vehicle emissions. Recently, scholars begin to calculate car emissions and traffic congestion caused by ride-sharing service through real ride data (Cai et al., Citation2019; Li et al., Citation2016; Yu et al., Citation2017). Yu et al. (Citation2017) and Cai et al. (Citation2019) combined real ride data with simulating ride sharing and conclude emission reduction. Li et al. (Citation2016) suggest that ride-sharing service can add to urban traffic congestion, weaken public transportation and cause emission increase. Rayle et al. (Citation2016) compared survey data with matched taxi rides data and found ride-sharing service can replace some transport mode.
As a new form of public transport mode, the studies on ride-sharing service are relatively limited. Numerous works have concentrated on vehicles influence on air quality as they are the dominant source of many air pollutants in urban areas (National Research Council (US), Citation2002). These studies focus on various aspects, such as exhaust emissions, vehicle speed, and traffic congestion. Exhaust emission increases when the vehicle speed accelerates and decelerates (Barth & Boriboonsomsin, Citation2009). Madireddy et al. (Citation2011) also found that smoother traffic flow with lower speed limit leads to lower CO2 and NOx. The traffic congestion would impede traffic flow, increase the fuel consumption and emissions (Treiber, Kesting, & Thiemann, Citation2008). As a fact, the increasing vehicle ownership around the United States contributes to traffic congestion and also leads to more fuel consumption and emissions over the past 20 years (Schrank & Lomax, Citation2009).
Recently, scholars began to pay attention to whether TNCs live up to their propagation. Just as TNCs’ advertisement, ride-sharing service may reduce the purchase of household vehicles (Chen & Kockelman, Citation2016). While it may have negative effects on traffic congestion. Wu et al. (Citation2018) found no evidence prove ride-sharing service can ease urban traffic congestion as TNCs propagated. What’s more, Schaller (Citation2018) conducted a research on Uber and Lyft and the results shows that TNCs have worsen traffic congestion in major American cities.
2.2. Weather and air quality
Weather, as another important factor that influences urban air quality, is also intensively studied. Accordingly, two major types of studies on the impacts of weather conditions on air quality can be identified. The first theme of researches investigated the impacts of weather on air quality mainly referring to the direct effects. Different meteorology factors have different influence on different air pollutants. Temperature is a predominant factor that influencing Ozone (Cox & Chu, Citation1996). The concentration of SO2, CO and PM10 are significantly with high humidity (Elminir, Citation2005). Various physical and chemical reactions of particle matters can occur at different temperatures (Grell & Freitas, Citation2014) and thus result in concentration changes. Wind speed has two sides. It may reduce the concentrations of pollutants when wind speed is low while sometimes it may transport pollutants from other regions when wind speed is high (Zhang et al., Citation2018). The second kind of researches investigated the impacts of weather on air quality referring to the indirect effects caused by transport. Bad weather can cause serious traffic congestion, affecting travel demand and traffic flow (Maze, Agarwal, & Burchett, Citation2006). Like rain can lower the traffic speed and even decrease travel demand (Koetse & Rietveld, Citation2009). Cools, Moons, and Wets (Citation2010) examined how weather conditions affect traffic intensity in Belgium and the results show that rainfall and wind speed could diminish traffic intensity while high temperature could increase traffic intensity. All these changes can affect air quality indirectly.
As mentioned above, most studies on ride-sharing service either used traditional survey-based data or scenario analysis without considering weather conditions. This may result in a biased result as air quality is closely related with weather. Moreover, traffic congestion seems to be the sole focus of the existing studies concerning ride-sharing service. Few studies have been conducted including real-world orders. The work by Yu et al. (Citation2017) utilized daily trip statistics for the analysis. However, the result is combined with data from phone-questionnaire and spatial analysis is not included. More detailed analyses at regional level and investigation into the impacts of weather on the environmental impact of ride-sharing service is also essential. Thus, this study is proposed to fill some of these gaps.
3. The study case and methodology
3.1. The study case
Shenzhen (longitude 113°43′∼114°38′E, latitude 22°24′∼22°52′N, subtropical monsoon climate, an area of 1997.47 km2), is located in the south of Guangdong Province and south to Hong Kong. By the end of 2017, Shenzhen has a population of 12.53 million (with less than 4.4 million registered population among the permanent residents). As most people are not indigenous, it is also known as an “immigrant city”. The main mode of travel in Shenzhen is public transportation. According to Shenzhen Statistical Bulletin (Citation2018), there are 17,430 buses and 18,379 taxis (including ride-sharing cars) in 2017. Among them, all buses are electric buses, and taxis are a mixed operation of traditional cars and electric ones. At the same time, according to 2016 Shenzhen environmental status report, only SO2 and NO2 meet the standards of WHO. There exists a little difference between Shenzhen and other international megacities, especially fine particulate matter. The annual average PM2.5 concentration is 15–20 µg/m3 higher than that of these representative cities, such as Tokyo, San Francisco, and New York in the world. The concentrations of PM2.5 at each monitoring station during this period are shown in ().
Figure 1. Shenzhen PM2.5 concentration and WHO standard (2017 WHO 24-hour guideline value of PM2.5 is no more than 25 μg/m3).

DIDI, founded in 2012, is a transportation network company (TNC), based on the sharing economy that provides travelling services by a mobile phone application. After acquiring Kuaidi and Uber, DIDI becomes the biggest TNC in China, which accounts for over 80% market share in ride-sharing service and 99% of taxis service. Before our research, DIDI has acquired Uber in China.
3.2. Data and variable
To examine how ride-sharing service affects air quality in Shenzhen, our analysis is divided into two parts. First, we study whether ride-sharing service activity affects local air quality proxies. This gives us a hint about the relationship between ride-sharing service and air quality. But it’s still not conclusive as ride-sharing service may be a substitute of private vehicles. To test the overall effects of ride-sharing service on air quality, we conduct a policy analysis in the second part.
Ride-sharing service activity is represented by DIDI order data from DIDI Chuxing (Shenzhen). This includes full anonymous operation orders (3.9 million valid pieces) in Shenzhen from September 19th to September 25th, 2016. Each order contains the longitudes and latitudes where it starts and ends. So we can match the orders with local weather and air quality monitoring station according to the principle of proximity. The temporal and spatial distribution are shown in ().
shows the temporal distribution of DIDI daily orders. presents the spatial distribution of DIDI daily orders.
As shown in (), there are 2 peak periods (8:00–9:00&18:00–19:00) from Monday to Thursday. There are 3 peak periods (8:00–9:00&18:00–19:00&22:00) on Friday. There are 2 peak periods (18:00–19:00&22:00) on Saturday. There is 1 peak period (17:00–19:00) on Sunday. From (), it can be seen that orders are mainly distributed in the southern, northern and central core areas. There are less orders in the east and northwest.
The air quality proxies are collected from Shenzhen Ecological Environment Bureau official website. The data of 11 monitoring stations are displayed independently and collected according to daily average. The spatial distribution of air monitor stations is relatively evenly distributed in densely populated areas and consistent with (). Each station collects PM2.5, PM10, SO2, NO2, CO, and O3, and also provides AQI based on criteria published by China Environmental Monitoring Center. And we use these air pollutants monitoring data as air quality proxies in this paper. The weather control includes wind speed, temperature, relative humidity, rainfall and air pressure, which are collected from Shenzhen Meteorological Bureau. There are more than 100 weather observation stations. The weather and air quality observations are matched according to the principle of proximity. All the other Shenzhen data mentioned in this article are from various statistical bulletins of Shenzhen.
The policy of ride-sharing service in Shenzhen was issued on December 28th, 2016 and became effective on December 29th, 2016. So, meteorological records and air quality data in the second part were collected before and after the policy. We skipped three months data after the policy for two reasons: 1) The Spring Festival (28 January 2017) is soon after the release of the policy. According to officials, the Spring Festival travel rush lasts for 40 days. During this period, as the Spring Festival approaches, millions of people who work or study out of their hometowns will rush home to reunite with their families. Given that Shenzhen is an immigrant city, most people will leave for hometown before the Spring Festival and return to Shenzhen after the holiday. The time span may be longer. According to the Shenzhen Monthly Statistics Report (Citation2017), the volume of road passenger transportation has been declining since reaching the peak in January and did not start to rebound until April. The ride activities in Shenzhen during this period would be greatly reduced as both drivers and passengers would be largely reduced. 2) Though the policy was effective on December 29th, 2016, the unqualified drivers and ride-sharing vehicles may not quit immediately. Therefore, based on the amount of data and the actual situation, a 3-month data after the policy will be removed to eliminate interference. The second part contains data collected during September 1st to December 28th, 2016 (before policy) and April 1st to July 31st, 2017 (after policy).
To explain the spatial variation of air quality in Shenzhen, this paper uses different pollutant concentration indexes, namely PM2.5, PM10, SO2, NO2, CO, O3, and AQI, to represent the air quality. DIDI orders (lnDD) represents the ride-sharing service activity and the binary variable policy indicates the implementation of ride-sharing service policy. Temperature(T), air pressure(P), daily rainfall(R), relative humidity(R) and wind speed (WS) are weather controls (details in ).
Table 1. Definition of variables
Descriptive statistics for all variables of the two parts are provided in .
Table 2. Descriptive statistics of variables
3.3. Methodology
Air pollutants naturally have spillover effects. Meanwhile, DIDI orders and weather conditions can also be spread and spilled over adjacent areas. It means the observations can be affected by its neighbouring areas. Thus, the estimation of spatial econometric models is better than the non-spatial econometric models. We should build spatial models to measure the impacts of air pollutants.
Prior to the spatial statistics analysis, it’s necessary to build a spatial weight matrix to analyse the spatial relationship (Elhorst, Citation2010). In this paper, the spatial weight matrix is based on the geographical distance of the air monitoring sites. Since the distance between sites is fixed, the matrix is a symmetrical matrix written as follows:
where wij = wji.
In EquationEquation (2)(2)
(2) , i and j represent each site; dij is the linear distance from i to j. Therefore, this matrix indicates that as the distance increases, the correlation between sites becomes weaker.
SLM (spatial lag model), SEM (spatial error model), and SDM (spatial Durbin model) are the most widely used spatial econometrics models. SLM describes that the dependent variable of a specific space not only depends on its own independent variables, but also on the dependent variable in the surrounding spaces. SEM describes that the determinants of the omitted explained variable (i.e., the error term) are spatially related. SDM describes that the dependent variable of a specific space not only depends on the independent variable of the space, but also depends on the independent variables and dependent variable of other surrounding spaces. These basic models are as follows:
In the equations, Y presents an N × 1 vector of dependent variable; X presents an N × m matrix; ρ presents the spatial autocorrelation parameter; ,
and
all present an N × 1 vector; W presents an N × N spatial weight matrix. If
, the SDM turns into spatial lag model (SLM). If
and
, the SDM turns into spatial error model (SEM). Obviously, SDM is a more generalized form than SLM and SEM.
Based on the above analysis, SDM is built in the following analysis. As for the fixed effects or random effects, Elhorst (Citation2014) has pointed out that the fixed effects model is generally more appropriate than the random effects model when studies apply space-time data of adjacent spatial units in unbroken study areas. Hence, we also chose the fixed effects model in the following work to capture the possible spatial heterogeneity due to other investigated air quality influencing factors, for instance, insudtries, buildings and energy consumption. The EquationEquation (6)(6)
(6) is used to model the ridesharing service (lnDD) on air quality(Y). Air quality includes different air quality proxies, namely PM2.5, PM10, SO2, NO2, CO, O3, and AQI.
The EquationEquation (7)(7)
(7) is used to model the ride-sharing service policy effects on air quality. The difference between EquationEquation (7)
(7)
(7) and EquationEquation (6)
(6)
(6) is that DIDI orders(lnDD) is replaced by a policy dummy(policy), which is used to evaluate how the new policy of ride-sharing service affects air quality.
4. Results
4.1. Estimated ride-sharing service activity effects on air quality
Our works first start with the effects of ride-sharing service activity on air quality with DIDI orders as ride-sharing service activity proxy. To conduct further analysis, we test the spatial autocorrelation by global Moran’s I to choose the model. As shown in (), PM2.5, PM10, O3, and AQI have a significant global spatial autocorrelation at 0.01 significant level which implies a spatial model is necessary for these dependent variables. To explore the relationship between ride-sharing service activity(lnDD) and PM2.5, PM10, O3 and AQI, SDM is constructed. For other air quality proxies, namely SO2, NO2, and CO, the global spatial autocorrelation is not significant, OLS analysis is applied. Clearly, the R-sq of SO2, NO2, and CO are lower than other models. It suggests that in a relatively small scale, the local air pollutants are prone to the air pollution around it. When the spatial influence is considered, the fact that the R-sq of the model has increased a lot implies spatial models are more appropriate in this condition.
Table 3. Measures of global spatial autocorrelation for each pollutant of seven days
The regression results of ride-sharing service activity on air quality are presented in (). Among model 1∼7, the spatial lagged coefficients(ρ) for PM2.5, PM10, O3 and AQI, are statistically significant and positive. It implies these air quality proxies have strong spatial spillover effects which are consistent with Moran’s I results in (). Take PM2.5 as an example. Every 1% increase in PM2.5 concentration in the adjacent areas will cause a 0.738% increase across the local.
Table 4. The effects of ride-sharing service activity on air quality
PM2.5 The estimated coefficient of lnDD is positively significant at 0.01 level, suggesting that ride-sharing service activity is an important factor for PM2.5. The value means that every 1% increase in DIDI order will cause PM2.5 increase by 15.437 µg/m3. The coefficient of W·lnDD which means the spatial spillover effect of ride-sharing service of adjacent areas is negatively significant at 0.01 level. It may be due to the fact that an increase in orders in one place will leads to a decrease in adjacent places as the total number of vehicles in one city is relatively stable in a short period. Only rain(R) has a positively significant spillover effect at 0.01 level.
PM10 The model 2 shows that ride-sharing service activity has a positively significant effect on PM10 at 0.05 level. The coefficient of lnDD means that a 1% increase in DIDI orders would result in a 20.276 µg/m3 increase in PM10. Similarly, the spillover effect of lnDD on PM10 is negatively significant at 0.01 level. Besides, the coefficients of pressure(P) and relative humidity(H) are both positively significant at 0.05 level. The spillover effects of wind speed (WS) is positively significant at 0.05 level, implying that an increase in wind speed of adjacent areas would cause an increase in PM10 in local areas. One possible explanation is that wind can blow away the pollutants of adjacent areas to local.
O3 Similar to PM10, the coefficient of lnDD is positively significant at 0.05 level. And every 1% increase in DIDI orders would cause 26.296 µg/m3 increase in O3. The spillover effect of lnDD on O3 is negatively significant at 0.05 level, suggesting that an increase in ride-sharing service activity in adjacent areas can lead to a decrease in O3 in local. The spillover effects of relative humidity(H) is positively significant at 0.05 level, implying that an increase in relative humidity of adjacent areas would cause an increase in O3 in local area.
SO2 The spatial autocorrelation test of SO2 is not significant and we apply the OLS to analyse the effects of ride-sharing service activity on SO2. The regression result in model 4 shows that lnDD only has a weak positively significant effect on SO2. It’s not surprising that gasoline sulphur content has decreased a lot since the city has applied new gasoline standard. And the main source of SO2 pollutants comes from diesel consumption of cargo ships as Shenzhen is a port city.
NO2 Similarly, we apply OLS on NO2 as the spatial autocorrelation test is not significant. The coefficient of lnDD is positively significant at 0.01 level, which means that 1% increase in ride-sharing service activity will significantly increase the concentration of NO2 by 2.598 µg/m3.
CO In the same way, we apply OLS on CO as the spatial autocorrelation test is not significant. The result of model 6 shows that ride-sharing service activity has a weak negative effect on CO.
AQI The result of model 7 shows that lnDD has a positively significant effect on AQI. This is consistent with model 1∼6 since AQI is the comprehensive index of air pollutants such as PM2.5, PM10, O3, SO2, NO2, and CO as lnDD has a significant positive effect on most of these air pollutants. The coefficient of lnDD means 1% increase in DIDI orders will lead to the overall AQI increase by 28.643.
4.2. Estimated ride-sharing service policy effects on air quality
As mentioned above, we find that the increase of ride-sharing service activity will significantly increase most air pollutants and deteriorate air quality. However, it fails to reach the conclusion that ride-sharing service has deteriorated the air quality as ride-sharing service vehicles may behave the same as ordinary private cars. They may substitute the self-driving travels which may even lead to a decrease in the overall pollutants as private vehicle ownership may decrease a lot when ride-sharing service is convenient in city. However, the ride-sharing service can also be substitute of public transport which increases the vehicles on road and leads to more exhausts. So we further study the overall effects of ridesharing service on air quality with an analysis of ride-sharing service policy which puts more restriction on the ride-sharing service industry.
The ride-sharing service policy has reduced the overall ride-sharing service activity by putting more restrictions on vehicles and drivers. The comparison of the requirements before and after the new policy are shown in (). Before the policy, drivers are only required for three years of driving experience or more. After the policy, in addition to driving age, drivers are also required for local household registration. Moreover, there are no separate policy for electric vehicles before the policy. After the policy, not only the electric vehicles have separate requirements, but also the requirements for fuel vehicles and hybrid vehicles have updated.
Table 5. Comparison of the requirements before and after the new policy
To conduct further analysis, we introduce the policy dummy policy and examine the spatial spillover effects with global Moran’s I. As shown in (), all the air quality proxies have a significant global spatial autocorrelation at 0.01 level. So SDM is chosen to analyze the relationship between policy and air quality proxies.
Table 6. Measures of global spatial autocorrelation for each pollutant of eight months
The results of ride-sharing service on air quality are shown in (). Consistent with Moran’s I results in (), the spatial lagged coefficients(ρ) for all the dependent variables are statistically significant and positive, which implies that these pollutants have strong spatial spillover effects. Take PM2.5 as an example. Every 1% increase in PM2.5 concentration in the adjacent areas will cause a 0.859% increase across the local. So is similar to other dependent variables.
Table 7. Spatial results of overall effects of ride-sharing service on air quality
PM2.5 The estimated coefficient of policy is negatively significant at 0.01 level, suggesting that PM2.5 has significantly decreased after the implementation of the policy. The coefficient of policy means that PM2.5 would have a 0.679 µg/m3 decrease after the policy implemented. The coefficient of temperature(T), relative humidity(H) and wind speed (WS) are negatively significant at 0.01, 0.01 and 0.05 level respectively. The spatial lagged coefficients of rainfall(R) and WS are negatively significant at 0.05 and 0.01 level while T is positively significant at 0.01 level.
PM10 The model 9 shows that the estimated coefficient of policy is negatively significant at 0.01 level, suggesting that PM10 has significantly decreased after the policy. The coefficient of policy means that PM10 would have a 1.468 µg/m3 decrease after the policy implemented. The coefficient of temperature(T), relative humidity(H) and wind speed (WS) are all negatively significant at 0.01 level. The spatial lagged coefficients of rainfall(R) and WS are negatively significant at 0.01 level while T is positively significant at 0.05 level.
O3 Unlike other dependent variables, the coefficient of policy is not significant. It suggests that there is no significant change on the concentration of O3 after implementing the new policy. The estimated coefficients of temperature(T), pressure(P) and wind speed (WS) are positively significant while relative humidity(H) is negatively significant. The spatial lagged coefficients of T, P and WS are negatively significant at 0.01, 0.05 and 0.01 level respectively.
SO2 In contrast to other dependent variables, the estimated coefficient of policy is positively significant at 0.01 level. The coefficient of policy means that SO2 would have a 0.208 µg/m3 increase after the policy. Given that Shenzhen is a port city and has a lot of ship business which is the main source of SO2. There is no wonder that the ride-sharing service policy has no limitation on ship fuel consumption. Similar to PM10, the coefficient of temperature(T), relative humidity(H) and wind speed(WS) are all negatively significant at 0.01 level. The coefficient of pressure(P) is positively significant at 0.01 level. The spillover effects of P, H and WS are all negatively significant at 0.01, 0.05 and 0.05 level while T is positively significant at 0.01 level.
NO2 The result of model 12 shows that the coefficient of policy is negatively significant at 0.01 level, suggesting that NO2 has significantly decreased after the policy. The coefficient of policy means that NO2 would have a 1.395 µg/m3 decrease after the policy. Both estimated coefficients and spatial lagged coefficients of the other variables are significant at 0.05 level. It implies that NO2 is very susceptible to weather conditions. The local NO2 can not only be influenced by the local weather conditions directly but also by the indirect influence of adjacent weather conditions.
CO The coefficient of policy is negatively significant at 0.05 level in model 13. The coefficient of policy means that CO would have a 0.045 mg/m3 decrease after the policy. Similar to NO2, O3 is easily affected by weather conditions as well. The coefficients of temperature(T), pressure(P), relative humidity(H) and wind speed(WS) are all negatively significant. The spatial lagged coefficients of T, P, and H are all negatively significant at 0.01 level.
AQI The regression result in model 14 shows that policy only has a weak significant negative effect on AQI. It’s consistent with model 8∼13 as AQI is a comprehensive index. The coefficient of policy means that the AQI would decrease by 0.614 after the policy implements. The coefficients of rainfall(R) and relative humidity(H) are at 0.05 significant level. The spatial lagged coefficients of rainfall(R) and wind speed(WS) are both negatively significant at 0.01 level.
5. Discussion and conclusions
5.1. Discussion
Our research finds that the air pollutants are significantly spatial autocorrelated which is consistent with previous studies (Fang, Liu, Li, Sun, & Zhuang, Citation2015; Liu et al., Citation2017). The significant spatial autocorrelation for air pollutants means that local air quality is significantly influenced by its adjacent areas. To reduce the exhaust and improve air quality, it’s important to build a cross-region plan. The spatial autocorrelation is generally existed in air quality researches. It’s important to consider the spatial spillover effects when analyzing the factors that affect local air quality.
Results from the ride-sharing service activity effects suggest that increase in ride-sharing service activity will significantly increase the concentration of most air pollutants, namely PM2.5, PM10, O3, NO2 and AQI. However, it’s not enough to conclude that the ride-sharing service would worse the air quality. It is difficult to tell which part of vehicles would produce more emissions as ridesharing vehicles are originally from private vehicles. Moreover, in many cases, ride-sharing service works as a substitute of self-driving travels. Ride-sharing service can help reduce the purchase of private vehicles and may even result in a decrease on exhaust emissions if the overall vehicle demand in city decreased. Meanwhile, ride-sharing service can also be a substitute of public transport. This may lead to more vehicles on road and produce more exhausts. Therefore, we conduct an analysis of the ride-sharing service policy to measure the overall effects of ride-sharing service on air quality.
Results from the analysis of policy effects suggest that after the ridesharing service policy implemented, most air pollutants, namely PM2.5, PM10, NO2, CO and AQI, have declined except SO2. It confirmed the effectiveness of the new policy on ride-sharing service industry. In fact, prior to the promulgation of the new policy, ride-sharing service has occupied a number of passengers who originally travel by public transport. According to the Shenzhen Statistical Bulletin (Citation2018), compared with 2016, the number of public transportation vehicles (buses and taxis) in Shenzhen increased by 7.5%, and passenger traffic dropped by 9.68%, while taxi traffic dropped by only 0.68%. It proves that:1) It is the ride-sharing service activity not the number of vehicles that deteriorate the air quality. 2) Ride-sharing service is more a substitute of public transport than a complement. These results are consistent with previous studies (Schaller, Citation2018; Wu et al., Citation2018).
Based on the results, it’s concluded that ride-sharing service led to an increase to the air pollution. Barwick, Shanjun, Rao, and Zahur (Citation2017) calculated the health expenditure of Chinese people due to air pollution through dose-response. They pointed out that a typical Chinese household with three family members is willing to pay 160 Yuan per 10 μg/m3 to reduce PM2.5 concentration. According to the PM2.5 source research reported by the Shenzhen Municipal Ecological Environment Bureau (Citation2015), motor vehicle exhaust emissions accounted for 41%. Of this, 52% are private cars and operating vehicles. At a rough estimate, the pollution caused by ride-sharing service can account for 5.5%~10.4% of the total air pollution in Shenzhen. Based on these values, the people of Shenzhen can spend up to 1.54 billion Yuan in 2017 due to air pollution. Therefore, the total willingness to pay of Chinese households for the treatment of air quality pollution generated by ride-sharing service is between 83.78 million Yuan to 162.47 million Yuan, accounting for 0.54% to 1.04% of Shenzhen’s public health expenditure in 2017.
We also notice that the coefficient of SO2 turns significantly positive after the new policy. This may be due to some exterior factors. One possible explanation is that Shenzhen is a port city which has a large number of shipping business. The main fuel for ships is diesel which is the main source of SO2. At the same time, in the analysis of ride-sharing service activity, the coefficient of lnDD is weak significant with SO2.
6. Conclusions
Although ride-sharing service has attracted much attention from scholars, relatively limited studies have been conducted with respect to environmental impacts of ride-sharing service. Therefore, this paper explores the impacts of ridesharing service on air quality in Shenzhen. We apply spatial panel models to measure the relationship between ride-sharing service and air quality. Our results show that ride-sharing service activity has a positive effect on most air pollutants, namely PM2.5, PM10, O3, NO2 and AQI. That is, an increase in ride-sharing service activity would positively increase the concentration of most air pollutants. We further analyze the overall ride-sharing service effects on air quality with a policy analysis. The results show that most air pollutants, namely PM2.5, PM10, NO2, CO and AQI, significantly decrease after the restriction. It indicates that ride-sharing service behaves more a substitute of public transport and has increased the vehicles on road. Our research finally confirmed that ride-sharing service activity has deteriorated the urban air quality which is contrary to TNCs propagation.
The findings of this study enrich the existing researches concerning the impacts of ride-sharing service on air quality with empirical evidence derived from direct association between ride-sharing service activity and air quality. This provided real-world ride-sharing service trip data on air quality effects analysis offers a few implications for the policy-makers. First, to improve air quality, the regional governments should cooperate with each other and establish joint prevention and control mechanism as the air pollutants have a significant spillover effect. Second, the urban air quality in Shenzhen has significantly improved after the ride-sharing service policy. As a by-product of this policy, it also supports the policy which has restricted more on fuel vehicles than electric vehicles in ride-sharing service industry for environmental reasons. One limitation of this study is that due to the difficulty of data collection, our DIDI data only covered one week in Shenzhen. If possible, using more data on a larger scale (e.g., more cities or longer periods) may yield more valuable results. Another limitation is that weather may have interaction effects on air quality. We try to construct a relationship between ride-sharing service and air quality under different weather conditions. The results suggest that weather may have complex impacts on air quality. Future studies can continue to study the effects of different weather on ride-sharing service and air quality.
Acknowledgments
The authors would like to thank the editors and the anonymous reviewers at first. This study was supported by the National Social Science Fund of China (Grand NO. 17BJL041) and the discipline construction project on combating climate change and low-carbon economics of HIT, Shenzhen, China. We would like to thank DIDI Chuxing (Shenzhen) and Shenzhen Meteorological Bureau for offering data in this study.
Disclosure statement
No potential conflict of interest was reported by the author(s).
Additional information
Funding
Notes on contributors
Zixuan Wang
Zixuan Wang is a PhD candidate of management science and engineering in School of Economics and Management at Harbin Institute of Technology (Shenzhen), China. Her Primary research interests include marketing research, environmental management, and big data analytics.
Li Li
Dr. Li Li is a Professor of Finance in School of Economics and Management at Harbin Institute of Technology (Shenzhen), China. He received his BS in Engineering from Central South University in China and his Ph.D. in Economics from St. Petersburg Mining University in Russia. His primary research interests are financial market analysis, currency banking and financial decision-making, resource and environmental management. His published articles appear in Energy, Journal of Cleaner Production, and Structural Change and Economic Dynamics among others.
Jie Tang
Dr. Jie Tang is a distinguished Professor of Economics in School of Economics and Management at Harbin Institute of Technology (Shenzhen), China. He is a former vice mayor of Shenzhen, China. He received his BS and Ph.D. in Economics from Nankai University in China. His primary research interests are green economy, and innovation management policy. He has published some books and journal articles.
Binglei Xie
Dr. Binglei Xie is a professor of Transportation Planning and Management in School of Architecture at Harbin Institute of Technology (Shenzhen), China. He received his BS in Applied Mathematics and his Ph.D. from Southwest Jiaotong University in China. His primary research interests are travel behavior analysis, emergency transportation management and integrated land use/transport planning. His published articles appear in Transportation Research Part E , Advanced Engineering Informatics, and Journal of Advanced Transportation among others.
Jiaoju Ge
Dr. Jiaoju Ge is an Associate Professor of Business Administration in School of Economics and Management at Harbin Institute of Technology (Shenzhen), China. She received her BS in Economics from Sichuan University in China and her Ph.D. from the University of Florida in USA. Her primary research interest is marketing research with the interface of big data, finance, and policy. Her published articles appear in International Journal of Advertising, Applied Economics, China Agricultural Economic Review, Agribusiness, and Water Policy among others.
References
- Alonso-Mora, J., Samaranayake, S., Wallar, A., Frazzoli, E., & Rus, D. (2017). On-demand high-capacity ride-sharing via dynamic trip-vehicle assignment. Proceedings of the National Academy of Sciences, 114(3), 462–467.
- Barnes, S. J., Guo, Y., & Borgo, R. (2020). Sharing the air: Transient impacts of ride-hailing introduction on pollution in China. Transportation Research Part D: Transport and Environment, 86, 102434.
- Barth, M., & Boriboonsomsin, K. (2009). Energy and emissions impacts of a freeway-based dynamic eco-driving system. Transportation Research Part D: Transport and Environment, 14(6), 400–410.
- Barwick, P., Shanjun, L., Rao, D., & Zahur, N. (2017). Air pollution, health spending and willingness to pay for clean air in China. SSRN Electronic Journal. doi:10.2139/ssrn.2999068
- Bliss, L. (2018). Uber and Lyft could do a lot more for the planet. Retrieved April 5, 2019, from https://www.bloomberg.com/news/articles/2018-04-30/uber-lyft-and-via-aren-t-eco-friendly-yet
- Button, K. (1990). Environmental externalities and transport policy. Oxford Review of Economic Policy, 6(2), 61–75.
- Cai, H., Wang, X., Adriaens, P., & Xu, M. (2019). Environmental benefits of taxi ride sharing in Beijing. Energy, 174, 503–508.
- Castiglione, J., Cooper, D., Sana, B., Tischler, D., Chang, T., Erhardt, G. D., … Mucci, A. (2018). TNCs & Congestion.
- Chen, J., Zhou, C., Wang, S., & Li, S. (2018). Impacts of energy consumption structure, energy intensity, economic growth, urbanization on PM2.5 concentrations in countries globally. Applied Energy, 230, 94–105.
- Chen, T. D., & Kockelman, K. M. (2016). Carsharings life-cycle impacts on energy use and greenhouse gas emissions. Transportation Research Part D: Transport and Environment, 47, 276–284.
- Chen, W., Chen, J., & Yin, G. (2020). Exploring side effects of ridesharing services in urban China: Role of pollution–averting behavior. Electronic Commerce Research. doi:10.1007/s10660-020-09443-y
- Cools, M., Moons, E., & Wets, G. (2010). Assessing the impact of weather on traffic intensity. Weather, Climate, and Society, 2(1), 60–68.
- Cox, W. M., & Chu, S.-H. (1996). Assessment of interannual ozone variation in urban areas from a climatological perspective. Atmospheric Environment, 30(14), 2615–2625.
- Deng, X. (2017). Curbing congestion and vehicular emissions in China: A call for economic measures. Asia & the Pacific Policy Studies, 4(2), 354–361.
- DIDI Chuxing. (2018). DiDi Chuxing corporate citizenship report 2017. Retrieved February 4, 2019, from http://img-ys011.didistatic.com/static/didiglobal/do1_p53rQtxhA6BjW6uWpF6t
- Edelman, B. G., & Geradin, D. (2015). Efficiencies and regulatory shortcuts: How should we regulate companies like Airbnb and Uber. Stanford Technology Law Review, 19, 293.
- Elhorst, J. P. (2010). Applied spatial econometrics: Raising the bar. Spatial Economic Analysis, 5(1), 9–28.
- Elhorst, J. P. (2014). Spatial econometrics: From cross-sectional data to spatial panels. New York: Springer.
- Elminir, H. K. (2005). Dependence of urban air pollutants on meteorology. Science of the Total Environment, 350(1–3), 225–237.
- EPA US. (2006). Air quality criteria for ozone and related photochemical oxidants. Office of Research and Development, National Center for Environmental Assessment (NCEA), 1, 821.
- Erhardt, G. D., Roy, S., Cooper, D., Sana, B., Chen, M., & Castiglione, J. (2019). Do transportation network companies decrease or increase congestion?. Science Advances, 5(5), eaau2670.
- Fahmy, H. (2020). Is the sharing economy causing a regime switch in consumption?. Journal of Applied Economics, 23(1), 281–298.
- Fang, C., Liu, H., Li, G., Sun, D., & Zhuang, M. (2015). Estimating the impact of urbanization on air quality in China using spatial regression models. Sustainability (2071-1050), 7(11), 15570–15592.
- Gan, T., Liang, W., Yang, H., & Liao, X. (2020). The effect of economic development on haze pollution (PM2.5) based on a spatial perspective: Urbanization as a mediating variable. Journal of Cleaner Production, 266, 121880.
- Grell, G. A., Freitas, S.R. (2014). A scale and aerosol aware stochastic convective parameterization for weather and air quality modeling. Atmospheric Chemistry and Physics, 14(10), 5233–5250.
- Guo, H., Zhang, Q.-Y., Shi, Y., & Wang, D.-H. (2007). Evaluation of the International Vehicle Emission (IVE) model with on-road remote sensing measurements. Journal of Environmental Sciences, 19(7), 818–826.
- Han, H., Yong, G., Wang, H., & Ouyang, M. (2014). Regional disparity of urban passenger transport associated GHG(greenhouse gas) emissions in China: A review. Energy, 68, 783–793.
- Hao, Y., & Liu, Y.-M. (2016). The influential factors of urban PM2.5 concentrations in China: A spatial econometric analysis. Journal of Cleaner Production, 112, 1443–1453.
- Hu, D., Wu, J., Tian, K., Liao, L., Xu, M., & Du, Y. (2017). Urban air quality, meteorology and traffic linkages: Evidence from a sixteen-day particulate matter pollution event in December 2015, Beijing. Journal of Environmental Sciences, 59, 30–38.
- Huang, F., Li, X., Wang, C., Xu, Q., Wang, W., Luo, Y., … Chen, S. (2015). PM2.5 spatiotemporal variations and the relationship with meteorological factors during 2013-2014 in Beijing, China. Plos One, 10(11), e0141642.
- Kerber, R. (2019). Can Uber and Lyft help combat climate change? Green investors are not convinced. Independent. Retrieved May 10, 2019, from https://www.independent.co.uk/news/business/analysis-and-features/uber-lyft-climate-change-ipo-environment-global-warming-a8852396.html
- Kitchel, A. S. (2017). The Uber effect: How transportation networking companies impact automotive fuel consumption. Georgetown University.
- Koetse, M. J., & Rietveld, P. (2009). The impact of climate change and weather on transport: An overview of empirical findings. Transportation Research Part D: Transport and Environment, 14(3), 205–221.
- Kousoulidou, M., Ntziachristos, L., Mellios, G., & Samaras, Z. (2008). Road-transport emission projections to 2020 in European urban environments. Atmospheric Environment, 42(32), 7465–7475.
- Kumar, P., Fennell, P., & Britter, R. (2008). Effect of wind direction and speed on the dispersion of nucleation and accumulation mode particles in an urban street canyon. Science of the Total Environment, 402(1), 82–94.
- Li, L., Qian, J., Ou, C. Q., Zhou, Y. X., Guo, C., & Guo, Y. (2014). Spatial and temporal analysis of air pollution index and its timescale-dependent relationship with meteorological factors in Guangzhou, China, 2001-2011. Environmental Pollution, 190, 75–81.
- Li, Z., Hong, Y., & Zhang, Z. (2016). An empirical analysis of on-demand ride sharing and traffic congestion. In Proceeding International Conference on Information Systems, Dublin, Ireland.
- Liu, H., Chen, X., Wang, Y., & Han, S. (2013). Vehicle emission and near-road air quality modeling for Shanghai, China: Based on global positioning system data from taxis and revised MOVES emission inventory. Transportation Research Record, 2340(1), 38–48.
- Liu, H., Fang, C., Zhang, X., Wang, Z., Li, F., & Li, F. (2017). The effect of natural and anthropogenic factors on haze pollution in Chinese cities: A spatial econometrics approach. Journal of Cleaner Production, 165, 323–333.
- Lyons, T., Kenworthy, J., Moy, C., & Dos Santos, F. (2003). An international urban air pollution model for the transportation sector. Transportation Research Part D: Transport and Environment, 8(3), 159–167.
- Madireddy, M., Coensel, B. D., Can, A., Degraeuwe, B., Beusen, B., Vlieger, I. D., & Botteldooren, D. (2011). Assessment of the impact of speed limit reduction and traffic signal coordination on vehicle emissions using an integrated approach. Transportation Research Part D: Transport and Environment, 16(7), 504–508.
- Maze, T. H., Agarwal, M., & Burchett, G. (2006). Whether weather matters to traffic demand, traffic safety, and traffic operations and flow. Transportation Research Record, 1948(1), 170–176.
- Ministry of Ecology and Environment of China. (2018). China vehicle environmental management annual report (2018). Retrieved June 07, 2021, from https://www.mee.gov.cn/xxgk2018/xxgk/xxgk15/201806/t20180601_630215.html
- National Bureau of Statistics China. (2014). China city statistical yearbook. Beijing: China Statistics Press.
- National Research Council (US). (2002). Committee for the evaluation of the congestion mitigation, air quality improvement program, & national research council. In The congestion mitigation and air quality improvement program: Assessing 10 years of experience (Vol. 264). Washington, DC: Transportation Research Board.
- Proost, S., & Van Dender, K. (2012). Energy and environment challenges in the transport sector. Economics of Transportation, 1(1–2), 77–87.
- Rauch, D., & Schleicher, D. (2015). Like Uber, but for local governmental policy: The future of local policy of the ‘sharing economy’. George Mason Law & Economics Research Paper.
- Rayle, L., Dai, D., Chan, N., Cervero, R., & Shaheen, S. (2016). Just a better taxi? A survey-based comparison of taxis, transit, and ridesourcing services in San Francisco. Transport Policy, 45, 168–178.
- Rodier, C., Alemi, F., & Smith, D. (2016). Dynamic ridesharing: Exploration of potential for reduction in vehicle miles traveled. Transportation Research Record, 2542(1), 120–126.
- Schaller, B. (2018). The new automobility: Lyft, Uber and the future of American cities.
- Schrank, D. L., & Lomax, T. J. (2009). Urban mobility report. Texas Transportation Institute, The Texas A&M University System.
- Shaheen, S., & Chan, N. (2015). Mobility and the sharing economy: Impacts synopsis. Berkeley, CA: Transportation Sustainability Research Center, University of California.
- Shenzhen Monthly Statistics Report. Retrieved July 30, 2021, from http://tjj.sz.gov.cn/zwgk/zfxxgkml/tjsj/tjyb/index_5.html
- Shenzhen Municipal Ecological Environment Bureau. (2015). Motor vehicles are the primary source of PM2.5 pollution in Shenzhen. Retrieved June 30, 2021, from http://gdee.gd.gov.cn/shenzhen3073/content/post_2328408.html
- Shenzhen Statistical Bulletin 2018, Retrieved July 29, 2021, from http://www.sz.gov.cn/cn/xxgk/zfxxgj/tjsj/tjgb/content/post_1333687.html
- Shenzhen Statistics. (2017). Shenzhen Monthly Statistics Report Retrieved June 30, 2021, from http://tjj.sz.gov.cn/zwgk/zfxxgkml/tjsj/tjyb/index_5.html,date
- Shi, T., Zhang, W., Zhou, Q., & Wang, K. (2020). Industrial structure, urban governance and haze pollution: Spatiotemporal evidence from China. Science of the Total Environment, 742, 139228.
- Sources of atmospheric PM2.5 in Shenzhen. (2015). Retrieved August 06, 2021, from http://gdee.gd.gov.cn/shenzhen3073/content/post_2328408.html
- Sui, Y., Zhang, H., Song, X., Shao, F., Yu, X., Shibasaki, R., & Li, Y. (2019). GPS data in urban online ride-hailing: A comparative analysis on fuel consumption and emissions. Journal of Cleaner Production, 227, 495–505.
- Treiber, M., Kesting, A., & Thiemann, C. (2008). How much does traffic congestion increase fuel consumption and emissions? Applying a fuel consumption model to the NGSIM trajectory data. In 87th Annual Meeting of the Transportation Research Board, Washington, DC, 71.
- Uber. (2020). Your city. Our promise. Author. Retrieved February 4, 2020, from https://www.uber.com/us/en/about/sustainability
- Wallsten, S. (2015). The competitive effects of the sharing economy: How is Uber changing taxis. Technology Policy Institute, 22, 1–21.
- World Bank Group. (2018). Reducing Traffic Congestion and Emission in Chinese Cities. Retrieved March 30, 2019, from https://www.worldbank.org/en/news/feature/2018/11/16/reducing-traffic-congestion-and-emission-in-chinese-cities
- Wu, T., Shen, Q., Xu, M., Peng, T., & Ou, X. (2018). Development and application of an energy use and CO2 emissions reduction evaluation model for China’s online car hailing services. Energy, 154, 298–307.
- Xue, M., Yu, B., Du, Y., Wang, B., Tang, B., & Wei, Y.-M. (2018). Possible emission reductions from ride-sourcing travel in a global megacity: The case of Beijing. The Journal of Environment & Development, 27(2), 156–185.
- Yu, B., Ma, Y., Xue, M., Tang, B., Wang, B., Yan, J., & Wei, Y.-M. (2017). Environmental benefits from ridesharing: A case of Beijing. Applied Energy, 191, 141–152.
- Zhang, B., Jiao, L., Xu, G., Zhao, S., Tang, X., Zhou, Y., & Gong, C. (2018). Influences of wind and precipitation on different-sized particulate matter concentrations (PM2.5, PM10, PM2.5–10). Meteorology and Atmospheric Physics, 130(3), 383–392.
- Zhang, H., Wang, Y., Hu, J., Ying, Q., & Hu, X. M. (2015a). Relationships between meteorological parameters and criteria air pollutants in three megacities in China. Environmental Research, 140, 242–254.
- Zhang, Y., Wang, X., Li, G., Yang, W., Huang, Z., Zhang, Z., … Zhang, Z. (2015b). Emission factors of fine particles, carbonaceous aerosols and traces gases from road vehicles: Recent tests in an urban tunnel in the Pearl River Delta, China. Atmospheric Environment, 122, 876–884.