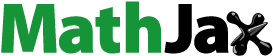
ABSTRACT
Many studies have documented the missing relationship between PCE inflation and the labor market slack in the last 20 years. This paper first presents evidence of a strong sensitivity of price inflation to labor market tightness once we remove the late 90s from our sample and account for the Phillips curve’s endogeneity and nonlinearity. However, the Phillips curve can neither explain nor forecast the Core PCE inflation rate since 1995. This shortcoming is of great concern, given that the Fed utilizes the Core PCE as its primary tool to measure inflation and formulate its monetary policy. Second, we propose the Underlying Inflation Gauge (UIG) and the Median CPI to measure inflation better. We show that the Convex Phillips curve successfully explains and forecasts more than 90% of the UIG inflation movements from 1995:05 to 2019:03
1. Introduction
The effect of unemployment on inflation is a crucial topic in macroeconomic and monetary policy. The simple policy rule is that when the economy operates above (below) its potential level, the inflation rate is expected to be above (below) its two percent target goal. Thus, the Fed adopts a contractionary (expansionary) monetary policy to suppress (stimulate) aggregate spending and move the inflation rate back toward its long-term target rate. The Phillips curve has been the practical and academic tool to measure the relationship between inflation and the slack in the labor and output markets. In the last three decades, this relationship has been weakened as the inflation rate exhibits a fading sensitivity to the labor market tightness. Recently, the Core PCE, the Fed’s measure for inflation, has been below its two percent target despite one of the most extended recoveries in the U.S. economy’s history. For example, the average inflation rate between Q1 2011 and Q1 2020 is only 1.665%. Furthermore, the inflation rate averaged more than 20 points below its 2% target despite the tight labor market where U3 was below its natural rate for an extended period between Q1 2017 and Q4 2019. In addition, the Fed’s FOMC Projections have overestimated the inflation rate by 0.182% since 2016.
Many studies have suggested several possible explanations for the missing relationship between inflation and the labor market slack. Potential causes include better-anchored inflation expectations (Davis, Citation2014), atomization, domestic influence of global activity, and labor market conditions, as stated by Ciccarelli and Mojon (Citation2010), Gurkaynak, Levin, and Swanson (Citation2010), and Jašová, Moessner, and Takáts (Citation2018). Globalization has affected domestic inflation with a cheaper inflow of imports, increased competition from labor-abundant economies, and less sensitivity of the labor cost relative to the business cycle state.
In this paper, we document that the relationship between the Core PCE inflation rate and the slack in the labor market is strong and statistically significant when excluding the observations from the late 90s and accounting for endogeneity and nonlinearity. However, we show that the Phillips curve equation can neither explain nor forecast the Core PCE inflation rate since 1995. We believe that the PCE inflation’s main shortcomings stem from the fact that it focuses only on the price components and excludes both the leading signals of structural changes in the inflation rate and the driving forces behind the large fluctuations in the inflation rate. This shortcoming is of great concern, given that Core PCE strongly influences the Fed’s monetary policy. Next, we provide quantitative support to targeting a more aggregate measure of the inflation rate, namely the Underlying Inflation Gauge (UIG). Amstad, Potter, and Rich (Citation2017, p.2) stated that “The design of the UIG is based on the premise that movements in underlying inflation are accompanied by related persistent changes in other economic and financial series.” We also examine the effectiveness of the Median CPI (CPIM) introduced by the Federal Reserve Bank of Cleveland as another measure of the underlying inflation.
This paper presents evidence that the Phillips curve’s different specifications successfully explain the UIG and the CPIM inflation movements from 1995:05 to 2019:03. In addition, most of the labor market slack terms are statistically significant, indicating a strong sensitivity of price inflation to labor market tightness. The CPIM exhibits the most robust and most persistent effect of the slack in the labor market on the inflation rate. The in- and out-of-sample forecasting measures confirm the Phillips curve’s superior ability to estimate and forecast the UIG inflation.
The paper proceeds as follows. In Section 2, we review the current literature. In Section 3, we describe the empirical models and the data utilized in estimating the different specifications of the Phillips curve with the PCE and the UIG inflation rates. In Section 3, we also account for the endogeneity problem, test the robustness of our results for different subperiods, and evaluate the performance of the in-sample and out-of-sample forecasts of the Phillips curve with the Core PCE, UIG, and CPIM as our measurements for inflation. In Section 4, we discuss the main findings of the paper and conclude.
2. Theoretical review
Inflation has become much less sensitive to movements in unemployment in the last two decades. Blanchard (Citation2016, p.31) stated that “The slope of the Phillips curve, i.e., the effect of the unemployment rate on inflation given expected inflation, has substantially declined. But the decline dates back to the 1980s rather than to the crisis. There is no further evidence of a decline during the crisis.” Atkeson and Ohanian (Citation2001) showed that the Phillips curve slope is unstable between 1960 and 2000. Roberts (Citation2006) showed that the Phillips curve slope decreased by 50% to 67% between 1960:Q1–1983:Q4 and 1984:Q1–2002:Q4. Kuttner and Robinson (Citation2010) confirmed the New Keynesian Phillips curve slope’s flattening slope using 2007:Q3 vintage data relative to that during the sample between 1960:Q1 and 1997:Q4. The most common explanations for this change were sticky prices and wages, better-anchored inflation expectations, globalization, and inaccurate measurements for inflation and the slack in the economy.
2.1. Sticky prices and wages
Tobin (Citation1972), Caballero and Engle (Citation1992), Tsiddon (Citation1993), Ball, Mankiw, & Romer, Citation1988), and others attribute the downward price rigidity to the broad practice of periodic adjustments of nominal prices in a trend inflationary economy. Under this practice, symmetric shocks to nominal prices will trigger faster adjustments in the case of positive shocks. The rationale of such an asymmetric response can be attributed to the decline in relative prices between the periodic adjustments of the nominal prices. Thus, firms will be more inclined to respond to positive shocks that deviate the current relative price further away from its optimal one. In contrast, firms do not need to speed up their nominal price adjustments in response to negative shocks that will be corrected at the predetermined price adjustment. Ball et al. (Citation1988) confirm that nominal price adjustments happen faster and more frequently when economies experience a higher level and volatility of the inflation rate. Based on these results, Ball and Mazumder (Citation2011) show that the Phillips curve slope is steeper when economies experience a higher level and volatility of inflation. Downward wage rigidity also contributes to the explanation of the curvature of the Phillips curve. Ball and Mazumder (Citation2011) show that nominal wage adjustments happen more slowly and less frequently when economies experience a lower inflation rate. Phillips (Citation1958) attributed the wage rigidity to the workers’ reluctance to accept lower compensations when inflation and employment rates are low during recessions. Daly and Hobijn (Citation2014) investigated the downward wage rigidity during the last three recessions in the United States. They attribute the significant curvature of the short-run Phillips curve to the increasing downward wage rigidity triggered by recessions. They also document that the wage rigidity during recessions slows wage inflation during the subsequent recoveries and prolongs the Phillips curve’s curvature. Gagnon and Collins (Citation2019) estimated a generalized version of the Phillips curve. Their specification allowed the change in the inflation rate to respond asymmetrically to the unemployment gap based on the level of the inflation rate, the sign of the unemployment gap, the Federal Reserve’s interpretation of its mandate, and the public’s perception of Fed policy. Gagnon and Collins did not reject the hypothesis that the slope of the Phillips curve has become nonlinear and flat under the low inflationary environment since the late nineties.
2.2. Anchored inflation expectations
Anchored inflation expectations mean that inflation expectations are less responsive to inflation surprises and more tied to the Fed’s 2% inflation target. Although the Fed formally targeted inflation at the 2% level in 2012, the Fed publicly introduced and discussed this policy in 2000. Bernanke (Citation2007) stated that “I use the term ‘anchored’ to mean relatively insensitive to incoming data. So, for example, if the public experiences a spell of inflation higher than their long-run expectation, but their long-run expectation of inflation changes little as a result, then inflation expectations are well anchored.” However, Bernanke (Citation2007) emphasized that long-term inflation expectations are not entirely anchored as they gradually respond to persistent changes in the economic environment and the conduct of monetary policy. Benati (Citation2008), Gurkaynak et al. (Citation2010), Davis (Citation2012), and Davis (Citation2014) show that inflation expectations have become more anchored to inflation targets and less responsive to endogenous and exogenous shocks in the United States and many of the developed and developing countries since adopting inflation targeting. Ball and Mazumder (Citation2011) show that inflation expectations are becoming less sensitive to the Core PCE inflation changes. For example, inflation expectation’s response to a one-unit change in Core inflation decreased from 1.0 to 55 from 1985 to 2010. They also confirm that expectations do not respond to transitory shocks since the early 90s and are tied to the Fed’s 2% inflation target. Thus, anchoring inflation presents a possible explanation of the Phillips curve’s flattening in the last two decades. With more substantial anchored inflation expectations to the Fed’s 2% inflation target, current and future inflation become less responsive to the economy’s slack as measured by the output or the employment gap.
2.3. Globalization
Obstfeld (Citation2019) and Forbes (Citation2019) state that globalization weakens the link between domestic economics slack and the Core PCE inflations through different channels. First, the increased global competition, mobility of trade across borders, and usage of supply chains significantly diminished the bargaining power of labor and firms’ ability to adjust their prices in response to changes in marginal cost. Second, the share of imports in domestic production and final consumption has continuously increased in the last two decades. In addition, during the previous four decades, the U.S. economy has become more exposed to exogenous shocks as its PPP-adjusted share of the global economic activities declined by approximately 5%. More importantly, the percentage of U.S. intermediate imports in GDP has increased by almost 50% since 1995. Thus, inflation not only depends on the slack in the domestic market but also on foreign prices. Third, the strong correlation between commodity prices and the growth rates in the emerging markets has increased the volatility of the commodity prices and their impact on domestic inflation.
Many recent studies try to incorporate the globalization of inflation into the specification of the Phillips curve. For example, Auer, Borio, and Filardo (Citation2017) demonstrate that the growth of trade in intermediate goods and services has been the driving force of the increasing (decreasing) role of the global (domestic) slack in the Phillips curve equation. Auer et al. (Citation2017) attribute the increasing importance of the trade in intermediate goods and services to the expansion of global value chains in response to the broad integration of production processes worldwide. Auer, Levchenko, and Saure (Citation2019) document that globalization explains 51% of the variance of producer price inflation (PPI) in 30 countries. Half of this variance can be attributed to the cross-border propagation of cost shocks through international input–output linkages. They also show that common sectoral shocks, such as those initiated in the energy industry, are the driving force behind PPI’s comovement in the 30 countries. Similarly, Ciccarelli and Mojon (Citation2010) show that inflation in 22 OECD countries has a common factor that can explain 70% of the inflation of their variance from 1960 to 2008. Incorporating the common global factor in the inflation equation for the 22 OECD countries improves the out-of-sample forecasting of the inflation rates. Borio and Filardo (Citation2007), Jašová et al. (Citation2018), and Forbes (Citation2019) incorporate a wide variety of variables to capture the growing impact of globalization on the Phillips equation. These variables include global commodity prices, global slack, exchange rates, import and oil prices, global output gap, and nonlinear exchange rate pass-through. The three studies document the importance of globalization in explaining and forecasting domestic inflation. They also confirm the decreasing role of the domestic slack in explaining the inflation rate.
2.4. Inaccurate measurements
A few researchers argue that the commonly used measurements of inflation, unemployment gap and inflationary expectations, in the Phillips equation, are not the best proxies for capturing the general movements and expectations of the inflation rate and the economy’s slack. For example, Gordon (Citation2013) and Ball and Mazumder (Citation2019) show that short-term unemployment is more effective in capturing the slack’s impact on the Phillips equation’s labor market. Their argument is based on the fact that those who have been unemployed for more than 27 weeks and still looking for a job are not competitive and seriously looking for a job after being unemployed for a long time. Thus, the excess supply or shortage in the labor market should only account for the percentage of short-term unemployed workers in the labor market. Ball and Mazumder (Citation2019, p.6) stated that “Long-term unemployment rose sharply over 2008–9, so the rise in total unemployment was substantial compared to the rise in short-term unemployment. Long-term unemployment has continued to be unusually high relative to short-term unemployment even as total unemployment has returned to prerecession levels. Overall, labor market slack since 2008 is less severe if it is measured by short-term rather than total unemployment, so the Phillips curve predicts a smaller fall in inflation in this case.” Ball and Mazumder (Citation2011) show that the weighted median of industry price changes calculated by the Federal Reserve Bank of Cleveland is more efficient than Core CPI in capturing the underlying inflation trend and removing the inflation rate’s transitory components. Ball and Mazumder (Citation2019) show the weighted median of industry price changes outperforms both the CPI less food and energy and the PCE deflator less food and energy in terms of the magnitude of the short-term unemployment in the Phillips Equationequation(3)
(3) .
3. Empirical specifications and results
In this section, we first replicate Hooper, Mishkin, & Sufi, Citation2020) estimation of the six specifications of the price Phillips curve for the full sample from 1961 to 2018 and two subsamples from 1961 to 1994 and 1995 to 2019.
Our selection of the starting date of the second subsample is dictated by the inception of our preferred UIG measure of inflation. We also replace the Core CPE with our preferred UIG and the CPIM inflation rates and re-estimate the six specifications for the subsample from 1995 to 2019. Then, we tackle the endogeneity and identification problems when estimating the slope of the Phillips curve. Next, we test our estimate’s subsample stability and evaluate the in-sample and out-of-sample performance of our estimates.
3.1. Estimating the linear and nonlinear expectations of augmented Phillips curve models
We start our analysis by closely following Hooper et al., Citation2020) model and data specifications for the six linear and nonlinear Phillips curve models as follows:
3.1.1. The linear Phillips equation
where is the Core PCE inflation,
is the U3 unemployment rate,
is the CBO estimate of the natural rate of unemployment,
is a dummy variable that accounts for Nixon era price and wage controls.
is the quarterly annualized (log) change in the relative price of imported goods, expressed as the ratio of imported goods prices to domestic goods prices. In addition,
is the expected rate of inflation measured by the Fed’s FRBUS model of the U.S. economy.
To introduce nonlinearity to the Phillips, we estimate the following five specifications:
3.1.2. The spline Phillips equation
where is a dummy variable that takes the value of one when
and zero elsewhere. The spline equation introduces the nonlinearity by allowing the Phillips’s slope to vary based on whether the unemployment gap is positive (
, or nonpositive (
.
3.1.3. The cubic Phillips equation
The cubic specification includes the quadratic and the cubic unemployment gap in the Phillips equation to capture the curve’s potential curvature.
3.1.4. The convex1 Phillips equation
3.1.5. The convex2 Phillips equation
The two convex specifications replace the unemployment gap by the ratio of the unemployment gap to the current level of unemployment and the natural log of the unemployment gap to the natural rate of unemployment.
3.1.6. The piecewise quadratic Phillips equation
where is a dummy variable that takes the value of one when
and zero elsewhere.
The piecewise quadratic equation allows for nonlinearity of the curve by including the squared unemployment gap when . In line with Hooper et al. (Citation2020), we estimate the slope of the Phillips curve for the six specifications of the Phillips curve at four states where the economy experiences a slack in the labor market (
), full employment (
, tight labor market (
, and a very tight labor market (
. Next, we test whether the slopes are statistically different from zero. Given that the slopes are nonlinear functions of model estimated parameters, we employ the Delta method to calculate their standard deviation. We also report the average responses in the four phases and continue to assume that
as utilized in Hooper et al. (Citation2020).
Table 1. Phillips curve specifications: core PCE inflation (1961:01–2019:03)
Table 2. Phillips curve specifications: core PCE inflation (1961:01–1994:04)
Table 3. Phillips curve specifications: core PCE inflation (1995:01–2019:03)
3.2. Different measures for inflation
The most common measure of underlying inflation is the Core PCE. Core PCE excludes the most volatile categories, food and energy, to derive inflation’s underlying trend. The Federal Reserve Bank of Cleveland and Dallas introduced different measures for the underlying inflation, such as the CPIM, where expenditure weight is in the 50th percentile of price changes. Rich and Steindel (Citation2007) show that excluding food and energy does not generate a better Core PCE performance relative to the other Core inflation measures such as Median PCE and Exponentially Smoothed PCE. Rich and Steindel (Citation2007) attribute their finding to the changing nature of the transitory price movements and the fact the some of the omitted movements in food and energy might impact the underlying inflation. Amstad et al. (Citation2017) constructed the UIG from a comprehensive set of variables covering 223 disaggregated price data series in the CPI and 346 series of macroeconomic and financial data. Amstad et al. (Citation2017) utilize the factor model to extract the underlying inflation at frequencies of 12 months or longer. The authors attribute the factor analysis’s superior ability to remove the underlying inflation to the following three reasons: The factor analysis’s ability to deal with a significant amount of information, flexible methodology to extract the Core components of inflations without any predetermined criteria, and ability to remove the persistence components of the large fluctuations of the price level rather than excluding or trimming them. The authors also show that the UIG is less volatile and has a better forecasting accuracy than the Core PCE and the trimmed and medium CPI and PCE published by the Federal Reserve Bank of Cleveland and Dallas. To examine our results’ sensitivity to different measures of inflation, we replace the Core PCE with the “full-data-set” UIG inflation and the CPIM and re-estimate the six Phillips specifications for the second subsample from 1995 to 2019.
First, we investigate whether a change in UIG or CPIM can predict a change in Core PCE. The Granger causality tests are applied for all the lagged UIG and CPIM using the F test in the Core PCE equation. We utilize Akaike (AIC) and the Schwarz (BIC) criteria to set the number of lags for each regression where the maximum lag is set up to four. shows that the Granger causality test detects the strong influence of both the UIG and CPIM on the core PCE, especially when the number of lags exceeds two.
Table 4. Results of Granger causality (1995:01–2019:03)
Next,
Table 5. Phillips curve specifications: UIG inflation (1995:01–2019:03)
Table 6. Phillips curve specifications: CPI median inflation (1995:01–2019:03)
3.3. Endogeneity and globalization of the inflation rate
Many studies have raised concern about the Phillips curve’s identification problem due to the Fed’s active policy to minimize the output and the inflation gaps in response to supply shocks. As pointed out by McLeay and Tenreyro (Citation2019), Hooper et al. (Citation2020), Eberly, Stock, & Wright, Citation2020), and others, the active role of monetary policy to stimulate the economy and increase inflation when the output (unemployment) gap is negative (positive) has generated a strong correlation between the slack term and the disturbances in the Phillips curve. Without the assumption that the disturbances and the regressors are uncorrelated, none of the OLS estimated coefficients would be consistent or unbiased. In our paper, we apply two strategies to account for the endogeneity bias in the Phillips equation. First, we isolate the effect of supply shock when estimating the slope of the Phillips curve. We accomplish this task by including various supply shock measures such as import and oil price inflation, exchange rate, global output gap, and inflation gap. We determine the final selection of these variables based on their statistical significance and their contribution to the Phillips curve equation’s overall fit. Second, we utilize instrumental variables and re-estimate the Phillips equation with the GMM method (IV-GMM). As pointed out by Galí and Gambetti (Citation2019), Hooper et al. (Citation2020), and Eberly (2019), the instrumental variables should be independent of the contemporaneous supply shocks. In line with Eberly (2019) and Galí and Gambetti (Citation2019), we utilize monetary policy shocks as our instrumental variable. We define the instrumental shocks as the residuals from regressing the Wu–Xia Shadow Federal Rate on different measures of supply shocks. We also include the lagged unemployment gap to estimate the slope of the Phillips curve correctly. The rationale of having the lagged unemployment gap is clearly explained by McLeay and Tenreyro (Citation2019). McLeay and Tenreyro (Citation2019, p 12) stated that “This policy-induced persistence means that the lagged output gap can be used as an instrument for the current output gap. Intuitively, the policymaker chooses to create an output gap even after the cost-push shock has disappeared. They commit to do so in order to achieve better inflation outcomes when the shock originally occurs.”
In our analysis, IV-GMM employs the following set of variables:
3.3.1. Shadow fed funds rate
Wu and Xia (Citation2015) introduce a proxy rate that can move below the Zero Lower Boundary during recessions. This modification allows the Federal Funds Rate to capture the expansionary monetary policy’s effect when the actual rate approaches the Zero boundary. Wu and Xia (Citation2015) created a shadow rate term structure model (SRTSM) that can be applied directly to discrete-time data. They developed a closed-form expression to approximate the forward rate in the SRTSM and used an extended Kalman filter for estimation.
3.3.2. Relative foreign prices
Laubach and Williams (Citation2003) capture the impact of relative foreign prices in their model by including both the Core import price inflation of nonpetroleum goods ( and crude oil import price inflation (
.
3.3.3. Global output gap (
)
We follow Jašová et al. (Citation2018) to calculate the Global output gap by employing the standard univariate Hodrick–Prescott filtering method for the main U.S. trade partners: Canada, China, Germany, Japan, Korea, Mexico, and the U.K. We use the standard smoothing parameter λ set to 1600. Then, we combine all the gaps weighted by their import weight to create the global output gap.
3.3.4. Global inflation (

We measure the global inflation as the average CPI inflation rate of the seven main trade partners weighted by their import share of the total imports from the seven countries.
3.3.5. Exchange rate (EX)
Dornbusch and Krugman (Citation1976) stated, “The link between exchange-rate deterioration and domestic inflation takes on importance in the context of our earlier argument that an expansionary monetary policy leads to a fall in the exchange rate. The current line of argument establishes a direct, short-run link between monetary policy and inflation. The conventional case for stabilization policy, including the monetary policy – that it acts promptly on quantities and only slowly on prices – is, therefore, lost” (1976, p. 9). Thus, we account for the domestic inflation rate’s response to the U.S. exchange rates’ changes due to imbalances in the current and capital accounts and changes in the monetary policy by including the percentage change in the BIS effective exchange rate in our equations.
3.3.6. 

We also include the NBER-based Recession Indicators to account for the U.S. Business Cycle Expansions and Contractions.
In summary, IV-GMM Phillips equations can be defined as follows:
We also define the monetary policy shock as the residuals from regressing the shadow Federal Funds rate on its four lags, four lags of , four lags of
, one lag of
,
,
,
, and
. The set of instrumental variables in the GMM estimation includes the first four lags of both the monetary shocks and the slack variable and the remaining predetermined regressors on the Phillips equation.
reports IV-GMM and the OLS estimation of the convex1 specification for the Core PCE, UIG, and the CPIM for the period from 1995 to 2019. The table shows that the Core PCE Phillips curve slope is statistically significant only at the 10% level. In contrast, the slopes of the UIG and CPIM are statistically different from zero at the 5% and 1% level. Also, the null hypothesis that the instruments are weak in the IV procedure’s first stage is rejected for all the specifications. also confirms the poor performance of the PCE equation as illustrated by the very low R2_adjusted and the high RMSE. The UIG Phillips equation outperformed the CPIM in terms of R2_adjusted (.948 versus 601) and RMSE (0.152 versus 0.395). We also report the Bayesian Information Criterion (BIC), given that the different specifications are nonnested. The smallest BIC values for the UIG confirm the outperformance of the UIG. However, it is important to emphasize that we are not utilizing R2-squared statistics to select the specification with the highest overall fit but to select the inflation rate that the Fed and the public can effectively predict and target.
Table 7. Phillips curve convex1 specification PCE, UIG, CPIM inflation (1996:03–2019:03)
confirms the superior performance of the UIG Phillips equation in explaining the UIG inflation. However, the CPIM Phillips equation has a much steeper average slope than the UIG equation (–0.369 versus – 0.11). Finally, J-statistics cannot reject the null and confirm the IV-GMM estimate’s quality and the satisfaction of the moment conditions underlying GMM.
3.4. Subsample stability of the Phillips curve’s slope
This section examines the stability of the slope of the Core PCE, UIG, and CPIM Phillips curves between 1995 and 2019. Many of the recent studies show that the slope of the Phillips curve is flat and statistically insignificant. The stability of the relationship between inflation and unemployment is crucial for policymakers to achieve the Fed’s dual mandate. Subsample stability tests can detect the impact of early or late observations on the causality between inflation and unemployment. To further explore potential structural and coefficient instability in the inflation rates, we employ the recursive version of Bai and Perron (Citation1998, Citation2003) tests, which determines the structural breaks even though the exact break dates are unknown. We set the maximum number of breaks (k) to 5, the trimming value at 0.15 (i.e., each regime contains at least 15% of the observations), and the breaking parameter to 5. We also employ the AIC to determine the lag length in each equation. reports the number of breaks by repeatedly testing the null of no breaks against k breaks’ alternative, increasing k until the null cannot be rejected or until the maximum number of permitted breaks is reached. Among all cases where the null is rejected (each case with a different total number of breaks), we report the one associated with the minimum Bayesian Information Criterion (BIC), as proposed by Yao (Citation1988). the BIC statistics select five breaks for the PCE and CPIM and three breaks for the UIG. The table also reports the 95% confidence interval for the breaks in the three inflation rates. In sum, the analysis confirms the time-varying nature and the structural instability of the inflation rates.
Table 8. Bai-Perron test for multiple structural break
To account for the potential structural instability in PC equations, we run three rolling IV-GMM estimations: forward, window, and backward. We begin by estimating EquationEquation 7(7)
(7) over the first 13 years from 1996:03 to 2009:03. We then add one observation to the end date and re-estimate the equation. We repeat this process for 41 samples until the last sample is estimated from 1996:03 to 2019:03. This sequence of results is referred to as the rolling forward regression. Next, we estimate EquationEquation 7
(7)
(7) over a moving window of 53 observations in length. We begin by assessing EquationEquation 7
(7)
(7) over the first 53 observations from 1996:03 to 2009:03. Then, we drop one observation from the start of the previous sample for each regression and add one to the end. We repeat this process for 41 windows until the last sample from 2006:03 to 2019:03 is covered. This sequence of results is referred to as the rolling window regression. Finally, we estimate EquationEquation 7
(7)
(7) from 2006:03 to 2019:03. We then add one observation to the start date of the previous sample, in backward chronological order. We repeat this process for 41 samples until the entire sample from 1996:03 to 2019:03 is covered. This sequence of results is referred to as the rolling backward regression. The three rolling iterations assigned different weights to the other time periods and can locate most of the structural changes in the relationship between inflation and unemployment. For example, the forward (backward) regressions assign more weights to the sample’s early (late) observations. In contrast, the window regressions are more sensitive to dropping and adding new observations at each iteration. In addition, the window regressions present estimates that are less influenced by either early or late observations.
reports the estimated coefficients on the unemployment gap ratio to U3 and their p-values in the Core PCE, UIG, and CPIM. also reports the number of the statistically significant coefficients in the 41 forward, window, and backward iterations and their average value for the three inflation measures. illustrates the R2_adjusted of the Core PCE, UIG, and CPIM convex1 Phillips curves. In addition, shows the average R2_Adjusted obtained from the forward, window, and backward iterations are 0.17, 0.96. and 0.65 in the case of the Core PCE, UIG, and CPIM. The results reveal interesting empirical findings. First, the CPIM equation exhibits the steepest Phillips curve’s slope, and the coefficients on the slack economic terms are statistically significant 100 percent of the time. Second, the relationship between the Core PCE inflation and the economic slack was fragile during the late 90s and the early years of the 2000s. When our sample includes these early observations, the slack term’s coefficients are statistically insignificant most of the time, as illustrated by the forward regressions. In contrast, the relationship between the Core PCE inflation and the slack term is much stronger during the last 10 years from 2009 and 2019, as indicated by the fact that when backward and window iterations include these sample periods, the slack term’s coefficients are statistically significant in 39 out of the 41 iterations. Further, the absolute values of the slack’s coefficients in the Core PCE equation are larger in the case of the window (–1.359) and backward (–0.789) iterations than that in the case of the forward iterations (–0.540). Third, a similar pattern is observed for the backward iterations of UIG inflation, where the estimated coefficients on the slack term are statistically significant in the 41 iterations and have an average of – 0.443. The estimated coefficients in both the forward and window iterations are statistically significant in 31 iterations. However, the average value (–0.777) of the window rolling estimated coefficients far exceeds those of the forward interactions (–0.310). Fourth, the changes in the historical inflation rate can be better explained in the case of the UIG as illustrated by the average R2_Adjusted
Table 9. Phillips curve convex1 specification GMM estimation
Table 10. Average R2_Adjusted
Figure 2. Estimated confficients on the (unemployment gap/U3) and their P-Values.

Figure 3. R2 adjust: core PCE, UIG, and CPIM.

To have a closer look at the relationship between the three inflation rates and the unemployment rate, we plot the Phillips curve slope from the window rolling in . The figure confirms that the slope of the CPIM Phillips curve has been getting steeper since 2010. The slope of the Core PCE and UIG shared the same trend until the end of 2017 and got flatter during 2018 and 2019. For the most recent iteration from 2006:03 to 2019:03, the slopes of the Core PCE, UIG, and CPIM are – 0.15, – 0.20, and – 0.27, respectively. In summary, the relationship between both the UIG and the Core PCE and the slack term during the early periods of our samples during the nineties is weak. Thus, these early observations could mask the relationship between the Core PCE and UIG inflation rates and the unemployment rate during the last decade as illustrated flattened the slope of the Phillips curve.
3.5. Out-of-sample performance of the Phillips curve equation
We conclude our empirical work with the out-of-sample evaluation of the Core PCE, UIG, and CPIM Phillips curve. We restricted our analysis to the rolling window regressions where the sample results represent each iterated sample period with less bias to the early or the late observations. At each window rolling, we calculate the four-step dynamic forecasts. Next, we evaluate the relative efficiency of the out-of-sample forecasts. We calculate Theil U, the mean absolute prediction error (MAPE), and the root mean squared prediction error (RMSPE). Given the different inflation rates utilized in our study, Theil U provides a scale-independent measure that can facilitate the comparison of the out-of-sample forecasts of the Core PCE, UIG, and CPIM Phillips curves. confirms the UIG Phillips equation’s better performance as illustrated by the lowest values of the Theil U. In contrast, the Core PCE did worse than naive forecasting, as illustrated by the Theil U that reasonably exceeds one. The table also shows that UIG’s MAPE and RMSPE are less than half of the Core PCE and CPIM. In addition, CPIM’s forecasting performance is better than the Core PCE’s performance, but inferior to UIG’s performance. plots the one-, two-, three-, and four-quarter forecasts against their actual inflation rate. The figure confirms the accurate estimates generated by the UIG Phillips curve.
Table 11. Comparison of Core CPI, UIG, and CPIM rolling out-of-sample forecasts: Window regression
4. Conclusion
The missing relationship between the inflation rate and the labor market slack has been the focus of numerous studies in the last decade. The most common explanations for the Phillips curve’s flattening are sticky prices and wages, better-anchored inflation expectations, globalization, inaccurate measurements for inflation, and the slack in the economy. This paper shows that the relationship between the Core PCE inflation and the slack term is much stronger once we account for nonlinearity, endogeneity, globalization, and subsample instability. However, the Core PCE’s current utilization to measure the relationship between inflation and unemployment poses a challenging task to policymakers and the public. The Core PCE Phillips curve exhibits poor in-sample and out-of-sample performance for the sample periods extending from 1995 to 2019. In this paper, we propose the UIG and CPIM as more reliable measures of inflation. The Convex Phillips curve has been successful in explaining and forecasting the UIG inflation rate since 1995. In addition, the CPIM persistently exhibits a steeper and statistically significant slope for the Phillips curve. Thus, our study emphasizes the importance of looking behind the Core PCE as the primary measure for the inflation rate and confirms that the Phillips curve remains a useful monetary policy tool.
Disclosure statement
No potential conflict of interest was reported by the author(s).
Additional information
Notes on contributors
Hany Guirguis
Professor Hany S. Guirguis received his PhD. in Economics from the University of Oregon. Dr. Guirguis has taught numerous graduate and undergraduate courses in finance, econometrics, statistics, accounting, and economics. He specializes in financial modeling and applied econometrics and has published more than fifty articles in several finance and economics journals.
Tin Shan (Michael) Suen
Professor Suen has more than 25 years of capital markets experience and more than 10 years of academic experience. His expertise covers applied econometric and data science. Research interest includes applied empirical research related to assets management and applied econometric analysis.
References
- Amstad, M., Potter, S., & Rich, R. (2017). The New York fed staff Underlying Inflation Gauge (UIG). Economic Policy Review, 23, 1–32.
- Atkeson, A., & Ohanian, L. (2001). Are Phillips curves useful for forecasting inflation? Federal Reserve Bank of Minneapolis Quarterly Review, 25, 2–11.
- Auer, R. A., Borio, C., & Filardo, A. (2017). The globalisation of inflation: the growing importance of global value chains. Federal Reserve Bank of Dallas Working Paper No. 300.
- Auer, R. A., Levchenko, A. A., & Saure, P. (2019). International inflation spillovers through input linkages. The Review of Economics and Statistics, 101(3), 507–521.
- Bai, J., & Perron, P. (1998). Estimating and testing linear models with multiple structural changes. Econometrica, 66(1), 47–78.
- Bai, J., & Perron, P. (2003). Computation and analysis of multiple structural change models. Journal of Applied Econometrics, 18(1), 1–22.
- Ball, L., Mankiw, N. G., & Romer, D. (1988). The new keynesian economics and the output-inflation trade-off. Bpea, 1, 1–65.
- Ball, L., & Mazumder, S. (2011). Inflation dynamics and the great recession. Brookings Papers on Economic Activity, 42(1), 337–381.
- Ball, L., & Mazumder, S. (2019). A Phillips Curve with anchored expectations and short‐term unemployment. Journal of Money, Credit, and Banking, 51(1), 111–137.
- Benati, L. (2008). Investigating inflation persistence across monetary regimes. Quarterly Journal of Economics, 123(3), 1005–1060.
- Bernanke, B. (2007). Inflation expectations and inflation forecasting. Speech at the Monetary Economics Workshop of the NBER Summer Institute, Cambridge, Massachusetts (July 10).
- Blanchard, O. (2016). The Phillips curve: back to the ‘60s? American Economic Review, 106(5), 31–34.
- Borio, C., & Filardo, A. (2007). Globalisation and inflation: new crosscountry evidence on the global determinants of domestic inflation. BIS Working Papers No 227.
- Caballero, R., & Engle, E. M. R. A., (1992). Price rigidities, asymmetries, and output fluctuations. NBER Working Paper # 4091.
- Ciccarelli, M., & Mojon, B. (2010). Global Inflation. The Review of Economics and Statistics, 92(3), 524–535.
- Daly, M. C., & Hobijn, B. (2014). Downward nominal wage rigidities bend the Phillips Curve. FRB San Francisco Working Paper 2013-08.
- Davis, J. S. (2012). Inflation expectations have become more anchored over time. Federal Reserve Bank of Dallas Economic Letter, 7(13), 1–4.
- Davis, J. S. (2014). Inflation targeting and the anchoring of inflation expectations: cross-country evidence from consensus forecasts. In Federal Reserve Bank of Dallas Working Paper,174.
- Dornbusch, R., & Krugman, P. (1976). Flexible exchange rates in the short run. Brookings Papers on Economic Activity, 7(3), 537–575.
- Eberly, J. C., Stock, J. H., & Wright, J. H. (2020). The federal reserve’s current framework for monetary policy: a review and assessment. International Journal of Central Banking, 16, 5–71.
- Forbes, K. J. (2019). Has globalization changed the inflation process? Monetary and Economic Department, BIS Working Papers, 791.
- Gagnon, J., & Collins, C. G., (2019) Low inflation bends the Phillips Curve. Peterson Institute for International Economics Working Paper No. 19-6, doi: 10.2139/ssrn.3363928.
- Galí, J., & Gambetti, L. (2019). Has the U.S. wage Phillips curve flattened? A semi-structural exploration. National Bureau of Economic Research working paper 25476.
- Gordon, R. (2013). The phillips curve is alive and well: inflation and nairu during the slow recovery. National Bureau of Economic Research. Working Paper 19390.
- Gurkaynak, R., Levin, A., & Swanson, E. (2010). Targeting anchor long-run inflation expectations? evidence from long-term bond yields in the U.S., U.K., and Sweden. Journal of the European Economic Association, 8, 1208–1242.
- Hooper, P., Mishkin, F. S., & Sufi, A. (2020). Prospects for inflation in a high pressure economy: is the Phillips curve dead or is it just hibernating. Research in Economics, 74(1), 26–62.
- Jašová, M., Moessner, R., & Takáts, E. (2018). Domestic and global output gaps as inflation drivers: What does the Phillips curve tell? Bank for International Settlements, Working Papers, No 748.
- Kuttner, K., & Robinson, T. (2010). Understanding the flattening Phillips curve. North American Journal of Economics and Finance, 21(2), 110–125.
- Laubach, T., & Williams, J. C. (2003). Measuring the natural rate of interest. Review of Economics and Statistics, 85(4), 1063–1070.
- McLeay, M., & Tenreyro, S. (2019). Optimal inflation and the identification of the Phillips Curve. National Bureau of Economic Research working paper 25892.
- Newey, W. K., & West, K. D. (1987). A simple, positive semi-definite, heteroskedasticity and autocorrelation consistent covariance matrix. Econometrica, 55(3), 703–708.
- Obstfeld, M. (2019). Global dimensions of U.S. Monetary Policy. NBER Working Paper No. 26039.
- Phillips, A. W. (1958). The relationship between unemployment and the rate of change of money wages in the United Kingdom 1861–1957. Economica, 25, 283–299.
- Rich, R., & Steindel, C. (2007). A comparison of measures of core inflation. Economic Policy Review, Federal Reserve Bank of New York, 13, 19–38.
- Roberts, J. (2006). Monetary policy and inflation dynamics. International Journal of Central Banking, 2, 193–230. dx
- Tobin, J. (1972). Inflation and Unemployment. American Economic Review, 62, 1–18.
- Tsiddon, D. (1993). The (Mis)behavior of the Aggregate Price Level. Review of Economic Studies, 60(4), 889–902.
- White, H. (1980). A heteroscedasticity-consistent covariance matrix estimator and a direct test for heteroscedasticity. Econometrica, 48(4), 817–838.
- Wu, J., & Xia, F. (2015). Measuring the macroeconomic impact of monetary policy at the zero lower bound. Journal of Money, Credit, and Banking, 48(2–3), 253–291.
- Yao, Y. C. (1988). Estimating the Number of Change-points via Schwarz Criterion. Statistics and Probability Letters, 6(3), 181–189.