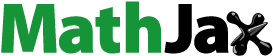
ABSTRACT
Innovation capability of manufacturing industry is one of the most important factors that determine a country’s competitiveness. This paper expounds the theoretical and empirical relationship among innovation capability (IC), capital enrichment (CE) and total factor productivity (TFP) by using the yearly panel data of 28 Chinese manufacturing segments from 2011 to 2018. The intermediary effect model and threshold model are used for empirical analysis, findings suggest that 1) IC not only directly promotes TFP but also indirectly through CE, indicating that CE has an intermediary effect on the relationship between IC and TFP. 2) The positive effect of CE on TFP is influenced by the “double threshold effect” of IC. Compared with industries having low IC, this positive effect in high IC industries is first enhanced then weakened and later re-enhanced. 3) There is a significant difference in IC and CE among different manufacturing segments.
1. Introduction
IC is of great significance for manufacturing industry to raise its competitiveness to serve not only the Chinese economy but also contribute to the world's economy as the largest exporter in the world. IC is a strategic tool to move towards the high-end of the global value chain and an essential way to improve TFP. It is a powerful driving force for economic transformation and upgrading. Since the 1978 economic reforms, China’s GDP grew from RMB 367.8 billion to RMB 99 trillion in 2018, with an average growth rate of 9.5%, making it the largest emerging market economy in the world. Manufacturing value-added has steadily ranked first since 2010 and the TFP has improved significantly which received extensive attention from the other countries. Innovation drives less productive industries out of the market as survival is for the fittest only in contemporary fierce competition, and produces high-tech and more productive companies as a result (Bloom & Van Reenen, Citation2007). It promotes industrial development and drives innovation-led demand and economic growth (Aghion Citation2014; Aghion & Howitt, Citation2009; Antonelli, Citation2003). Brandt, Van Biesebroeck, and Zhang (Citation2012) find that 60% of the TFP growth in China’s manufacturing segments is contributed by new entrants with innovative ways of doing business, fully demonstrating the positive effect of innovation on economic growth.
Seen from the action path, innovation is the recombination of production factors (Schumpeter Citation1912), which can improve the learning process of an organization (Torres, Gutierrez, & Dominguez, Citation2010). Chang and Oxley (Citation2014) and Feder (Citation2018) suggest that innovation changes the original production factor combination, leading to reduce the unit factor cost by optimizing configuration reflected in profit increments. Innovation development causes capital inflow and spatial allocation, which accelerates the reallocation of the labor force and capital elements (Hausmann & Rodrik, Citation2003; Hsieh and Klenow Citation2009). The promotional effect of the increase in capital on TFP has been confirmed in endogenous growth theory (Howitt, Citation1998). Empirical studies also verify the positive correlation between these two variables from different perspectives (Salinas-Jimenez et al. Citation2006; Kose, Prasad, & Terrones, Citation2009; Chemmanur, Loutskina, & Tian, Citation2014; Raymond Citation2015; Feder, Citation2018). Naomi (Citation2016) further finds that innovative capital plays an important role in the growth of TFP.
However, influenced by financing constraints, factor endowments, market system, and other factors (Bernstein, Citation2015; Brown James & Petersen, Citation2011; Cornaggia, Mao, Tian, & Wolfe, Citation2015; Qian Xuesong et al. Citation2018; Su Hang et al. Citation2017), China’s manufacturing industry is in the period of surplus capital consumption. The problem of insufficient investment in innovative capital has not been solved effectively. According to the data provided by the World Bank, WTO Database, and Chinese National Bureau of Statistics, the R&D investment intensity in 2017 was only 1.98%, far below that in South Korea (3.67%), Germany (3.36%), Japan (3.05%), United States (2.58%), and other nations with powerful manufacturing industries. The positive effect of capital on TFP is seriously hindered and resulting in TFP in China at a low level (Hsieh & Klenow Peter, Citation2009; Young, Citation2003).
Given the background of continuous escalation of China-U.S. trade friction, will capital be enriched in the Chinese manufacturing industry? If yes, how do IC and CE impact manufacturing TFP? Is the promotional impact of CE on TFP affected by IC? If yes, what is its specific form or influencing mechanism? These are some of the research questions which we attempt to answer in this study. Based on the panel data from 2011 to 2018, this paper firstly analyzes the interaction mechanism between IC, CE and TFP then use the panel threshold model to explore whether the relationship between CE and TFP is affected by the threshold effect of IC. This paper contributes to the existing body of knowledge in some ways as follows:
First, contemporary research lacks theoretical background and empirical support on the phenomenon of CE. Based on the panel data of 28 Chinese manufacturing segments, this paper provides evidence for the existence of CE. It further analyzes the transmission mechanism among IC, CE, and TFP, and explores the intermediary effect of CE on the relationship between IC and TFP.
Second, the previous studies have ignored the non-linear relationship between CE and TFP (differential effect of CE on TFP under different levels of IC). This non-linear relationship has an important theoretical and practical significance for maximizing the role of CE. This paper uses the panel threshold regression model to estimate the double threshold effect of IC on TFP, which obtains more detailed research conclusions.
Third, further analysis reveals that the average IC and CE levels vary greatly among different manufacturing industries. Based on empirical findings, we put forward corresponding suggestions from IC and CE perspective, for theoretical reference and policy-making.
2. Literature and hypotheses development
Existing research on the relationship between innovation and economic development is mainly divided into two factions, the first is the Neoclassical Economic Growth Theory proposed by Solow, and the second is the Theory of Economic Development put forward by Schumpeter. Neoclassical Economic Growth Theory assumes that technological progress is an exogenous variable and independent of factor input, so technological progress is the fundamental driving force of economic growth (Solow, Citation1956). While the Theory of Economic Development reveals the influence of innovation on economic development for the first time by using innovation theory. Subsequently, the new Schumpeter Growth Theory presented by Romer (Citation1986) and Lucas (Citation1988) further demonstrates the promotional effect of innovation on sustained economic growth. They propose that innovation is the result of investment in new technology and knowledge that has a fundamental impact on economic development as a result (Romer, Citation1986).
The continuous emergence of innovation provides the possibility for technologically backward countries or regions to catch up in technology and economic development with advanced countries (Heshmati and Kumbhakar Citation2010; Madsen, Ang, & Banerjee, Citation2010). Gancia and Zilibotti (Citation2005) report the key role of technological progress in a country’s long-term stable economic growth based on endogenous economic growth theory. Griffith, Huergo, Mairesse, and Peters (Citation2006), Comin and Hobijn (Citation2010), and Chang and Oxley (Citation2014) point out that innovation promotes productivity growth through the optimized combination of production factors. The factor inflow caused by innovation improves industry productivity (Collardwexler & Loecker, Citation2013; Crespi & Zuniga, Citation2012). Meanwhile, some studies find that innovation can narrow the technological gap with technology leaders and promote the TFP growth (Li, Loyalka, Rozelle, & Wu, Citation2017; Miyagiwa, Huasheng, & Vandenbussche, Citation2010; Stollinger, Citation2013; Tientao Citation2016). Kale and Rath (Citation2018) indicate that there is a long-term co-integration relationship between innovation and TFP growth and an increase in overall innovation activities promotes the growth in TFP. The IC determines an innovation-driven effect on TFP (Roper, Du, & Love, Citation2008).
H1: IC of Chinese manufacturing industry plays a positive effect on TFP.
The manufacturing industry is a leading force of national innovation, and the impact of its IC on TFP cannot be ignored (Kale & Rath, Citation2018). However, the IC itself is not exogenous (Rui Citation2019). With the improvement in IC, the technology spillover effect and value-added become higher and higher. The capital allocation efficiency in fields of high value-added, high-tech, and high-branding determined by core technology also becomes improved. Given the profit-seeking nature of capital, it makes the highly innovative industries attract more capital which further promotes technological progress and innovation behavior through capital deepening (Laursen, Masciarelli, & Prencipe, Citation2012). This conforms to the judgment standard of capital allocation efficiency proposed by Jeffrey Wurgler (Citation2001) i.e., capital always goes to industries with a higher return on investment (ROI) which contributes to TFP growth also.
As new capital flows towards innovative industries only, the increase in capital (accumulation) represents the increase in technological innovation in certain cases as supported by Blackburn and Cipriani (Citation2005). It means that CE affects TFP indirectly through innovation. Some empirical studies demonstrate the positive effect of capital on TFP from the perspective of R&D (e.g., Bagherzadeh, Citation2012; Medda et al. Citation2014; Vithessonthi et al. Citation2016). Tientao et al. (Citation2016) point out that each 10% increase in R&D capital can improve TFP by 0.77%, while others believe that actual improvement may be less than that (e.g., Borgo, Goodridge, Haskel, & Pesole, Citation2013; Goodridge, Haskel, & Wallis, Citation2018).
According to the Insufficient Effective Demand Theory proposed by Keynes, there is the law of diminishing marginal utility of capital (Keynes, Citation1936). Under the premise that technical equipment and other conditions remain unchanged, the expected profit rate generated by capital investment will continue to decline with the expansion of capital in the industry. So the unilateral increase in capital scale may have a limited effect on TFP. On the premise that spontaneous innovation cannot be followed up, it is difficult to achieve effective economic growth by relying solely on increasing capital investment. From the perspective of economics, assuming that there are two economic industries and
, the production function model is as follows.
Where ,
and
represents the output, the amount of capital and labor, respectively.
is capital intensity.
Assuming that the two industries have the same wage , and the interest rate
, then the per capita capital
is
. The output value of the above two economic sectors is
and
, respectively.
Where is the price. The marginal capital-output of the whole society is as follows.
Assuming that the per capita capital stock is at the
period, and the per capita output is
at the
period. The exogenous technological progress or innovation occurs in
, then the capital intensity
of the entire society increases. According to the equation
and
, the per capita output
will increase, as will the growth rate of TFP. Then the following equation s obtained.
It means that in industries with high IC, the marginal capital-output increases, which will affect the promotion effect of CE on TFP. The faster CE grows , the faster the per capita output increases
, and the faster the growth rate of TFP
. Therefore, the impact of IC on TFP is likely to play a role through CE, especially the marginal capital-output. Based on these arguments, the following hypothesis is proposed.
H2: CE plays an intermediary role in the relationship between IC and TFP.
There are significant differences in size, technology usage, technological change, industrial policy, factor market, and other factors among various industries (Aghion, Dewatripont, Du, Harrison Ann, & Legros, Citation2015; Banerjee et al. Citation2005; Restuccia et al. Citation2008). The IC level is also different among different industries, and CE may have varying levels of effects on TFP.
From the above discussion, it can be found that the impact of CE on TFP is constrained by IC. Once the innovation activities are interrupted, the organization will suffer heavy losses due to excessive adjustment costs (Booth, Ntantamis, & Zhou, Citation2015; Seybert, Citation2010), which will go against the TFP growth. Therefore, it is necessary to strengthen the matching increase between innovation and capital by increasing innovation investment and capital allocation efficiency to promote the transfer of production factors to high-profit industries (Kong, Mi, & Gao, Citation2015). In this way, the overall productivity tends to rise and total income expands (Kirchner, Citation2016; Sulistyo & Siyamtinah, Citation2016; Yu, Zhang, & Sun, Citation2019).
For manufacturing industries with low levels of IC, the CE space is compressed which is likely to cause repeated divestment or capital outflow. The decrease in capital allocation efficiency may result in the low development trap of TFP (Brandt et al., Citation2012). More seriously, it may result in the hollow development of the whole industry and create an imbalance in the economic structure. Considering the effect of CE on TFP (Brandt et al., Citation2012; Hsieh & Klenow Peter, Citation2009; Vollrath, Citation2009), the decrease in capital allocation efficiency will have a negative effect on TFP.
On the other hand, for industries with higher levels of IC, capital investment behavior is more active which is conducive to absorbing more capital into the industry, promoting the inflow of capital and its spatial allocation. It has a significant positive effect on utilization efficiency and reallocation of labor, technology, and other elements. Under the premise of high IC, CE greatly reduces factor distortion, which is crucial to TFP growth (Bartelsman Citation2013; Boedo & Mukoyama, Citation2012; Zhu, Citation2012). The benign interaction between IC and CE can improve the allocation efficiency of capital and return on invested capital. This means that compared with industries having low IC, industries with high IC can obtain more capital investment, higher capital allocation efficiency, and return rate. Coupled with the national policy guidance and support for such industries, this is a decisive factor of TFP (Ruttan & Hayami, Citation1984), which further stimulates the production, dissemination, diffusion, and application of scientific and technological knowledge (Fagerberg & Srholec, Citation2008; Niosi & Richard, Citation2008). Therefore, higher IC is conducive to giving full play to advantageous effects of CE and achieving a high level of TFP. Based on these arguments, this paper proposes the following research hypothesis
H3: The positive effect of CE on TFP is influenced by the threshold effect of IC.
3. Research design
3.1. Basic model construction
According to the definition proposed by Baron and Kenny (Citation1986) and MacKinnon and Fairchild (Citation2009), the basic premise of intermediary effect is that the explanatory variable is significantly correlated with the explained variable. Meanwhile, hypothesis 1 suggests that IC has a positive effect on TFP. So the relationship between IC and TFP is firstly discussed. Based on the theoretical analysis above, the following basic model is constructed.
Where TFP is the total factor productivity, is the constant term,
is the slope term,
is the innovation capability of
manufacturing industry at the time
,
is the control variables, and
represents the stochastic disturbance term.
Referring to Imbriani, Pittiglio, and Reganati (Citation2010), Ozturk (Citation2015), Brandt and Thun (Citation2016), the control variables include 1) R&D density, measured by the ratio of R&D expenditure to total industry output; 2) fixed asset value ratio, measured by the ratio of total industry output to average annual fixed assets; 3) human capital, measured by the ratio of number of R&D staff to the average number of employees; 4) degree of openness, measured by the ratio of total exports to total industry output.
H1 and H2 imply that the IC of manufacturing industry can significantly increase TFP and plays this role through CE. To investigate this mechanism, the CE is first included in the regression equation, as shown in Equationequation 7(7)
(7) . Then, IC is introduced to construct a new regression equation, as shown in Equationequation 8
(8)
(8) . To examine its channel effect, the interaction term of IC and CE (lninnov*lncapital) is introduced to check whether IC affects TFP through CE, as shown in Equationequation 9
(9)
(9) . The centralization method is used to avoid multicollinearity issues possibly caused by introducing the interaction term (Dalal & Zickar, Citation2012).
3.2. Intermediary effect model
According to the theoretical analysis above, it can be found that CE may play an intermediary effect on the relationship between IC and TFP. Then, CE can be seen as an intermediary variable and the following model is constructed by adapting from the intermediary effect test method proposed by Baron and Kenny (Citation1986), Sobel (Citation1987), and Wen, Zhang, Hou, and Liu (Citation2004).
Where in model (6) is the total effect of IC on TFP,
in model (8) is the direct effect of IC on TFP,
is the intermediary effect and its relative value is the ratio of the intermediary effect to the total effect. To test the significance of the intermediary effect, “Sobel statistics” is used for calculation. The critical probability of Sobel statistics can be judged by the critical value table proposed by MacKinnon, Lockwood, Hoffman Jeanne, West Stephen, and Sheets (Citation2002).
Where is the standard error of
,
is the estimator of
,
is the estimator of
,
and
represents the standard error of
and
, respectively.
3.3. Panel threshold model
According to hypothesis 3 and the analysis above, the influence of CE on TFP is likely to be affected by the threshold effect of IC. It refers to the phenomenon that when one economic parameter reaches a certain value, another economic parameter suddenly shifts to other forms of development, i.e., structural mutation. Referring to Hansen (Citation1999) and Wang (Citation2015), this paper takes IC as a threshold variable and estimates the panel threshold model, which overcomes the bias of subjectively setting the structural mutation point. It can capture the structural mutation that may occur and evaluate the nonlinear relationship between CE and TFP accurately. The single threshold model is as follows.
Since there may be multiple threshold values for IC, the above model is extended to build the following multi-threshold panel model as shown in Equationequation 13(13)
(13) .
Where is the unknown threshold value, I (·) is the indicator index, its value is 1 if it satisfies the condition in parentheses, otherwise 0.
3.4. Data and variables
3.4.1. Sample and data
Due to the unbalanced regional economy and the existence of industry monopoly protection, such as the tobacco industry, the petroleum processing, coking, and nuclear fuel processing industry, there is a significant difference in the CE levels and innovation capabilities of different manufacturing segments. Therefore, this paper adopts the industry-level data for empirical analysis. Consistent with the 2012 industry classification standard of the China Securities Regulatory Commission (CSRC), the manufacturing is divided into 31 segments.
To ensure the consistency of the statistical caliber, the automobile manufacturing industry, and the railways, shipbuilding, aerospace, and other transportation equipment manufacturing industries are unified into the transportation equipment manufacturing industry. The rubber products industry and the plastic products industry are unified into the rubber and plastic products industry. Waste resource comprehensive utilization industry, metal products, and machinery and equipment repair industry are eliminated. Finally, 28 manufacturing industry segments are included. The sample period ranges from 2011 to 2018 and missing values are calculated (included) by linear interpolation.
All data are from the National Bureau of Statistics, the China Statistical Yearbook, the China Science and Technology Statistical Yearbook, and the China Industrial Economic Statistical Yearbook.
3.4.2. Innovation capability
The formation of IC is a result of the combination of multiple factors about which research literature is abundantly available (Croitoru, Citation2017; Jones, Citation2019; Martin, Citation1986). Adapted from Guan (Citation2003), Connie (Citation2014), Schoenherr and Swink (Citation2015), and Tang, Xiang, and Li (Citation2019), this study calculates IC with innovation resource input, innovation implementation capability, and innovation output by combining with characteristics of manufacturing industry, influencing factors and its management process. The integration of these complementary capabilities promotes the transformation of innovation into competitive advantages, and the evaluation index system is shown in .
Table 1. Evaluation index system of IC of manufacturing industry
First, innovation activities require a large and stable amount of capital and personnel resources (Brown James & Petersen, Citation2011; Zhang, Zhao, & Lyles, Citation2018). Traditional innovation research regards formal R&D as the main measure of innovation, ignoring diversified innovation activities that are based on non-R&D. The third European innovation survey (CIS-3) found that about half of the corporate innovations during 1998–2000 were based on non-R&D, and the Innobarometer survey for EU countries also reached similar conclusions (Arundel, Catalina, & Minna, Citation2008). This paper includes not only R&D but also other diversified non-R&D aspects of innovation to calculate IC.
The second part of IC measurement includes the capacity of innovation implementation capability. Referring to Nunes, Serrasqueiro, & Leitão (Citation2012), the R&D capacity and production & marketing capacity is selected. Kamboj & Rahman Citation2017 emphasize the importance of marketing capacity to IC, which is used to identify market gaps to be filled with new offerings by technology introduction, digestion, and absorption, etc., and achieve innovative commercial value.
The third part of IC measurement includes patent and non-patent indicators of innovation output. Some scholars believe that patents may represent strategic innovation behavior (Hall et al. Citation2012) which reflects the supportive government policies (Li & Zheng, Citation2016). Another study calls patents as a compromised proxy (Roper & Hewitt-Dundas, Citation2015). Given the imperfect market mechanism in China, industry development is susceptible to government intervention and national policies (De Vries, Bekkers, & Tummers, Citation2016). That is why we include measures of output innovation other than patents also to calculate IC, which reflects the ability of IC to transform into market-oriented products that bring profits to enterprises (Adams, Bessant, & Phelps, Citation2006).
Since the measurement units of different indexes are not the same, the entropy method is used to determine the normalized distribution function values.
First, assuming that there are m evaluation indicators, n number of manufacturing segments, and the original matrix is then
is obtained after normalization.
Second, the entropy value of the ith index is determined according to the following equation.
Where and
. When
is 0,
is 0. Then, the entropy weight of the
index is calculated according to the following equation.
The entropy weights of innovation resource input, innovation implementation capability, and innovation output are 0.191, 0.313, and 0.496, respectively. The IC is obtained by the following equation.
3.4.3. Capital enrichment
The annual average capital distribution of 28 manufacturing segments in 2011–2018 is shown in .
As evident from , when the total capital of the industry is selected as a benchmark, the capital in the chemical raw materials and products manufacturing industry, the communication equipment, computer, and other electronic equipment manufacturing, the transportation equipment industries are far above the industry median. On the other hand, if the capital owned by a single enterprise is taken as a benchmark, the capital in the tobacco industry, petroleum processing, coking, nuclear fuel processing industry, and the ferrous metal smelting and rolling processing industry is much higher than the industry median. Taken “the capital occupied by the industrial value-added of 100 million yuan” as a benchmark, the capital in the papermaking and paper products industry, the pharmaceutical manufacturing industry, and the rubber and plastic products industry is much higher than the median.
Further analysis reveals that the above-mentioned industries possess one or more of the following characteristics: high-profit margins, knowledge or technology-intensive, national policy support, and resource-based national monopoly industry. Take the tobacco industry as an example, it is a special industry with a state monopoly and its profit rate is higher. The profit-seeking nature of capital should result in continuous capital accumulation in this industry. However, from the perspective of the other two benchmarks, the total capital of the industry and the capital occupied by the industrial value-added of 100 million yuan are RMB 104,645.70 million and RMB 1.72 million, respectively. Both are below the industry median. It shows that the amount of capital and the number of enterprises vary greatly in different industries and the profit-seeking nature of capital may lead to an imbalance in the capital flows.
Therefore, the phenomenon of CE exists which is the result of capital inflows in an industry. Based on the Neoclassical Economic Theory and Factor Endowment Theory, capital flow is affected by factor input and marginal factor output. Assuming that a closed country economy consists of two industries and
, and it is a developed and fully competitive capital market. The output level of
is
, and the labor and capital endowments are
and
, respectively. The output level of
is
, and the labor and capital endowments are
and
, respectively. Meanwhile,
. Given the profit-seeking nature of capital, it always flows to industries with higher ROI. When other conditions are the same, the main determinant of the marginal capital-output lies in its abundance. Then, the marginal capital-output
in the industry
is lower than
in the industry
. As a result, capital flows to
from
, causing the convergence of prices of homogeneous production factors. Finally, the balanced capital allocation in
and
is formed to achieve the Pareto optimal. describes how CE has been operationalized.
Figure 2. The process of how CE has been operationalized.

The most intuitive manifestation is the increase of total capital amount, based on different sources of capital (state capital, collective capital, corporate capital, individuals, Hong Kong, Macao, and Taiwan capital and foreign capital). This paper defines the above total capital as the CE level.
3.4.4. TFP
This paper uses the C-D (Cob-Douglas) production function to measure TFP (Giannetti, Liao, & Yu, Citation2015; Karthik et al. Citation2015) and uses the DEA method to calculate it. The output index represents the total industrial output value of the
industry in period
. Due to a lack of data, we use “industrial sales output” for estimation purposes. The labor input index
represents the average number of employees and
is the capital input index estimated by the internationally accepted perpetual inventory method, which is only capital in the production process. The calculation equation is as follows:
Where is the capital depreciation rate in the
period of the
industry,
is the investment in fixed assets which is converted based on the unchanged price in 2011. The Kohli method is used to estimate the capital stock in the initial year as shown in Equationequation (20)
(20)
(20) . Moreover,
is the real growth rate of fixed asset investment at a fixed price. As there are significant differences in the capital during different years, the capital depreciation rate is also changing. This study calculates the capital depreciation rate of different industries to improve the accuracy and authenticity of the results.
4. Empirical analysis
4.1. Descriptive statistics
Before the empirical analysis, descriptive statistics are calculated on the main variables and the results are shown in .
Table 2. Descriptive statistical results of main variables
shows the scatter plot of IC and TFP, CE and TFP, and IC and CE, respectively. It is evident that all three variables have a positive correlation with each other which is consistent with the main idea of our paper. However, what is the transmission mechanism among IC, CE, and TFP? Whether there is any threshold effect? These questions still need to be answered.
4.2. Transmission mechanism analysis
The above scatter-plots show that the correlation between the main variables is consistent with the hypotheses proposed above. However, H1 and H2 state that the IC not only directly affects TFP but also affects TFP indirectly through CE which is influenced by the threshold effect of IC. Therefore, this paper further explores the transmission mechanism among IC, CE, and TFP and the threshold effect by using panel data of the Chinese manufacturing industry. Firstly, it analyzes the transmission mechanism and introduces the interaction term between IC and CE. Secondly, it tests the intermediary effect of CE. Thirdly, it tests the form of a panel threshold model to determine the threshold number of IC and adopts the fixed-effect model to estimate its threshold effect. The centralized treatment method is used to reduce possible collinearity problems.
The Model (1) in shows the direct influence of IC on TFP after controlling other variables that may affect it. The result shows that the IC and TFP are significantly positively correlated at 1%, indicating that the stronger the IC the higher will be the contribution to TFP. The Model (2) presents the impact of CE on TFP. There is a significant positive correlation between these two variables at 1%. It explains that the higher the level of CE the stronger the promotional effect on TFP will be which is consistent with previous research findings (Salinas-Jiménez et al., Citation2006). The CE has indeed brought an important impetus to economic growth.
Table 3. Basic regression results of variables
This study emphasizes on the transmission mechanism of IC affecting TFP through CE. If it is right, in the regression model where TFP is used as an explained variable, if the CE is removed, the effect of IC on TFP should remain significant. After introducing CE, as shown in the Model (3), the significance level of IC drops from 1% to 5%, verifying the mechanism that IC affects TFP through CE. By further introducing the interaction term of lninnov*lncapital, as shown in the Model (4), the regression coefficient of IC becomes significantly negative and the coefficient of the interaction term significantly positive. Actually, the model changes from linear to nonlinear after introducing the interaction term. So the relationship between CE and TFP cannot be directly judged based on the coefficient, because it no longer has a clear economic significance. At this time, the marginal effect should be considered. Since IC is significantly negative and the interaction term is significantly positive, it suggests that IC can regulate the marginal impact of CE on TFP. With the decline of IC, the promotional effect of CE on TFP becomes weak and insignificant.
For further analysis and verification of H2, this paper examines the intermediary effect of CE on the relationship between IC and TFP. In the Model (5), the coefficient of IC is positive and significant at 1%, demonstrating that the IC has greatly enhanced the level of CE. Additionally, the coefficients of IC and CE on TFP are both positive and significant at 5% showing that the CE indeed has an intermediary effect on the relationship between IC and TFP which is consistent with our H2. The Z value in the Sobel test is 2.5559 and significant at 1%, indicating that the intermediary effect of CE is significant. The proportion of the intermediary effect in the total effect is 49.49%, showing that CE plays an extremely important intermediary role in the influence of IC on TFP and it is manifested as the transmission mechanism of IC→CE→TFP. It once again proves the validity of H2.
4.3. Threshold model test
To explore whether the impact of CE on TFP is influenced by the threshold effect of IC, the panel threshold model test is used. Taking IC as the threshold variable, the residual is obtained by model estimation and then IC corresponding to the minimum sum of squared residuals is the threshold value. When IC exceeds this special value, the relationship between CE on TFP will be changed.
This paper utilizes single, double, and triple-threshold tests to determine the number of thresholds and the form of panel threshold model following recent literature (Wang, Citation2015). The results of the F statistic, P-values, and critical values are shown in . The P-value of the single-threshold under “self-sampling” is 0.0000, and the threshold value is 10.8720 which is significant at 1%. The P-value of the double-threshold under “self-sampling” is 0.0467, and the threshold values are 10.8827 and 10.9135, significant at 5%. The triple-threshold model does not pass the significance test, indicating that the IC has a double-threshold effect. On this basis, the Likelihood-Ratio (LR) method is used to describe the confidence interval graph as shown in .
Table 4. Threshold estimation results
4.4. Threshold model analysis
The above results show that the effect of CE on TFP is influenced by the double threshold effect of IC which partially supports H3. The panel threshold regression model is used for further analysis and the results as shown in the Model (6), . CE has a significant positive impact on TFP and is influenced by the double-threshold effect of IC. After performing the t-test on regression coefficients, the P-value is 0.0463, indicating that the effect of CE on TFP is significantly different when the IC is at different thresholds.
Table 5. Panel threshold regression results
When the IC is less than the single-threshold value of 10.8720, the coefficient of CE is 0.0731 which has a significant positive effect on improving TFP. When the IC is greater than or equal to 10.8720 but less than the double-threshold value of 10.8827, the coefficient of CE increases to 0.1152. When the IC is greater than or equal to 10.8827 but is less than the triple-threshold value of 10.9135, the coefficient of CE drops to 0.0740. As the value of IC passes the triple threshold value of 10.9135, the coefficient of CE increases again. All of the coefficients before and after the threshold values are significant at 1%. Therefore, the promotional effect of CE on TFP changes with the threshold effect of IC. They are not simply the linear relationship but are in the segmentation function relationship with the interval of IC. The trend can be divided into three stages: first enhanced then weakened and later re-enhanced again.
In the first stage, when IC is at a low level (IC < 10.8720), the capital allocation efficiency is low and the R&D input in high value-added or high-tech areas is insufficient. In this stage, the innovation behavior is simply technology imitation rather than self-innovation. So the TFP is at a low level and the promotional effect of capital on TFP is limited. With the improvement of industrial IC, after crossing a certain threshold, the return on investment improves which brings potential economic benefits. Together with the profit-seeking nature of capital, it affects the capital allocation efficiency and attracts more capital to form CE. The effect of CE on TFP changes qualitatively and positively. Therefore, compared with industries with low levels of IC, the promotional effect of CE on TFP in high-IC-industries is stronger.
In the second stage, the IC reaches the interval of (10.8827, 10.9135). The scale of CE continues to expand but with the diminishing marginal utility that hinders the promotion of TFP. In this stage, the effect of CE on TFP is weaker than in the previous stage. It also reveals that this phase is a screening process for the development of industries. Though the capital scale is increasing, it cannot be well used for blind expansion; the CE will result in inefficient capital allocation which is an important factor for TFP (Hsieh & Klenow Peter, Citation2009). Therefore, compared with industries with low IC, the positive effect of CE on TFP is gradually weakened in high IC industries.
In the third stage, when the IC is more than 10.9135, the industry may enter a relatively stable stage in its life cycle. By giving full play to the capital advantage, technological advantage, enterprise-scale advantage, or production capacity advantage, the capital will be invested in projects with the high-profit rates which will further enhance the positive effect of CE on TFP.
4.5. Robustness test
To check the robustness of the threshold model results, the following methods are used here.
4.5.1. Shifting mean values
Considering the fact that the data fluctuates greatly every year, this paper proceeds it three times and re-estimates the panel threshold model. The results are shown in the Model (7) of . After adding CE, the positive effect of IC on TFP becomes insignificant, which proving once again that IC affects TFP through CE. At the same time, there is a significant positive correlation between CE and TFP which is affected by the double-threshold effect of IC.
4.5.2. Adding more control variables
Earlier this paper uses R&D density, fixed asset value ratio, human capital and degree of openness as control variables, now it adds others including the total amount of export delivery, industry scale, and industry concentration into the model to check for the omitted variable bias (Hou Xiaohui Citation2011; Yu Donghua et al. Citation2019). The results are shown in the Model (8) of . The regression coefficient of IC is negative now but not significant. The transmission mechanism of IC still exists, and the acceleration effect of CE on TFP is significant at the 1% level before and after the double-threshold of IC. The effect is first enhanced, then weakened, and later re-enhanced again consistent with the above regression model.
4.5.3. Considering the endogeneity problems
The above research results show that IC not only directly promotes TFP but also indirectly through CE. Considering the possible endogeneity problems related to the regressions performed before, this paper adopts the instrumental variable method for re-estimation. The system GMM model is finally selected.
Referring to Wintoki, Linck, and Netter (Citation2012), the explanatory variable should be lagged by two periods to meet the exogenous requirements of instrumental variables. The results are shown in the . It can be found that the coefficients of IC on TFP and CE are both positive and significant at 1%, shown in the Model (9) and Model (10). The coefficient of IC on TFP drops to 0.0023 and significant at 5% after including CE in the same model. The Z value in the Sobel test is significant at 1%, and the proportion of the intermediary effect in the total effect is 84.15%, indicating that the intermediary effect of CE on the relationship between IC and TFP still exists after controlling the endogeneity problems.
Table 6. Regression results of the system GMM model
4.6. Further analysis
To systematically analyze IC and CE levels of 28 manufacturing segments, the average values of these variables during 2011–2018 are shown in .
From IC perspective, the industries with strong innovation abilities are mostly the technology-intensive industries including the communication equipment, computer, and other electronic equipment manufacturing industry (11.72), the electrical machinery and equipment manufacturing industry (11.20), the transportation equipment manufacturing industry (11.16), the general equipment manufacturing industry (10.69), the chemical raw materials and products manufacturing industry (10.63), the special equipment manufacturing industry (10.61), and the pharmaceutical manufacturing industry (10.18). The IC levels in the tobacco industry, the handcrafts, and other manufacturing industries, and the wood processing and wood, bamboo, rattan, and palm grass products industry are at the lowest, having values of 7.48, 7.86 and 7.99, respectively.
The levels of CE are higher in industries with strong innovation abilities including the chemical raw materials and products manufacturing industry (9.56), the communication equipment, computer, and other electronic equipment manufacturing (9.44), the transportation equipment manufacturing industry (9.37), and the electrical machinery and equipment manufacturing industry (9.26). Whereas the handcrafts and other manufacturing industry (6.19), the furniture manufacturing industry (6.95), the tobacco industry (6.95) exhibit low levels of CE. In industries with high levels of IC and CE, TFP is also at higher levels.
5. Conclusions
This study explores how IC and CE interact to affect TFP in Chinese manufacturing segments. We find that innovation capability exhibits a double-threshold effect on the relationship between CE and TFP.
First, IC not only promotes TFP directly but also plays a role through CE indirectly. So the CE has an intermediary effect on the relationship between IC and TFP, which accounts for 49.49% of the total effect.
Second, CE has a significant positive effect on TFP, which is affected by the double threshold effect of IC. The single-threshold value is 10.8720, and the double-threshold values are 10.8827 and 10.9135, respectively, significant at the 1% level. The promotion effect of CE on TFP in industries with high levels of IC is first enhanced, then weakened, and later re-enhanced again.
Third, IC and CE levels in industries of the communication equipment, computer, and other electronic equipment manufacturing industry, the electrical machinery and equipment manufacturing industry, the transportation equipment manufacturing industry, the general equipment manufacturing industry, and the chemical raw materials and products manufacturing industry are at a higher-end as compared to the furniture manufacturing industry, the tobacco industry, and the handcrafts and other manufacturing industry. These results are robust to the shifting mean values, adding more control variables, and lagging explained variables.
Acknowledgments
We would like to thank the National Social Science Foundation Youth Project (No.19CTQ030) for its support.
Disclosure statement
No potential conflict of interest was reported by the author(s).
Additional information
Funding
Notes on contributors
Lina Ma
Lina Ma is a lecturer at Wuhan Textile University and a Certified Public Accountant (CPA) in China. She has recently published in Journals like Journal of Intelligent and Fuzzy Systems, European Journal of Innovation Management, Chinese Political Science Review, and Science of the Total Environment. Her main research directions are Financial Markets, Corporate Governance, and Financial Engineering.
Fengju Xu
Fengju Xu is a professor at Wuhan University of Technology. She has published papers in journals like Journal of Business, Sustainability, and International Journal of Financial Studies. Her research directions are Financial Markets, Corporate Governance, and Internal Control.
Najaf Iqbal
Najaf Iqbal works in Anhui University of Finance and Economics. He has recently published in Journals like Science of the Total Environment, Environmental Research, and Journal of Intelligent and Fuzzy Systems. His research interests include “during and post COVID-19 socio-economy”, Corporate Finance and Climate Change.
References
- Adams, R., Bessant, J., & Phelps, R. (2006). Innovation management measurement: A review. International Journal of Management Reviews, 8(1), 21–47.
- Aghion, P., Akcigit, U., & Howitt, P. (2014). What do we learn from schumpeterian growth theory? Handbook of Economic Growth, 2, 515–563.
- Aghion, P., Dewatripont, M., Du, L., Harrison Ann, E., & Legros, P. (2015). Industrial policy and competition. American Economic Journal: Macroeconomics, 7(4), 1–32.
- Aghion, P., & Howitt, P. (2009). The economics of growth. Cambridge: MIT Press Books.
- Antonelli, C. (2003). The economics of innovation, new technologies and structural change. London: Routledge.
- Arundel, A., Catalina, B., & Minna, K. (2008), “Neglected innovators: How do innovative firms that do not perform R&D innovate? Results of an analysis of the innobarometer 2007 survey no. 215”, Brussels: European Commission, DG Enterprise, Inno-Metrics Thematic Paper.
- Bagherzadeh, A. (2012). The analysis of the effects of domestic and foreign investment in R&D on agricultural TFP in Iran. International Journal of Agricultural Management & Development, 2(2), 170–177.
- Banerjee, A. V., & Duflo, E. (2005). Growth theory through the lens of development economics. In Handbook of economic growth (Vol. 1 (Cambridge, MA 02142, USA) (), pp. 473–552). No. A.
- Baron, R. M., & Kenny, D. A. (1986). The moderator-mediator variable distinction in social psychological research: Conceptual, strategic, and statistical considerations. Journal of Personality and Social Psychology, 51, 1173–1182.
- Bartelsman, E., Haltiwanger, J., & Scarpetta, S. (2013). Cross-country differences in productivity: The role of allocation and selection. American Economic Review, 103(1), 305–334.
- Bernstein, S. (2015). Does going public affect innovation? Journal of Finance, 70(4), 1365–1403.
- Blackburn, K., & Cipriani, G. P. (2005). Intergenerational transfers and demographic transition. Journal of Development Economics, 78(1), 191–214.
- Bloom, N., & Van Reenen, J. (2007). Measuring and explaining management practices across firms and countries. The Quarterly Journal of Economics, 122(4), 1351–1408.
- Boedo, H., & Mukoyama, T. (2012). Evaluating the effects of entry regulations and firing costs on international income differences. Journal of Economic Growth, 17(2), 143–170.
- Booth, L., Ntantamis, C., & Zhou, J. (2015). Financial constraints, R&D investment, and the value of cash holdings. Quarterly Journal of Finance, 5(4), 1–24.
- Borgo, M. D., Goodridge, P., Haskel, J., & Pesole, A. (2013). Productivity and growth in UK industries: An intangible investment approach. Oxford Bulletin of Economics & Statistics, 75(6), 806–834.
- Brandt, L., & Thun, E. (2016). Constructing a ladder for growth: Policy, markets, and industrial upgrading in China. World Development, 80, 78–95.
- Brandt, L., Van Biesebroeck, J., & Zhang, Y. (2012). Creative accounting or creative destruction? Firm-level productivity growth in Chinese manufacturing. Journal of Development Economics, 97(2), 0–351.
- Brown James, R., & Petersen, B. C. (2011). Cash holdings and R&D smoothing. Journal of Corporate Finance, 17(3), 694–709.
- Chang, C. L., & Oxley, L. (2014). Industrial agglomeration, geographic innovation and total factor productivity: The case of Taiwan. Mathematics & Computers in Simulation, 79(9), 2787–2796.
- Chemmanur, T. J., Loutskina, E., & Tian, X. (2014). Corporate venture capital, value creation, and innovation. Review of Financial Studies, 27(8), 2434–2473.
- Collardwexler, A., & Loecker, J. D. (2013). Reallocation and technology: Evidence from the U.S. steel industry. American Economic Review, 105(1), 131–171.
- Comin, D., & Hobijn, B. (2010). An exploration of technology diffusion. American Economic Review, 100(5), 2031–2059.
- Cornaggia, J., Mao, Y., Tian, X., & Wolfe, B. (2015). Does banking competition affect innovation? Journal of Financial Economics, 115(1), 189–209.
- Crespi, G., & Zuniga, P. (2012). Innovation and productivity: Evidence from six Latin American countries. World Development, 40(2), 273–290.
- Croitoru, A. (2017). Schumpeter, Joseph Alois, 1939, ‘business cycles: A theoretical, historical, and statistical analysis of the capitalist process’, New York and London, mcgraw-hill book company inc. Journal of Comparative Research in Anthropology and Sociology, 8(1), 67–80.
- Dalal, D. K., & Zickar, M. J. (2012). Some common myths about centering predictor variables in moderated multiple regression and polynomial regression. Organizational Research Methods, 15(47), 339–362.
- De Vries, H., Bekkers, V., & Tummers, L. (2016). Innovation in the public sector: A systematic review and future research agenda. Public Administration, 94(1), 146–166.
- Fagerberg, J., & Srholec, M. (2008). National innovation systems, capabilities and economic development. Research Policy, 37(9), 1417–1435.
- Feder, C. (2018). The effects of disruptive innovations on productivity. Technological Forecasting & Social Change, 126, 186–193.
- Gancia, G., & Zilibotti, F. (2005). Horizontal innovation in the theory of growth and development Aghion, P., and Durlauf, S. N. In Handbook of economic growth (Vol. 1, pp. 111–170). No. A.
- Giannetti, M., Liao, G., & Yu, X. (2015). The brain gain of corporate boards: Evidence from China. Journal of Finance, 70(4), 1629–1682.
- Goodridge, P., Haskel, J., & Wallis, G. (2018). Accounting for the UK productivity puzzle: A decomposition and predictions. Economica, 85(339), 581–605.
- Griffith, R., Huergo, E., Mairesse, J., & Peters, B. (2006). Innovation and productivity across four European countries. Oxford Review of Economic Policy, 22(4), 483–498.
- Guan, J., & Ma, N. (2003). Innovative capability and export performance of Chinese firms. Technovation, 23(6), 124–128.
- Hall, B. H., & Harhoff, D. (2012). Recent research on the economics of patents. Annual Review of Economics, 4(1), 541–565.
- Hansen, B. E. (1999). Threshold effects in non-dynamic panels: Estimation, testing, and inference. Journal of Econometrics, 93(2), 345–368.
- Hausmann, R., & Rodrik, D. (2003). Economic development as self-discovery. Journal of Development Economics, 72(2), 603–633.
- Heshmati, A., & Kumbhakar Subal, C. (2010). Technical change and total factor productivity growth: The case of Chinese provinces. Technological Forecasting & Social Change, 78(4), 575–590.
- Hou, X., WanLi, L., & Wang, Q. (2011). Ownership, market power and TFP of Chinese commercial banks: The generalized Malmquist index analysis based on the SFA panel model. The Journal of World Economy, 34(No. 2), 135–157.
- Howitt, P. (1998). Endogenous growth theory. Boston: MIT Press.
- Hsieh, C.-T., & Klenow Peter, J. (2009). Misallocation and manufacturing TFP in China and India. The Quarterly Journal of Economics, 124(4), 1403–1448.
- Imbriani, C., Pittiglio, R., & Reganati, F. (2010), “Outward FDI and home-country performance: Evidence from Italian manufacturing and service firms”, The 6th International Scientific Conference, Vilnius, Lithuania.
- Jeffrey, W. (2001). Financial markets and the allocation of capital. Journal of Financial Economics, 58(1), 187–214.
- Jones, C. I. (2019). Paul Romer: Ideas, nonrivalry, and endogenous growth. The Scandinavian Journal of Economics, 121(3), 859–883.
- Kale, S., & Rath, B. (2018). Does innovation matter for total factor productivity growth in India? Evidence from ARDL bound testing approach. International Journal of Emerging Markets, 13(5), 1311–1329.
- Kamboj S and Rahman Z. (2017). Market orientation, marketing capabilities and sustainable innovation. MRR, 40(6), 698–724. 10.1108/MRR-09-2014-0225
- Keynes, J. M. (1936). The general theory of employment interest and money. London: Macmillan & Co Ltd.
- Kirchner, S. (2016). Linking institutions and firm-level outcomes: The roles of diverse innovative capability profiles in Germany’s economy. Innovation: The European Journal of Social Science Research, 29(4), 462–480.
- Kong, X., Mi, M., & Gao, T. (2015). The suitable degree of technology progress and innovation driven industrial structure adjustment: An empirical analysis based on biased technology progress. China Industrial Economy, 11, 62–77.
- Kose, M. A., Prasad, E. S., & Terrones, M. E. (2009). Does openness to international financial flows raise productivity growth? Journal of International Money and Finance, 28(4), 554–580.
- Krishnan, K., Nandy, D. K., & Puri, M. (2015). Does financing spur small business productivity? Evidence from a natural experiment. Review of Financial Studies, 28(No. 6), 1768–1809.
- Laursen, K., Masciarelli, F., & Prencipe, A. (2012). Regions matter: How localized social capital affects innovation and external knowledge acquisition. Organization Science, 23(1), 177–193.
- Li, H., Loyalka, P., Rozelle, S., & Wu, B. Z. (2017). Human capital and China’s future growth. Journal of Economic Perspectives, 31(1), 25–48.
- Li, W., & Zheng, M. (2016). Is it substantive innovation or strategic innovation? Impact of macroeconomic policies on micro-enterprises’ innovation. Economic Research Journal, 4, 60–73.
- Lucas, R. E. (1988). On the mechanics of economic development. Journal of Monetary Economics, 22(1), 3–42.
- MacKinnon, D. P., & Fairchild, A. J. (2009). Current directions in mediation analysis. Current Directions in Psychological Science, 18(1), 16–20.
- MacKinnon, D. P., Lockwood, C. M., Hoffman Jeanne, M., West Stephen, G., & Sheets, V. (2002). A comparison of methods to test mediation and other intervening variable effects. Psychological Methods, 1, 83–104.
- Madsen, J. B., Ang, J. B., & Banerjee, R. (2010). Four centuries of British economic growth: The roles of technology and population. Journal of Economic Growth, 15(4), 263–290.
- Martin, A. (1986). Joseph Alois Schumpeter: A bibliography, 1905-1984 compiled by Michael I.Stevenson. Business History Review, 60(1), 150–151.
- Medda, G., & Piga, C. A. (2014). Technological spillovers and productivity in Italian manufacturing firms. Journal of Productivity Analysis, 41(3), 419–434.
- Miyagiwa, K., Huasheng, S., & Vandenbussche, H. (2010). Innovation, anti-dumping, and retaliation. CEPR Discussion Papers, 1, 1–41.
- Naomi, F. (2016), “Heterogeneity in Japanese TFP, part II: Regulation, capital allocation, and TFP in Japan”, Columbia University Unpublished Working Paper.
- Niosi, J., & Richard, R. (2008). Nelson: Technology, institutions and economic growth. Journal of Evolutionary Economics, 18(5), 647–651.
- Ozturk, A. (2015). Examining the economic growth and the middle-income trap from the perspective of the middle class. International Business Review, 25(3), 726–738.
- Qian, X., Kang, J., Tang, Y., & Cao, X. (2018). Industrial policy, efficiency of capital allocation and firm’s total factor productivity: Evidence from a natural experiment in China. China Industrial Economy, 365(8), 44–61.
- Raymond, W., Mairesse, J., Mohnen, P., & Palm, F. (2015). Dynamic models of R&D, innovation and productivity: Panel data evidence for Dutch and French manufacturing. European Economic Review, 78, 285–306.
- Restuccia, D., & Rogerson, R. (2008). Policy distortions and aggregate productivity with heterogeneous establishments. Review of Economic Dynamics, 11(4), 707–720.
- Romer, P. M. (1986). Increasing returns and long-run growth. Journal of Political Economy, 94(5), 1002–1037.
- Roper, S., Du, J., & Love, J. H. (2008). Modelling the innovation value chain. Research Policy, 37(6–7), 961–977.
- Roper, S., & Hewitt-Dundas, N. (2015). Knowledge stocks, knowledge flows and innovation: Evidence from matched patents and innovation panel data. Research Policy, 44(7), 1327–1340.
- Ruttan, V. W., & Hayami, Y. (1984). Toward a theory of induced institutional innovation. Journal of Development Studies, 20(4), 203–223.
- Salinas-Jiménez, M. M., Alvarez-Ayuso, I., & Delgado-Rodríguez Ma, J. (2006). Capital accumulation and TFP growth in the EU: A production frontier approach. Journal of Policy Modeling, 28(2), 195–205.
- Schoenherr, T., & Swink, M. (2015). The role of supply chain intelligence and adaptability in new product launch success. Decision Sciences, 46(5), 901–936.
- Schumpeter Joseph, A. (1912). The theory economic development. Cambridge: Harford University Press.
- Seybert, N. (2010). R&D capitalization and reputation driven real earnings management. Accounting Review, 85(2), 671–693.
- Sobel, M. E. (1987). Direct and indirect effects in linear structural equation models. Sociological Methods & Research, 16(1), 155–176.
- Solow, R. M. (1956). A contribution to the theory of economic growth. The Quarterly Journal of Economics, 70(1), 65–94.
- Stollinger, R. (2013). International spillovers in a world of technology clubs. Structural Change & Economic Dynamics, 56(8), 19–35.
- Su, H., Zheng, L., & Mou, Y. (2017). Factor endowment and manufacturing industry grading in China: Analysis based on WIOD and Chinese industrial enterprise database. Management World, 4, 70–79.
- Sulistyo, H., & Siyamtinah. (2016). Innovation capability of SMEs through entrepreneurship, marketing capability, relational capital and empowerment. Asia Pacific Management Review, 21(4), 196–203.
- Tang, X., Xiang, N., & Li, Y. (2019). Influencing factors of the innovation capability of Beijing modern manufacturing industry under the low carbon economy. Science Research Management, 40(7), 87–96.
- Tientao, A., Legros, D., & Pichery, M. C. (2016). Technology spillover and TFP growth: A spatial Durbin model. Journal of International Economics, 145(5), 21–31.
- Torres, I. T., Gutierrez, L., & Dominguez, M. C. H. (2010). Innovation and operative real options as ways to affect organisational learning. International Journal of Technology Management, 49(4), 421–438.
- Vithessonthi, C., & Racela, O. C. (2016). Short and long run effects of internationalization and R&D intensity on firm performance. Journal of Multinational Financial Management, 78(34), 28–45.
- Vollrath, D. (2009). How important are dual economy effects for aggregate productivity? Journal of Development Economics, 88(2), 325–334.
- Wang, Q. (2015). Fixed-effect panel threshold model using stata. Stata Journal, 15(65), 121–134.
- Wen, Z. L., Zhang, L., Hou, J. Q., & Liu, H. Y. (2004). Testing and application of the mediating effects. Acta Psychologica Sinica, 36(5), 614–620.
- Wintoki, M. B., Linck, J. S., & Netter, J. M. (2012). Endogeneity and the dynamics of internal corporate governance. Journal of Financial Economics, 105(3), 298–310.
- Young, A. (2003). Gold into base metals: Productivity growth in the people’s republic of China during the reform period. Journal of Political Economy, 111(6), 1220–1261.
- Yu, D., Zhang, X., & Sun, T. (2019). Capital deepening, biased technological progress and total factor productivity growth. The Journal of World Economy, 42(8), 50–71.
- Zhang, M., Zhao, X., & Lyles, M. (2018). Effects of absorptive capability, trust and information systems on product innovation. International Journal of Operation and Production Management, 38(2), 493–512.
- Zhao, R. (2019). Technology and economic growth: From Robert Solow to Paul Romer. Human Behavior and Emerging Technologies, 1(No. 1), 116–119.
- Zheng, C. (2014). The inner circle of technology innovation: A case study of two Chinese firms. Technological Forecasting & Social Change, 82, 140–148.
- Zhu, X. (2012). Understanding China’s growth: Past, present and future. Journal of Economic Perspectives, 26(4), 103–124.