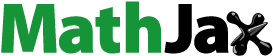
ABSTRACT
Persistent increases in basic food prices have become a critical challenge in Ethiopia since 2006. This paper assesses whether the structure of the grain market has contributed to the price increases. Traders having market power could create commodity price stickiness, implying that what goes up does not come down, leading to price increases. The study examines price linkages between principal grain markets in Ethiopia, using monthly prices from the wheat, maize, and teff markets. The Engle-Granger cointegration test is used to check for cointegration, while the Threshold Autoregressive Model is employed to investigate potential asymmetric price transmission. The findings indicate that major grain markets in Ethiopia are well integrated. Moreover, the threshold cointegration model reveals that they are characterized by symmetric adjustment. Hence, I argue that there is insufficient evidence to support the claim that market structure contributes to the price increase in Ethiopian grain markets.
1. Introduction
In Ethiopia, persistent increases in basic food grain prices have become a critical challenge since 2006. For instance, as illustrated in below, the 2020 national average wholesale prices of both wheat and maize have increased by about four times compared to their 2010 levels. Moreover, the rate of growth of food price inflation in Ethiopia has been among the highest in sub-Saharan Africa (SSA). For instance, according to estimates from the World Bank, the average annual consumer price inflation in Ethiopia grew from 8% to 16% between 2010 and 2020, while generally, it declined from 4.0% to 3.3% in the SSA region over the same period. Understanding what explains the persistent increase in food prices in Ethiopia might contribute to designing targeted government interventions that stabilize the market.
Figure 1. National nominal average monthly commodity price trends in Ethiopia (Jan 2010 – Oct 2020).

According to the literature, food prices in any market may change as a result of various factors including, price-shock diffusion from international markets, changes in the exchange rate and the domestic demand and supply conditions, and market structure. If a country is a net importer of food commodities, high world-price-shock transmission to the domestic market may affect commodity prices (Baquedano & Liefert, Citation2014; Ceballos, Hernandez, Minot, & Robles, Citation2017; Conforti, Citation2004; Minot, Citation2011; Mundlak & Larson, Citation1992). However, the extent and speed of price shock diffusion from international to domestic market depends on several factors, including the exchange rate, border policies (such as import tariffs), and transfer costs (Baquedano & Liefert, Citation2014; Dawe, Citation2008; Ozturk, Citation2020; Rapsomanikis, Hallam, & Conforti, Citation2006). For instance, when a local currency appreciates (depreciates) against the US dollar, an increase in the commodity price in the local currency would be less (more) than an increase in the international price in dollars (Ozturk, Citation2020).
Domestic demand and supply dynamics are also important. In a country with a self-sufficient position, domestic demand, and supply factors (such as income growth, population growth, weather shocks, and production input costs) determine commodity price formation and stability (Brækkan, Thyholdt, Asche, & Myrland, Citation2018). The other important factor in commodity price formation, and what motivated this study, is market structure (Abdulia, Citation2000). In a non-competitive market structure, middlemen with high market power may dominate the pricing of the commodities along the supply chain or across spatially distinct markets. In such circumstances, price increases in some markets may completely and quickly transmit to other markets, whereas price reduction might remain sticky, implying, what goes up does not come down, hence leading to price increases.
In Ethiopia, a profound agricultural market liberalization process started in the early 1990s, when all the restrictions on official prices, quotas, and private trade were removed (Gabre-Madhin & Goggin, Citation2005; Negassa, Myers, & Gabre-Madhin, Citation2004). The reform has massively changed the structure of the grain market in the country (Shahidur & Asfaw, Citation2011). After the reform, private sector participation in grain marketing activities has increased, and this contributed to an increase in market integration and grain production (Kindie, Citation2008; Negassa & Myers, Citation2007). On the other side, however, the reform created a grain market structure where market power is concentrated around a few dominant firms because of a shortage of initial capital, which is the major obstacle to entering the market (Gebremeskel, Jayne, & Shaffer, Citation1998; Sassi & Mamo, Citation2019). This may create a substantial impact on the price transmission process, implying price shocks in some markets affect price behavior in others (e.g., von Cramon-taubadel & Meyer, Citation2004). In fact, there is a growing perception in the Ethiopian grain market that once prices have started to increase, they never come down, even during the harvest seasons when producer prices are falling (Sassi & Mamo, Citation2019; Tadesse & Guttormsen, Citation2011).
Often economists who assess overall market performance investigate price transmission mechanisms in the market. In perfectly integrated markets, price changes in one market completely and quickly transmit to another market (Fackler & Goodwin, Citation2001). Moreover, in well-integrated markets, price shocks in one market would elicit the same response in other markets, regardless of whether the shock reflected a decrease or an increase in prices (e.g., von Cramon-taubadel & Meyer, Citation2004). Such a price adjustment is called symmetric price transmission. However, as documented in the literature on price transmission, certain characteristics associated with imperfect competition (e.g., market concentration), government intervention, transaction cost, and inventory behavior of traders can contribute to asymmetric price responses (Abdulia, Citation2000). Moreover, the presence of transaction costs may prevent economic agents from continually adjusting. Only when the deviation from the equilibrium surpasses a critical threshold do the benefits of adjustment exceed the costs, and economic agents act to move the system back to equilibrium (Abdulia, Citation2000). The above implies that while investigating price transmission, it is relevant to consider price asymmetry and/or nonlinearity.
Previous price transmission studies in the Ethiopian grain market include explorations of price transmission along the vertical chain (Sassi & Mamo, Citation2019; Usman & Haile, Citation2017) and across spatially distinct markets (Getnet, Citation2007; Getnet, Verbeke, & Viaene, Citation2005; Kifle, Citation2015; Negassa & Myers, Citation2007; Yami, Citation2020). While these available studies might be sufficient to illustrate the presence or lack of effective price transmission in the Ethiopian grain market, they generally have two shortcomings.
First, the data used by most of these studies are old. Thus, a new study using the most up-to-date data may help us understand the causes of the price increases observed in the Ethiopian grain market in recent years. Second, most of the studies mentioned do not consider price asymmetry and/or nonlinearity in their analysis. Results obtained without considering price asymmetry and/or nonlinearity might be biased when an asymmetric response is misspecified as symmetric (e.g., Barrett & Li, Citation2002). Moreover, while three studies (i.e., Kifle, Citation2015; Sassi & Mamo, Citation2019; Usman & Haile, Citation2017) investigate price asymmetries, results from these are inconclusive due to the diversity of the good analyzed, methodology, and time periods considered.
The purpose of this article is thus to investigate the possible existence of asymmetric price transmission between major regional grain markets in Ethiopia. Asymmetric price transmission is investigated in the three important grain markets: wheat, maize, and teff. The main method used is the threshold cointegration model with both zero and non-zero thresholds. The threshold cointegration model is used because it is suitable for investigating the presence of any asymmetric price transmission between distinct markets across space.
The remainder of this paper is organized as follows. Section 2 is the literature review. Section 3 covers my methodology and the data used in the study is presented in Section 4. The empirical results are contained in section 5 and the concluding remarks in Section 6.
2. Literature review
This section presents theoretical concepts of market integration and spatial price transmission, followed by a general overview of grain markets in Ethiopia, and finally, a review of previous empirical evidence concerning these markets.
2.1. Market integration, spatial price transmission, and asymmetric price transmission
Economic theory postulates that the proper functioning of markets and marketing channels is vital for the optimal allocation of resources (Abdulia, Citation2000). Price transmission has become a common tool used to assess the proper functioning (or integration) of spatially separated markets. According to the well-known Law of One Price (LOP), under free trade, price differences of a homogeneous good in two distinct markets separated in space will be, at most, equal to the transaction costs involved in transferring the goods from one market to the another (Fackler & Goodwin, Citation2001; Serra & Gil, Citation2006). If the price spreads between the markets exceed the transaction costs, the activity of profit-seeking arbitrageurs will reduce the spread, allowing prices to move toward the LOP condition.
However, certain characteristics of agricultural production, marketing, and consumption, such as inadequate infrastructure, market-entry barriers, and unreliable market and price information, may render arbitrage a risky activity for traders (Abdulia, Citation2000). In such a circumstance, spatial markets may be partially integrated or completely segmented. When spatially separated markets are not well integrated, profitability opportunities will not be fully exploited by spatial arbitrageurs, thus resulting in efficiency losses (e.g., Fackler & Goodwin, Citation2001). Inefficient price signal transmission between markets can distort producer decisions and lead to inefficient product movement, and ultimately increase consumer prices (Goodwin & Schroeder, Citation1991). Hence, assessing whether spatial price transmission is efficient is highly relevant and has policy implications.
Spatial price transmission is characterized by the speed, extent, and nature of price signal transmission between markets. In the case of perfectly integrated markets, price changes in one market are completely and immediately transmitted to other markets. However, when markets are not well integrated, price transmission will be incomplete, and prices will take time to adjust. This might reflect inefficiency and welfare losses in the economic system.
Another concern that has driven the interest of price analysts when dealing with markets’ responses to one another is whether they adjust symmetrically or asymmetrically. If a shock to a market (for instance, to the central market) would elicit the same magnitude of response in local markets, regardless of whether the shock reflected an increase or decrease in prices, the transmission is called symmetric; otherwise, it is called asymmetric (Serra & Gil, Citation2006; von Cramon-taubadel & Meyer, Citation2004). von Cramon-taubadel and Meyer (Citation2004) indicate that symmetric adjustments are often assumed to be representative of competitive markets, whereas asymmetric price responses are linked with the existence of certain market imperfections (e.g., market power) that cause rational market participants to deviate from their preferred risk level. Moreover, the presence of asymmetry in price transmission implies potential welfare loss for some groups of market participants; welfare distribution could be different under asymmetry (von Cramon-taubadel & Meyer, Citation2004).
As documented in the literature on price transmission, several factors can contribute to asymmetric price responses. Some of the most common causes include imperfect competition; adjustment, search, and menu costs; government intervention; and inefficient inventory management (Frey & Manera, Citation2007; von Cramon-taubadel & Meyer, Citation2004). Non-competitive behavior (or market power) is the main cause of asymmetric price transmission identified in the literature (Sexton, Kling, & Carman, Citation1991; von Cramon-taubadel & Meyer, Citation2004). Oligopolistic intermediaries in spatial trade may act more quickly to shocks that squeeze their profit margin than to those that stretch their margin. Such marketing behavior can lead to asymmetric price transmission in the short run (Abdulia, Citation2000). In this case, increases in central market prices may more quickly and completely transmit to the local markets than will a corresponding decrease in prices. Asymmetric price transmission in the spatial market may also occur if local-market traders assume that competitors in the local market will follow a price rise but not a price decrease in the central market (Abdulia, Citation2000).
Adjustment costs are the second important cause of asymmetric price transmission (Abdulia, Citation2000). Adjustment (or transaction) costs are those incurred due to changing market conditions; these arise when firms change the quantities and/or outputs. If the cost of adjustment is different with respect to cost increases and decreases, asymmetric price transmission can occur (Abdulia, Citation2000). Search costs are another cause of asymmetric price transmission (von Cramon-taubadel & Meyer, Citation2004). These are costs incurred by consumers when searching for market information about a product they are interested in buying; customers may have incomplete information about a particular market since obtaining information is costly. Thus, a firm in a local market may possess local market power over their customers, who may have insufficient information on what other firms in other local markets charge for the same product.
Inventory management can also lead to asymmetric price transmission. For storable commodities such as grains, farmers (particularly in developing countries) may lack the storage and capital needed to get their goods to distant markets. They are thus left selling locally to intermediaries who now have more suppliers from which to choose (Abdulia, Citation2000). Moreover, if firms think the prices in the central market will increase, they will hold their products, and if they think the prices in the central markets will decrease, they sell their products (Abdulia, Citation2000). Finally, government policy intervention can also lead to asymmetric price transmission (Kinnucan & Forker, Citation1987; von Cramon-taubadel & Meyer, Citation2004). If the government intervenes in the market, for instance, by establishing price floors, firms may assume that the increase is permanent and respond asymmetrically.
2.2. An overview of grain market in Ethiopia and previous empirical evidence
Grain is a staple food crop in Ethiopia, accounting for more than 40% of a typical household’s food expenditure, and more than 60% of total caloric intake by households (Rashid, Citation2011). Grain production in Ethiopia is highly specialized due to the heterogeneous nature of the country’s agro-ecologies. Wheat, maize, and teff are the most-produced grains within the country.
In terms of production regions, the main wheat production zones (provinces) are Arsi and Bale (Negassa & Myers, Citation2007), located southeast of the capital city of Ethiopia (Addis Ababa). Figure A1 in the appendix shows wheat production and market flow maps. The major maize-producing zones are west Gojjam (in the north), Jimma (in the west), East Shewa (in the center), and East Wellega (in the west). Teff production is mainly concentrated in the center and the northwest of the country. In terms of consumption, wheat is preferred in three regions, Afar (in the northeast), Tigray, and Amhara (in the north), whereas teff is the preferred commodity in Addis Ababa, Amhara, and Tigray. Maize is preferred in the center, eastern, and southern parts of the country.
Differences in grain production and consumption regions in Ethiopia make spatial domestic-grain trade highly relevant. In general, grain trade in Ethiopia has a radial structure, where grain trades typically flow from the surplus areas to Addis Ababa either for consumption or transshipment to deficit areas (Negassa & Myers, Citation2007).Footnote1 While minor retailers and small wholesalers dominate the local and regional markets, larger firms engage in spatial arbitrage. However, these firms are relatively few due to a shortage of capital, creating a barrier to entry (Gebremeskel et al., Citation1998; Osborne, Citation2004). This, coupled with the poor market infrastructure in the country, makes it highly likely that the country’s spatial grain markets are inefficient.
In the empirical literature on price transmission in the Ethiopian grain market mentioned earlier, there are only three studies that consider asymmetric price transmission. Kifle (Citation2015) investigates asymmetry in the prices of white teff, red teff, and maize between the central market (Addis Ababa) and two local deficit markets (Mekelle and Dire Dawa). Usman and Haile (Citation2017) find that symmetric adjustment generally characterizes the price transmission along the supply chains of teff, wheat, and maize in Amhara and Oromia, the two major cereal markets in Ethiopia. Sassi and Mamo (Citation2019) identify asymmetric price transmission in the white teff market between producers and the wholesale market in Oromia and Tigray regions but identify symmetric price adjustment in the Amhara region and the Southern Nations, Nationalities, and People’s region. The remaining two studies, in addition to Kifle (Citation2015), focus on price transmission asymmetries along grain supply chains. In this study, I focus on spatial asymmetric price transmission in three important grain markets (wheat, maize, and teff) using more recent data and the threshold cointegration model proposed by Enders and Siklos (Citation2001) and discussed below.
3. Methodology
The empirical procedure followed in this study comprises a series of tests and model estimations. First, stationarity of prices is confirmed using the Augmented Dickey-Fuller (ADF) (Dickey & Fuller, Citation1981) and Perron (Citation1997) tests on individual price series. The Engle-Granger cointegration test is then employed to check the presence of linear long-run relationships between the central and local grain-market prices. Then, the threshold cointegration model is estimated to investigate the presence of possible asymmetric price adjustment between central- and local-market prices. For price pairs demonstrating asymmetric adjustment, the threshold asymmetric error correction model is estimated; otherwise, the traditional error correction model is estimated.
In a system of spatially related markets, as discussed in Asche, Gjølberg, and Guttormsen (Citation2012), there is sometimes a leading market that has a dominant influence on the other markets. The price changes in the leading market can affect the prices and quantities in the other markets, but the opposite is not the case. Based on the characteristics of the Ethiopian grain markets discussed above, the market in the capital Addis Ababa can be taken as the central market. Given the central wholesale price () and local market price (
) (expressed in log form), the basic price transmission model can be expressed as follows:
where is the error term, which is assumed to be independent and identically distributed with mean zero. Parameters
and
define the relationship between the prices or whether the markets are integrated. Specifically, the parameter
is interpreted as the price transmission elasticity. If
, the LOP holds (i.e., there is complete price transmission), while
implies there is no relationship between the prices.Footnote2 If
, there is a relationship between the prices, but their transmission is not complete.
Using Equation (1) for price transmission analysis raises two major conceptual and practical concerns. First, Equation (1) is a static model. However, price adjustment is a dynamic process, and hence temporary deviations from the long-run equilibrium are inevitable. Second, agricultural product prices appear to be non-stationary. The application of Equation (1) to a non-stationary price series may generate spurious results; estimated models will indicate a relationship between the prices when in fact, no theoretical relationship exists. A cointegration test provides a means to distinguish a true relationship from one that is spurious.Footnote3
There are two commonly used approaches to investigating a cointegrating relationship between prices: the Engle-Granger approach (Engle & Granger, Citation1987) and the Johansen cointegration approach (Johansen & Juselius, Citation1990). In this study, we use the Engle-Granger cointegration approach, of which the threshold cointegration model is an extension. The Engle-Granger approach involves extraction of the estimated residuals from the OLS regression in Equation (1) to estimate the parameter
in Equation (2):
where is a white-noise disturbance, and
are parameters included in the model to account for serial correlation. According to the Engle-Granger approach, rejection of the null hypothesis of no cointegration (
implies stationarity of the estimated residuals (i.e.,
, and hence cointegration of the prices.
The Engle-Granger model given in Equation (2) relies on an assumption of symmetric (or linear) price adjustment since is estimated as an average effect of the lagged error term
regardless of whether
is positive or negative. Taking asymmetry into consideration, Enders and Siklos (Citation2001) extend the Engle-Granger procedure to a Threshold Autoregressive (TAR) model given by Equation (3):
where is an indicator function that can be defined by either Equation (4) or Equation (5) as:
where is a threshold value, and
and
are the parameters to be estimated. If the indicator function is defined by Equation (4), the model in Equation (3) is said to be a TAR model, whereas if the indicator function is defined by Equation (5), the model in Equation (3) is known as a Momentum-Threshold Autoregressive (MTAR) model. In the literature, if
, the TAR model is said to be consistent TAR, whereas the MTAR model is called consistent MTAR.
In Equation (3), the parameter captures the local market price adjustment when the local market price is “too high” with respect to the central market price (i.e., when the price differential is above its long-run equilibrium value), while the parameter
is the adjustment when the local market price is “too low” with respect to the central market price (i.e., when the price differential is below its long-run equilibrium value).
As indicated by Equations (2) and (3), the Engle-Granger procedure is a special case of the Enders and Siklos’s (Citation2001) model when . From Equation (3), cointegration exists if the null hypothesis
is rejected. This leads to the test of symmetry
. If both hypotheses are rejected, the process is called threshold cointegration. According to Enders and Siklos (Citation2001), the cointegration test follows a non-standard F-test; hence, results are compared to the critical values given in Enders and Siklos (Citation2001). The symmetry test, however, is a standard F-test. Following the procedure proposed by Chan (Citation1993), the best threshold value is used. The estimated residuals (in the case of the TAR model) or the first differences of the estimated residuals (in the case of the MTAR model) are first sorted in ascending order, then 15% of the largest and smallest values are excluded before selecting the best threshold value, that is, the value that yields the lowest residual sum of squares.
The Granger representation theorem (Engle & Granger, Citation1987) states that an error correction model can be estimated when variables are cointegrated. The standard vector error correction model (VECM) can be written as:
where is the error correction term and the coefficients
and
capture the speed of error correction. Parameters
and
are intercepts,
capture short-run price dynamics, and
are added to capture serial correlation. The parameters
and
are optimal lag lengths to be chosen using the Schwarz information criteria (SC).
However, a conventional error correction model as given by Equations (6) and (7) cannot be used to consider the issue of asymmetric transmission since the error term has not been decomposed into positive and negative components. In this study, by following Balke and Fomby (Citation1997) and Enders and Granger (Citation1998), the conventional VECM is extended to a threshold VECM, which is then capable of analyzing the asymmetric price transmission by decomposing the error correction terms into positive and negative components, as presented by Equations (8) and (9).
where the error correction terms and
are defined as follows:
where is either the TAR or MTAR indicator function with the consistent threshold.
4. Data
The price series used in this study were obtained from the Food and Agriculture Organization Global Information and Early Warning System database.Footnote4 Based on data availability, monthly data covering the period from January 2010 to October 2020 were used for the wheat and maize markets, whereas monthly data that ranges from January 2010 to July 2018 were used for the teff market.Footnote5 Prices are measured in local currency per 100 kilograms. All the prices are deflated using the Ethiopian consumer price index (obtained from the International Monetary Fund database) to account for inflation.
Regional markets with the most populous towns are selected for this study, and the extent and nature of price transmission between the central market (i.e., Addis Ababa) and local markets are then considered. Based on data availability, five market pairs are considered for the wheat market. Three of these pairs represent trade flows to Addis Ababa from the wheat surplus regions surrounding Robe and Shashemene (located south of Addis Ababa) and Debre Markos (to the northwest). Two pairs represent trade flows from Addis Ababa to deficit areas surrounding Dire Dawa (in the east) and Jimma (in the west). Four market pairs are investigated for the teff market. The first pair represents trade flows from the teff surplus region surrounding Bahirdar (in the northwest) to Addis Ababa. The other three pairs represent trade flows from Addis Ababa to the teff deficit areas surrounding Mekelle (in the north) and Shashemene and Jimma. In the maize market, three price pairs are investigated.
below presents summary statistics of the prices considered in this study. As indicates, there is, in general, no noticeable difference in volatility between the markets in each of the commodity markets, measured with the standard deviation and coefficient of variation. illustrates the national trends for the nominal monthly average prices for wheat, teff, and maize at the wholesale level. indicates that each commodity prices show an increasing trend.
Table 1. Descriptive statistics of monthly real grain prices, Birr/kg (prices in log form)
5. Empirical results
The time-series properties of the prices were investigated individually using the ADF and Perron tests. The test statistics indicated that the prices are non-stationary in levels but stationary in their first differences.Footnote6 Hence, a cointegration test can be applied to determine the presence of long-run relationships between the central and local market prices in the Ethiopian grain market. reports the results of the Engle-Granger cointegration tests, including the results of the LOP tests.
Table 2. Engle-Granger(E-G) cointegration test results (
The Engle-Granger cointegration test results (reported in column 3 of ) rejected the null hypothesis of no cointegration between each pair of prices investigated in each commodity market, suggesting the presence of a linear long-run relationship between the central and local market prices. However, the Johansen likelihood test results (reported in column 4 of ) rejected the LOP, indicating the presence of incomplete price transmission between the central and local grain markets in Ethiopia. The incomplete price transmission might result from inefficient arbitrage due to market power in the grain markets or the presence of high transportation costs due to poor market infrastructure in Ethiopia.
Next, the estimated residuals from Equation (1) are estimated as a threshold model using both zero () and non-zero (
) threshold values in each commodity market. For brevity, only the model with the best fit, based on the value of the Akaike information criterion (AIC), are reported. reports the results of the selected model.Footnote7
Table 3. Consistent threshold cointegration & asymmetry test in grain market in Ethiopia
As reported in the table, the estimates of and
are significantly different from zero in general and satisfy the conditions of convergence (i.e.,
and
). Hence, we can proceed with the cointegration test. As reported in column 6 in , the estimated statistics for cointegration
suggest that each pair of prices investigated for each commodity market is cointegrated, which confirms the Engle-Granger cointegration test results.
Next, as cointegration was not rejected in each commodity market, symmetric versus asymmetric price adjustment is tested. Column 7 in reports the estimated F-statistic and the p-value of corresponding significance for the symmetry test . The results in the wheat market show that the null hypothesis of symmetric price transmission is not rejected in any of the market pairs investigated. In the teff market, symmetric price adjustment is rejected only in one out of the four pairs of prices investigated. In the maize market, however, asymmetric price transmission is confirmed in two out of the three pairs of prices investigated. This result indicates the possibility of asymmetric adjustment in the maize market, although it is necessary to consider more regional maize markets for a more robust conclusion about the nature of the price adjustment.
In general, the null hypothesis of symmetric price adjustment is not rejected in nine out of the twelve pairs investigated, which implies that symmetric price adjustment generally characterizes the Ethiopian grain market. This means that, in the Ethiopian grain markets, increases and decreases in central-market prices transmit to local markets at equal speed. This could indicate the existence of efficient price transmission in the Ethiopian grain markets. However, given the discussion above, the presence of efficient price adjustment in these markets is unexpected. The active presence of the state-led trading enterprise, Ethiopian Grain Trade Enterprise (EGTE), and government restrictions on traders holding large stocks of grain might contribute to the efficient adjustment.
Based on these results, I estimate symmetric or threshold asymmetric ECMs for each commodity market. present estimates of the ECMs from the wheat, teff, and maize markets, respectively. Results for the post-estimation tests in each estimated model (such as the Breusch-Godfrey serial correlation test, the Breusch-Pagan test, and the Jarque-Bera test) reveal that the estimated ECMs perform reasonably well, and there are no major misspecification problems.Footnote8
Table 4. Symmetric error correction models (wheat markets)
Table 5. Symmetric & asymmetric error correction models model (teff markets)
Table 6. Symmetric & asymmetric error correction models model (maize markets)
First, concerning the wheat market, symmetric ECMs are estimated for each pair of prices since symmetric transmission is not rejected. As shown in , the adjustment parameter, for the central and local markets is statistically significant at a 5% level (at least) in each of the local-market models. By contrast, in the central market models
is statistically significant only in some of the models, and its significance level is not much stronger than in the local models. This implies that the local market adjusts to changes in central-market prices, whereas the central market might not adjust to changes in local-market prices. The central market not adjusting to changes in the local market price is an indication of the central market’s leadership or market power, which is consistent with the central market being larger than local markets. Furthermore, the estimated speed-of-adjustment parameters for the local market models are low (ranging from 16% to 41%), indicating the presence of a slow correction speed following a shock in the central market. The joint significance of short-run, cross-price effects is indicated by
in for each pair of prices estimated. The reported values are the F-statistics, and the significant parameter indicates that the central and local market prices for wheat are not generally integrated in the short run.
In the teff market, the estimated ECMs (as results shown in ) indicated that the error correction term is statistically significant in both the central and local market models in each estimated model, indicating the presence of bi-directional adjustment. The joint significance of the short-run cross coefficients indicated that only the Addis and Jimma pair are integrated in the short run.
In the maize market, the estimated ECMs (results reported in ) indicate that in all pairs of prices estimated, the central market price does not adjust to local price changes, whereas the local market prices do adjust to changes in central market prices. This indicates the presence of uni-directional price adjustment in the maize market. The joint significance of the short-run cross-coefficients indicates that prices in the central and three local maize markets are not integrated in the short run.
6. Conclusion and discussion
Persistent increases in basic food crop prices have become a critical challenge in Ethiopia since 2006. This study examines the market dynamics of prominent grain categories and possible insights relevant to potential price-stabilization interventions by the government. Spatial markets for wheat, maize, and teff were evaluated, and symmetric and asymmetric convergences for disequilibria. Engle-Granger cointegration test, threshold cointegration, and asymmetric ECMs were estimated between the central (Addis Ababa) and major local grain markets.
The findings suggest that spatial market dependencies exist between the central and local markets and affect the formation of complete markets. Price transmission is similar for the wheat and teff markets as symmetric transmission characterizes the price transmission process. In the maize market, evidence of asymmetric price transmission is obtained, although considering more local markets is required to reach a more robust conclusion as only a few maize markets are investigated in this study. Overall, the findings of this study show that symmetric price adjustment characterizes the Ethiopian grain market, suggesting the existence of efficient price transmission between spatial grain markets in Ethiopia.
The presence of symmetric price adjustment in the Ethiopian grain market is surprising, given that the literature shows the existence of inefficiency in the market (Negassa & Myers, Citation2007; Sassi & Mamo, Citation2019). However, three main factors may contribute to this phenomenon. First, the state trading enterprise (the EGTE) plays an active role in the grain market. The EGTE occasionally buys and sells grains when there is a rise in price and bumpy harvest seasons due to drought (Kifle, Citation2015; Rashid, Citation2011). Second, the government imports grain (wheat particularly) from the international markets and distributes it at a subsidized price to wholesalers and millers to stabilize the markets (Rashid, Citation2011). Third, the government restricts traders from holding large stocks of grains on the assumption that such hoarding could exacerbate price increases.
Thus, this study argues that the sustained grain price increases that have been observed in the Ethiopian grain market have little or nothing to do with the grain market structure. Further investigation is needed to identify the contributing factors of the sustained increases in grain prices in Ethiopia. Possible contributing factors could be supply fluctuation due to drought, rainfall fluctuation, exchange rate fluctuation, world price shocks, and income and population growth.
Finally, the estimated results of the (asymmetric) ECMs suggest the leading role of the central wheat and maize markets since it was the local market prices that adjusted to changes in the central market, not vice versa. However, there exist bi-directional adjustments in the teff market.
Disclosure statement
No potential conflict of interest was reported by the author.
Additional information
Notes on contributors
Dejene Gizaw Kidane
Dejene Gizaw Kidane is a Ph.D. candidate at the School of Bussiness and Economics, UiT The Arctic University of Norway, Norway. His current research areas include market integration, agri-food market, international trade, price transmission, time-series, and econometrics.
Notes
1 However, there are also direct trade flows from the local surplus areas to the deficit areas without passing through Addis Ababa.
2 As it is common in most price transmission literature, transportation costs and quality differences are treated as constant in this study. Nevertheless, if transportation costs are not constant, this assumption may cause rejections of the LOP.
3 Cointegration implies if the prices are non-stationary of same order (i.e., order one, denoted ), only a stationary linear combination of them represents the true price relationship. Specifically, the prices in Equation (1) are said to be cointegrated if the error term,
, is stationarity (i.e.,
).
4 The FAO GIEWS collects monthly grain prices from the national grain board of several countries to support its technical activities. The FAO source in the case of Ethiopia is the Ethiopian Grain Trade Enterprise (EGTE).
5 There are three types of teff in Ethiopia, white, mixed, and red. White teff and mixed teff are the types widely consumed in Ethiopia. Although both have the same purpose, white teff has superior quality, and is preferred by consumers with relatively high purchasing power, whereas mixed teff is consumed mainly by consumers who have low purchasing power. In this study, mixed teff markets are considered since no previous studies have done so.
6 For brevity, the unit root test results are not reported here but can be obtained from the author upon request.
7 Results of all estimated models are available upon request from the author.
8 To save space and for clarity, the diagnostic tests were not reported here. However, can be obtained upon the request from the authors.
References
- Abdulia, A. (2000). Spatial price transmission and asymmetry in the Ghanaian maize market. Journal of Development Economics, 63(2), 327–349.
- Asche, F., Gjølberg, O., & Guttormsen, A. G. (2012). Testing the central market hypothesis: A multivariate analysis of Tanzanian sorghum markets. Journal of Agricultural Economics, 43(1), 115–123.
- Balke, N. S., & Fomby, T. B. (1997). Threshold cointegration. International Economic Review, 38(3), 627–645.
- Baquedano, F. G., & Liefert, W. M. (2014, February). Market integration and price transmission in consumer markets of developing countries. Food Policy, 44, 103–114.
- Barrett, C. B., & Li, J. R. (2002). Distinguishing between equilibrium and integration in spatial price analysis. American Journal of Agricultural Economics, 84(2), 292–307.
- Brækkan, E. H., Thyholdt, S. B., Asche, F., & Myrland, Ø. (2018). The demands they are a-changin’. European Review of Agricultural Economics, 45(4), 531–52.
- Ceballos, F., Hernandez, M. A., Minot, N., & Robles, M. (2017). Grain price and volatility transmission from international to domestic markets in developing countries. World Development, 94, 305–320.
- Chan, K. S. (1993). Consistency and limiting distribution of the least squares estimator of a threshold autoregressive model. The Annals of Statistics, 21(1), 520–533.
- Conforti, P. (2004). Price transmission in selected agricultural markets. Commodity and Trade Policy Research Working Paper 7. Rome: Food and Agriculture Organization (FAO).
- Dawe, D. (2008). Have recent increases in international cereal prices been transmitted to domestic economies? The experience in seven large Asian countries. ESA working paper No. 08-03, Development Economics Divisions, Food, and agriculture Organization.
- Dickey, D. A., & Fuller, W. A. (1981). Likelihood ratio statistics for autoregressive time series with a unit root. Econometrica, 49(4), 1057–1072.
- Enders, W., & Granger, C. W. J. (1998). Unit-root tests and asymmetric adjustment with an example using the term structure of interest rates. Journal of Business &economic Statistics, 16(3), 304–311.
- Enders, W., & Siklos, P. L. (2001). Cointegration and threshold adjustment. Journal of Business & Economic Statistics, 19(2), 166–176.
- Engle, R. F., & Granger, C. W. J. (1987). Cointegration and Error Correction: Representation, Estimation and Testing. Econometrica, 55(2), 251–276.
- Fackler, P. L., & Goodwin, B. K. (2001). Spatial price analysis. In B. Gardner & G. Rausser (Eds.), Handbook of Agricultural Economics (pp. 971–1024). Amsterdam: Elsevier Science B.V.
- Frey, G., & Manera, M. (2007). Econometric models of asymmetric price transmission. Journal of Economic Surveys, 21(2), 349–415.
- Gabre-Madhin, E. Z., & Goggin, I. (2005). Does Ethiopia need a commodity exchange? An integrated approach to market development. EDRI-ESSP Policy working Paper No. 4. Ethiopian Development Research Institute, Addiss Ababa, Ethiopia.
- Gebremeskel, D., Jayne, T., & Shaffer, J. (1998). Market structure, conduct, and performance: Constraints on performance of Ethiopian grain markets., Ministry of Economic Development and Cooperation (Ethiopia), Grain market Research Project, working paper No.8.
- Getnet, K. (2007). Spatial equilibrium of wheat markets in Ethiopia. African Development Review, 19(2), 281–303.
- Getnet, K., Verbeke, W., & Viaene, J. (2005). Modeling spatial price transmission in the grain markets of Ethiopia with an application to ARDL approach to white teff. Agricultural Economics, 33(s3), 491–502.
- Goodwin, B. K., & Schroeder, T. C. (1991). Cointegration tests and spatial price linkages in regional cattle markets. American Journal of Agricultural Economics, 72(2), 452–464.
- Johansen, S., & Juselius, K. (1990). Maximum likelihood Estimation and Inference on Cointegration. Oxford Bulletin of Economics and Statistics, 52(2), 169–210.
- Kifle, W. (2015). Price transmission asymmetry in spatial grain markets in Ethiopia. African Development Review, 27(2), 106–116.
- Kindie, G. (2008). From market liberalization to market development: The need for market institutions in Ethiopia. Economic Systems, 32(3), 239–252.
- Kinnucan, H. W., & Forker, O. D. (1987). Asymmetry in farm-retail price transmission for major dairy products. American Journal of Agricultural Economics, 69(2), 285–292.
- Minot, N. (2011). Transmission of world price changes to markets in sub-Saharan Africa. IFPRI Discussion Paper No. 1059. Washington D.C.: International Food Policy Research Institute.
- Mundlak, Y., & Larson, D. (1992). On transmission of world agriculture prices. The World Bank Economic Review, 6(3), 399–422.
- Negassa, A., & Myers, R. (2007). Estimating policy effects on spatial market efficiency: An extension to the parity bound model. American Journal of Agricultural Economics, 89(2), 338–352.
- Negassa, A., Myers, R., & Gabre-Madhin, E. (2004). Grain marketing policy changes and spatial efficiency of maize and wheat markets in Ethiopia International Food Policy Research Institute. Washington, D.C.
- Osborne, T. (2004). Market news in commodity price theory: Application to the Ethiopian grain market. The Review of Economic Studies, 71(1), 133–164.
- Ozturk, O. (2020). Market integration and spatial price transmission in grain markets of Turkey. Applied Economics, 52(18), 1936–1948”.
- Perron, P. (1997). Further evidence on breaking trend functions in macroeconomic variables. Journal of Econometrics, 80(2), 355–385.
- Rapsomanikis, G., Hallam, D., & Conforti, P. (2006). Market Integration and Price Transmission in Selected Food and Cash Crop Markets of Developing Countries: Review and Applications. InFAO commodity and trade policy research working paper No.7.
- Rashid, S. (2011). Inter-commodity price transmission and food price policies: An analysis of Ethiopia cereals markets. IFPRI Discussion Paper No. 1079. Washington, D.C.: International Food Policy Research Institute.
- Sassi, M., & Mamo, Y. A. (2019). Vertical price transmission in the white teff market in Ethiopia. Agrekon, 58(2), 229–243.
- Serra, T., & Gil, J. M. (2006). Local polynomial fitting and spatial price relationships: Price transmission in EU pork markets. European Review of Agricultural Economics, 33(3), 415–436.
- Sexton, R. J., Kling, C. L., & Carman, H. F. (1991). Market integration, efficiency of arbitrage and imperfect competition:methodology and application to US celery. American Journal of Agricultural Economics, 73(3), 568–580.
- Shahidur, R., & Asfaw, N. (2011). Policies and performance of ethiopian cereal markets. Ethiopia strategy support programme II (ESSP II). International food policy research institute, ESSP II Working Paper No.21.
- Tadesse, G., & Guttormsen, A. (2011). The behavior of commodity prices in Ethiopia. Agricultural Economics, 42(1), 87–97.
- Usman, M. A., & Haile, M. G. (2017). Producer to retailer price transmission in cereal markets of Ethiopia. Food Security, 9(4), 815–829.
- von Cramon-taubadel, S., & Meyer, J. (2004). Asymmetric price transmission: A survey. Journal of Agricultural Economics, 55(3), 581–611.
- Yami, M. (2020). Market integration and price transmission of maize in Ethiopia. Ethiopian Journal of Agricultural Science, 30(4), 277–292.