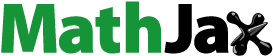
ABSTRACT
Based on the university enrollment expansion policy implemented by the Chinese government in 1999, I use the difference-in-difference method to explore the effects of human capital expansion on firms’ markup and the influencing mechanism. Findings suggest that human capital expansion significantly promotes the improvement of firms’ markup, which remains true after a series of robustness tests. The analysis of the influencing mechanism shows that on the one hand, human capital expansion promotes the improvement of firms’ production efficiency and improves their markup through the production efficiency channel. On the other hand, human capital expansion improves the market pricing power of firms and exerts a significant positive impact on their markup through the market pricing channel. Further analysis also shows the varying impacts of human capital expansion on the markup of different types of firms. This study enhances the understanding of the microeconomic effects of human capital expansion from the markup perspective.
1. Introduction
Firms’ markup measuring how much the product price deviates from the marginal cost is a key indicator that reflects the market influence and profitability of firms. Such a markup is also a concentrated embodiment of the division position of firms in the global value chain. Export firms’ markup even determines countries’ welfare levels in global trade (Edmond, Midrigan, & Xu, Citation2015). Maintaining a high markup is one of the important signs of firms’ dynamic competitiveness. The reason multinational companies can dominate the industry development pattern is that they have formed the dynamic competitiveness of “high markup, high profit, and continuous innovation.” Therefore, an in-depth analysis of the relevant influencing factors of firms’ markup is critical to considerably promote the increase of firms’ markup, improve their competitiveness and profitability, and realize their sustainable and stable development. Although existing studies explore the impact of relevant factors on firms’ markup from the perspectives of export behavior (Bellone, Musso, Nesta, & Warzynski, Citation2016; Melitz & Ottaviano, Citation2008), trade liberalization (De Loecker, Goldberg, Khandelwal, & Pavcnik, Citation2016; Fan, Gao, Li, & Luong, Citation2018; Xiang, Chen, Ho, & Yue, Citation2017), and minimum wage (Du & Wang, Citation2020), they disregard the role of human capital.
The university enrollment expansion policy implemented by the Chinese government starting in 1999 has led to a rapid increase in the supply of high-level human capital represented by college graduates from 2003 onward. This phenomenon provides us with a rare opportunity to analyze and understand the impact of human capital expansion on firms’ markup. As one of the most important policies of China’s higher education reform (Meng, Shen, & Xue, Citation2013), the university enrollment expansion policy directly led to an increase in enrollment from 1.083 million in 1998 to 1.597 million in 1999, an increase rate of 47.4%. Since then, enrollment in colleges and universities has continued to rapidly increase. By 2017, enrollment had reached 7.72 million, an increase of 612.8% over 1998. Corresponding to the rapid expansion of college enrollment, the number of college graduates in China also increased substantially beginning in 2003. The number of college graduates increased from 1.337 million in 2002 to 1.877 million in 2003, an increase of 40.4%. By 2017, the number of college graduates had reached 7.95 million.Footnote1
In theory, human capital expansion affects firms’ markup through various ways. The rapid increase in the supply of high-level human capital, represented by college graduates, after the implementation of the university enrollment expansion policy can relatively alleviate the shortage in high-skilled labor supply faced by firms and improve the average quality of labor they employ. On the one hand, the participation of numerous high-skilled human capital is conducive to the development and improvement of new and existing products, respectively, and promotes the enhancement of product quality (Can & Gozgor, Citation2018; Schott, Citation2004). The pricing power of firms is improved, and they can maintain high product prices. On the other hand, such a participation is conducive to the development of relevant innovation activities, thereby promoting the adoption of new production technology and improving total factor productivity (TFP) (Che & Zhang, Citation2018). Thus, the marginal cost of production is reduced. These aspects are advantageous to the improvement of firms’ markup. Affecting the product pricing and production efficiency of firms may be the internal channel for human capital expansion to impact firms’ markup. To explore whether this case is true, the current study uses a micro data set of Chinese manufacturing firms from 1999 to 2007 as basis to first estimate firm-level markup following the method of De Loecker and Warzynski (Citation2012). Thereafter, the current research uses the difference-in-difference (DID) method to identify the impact of human capital expansion on firms’ markup and its influence mechanism by taking the university enrollment expansion policy as a quasi-natural experiment.
This study is closely related to two streams of literature. The first stream is the literature on the economic effect of human capital, which is of wide concern in academic circles. Majority of the early related studies focus on the impact of human capital on economic growth and TFP. Endogenous economic growth theory emphasizes that human capital is the main source of economic growth (Romer, Citation1990), which is supported by numerous empirical studies. Barro (Citation1991) finds that human capital significantly increases countries’ economic growth rate based on cross-country data from 1960 to 1985. Miller and Upadhyay (Citation2000) conduct an empirical study based on cross-country panel data, and they show that human capital generally promotes TFP growth. Madsen (Citation2014) uses the data of 21 OECD countries from 1870 to 2009 and determines that human capital significantly increases TFP. Benhabib and Spiegel (Citation2005), Ciccone and Papaioannou (Citation2009) and Hanushek and Woessmann (, Citation2012) find that human capital can promote economic growth. However, most of the early studies in this field investigate the impact of human capital on economic growth or TFP from the macro level of a country or region. This type of analysis based on macro-level aggregated data often faces the interference of the endogenous problem of human capital, possibly leading to deviations in the corresponding conclusions (Che & Zhang, Citation2018; Kong, Zhang, & Zhang, Citation2020). On the one hand, a reverse causal relationship may exist between human capital accumulation and economic growth in a country or region. On the other hand, human capital accumulation and economic growth in a country or region may be affected simultaneously by missing variables.
With the availability of micro data in recent years, the related research begins to focus on the microeconomic effects of human capital. Che and Zhang (Citation2018) use the differences in human capital intensity in various industries as bases in taking the university enrollment expansion policy as a quasi-natural experiment to analyze the impact of human capital expansion on TFP of Chinese firms. They show that human capital expansion promotes the adoption of new production technology and significantly improves firms’ TFP. Kong et al. (Citation2020) utilize the data of Chinese listed companies as bases in using the DID method to explore the impact of human capital expansion on firm innovation, particularly by taking the university enrollment expansion policy as a quasi-natural experiment. They find that human capital expansion has a significant positive effect on firm innovation. Moreover, this positive effect is evident among firms headquartered in provinces with more science and engineering graduates, young firms that are willing to employ young graduates, and firms located near universities. Rong and Wu (Citation2020) also analyze the impact on firm innovation of the increase in human capital supply resulting from the university enrollment expansion policy. However, their research focuses considerably on how the reallocation of scientific researchers from the private to the public sector induced by China’s college expansion affects the innovation of private sector firms. Ashraf and Ray (Citation2017) and Sun, Li, and Ghosal (Citation2020) find that an increase in human capital supply promotes firm innovation.
The second stream is the literature analyzing the influencing factors of firms’ markup. Although numerous studies in this field are currently being conducted, early research focuses on the impact of productivity and export on firms’ markup. Bernard, Eaton, Jensen, and Kortum (Citation2003) analyze the relationship between firms’ export behavior and their productivity and markup under the framework of an imperfectly competitive model. They show that firms with high productivity are substantially capable of setting a high markup. By contrast, Melitz and Ottaviano (Citation2008) internalize firms’ markup into a model by introducing a quasi-linear demand system and theoretically prove that firms with high productivity have high markup. Görg and Warzynski (Citation2003) use firm data from the British manufacturing industry to analyze the influence of firms’ export on markup, and they determine that their export behavior is conducive to markup improvement. Kugler and Verhoogen (Citation2008), De Loecker and Warzynski (Citation2012), Kato (Citation2014) and Bellone et al. (Citation2016) study Colombian, Slovenian, Japanese, and French firms, respectively, and find that their exports significantly improve markup. Manova and Zhang (Citation2009) and Kilinç (Citation2019) go beyond simply focusing on the impact of firms’ exports on markup and further analyze the impact of the choice of export destination countries on markup.
Studies recently analyze the impact of relevant factors on firms’ markup from various perspectives. Du and Wang (Citation2020) empirically analyze the impact of minimum wage on firms’ markup based on firm-level data from China. They find that minimum wage has a significantly positive impact on firms’ markup through channels such as promoting their innovation activities, improving TFP, and enhancing the efficiency of labor resource allocation. Du, Luan, and Lu (Citation2020) use the data of Chinese manufacturing firms as bases to analyze the impact of economic policy uncertainty and financing constraints on firms’ markup. They find that economic policy uncertainty and financing constraints significantly reduce firms’ markup. Some other studies analyze the effects on firms’ markup of import competition (Caselli & Markups, Citation2020; Garcia-Marin & Voigtländer, Citation2019; Meinen, Citation2016), trade liberalization (De Loecker et al., Citation2016; Fan et al., Citation2018; Xiang et al., Citation2017), exchange rate shock (Weinberger, Citation2020), innovation (Máñez et al., Citation2020), and intangible asset investment (Altomonte, Favoino, & Morlacco et al., Citation2020).
This study contributes to the literature in three aspects. First, this study focuses on firms’ markup and uses the DID method to investigate in detail on how human capital expansion affects the markup of Chinese manufacturing firms by taking the university enrollment expansion policy as a quasi-natural experiment. After a series of robustness tests, this study verifies the conclusion that human capital expansion significantly improves firms’ markup. Cao and Mao (Citation2019) also construct the DID model to study the effects of the expansion of human capital on firms’ markups by taking the expansion of Chinese colleges as a quasi-natural experiment. This study further extends their research by constructing the triple difference model to mitigate the possible interference of other policies in the same period and making more robustness tests such as quantile regression, substitution of key indicators, excluding the impact of three-year graduates, and so on. Moreover, this study exerts effort to exclude the impact of China’s accession to the World Trade Organization (WTO) on the estimation results. Therefore, this study provides relatively more reliable evidence for understanding the impact of human capital expansion on firms’ markups. Through an in-depth investigation of the impact of human capital expansion on firm-level markup, this study breaks through the limitations of the existing literature that mainly analyzes the economic effects of human capital at the national or regional macro level and enriches the literature evaluating the impact of human capital on the micro performance of firms.
Second, this study focuses on the impact of human capital expansion on firms’ markup and explores the mechanism of such an expansion through the production efficiency and market pricing channels. On the one hand, human capital expansion promotes the improvement of firms’ production efficiency and enhances their markup through the production efficiency channel. On the other hand, human capital expansion improves the market pricing power of firms (i.e., price levels of products) and exerts a significant positive impact on their markup through the market pricing channel. Furthermore, human capital expansion mainly affects the market pricing power and production efficiency of firms by promoting the development of firm innovation activities. Evidently, an in-depth analysis of the impact mechanism is conducive to deepening the understanding of the relationship between human capital expansion and firms’ markup.
Third, this study discusses the heterogeneous impacts of human capital expansion on different types of firms (i.e., different financing constraint types, different scale types, different age types, different ownership types, and export and non-export firms). Through the analyses from multiple perspectives, this study provides a comprehensive understanding of the markup effects caused by human capital expansion.
The remainder of this paper is organized as follows. Section 2 describes the empirical model and data sources. Section 3 presents the empirical results. Section 4 examines the mechanism through which human capital expansion affects firms’ markup. Section 5 explores the heterogeneous effects of human capital expansion on the markup of different types of firms. Section 6 concludes.
2. Empirical model and data
2.1. Empirical model setting
The Chinese government’s university enrollment expansion policy in 1999 has led to the rapid increase in the supply of advanced human capital after 2003. Industries with different human capital intensities are significantly affected by the expansion of human capital, particularly industries with high human capital intensity (Che & Zhang, Citation2018). Thus, I set the following DID regression model:
where subscripts i, j, and t represent firm, three-digit ISIC industry, and year, respectively; the explained variable is the logarithm of the markup of firm i in industry j in period t; and
is the human capital intensity of industry j. Basing on the study of (Ciccone & Papaioannou, Citation2009), I use the proportion of workers with four years or more college education in various industries in the US in 1980 to measure
. The reason for using the human capital intensity of various industries in the US in the 1980s lies on the following considerations. The US labor market is highly flexible, and the country invented numerous technologies in the 1970s. In general, the human capital intensity of American industries can relatively better reflect the technological frontier of various industries. By contrast, if we use China’s data to measure the human capital intensity of various industries, the result is likely to be affected by other policy factors or distortion of labor market allocation (Che & Zhang, Citation2018).
is an indicator equal to 1 for 2003 and later years, and 0 for years before 2003;
represents the set of other control variables: firm size, factor intensity, average wage, firm age, government subsidies, and industry concentration (HHI);
is the firm fixed effect;
is the year fixed effect; and
is the random disturbance term. The estimation coefficient of the cross-term
measures the average difference of the markup between high and low human capital-intensive industries before and after the expansion of human capital.
2.2. Measurement of firms’ markup
The dependent variable in the regression EquationEquation (1)(1)
(1) is the logarithm of firms’ markup, so we need to first estimate firm-level markup. Following the method of De Loecker and Warzynski (Citation2012), I define firm-level markup as the ratio of product price to marginal cost. The markup
of firm
in period
is then defined as
where is the proportion of total expenditure of variable input (
) in the total sales revenue of the firm.
can be directly calculated from the production data of the firm. Therefore, to measure the firm-level markups, we only need to estimate the output elasticity (
) of one (or several) variable input(s) in the production.
To obtain the output elasticity of a variable input in the firm’s production, we should estimate the firm’s production function. However, production function estimation, the endogeneity between factor input and TFP can make OLS ineffective. I use the semi-parameter estimation method (ACF method for short) developed by Ackerberg, Caves, and Frazer (Citation2015) to solve the endogeneity problem in production function estimation. Specifically, the following translog production function is estimated using the ACF method:
where is the logarithm of the total output of firm
in period
,
is the logarithm of labor input,
is the logarithm of intermediate inputs,
is the logarithm of capital stock,
is the TFP, and
is the random error term. According to Lu and Yu (Citation2015), labor is not a variable input for Chinese firms, especially for state-owned enterprises (SOEs). As capital is a dynamic input, firms’ markup needs to be calculated by estimating the output elasticity
of the intermediate inputs (
). The output elasticity of intermediate inputs can be easily derived from EquationEquation (3)
(3)
(3) .
Firms’ markup can be estimated using EquationEquations (2)(2)
(2) and (Equation4
(4)
(4) ).
2.3. Data
The firm-level micro data used in this study come from the Annual Survey of Industrial Firms (ASIF) compiled by the National Bureau of Statistics of China. The ASIF covers all state-owned industrial firms and those of other ownership types with sales above RMB 5 million. Similar to the existing related research, considering the continuity of the relevant indicators and the reliability of the research results, I select the manufacturing firms in the ASIF database from 1999 to 2007 as the research samples. Following the studies of Feenstra, Li, and Yu (Citation2014), Yu (Citation2015) and Xiang et al. (Citation2017), I first clean up and screen the original data of the ASIF database. I exclude observations with missing values for key variables and those that fail to satisfy basic error checks. For example, I eliminate a firm if any of the following statements are true: (1) less than eight employees, (2) missing key financial variables (e.g., total fixed assets, value added, gross output, intermediate input, and employees), (3) total fixed assets greater than total assets, (4) paid-in capital less than zero, or (5) net value of fixed assets greater than total assets.
To obtain firm-level markup, the firm production function must be estimated. I use the ACF method to estimate the production function for each three-digit ISIC industry to better reflect the differences in production technology between industries. As the ASIF database does not contain any information on output and input prices of firms, I estimate the revenue-based production function shown in EquationEquation (3)(3)
(3) by using the ACF method for each industry. On the basis of the estimated production function, the output elasticity of intermediate inputs (see Table A1 in Appendix A for details) can easily be obtained from EquationEquation (4)
(4)
(4) . Firm-level markups can then be obtained further using EquationEquation (2)
(2)
(2) . reports the average markups (simple average) and quantiles for each two-digit code industry during the sample period. The average markups in all industries are between 1 and 2.
Table 1. Average markups and quantiles for each industry
In the following empirical analysis, combined with the needs of relevant analysis, this study uses the ASIF database as well as tariff data at the product level, trade data of firms, patent application data of firms, and number of full-time teachers and students in colleges and universities in each province of China. These data are from the Shanghai WTO Affairs Center, China Customs Trade Statistics database, patent database of all state-owned and large-scale industrial enterprises from 1985 to 2013 issued by China Intellectual Property Office, and annual education statistical yearbooks of all provinces in China.
3. Analysis and discussion of empirical results
3.1. Benchmark regression results
shows the corresponding estimation results of EquationEquation (1)(1)
(1) . In columns (1) and (3), industry and year fixed effects are included. In columns (2) and (4), firm and year fixed effects are included. In columns (1) and (2), without any control variables, I directly regress firms’ markup on the interaction term
. The results show that the estimated coefficient of the interaction term
is significantly positive, indicating that human capital expansion is conducive to the improvement of firms’ markup. In columns (3) and (4), I add the control variables, such as firm scale, factor intensity, average wage, firm age, government subsidies, and HHI. The regression results are similar to those in columns (1) and (2). The estimated coefficient of the interaction term remains significantly positive, which indicates that human capital expansion significantly improves firms’ markup. In particular, the regression results in column (4) show that the estimated coefficient of the interaction term
is 0.0267 with significance at the 1% level. This result indicates that the average markup growth between 2000–2002 and 2003–2007 of firms in the most human capital-intensive industry (i.e., chemicals, with human capital intensity of 0.27) is 0.6 percentage points (
) higher than that of firms in the least human capital-intensive industry (i.e., footwear, with human capital intensity of 0.037).
Table 2. Benchmark regression results
3.2. Parallel trend test
The premise of using the DID method to effectively identify policy effects is that the treatment and control groups have a common change trend before policy shocks. To ensure the effectiveness of the DID method used before, we must check whether firms’ markups in different human capital-intensive industries have a common change trend before the policy shock. Therefore, I consider the following dynamic effect regression equation
where Yr is the corresponding year dummy variable. The estimation of EquationEquation (5)(5)
(5) enables us to clearly understand how human capital expansion brought about by the university enrollment expansion affects firms’ markup each year compared with that in 2000. Moreover, the analysis of the change of firms’ markup before 2003 can help us identify whether the change of firms’ markup in different industries follows the same trend before human capital expansion.
I plot the estimation result of EquationEquation (5)(5)
(5) in , in which the corresponding regression coefficient depicts the marginal effect of human capital expansion on firms’ markup, and the real line depicts the 95% confidence interval of the estimation coefficient. Before 2003, the estimated coefficient of the impact of human capital expansion on firms’ markup is not significant. However, the estimated coefficients from 2003 are significantly positive. The corresponding estimated coefficient reaches the maximum in 2004 and decreases thereafter. Evidently, after the rapid expansion of college graduates caused by the implementation of the university enrollment expansion policy, the promotion effect of human capital on firms’ markup presents an inverted U-shaped dynamic change. Overall, the results in indicate that the change of firms’ markup of the treatment group (high human capital intensity industries) and of the control group (low human capital intensity industries) meets the parallel trend assumption before the policy shock.
3.3. Excluding the impact of China’s accession to the world trade organization
The sample period of this study is from 1999 to 2007.China formally joined the WTO at the end of 2001, and trade liberalization in China further accelerated thereafter. However, import competition brought about by trade liberalization significantly reduced Chinese firms’ markup (Xiang et al., Citation2017), possibly having an impact on previous analysis results. For robustness, this section attempts to use a variety of methods to exclude the impact of China’s accession to WTO on the previous regression results. First, I only select data in 2002 and later years to construct a post-WTO accession subsample. I re-estimate EquationEquation (1)(1)
(1) with the post-WTO accession subsample. The results in column (1) of show that the estimated coefficient of the interaction term
remains significantly positive. This result indicates that human capital expansion significantly improves firms’ markup, which is similar to the previous benchmark regression results.
Table 3. Robustness: China’s accession to WTO
Second, given that the main impact of trade liberalization after China’s WTO accession is on foreign trade firms, I remove the export firms from the total sample to construct a new subsample. The corresponding results are shown in column (2) of . The estimated coefficient of the interaction term still remains positive and is significant at the significance level of 10%.
Third, I follow Yu (Citation2015) and further match the ASIF database with the Customs Trade Statistics database and tariff data and construct the firm-level output and input tariffs to measure the degree of trade liberalization faced by firms. I re-estimate EquationEquation (1)(1)
(1) by adding firm-level output and input tariffs as control variables. The results in column (3) of show that the estimated coefficient of output tariff is significantly positive, indicating that the decline of output tariff significantly reduces firms’ markup. This result is consistent with the finding of Xiang et al. (Citation2017). The estimated coefficient of input tariff is not significant, indicating that its decline does not have a significant impact on firms’ markup. Moreover, the estimated coefficient of the interaction term remains significantly positive. This outcome indicates that the positive impact of human capital expansion on firms’ markup remains significant even if firm-level tariffs are controlled.
Finally, considering that the export market is often characterized by higher prices than the domestic market, the increase in markups of firms may be driven by China’s accession to WTO (which occurs roughly at the same time), and the ensuing export opportunities facing Chinese firms are not driven by the human capital expansion. To rule out that the possibility that the increase of firms’ markups during the sample period is not driven by China’s accession to WTO, I use the export propensity of industries (denoted by Export_p) instead of human capital intensity of industries and interact the export propensity of industries with the variable post03 to re-estimate EquationEquation (1)(1)
(1) . The results in column (4) of show that the estimated coefficient of the interaction term
is not significant. To an extent, this result indicates that the increase of firms’ markups during the sample period is not driven by China’s accession to WTO and the ensuing export opportunities facing Chinese firms.
Overall, the estimated results in have good robustness and show that the previous results are less likely to be affected by China’s WTO accession.
3.4. Estimation of the triple difference model
The previous analysis is based on the DID method, and one of its assumptions is that policy shock should be exogenous. If other policies have inconsistent effects on the treatment and control groups, then previous estimation results may be biased. To test the robustness of the previous estimation results, I further construct a triple difference model to mitigate the possible interference of other policies in the same period.
Owing to the unbalanced regional distribution of universities in China, the number of universities owned by different provinces and regions is relatively different, and majority of university graduates live and work in provinces where the universities they attend are located (Peking University, Citation2011). Therefore, the supply of skilled labor in provinces with more college graduates should increase more (Kong et al., Citation2020). In this sense, the shock of human capital supply caused by the university enrollment expansion policy will vary in different provinces and regions, which may lead to large differences in the impact of human capital expansion on firms in these areas. For example, majority of colleges and universities in China are concentrated in the central and eastern regions owing to the high-level economic development in these regions. With the implementation of the university enrollment expansion policy, the number of college graduates in the central and eastern regions increases substantially. Substantial increase in advanced human capital supply is likely to have considerable impact on the performance of firms in these provinces and regions. For this reason, I construct the following triple difference model:
where subscripts i, j, k, and t represent firm, industry, province, and year, respectively; DDDjkt is , and prok is a variable to measure the number of university graduates in different provinces. The estimated coefficient
measures the average difference of firms’ markup between high and low human capital-intensive industries in different provinces (with different number of college graduates) before and after the expansion of human capital.
Given the lack of data on the number of university graduates in each province in the sample period, I use the number of full-time college teachers (DDD_ T) and college students (DDD_ S) in each province to measure the difference in the human capital supply in each province. In general, if a province has more full-time college teachers or college students, then we are convinced that there will be more graduates in this province. After the university enrollment expansion policy, the supply of advanced human capital in this province also increases substantially. The estimation results in columns (1) and (2) of show that the estimated coefficients of DDD_ T and DDD_ S are significantly positive, similar to previous estimates. As the number of full-time college teachers and students in each province is likely to be affected simultaneously by other relevant policy factors, directly using such a number may be inappropriate to measure the difference inhuman capital supply in each province after the university enrollment expansion policy. On the one hand, I use the number of full-time college teachers and students in each province in 1998 (before the implementation of the university enrollment expansion policy) to measure the difference in human capital supply in each province after the policy’s implementation. The results in columns (3) and (4) of show that the estimated coefficients of DDD_ T_ 1998 and DDD_ S_1998 remain significantly positive. On the other hand, I use the average number of full-time college teachers and students in each province in the sample period to measure the difference in human capital supply in each province after the university enrollment expansion policy. The results in columns (5) and (6) of remain similar to the previous estimation.
Table 4. Results of the triple difference model
I also use the number of key universities owned by each province to measure prok to substantially measure the difference in human capital supply in each province after the university enrollment expansion policy. The corresponding estimation results also show that human capital expansion significantly improves firms’ markup (see Appendix B for details). Overall, the estimation results based on the triple difference model show that the previous benchmark regression results are relatively robust.
3.5. Quantile regression
Previous analysis is based on the results of “mean regression,” which is easily affected by extreme values (Koenker & Bassett, Citation1978). I further consider the quantile regression to considerably avoid the impact of extreme values on the previous regression results and comprehensively analyze the impact of human capital expansion on the entire conditional distribution of firms’ markup. The results in show that that at different quantiles, human capital expansion has a positive impact on firms’ markup. Although the estimated coefficients at the 10 and 25 quantiles are not significant, the impact of human capital expansion on firms’ markup is extremely significant in other quantiles. With an increase in quantile, the absolute value of the quantile regression coefficient of the interaction term shows an initial trend of increasing and decreasing thereafter. Thus, the positive effects of human capital expansion on both ends of the conditional distribution of markup is less than the effects on the middle part. In particular, the positive effects of human capital expansion on firms with high markup are relatively greater and more significant. By contrast, the positive effects of human capital expansion on firms with low markup is relatively small and not significant. These results show that previous regression results are relatively robust, even considering the quantile regression.
Table 5. Quantile regression results
3.6. Additional robustness tests
3.6.1. Using the accounting method to estimate firms’ markup
In the previous analysisFootnote2, I estimate firm-level markups following the method of (De Loecker & Warzynski, Citation2012). This method is also called the production method because it mainly relies on the estimation of production function. In the existing literature, the accounting method is another commonly used technique to estimate firms’ markup. This method mainly uses financial indicators, such as industrial added value, wage input, and cost of intermediate inputs, to calculate firms’ markup. Compared with the production method, the accounting method is relatively simple and the required financial index data are relatively easy to obtain. Therefore, the accounting method is also widely used in the relevant studies. In this section, I follow Domowitz, Hubbard, and Petersen (Citation1986) and use the accounting method to calculate firms’ markup (Table C1 in Appendix C reports the average markups calculated by the accounting method for each industry). I re-estimate EquationEquation (1)(1)
(1) using the markup estimated by the accounting method. The result in column (1) of shows that the estimated coefficient of the interaction term
remains significantly positive. This result shows that even if we use the accounting method to calculate firms’ markup, the previous benchmark regression results are robust.
Table 6. Further robustness tests
3.6.2. Controlling for province-year fixed effect
Che and Zhang, (Citation2018) believe that the regional distribution of industries may not be random, and regions with more human capital intensity industries in history may implement some industrial policies conducive to the growth of these industries. The heterogeneity of regional economic policies may have an impact on the previous estimation results. To alleviate the confounding effects caused by heterogeneous regional economic policies, I follow Kong et al. (Citation2020) and further control for the province-year fixed effect. The estimation results in column (2) of show that the estimated coefficient of the interaction term remains significantly positive. This outcome is similar to the previous regression results, thereby confirming that human capital expansion improves firms’ markup.
3.6.3. Excluding the impact of three-year graduates
China’s undergraduate education system lasts four years. That is, after the implementation of the university enrollment expansion policy in 1999, these undergraduate students should have graduated in 2003 and entered the labor market. However, this policy has affected the four-year undergraduate system and the three-year junior college (relatively small proportion). Students admitted to the three-year junior college program in 1999 should have graduated in 2002, which may have increased the labor supply before 2003. This outcome may interfere with previous estimation results. To address this concern, I exclude the transition years (i.e., 2002 and 2003) from the sample and re-estimate EquationEquation (1)(1)
(1) . The results in column (3) of show that the estimated coefficient of the interaction term
remains significantly positive, similar to previous regression results.
3.6.4. Controlling for the impact of SOE reforms
During the sample period, China has implemented significant reforms in SOEs, including privatizing or merging small and large SOEs. Previous results may have been attributed to the potential impact of the SOE reforms. To address this issue, I include an interaction term between government ownership and the post-2003 indicator. Government ownership (SOE_ R) is measured by the proportion of state-owned capital to total capital at the industry level. The estimation results in column (4) of show that the estimated coefficient of the interaction term remains positive and highly significant. This outcome indicates that the promotion effect of human capital expansion on firms’ markup is not substantially affected by SOE reforms.
3.6.5. Two-period difference method
Bertrand, Duflo, and Mullainathan (Citation2004) explain that using multi-period data for DID estimation may have sequence correlation problems. This study also uses multi-period data for DID estimation to determine the impact of human capital expansion on firms’ markup. The potential sequence correlation problems may exaggerate the significance of the estimated coefficient. To address this concern, I use the actual policy shock year 2003 as basis to divide the sample period into two periods: 2000–2002 and 2003–2007. The two-period difference method is further used for robustness testing, as shown in column (5) of . The estimated coefficient of the interaction term remains significantly positive, which once again shows that previous estimation results are robust.
4. Influence mechanism analysis
The previous analysis shows that human capital expansion significantly improves firms’ markup. I follow De Loecker and Warzynski (Citation2012) and define markup as the ratio of product price to marginal cost: . Markup mainly depends on the marginal cost of production and price level of products. The marginal cost of production is determined by production efficiency, whereas the price level of products is considerably determined by the market pricing power. In the case of the same production efficiency, the greater the market pricing power, the higher the markup will be, and the more market profits firms can obtain. When the market pricing power is certain, the marginal cost of firms with higher production efficiency is lower, and the markup will be higher. Therefore, any factor that affects firms’ pricing and production efficiency (i.e., marginal cost of production) impacts their markup, which can be summarized as the “market pricing” and “production efficiency” channels, respectively. I further investigate the mechanism of human capital expansion affecting firms’ markup from the market pricing and production efficiency channels.
The data used in this study are from ASIF. Given that this database does not directly contain information on the price and marginal cost of firms’ products, I follow the similar approach of De Loecker and Warzynski (Citation2012) and indirectly investigate the production efficiency and market pricing channels of human capital expansion affecting firms’ markup. De Loecker and Warzynski (Citation2012) indicate that the marginal cost of firm production mainly depends on productivity. We can identify the production efficiency channel of human capital expansion affecting markup from the perspective of firm productivity. De Loecker and Warzynski (Citation2012) explain that . When firm productivity is controlled for in the regression model, the difference in the marginal cost of firms can be controlled for and the differences of product prices can be indirectly identified. We can indirectly identify the market pricing channels through which human capital expansion affect firms’ markup by adding their TFP into the benchmark regression EquationEquation (1)
(1)
(1) as the control variable. The corresponding econometric model is as follows
where is the total factor productivity of firm i in industry j in period t, and other variables have the same meanings as previously presented. For firms’ TFP, I use the ACF method to estimate.
Columns (1) and (2) of present the estimation results of EquationEquations (7)(7)
(7) and (Equation8
(8)
(8) ), respectively. In column (1), the dependent variable is firms’ markup, and TFP is added as the control variable to exclude the impact of human capital expansion on firms’ production efficiency. The results show that the estimated coefficient of the interaction term
is significantly positive, indicating that human capital expansion has a significant positive impact on the market pricing power of firms. A possible reason is that the increase in advanced human capital supply enables firms to employ more high-quality labor force. This high-quality labor force can help firms improve the quality of their products (Can & Gozgor, Citation2018; Schott, Citation2004) and improve the pricing level of their products thereafter.
Table 7. Influencing mechanism analysis
The following question must be answered: Does human capital expansion actually promote the improvement of the quality of labor employed by firms? I conduct further test in this regard. In general, firms should pay relatively higher wages to employ high-skilled and high-quality labor. Hence, the average wage can be used as an approximate measure of the quality of labor employed by firms. In column (3) of , I further use the logarithm of firms’ average wage as the dependent variable and regress it on the interaction term . The estimated results show that human capital expansion significantly improves the average wage of firms, which is consistent with our expectation: an increase in advanced human capital supply improves the average quality of labor employed by firms. In the case of the same production cost, an increase of product pricing is conducive to improving firms’ markup. Hence, human capital expansion improves firms’ markup through the market pricing channel.
In column (2) of , the dependent variable is firms’ TFP. The estimation results show that human capital expansion is conducive to the improvement of firms’ production efficiency, which is consistent with the conclusion of Che and Zhang (Citation2018). Higher production efficiency means lower marginal cost of production, which is conducive to the improvement of firms’ markup. Therefore, human capital expansion also improves firms’ markup through the production efficiency channel.
In addition, we are markedly concerned with how human capital expansion affects the product price and production efficiency of firms. Human capital expansion is proven advantageous to improving the average quality of labor employed by firms. Che and Zhang (Citation2018) further explain that the massive increase in university-educated labor force can be beneficial for firms to carry out various innovative activities. For example, the participation of high-quality labor force helps firms to carry out more R&D activities, which is conducive to improving the production process and management efficiency, thereby improving existing products and creating new products. Therefore, promoting the development of firm innovation activities is likely an important way for human capital expansion to affect firm pricing power and production efficiency. For verification, I take the output value of new products, R&D investment, and number of patent applications of firms as dependent variables and regress them on the interaction term . The estimation results are reported in columns (4) to (6) of . First, human capital expansion significantly improves the output value of new products, indicating that human capital expansion is definitely conducive to the development of new products. Second, human capital expansion significantly promotes an increase in R&D investment. That is, from the perspective of innovation investment, human capital expansion is certainly beneficial for firms to implement additional R&D and innovation activities. Lastly, human capital expansion significantly improves the number of patent applications. The number of patent applications can substantially measure the innovation output of firms (Kong et al., Citation2020). From the actual innovation results, human capital expansion is conducive to the improvement of firm innovation output.
According to the regression results in , we generally find that human capital expansion is conducive to the development of firm innovation activities. Moreover, the production efficiency and market pricing channels are important for human capital expansion to improve firms’ markup.
5. Heterogeneous effects of human capital expansion on the markup of different types of firms
5.1. Analysis of different financing constraint types
Since the reform and opening up, the improper and excessive intervention of government in the financial system during the transformation from planned economy to market economy has resulted in Chinese characteristic financing constraints. Numerous Chinese firms face different degrees of financing constraints. Owing to the limitation of liquidity, firms facing financing constraints may be unable to fully enjoy the positive impact of the substantial increase in advanced human capital supply. Significant differences may be noted in the impact of human capital expansion on the markup of firms with different financing constraints. For verification, I follow Hadlock and Pierce (Citation2010) and first calculate the SA index to measure the degree of financing constraints faced by firms.Footnote3 Thereafter, I take the median of the SA index in the sample as the critical value to divide the sample into two types: firms with (1) high and (2) low financing constraints. I use the two types of subsamples to re-estimate EquationEquation (1)(1)
(1) .
The results in columns (1) and (2) of show that the estimated coefficient of the interaction term in the subsample of firms with low financing constraints is significantly positive and high (0.0306). The estimated coefficient in the subsample of firms with high financing constraints is low (0.0179) and only significant at the 10% level. These results indicate that human capital expansion has a heterogeneous effect on the markup of firms with different financing constraints. That is, the positive impact of human capital expansion on the markup of firms with low financing constraints is large. A possible reason is that as high-quality and highly skilled college graduates need to be paid relatively higher wages, if firms are faced with more serious financing constraints, then they may lack sufficient funds to employ enough highly skilled college graduates. Moreover, the promotion effect of human capital expansion on the markup of such firms is relatively limited.
Table 8. Heterogeneity analysis
5.2. Analysis of different scale types
Given that firms of varying sizes have considerable differences in production efficiency, attractiveness to senior talents, and market monopoly power, the impact of human capital expansion on the markup of firms with different sizes may also vary greatly. For verification, I take the median of firm size in the sample as the critical value to divide the sample into large- and small-scale firms and re-estimate EquationEquation (1)(1)
(1) . The results in columns (3) and (4) of show that human capital expansion mainly promotes the markup of small-scale firms and does not have a significant impact on the markup of large-scale firms. The reason may be that large-scale firms often have more standardized and mature management, are stronger, and with clearer development prospects. Moreover, the strong attraction of large-scale firms to high-quality human capital makes small-scale firms often in a state of talent shortage in the case of relatively insufficient supply of high-quality human capital. In general, the rapid increase in high-quality human capital supply brought by the implementation of the university enrollment expansion policy can address the shortage of high-quality labor force faced by small-scale firms as well as significantly improve their markup. Given that large-scale firms can employ many high-quality labor force, the impact of human capital expansion brought by the implementation of the university enrollment expansion policy on large-scale firms is not evident.
5.3. Analysis of export and non-export firms
The classic heterogeneous firm trade theory posits that firms first serve the domestic market, and only firms with high productivity choose to simultaneously serve this market and export (Melitz, Citation2003). Therefore, export firms have higher productivity than non-export firms. The existing literature on export premium (e.g., Bernard, Jensen, Redding, & Schott, Citation2007) shows that export firms are more human capital intensive, which to an extent shows that export firms need to hire more high-quality human capital than non-export firms. The increase in the supply of advanced human capital brought by the expansion of university enrollment is relatively more conducive to alleviating the human capital constraints faced by export firms. Thus, we expect that human capital expansion may have a greater effect on the markup of export firms compared with non-export firms. For verification, I divide all sample firms into non-export and export firms. Columns (1) and (2) of present the estimation results of EquationEquation (1)(1)
(1) by using the subsamples of non-export and export firms. For non-export firms, the estimated coefficient of the interaction term is significantly positive, but that for export firms is not significant. This result shows that human capital expansion only promotes the markup of non-export firms and has no significant effect on that of export firms. This outcome is inconsistent with our previous expectations.
Table 9. Heterogeneity analysis
The following question must also be answered: Why does human capital expansion insignificantly affect the markup of export firms? We are convinced that the reason may be related to the existence of numerous processing trade firms among China’s export firms. Yu (Citation2015) explains that in 2000–2006, China’s processing trade firms accounted for over 50% of all trading firms in the country. Different from general trade, processing trade firms mainly import raw materials or intermediate products and export after processing in their own countries to earn processing fees. Given that processing trade firms only need to engage in simple processing and assembly, they do not need to employ high-quality labor, which may make processing trade firms insensitive to the shock of advanced human capital supply after the implementation of the university enrollment expansion policy. To verify this scenario,I further match the ASIF database with the Customs Trade Statistics database and divide all export firms into general and processing trade firms according to their trade modes to re-estimate EquationEquation (1)(1)
(1) . The results in columns (3) and (4) of show that human capital expansion significantly promotes the markup of general trade firms but does not have a significant impact on the markup of processing trade firms. This result is consistent with our conjecture. We compare the estimated results in columns (1) and (3) and find that the promoting effect of human capital expansion on the markup of general trade firms is larger than that on the markup of non-export firms. This result further confirms our conjecture that the reason human capital expansion does not significantly affect the markup of export firms is the existence of numerous processing trade firms.
5.4. Analysis of different age types
Younger firms are less likely to survive in the market competition compared with older firms. However, conditional on surviving, Ouimet and Zarutskie (Citation2014) determine that surviving young firms exhibit a higher average growth rate because they attract more young workers who possess current technological skills owing to more recent education and are more risk-tolerant to take on high-risk but promising projects within firms. That is, young workers tend to be willing to join young firms. By developing new products or innovative production technologies, young workers can play an important role in young firms. College graduates who have just entered the labor market, as typical representatives of high-quality young labor force, are inclined to join young firms. The university enrollment expansion policy provides more educated young labor force for young firms. Therefore, we expect young firms to be considerably affected by human capital expansion. To verify this expectation, I take the median of firm age in the sample as the critical value to divide the sample into old and young firms and re-estimate EquationEquation (1)(1)
(1) . The results in columns (1) and (2) of show that although human capital expansion significantly promotes the markup of old and young firms, the positive effect of human capital expansion on the markup of young firms is greater than that on old firms. This result is consistent with our expectations.
Table 10. Heterogeneity analysis
5.5. Analysis of different ownership types
At present, China is in the transition period of a market economy, and firms of different ownership types have evident differences in production and operation. For example, SOEs enjoy better preferential treatment in terms of government policy support and bank credit support compared with private firms. China’s unique institutional setting makes the ownership structure an important factor affecting the performance of Chinese firms (Hu & Liu, Citation2014). When faced with the shock of human capital supply after the implementation of the university enrollment expansion policy, the reaction of firms of different ownership types may be relatively different. To analyze whether a difference exists in the impact of human capital expansion on the markup of firms with different ownership types, I divide all firms into SOEs and non-SOEs according to the proportion of registered capital and re-estimate EquationEquation (1)(1)
(1) .
The results in columns (3) and (4) of show that human capital expansion significantly promotes the markup of SOEs and non-SOEs. However, the positive effect of human capital expansion on the markup of SOEs is greater than that on non-SOEs. The possible main reasons are as follows. On the one hand, SOEs can often obtain financial support easily from the financial system dominated by state-owned banks. In addition, as the implicit guarantee of national credit for their repayment ability, SOEs can obtain sufficient and relatively low-cost financial support and will typically not suffer from financing constraints. Therefore, SOEs can employ numerous highly skilled college graduates after the implementation of the university enrollment expansion policy. By contrast, non-SOEs often suffer from financing constraints. When faced with the shock of human capital supply, non-SOEs may lack sufficient funds to hire ample high-quality college graduates, and the promoting effect of human capital expansion on the markup of non-SOEs is relatively small. On the other hand, college graduates in China’s labor market prefer to join SOEs owing to the stability of work and better welfare treatment in these enterprises. Therefore, SOEs can attract more high-quality human capital after the university enrollment expansion policy, which also explains why the positive impact of human capital expansion on the markup of SOEs is relatively greater than that on non-SOEs.
6. Conclusions
The university enrollment expansion policy implemented by the Chinese government in 1999 has led to a substantial increase in the supply of advanced human capital represented by college graduates from2003 onward. I take the shock of the rapid increase in the supply of college graduates in 2003 as a quasi-natural experiment and use the DID method to deeply analyze the effects of human capital expansion on firms’ markup and the influencing mechanism. The findings are as follows. First, human capital expansion significantly improves the markup of Chinese manufacturing firms, which remains true after a series of robustness tests, such as substitution of key indicators, triple difference model estimation, controlling for other policy changes, and quantile regression.
Second, production efficiency and market pricing channels are important for human capital expansion to improve firms’ markup. On the one hand, human capital expansion promotes the improvement of firms’ production efficiency and enhances their markup (production efficiency channel) thereafter. On the other hand, human capital expansion improves the market pricing power of firms and exerts a significant positive impact on their markup (market pricing channel). Human capital expansion mainly affects the pricing power and production efficiency of firms by promoting the development of firm innovation activities. I find that human capital expansion significantly improves the quality of labor force employed by firms, promotes R&D investment and the development of new products, and improves the innovation output (measured by the number of patent applications).
Third, considerable heterogeneity is observed in the impact of human capital expansion on different types of firms. (1) The promotion effect of human capital expansion on the markup of firms with low financing constraints is greater than that of firms with high financing constraints. (2) Human capital expansion mainly promotes the markup of small-scale firms and does not have a significant impact on that of large-scale firms. (3) Human capital expansion significantly promotes the markup of non-export firms and does not have a significant impact on the markup of export firms owing to the existence of numerous processing trade export enterprises. (4) The promoting effect of human capital expansion on the markup of young firms is greater than that of old firms. (5) The positive impact of human capital expansion on the markup of SOEs is greater than that of non-SOEs.
This study provides a new perspective for understanding the microeconomic effects of human capital expansion. It also has important policy implications. That is, human capital expansion is conducive to the improvement of firms’ markup. Therefore, the government should continue to attach importance to and promote the development of higher education. Although the Chinese government has exerted immense effort to improve the university admission rate for many years and China’s higher education has changed from elite to mass education, a large gap remains when compared with developed countries in terms of enrollment scale and quality of education. Although mass education means no screening to select the most talented students, it facilitates the transfer of human capital to more students and leads to more skilled labor in the market. From the perspective of promoting firms’ markup, accelerating the popularization of higher education and improving the level of human capital are the key tasks that the government should continuously promote.
Disclosure statement
No potential conflict of interest was reported by the author(s).
Additional information
Funding
Notes
1 For additional information on China’s university enrollment expansion policy in 1999, please refer to Che and Zhang (Citation2018), Rong and Wu (Citation2020) and Kong et al. (Citation2020).
2 See Appendix D for more robustness tests.
3 Following Hadlock and Pierce (Citation2010), we calculate the SA index as follows: , where S and A represent the size and age of firms, respectively. The SA index is negative, and the larger its absolute value is, the more serious financial constraints the firm is facing.
4 “985” university originated from 4 May 1998. At the meeting celebrating the 100th anniversary of the founding of Peking University, President Jiang Zemin made the following declaration to the entire society on behalf of the Communist Party of China and Central People’s Government of the People’s Republic of China: “in order to realize modernization, China needs to have a number of first-class universities with world advanced level.” In 1999, the State Council approved the “action plan for the revitalization of education in the 21st century” of the Ministry of Education, and the “985 Project” was officially launched. Meanwhile, “211” university, also known as the “211 project,” is a key construction project in the field of higher education initiated by Chinese government. It refers to the construction project of approximately 100 universities and several key disciplines facing the 21st century. It was officially launched in November 1995 with the approval of the State Council.
References
- Ackerberg, D. A., Caves, K., & Frazer, G. (2015). Identification properties of recent production function estimators[J]. Econometrica, 83(6), 2411–2451.
- Altomonte, C., Favoino, D., Morlacco, M., & Sonno, T.). Markups, intangible capital and heterogeneous financial frictions[J] (Working Paper 2 2 2022). Retrieved from http://www.tommasosonno.com/docs/MarkupsIntangibles_AFMS.pdf
- Ashraf, R., & Ray, R. (2017). Human capital, skilled immigrants, and innovation[R] (SSRN Working Paper).
- Barro, R. J. (1991). Economic growth in a cross section of countries[J]. The Quarterly Journal of Economics, 106(2), 407–443.
- Bellone, F., Musso, P., Nesta, L., & Warzynski, F. (2016). International trade and firm-level markups when location and quality matter[J]. Journal of Economic Geography, 16(1), 67–91.
- Benhabib, J., & Spiegel, M. M. (2005). Human capital and technology diffusion[M]. In P. Aghion & S. N. Durlauf (Eds.), Handbook of economic growth (pp. 936–966). Amsterdam: North Holland.
- Bernard, A. B., Eaton, J., Jensen, J. B., & Kortum, S. (2003). Plants and productivity in international trade[J]. American Economic Review, 93(4), 1268–1290.
- Bernard, A. B, Jensen, J. B., Redding, S. J., & Schott, P. K. (2007). Firms in international trade[J]. Journal of Economic Perspectives, 21(3), 105–130.
- Bertrand, M., Duflo, E., & Mullainathan, S. (2004). How much should we trust differences-in-differences estimates?[J]. The Quarterly Journal of Economics, 119(1), 249–275.
- Brandt, L., Van Biesebroeck, J., & Zhang, Y. (2012). Creative accounting or creative destruction? Firm-level productivity growth in Chinese manufacturing[J]. Journal of Development Economics, 97(2), 339–351.
- Can, M., & Gozgor, G. (2018). Effects of export product diversification on quality upgrading: An empirical study[J]. The Journal of International Trade & Economic Development, 27(3), 293–313.
- Cao Y.J. and Mao Q.L.(2019). How does human capital affect Chinese manufacturing firms’ markups? Evidence from China’s higher education reform[J]. Journal of Finance and Economics, 2019, 45(12):138–150.
- Caselli, M., & Markups, S. S. (2020). Import competition and exporting[J]. World Economy 43 (5) , 1–18.
- Che, Y., & Zhang, L. (2018). Human capital, technology adoption and firm performance: Impacts of China’s higher education expansion in the late 1990s[J]. The Economic Journal, 128(614), 2282–2320.
- Ciccone, A., & Papaioannou, E. (2009). Human capital, the structure of production, and growth[J]. The Review of Economics and Statistics, 91(1), 66–82.
- De Loecker, J., Goldberg, P. K., Khandelwal, A. K., & Pavcnik, N. (2016). Prices, markups, and trade reform[J]. Econometrica, 84(2), 445–510.
- De Loecker, J., & Warzynski, F. (2012). Markups and firm-level export status[J]. American Economic Review, 102(6), 2437–2471.
- Domowitz, I., Hubbard, R. G., & Petersen, B. C. (1986). Market structure and cyclical fluctuations in US manufacturing[R] (National Bureau of Economic Research Working Paper, No. 2115).
- Du, X., Luan, W., & Lu, Y. (2020). Impact of economic policy uncertainty and financial constraints on firms’ markups: Evidence from China[J]. Applied Economics Letters. forthcoming. doi:10.1080/13504851.2020.1854665.
- Du, P., & Wang, S. (2020). The effect of minimum wage on firm markup: Evidence from China[J]. Economic Modelling, 86, 241–250.
- Edmond, C., Midrigan, V., & Xu, D. Y. (2015). Competition, markups, and the gains from international trade[J]. American Economic Review, 105(10), 3183–3221.
- Fan, H., Gao, X., Li, Y. A., & Luong, T. A. (2018). Trade liberalization and markups: Micro evidence from China[J]. Journal of Comparative Economics, 46(1), 103–130.
- Feenstra, R. C., Li, Z., & Yu, M. J. (2014). Exports and credit constraints under incomplete information: Theory and evidence from China[J]. Review of Economics and Statistics, 96(4), 729–744.
- Garcia-Marin, A., & Voigtländer, N. (2019). Exporting and plant-level efficiency gains: It’s in the measure[J]. Journal of Political Economy, 127(4), 1777–1825.
- Görg, H., & Warzynski, F. (2003). Price cost margins and exporting behaviour: Evidence from firm level data[R]. Discussion Papers of DIW No. 365. German Institute for Economic Research.
- Hadlock, C. J., & Pierce, J. R. (2010). New evidence on measuring financial constraints: Moving beyond the KZ index[J]. The Review of Financial Studies, 23(5), 1909–1940.
- Hanushek, E. A., & Woessmann, L. (2012). Do better schools lead to more growth? Cognitive skills, economic outcomes, and causation[J]. Journal of Economic Growth, 17(4), 267–321.
- Hu, A. G., & Liu, Z. (2014). Trade liberalization and firm productivity: Evidence from Chinese manufacturing industries[J]. Review of International Economics, 22(3), 488–512.
- Kato, A. (). Does export yield productivity and markup premiums? Evidence from the Japanese manufacturing industry[R]. Research Institute of Economy, Trade and Industry (RIETI), Discussion Paper Series 14-E-037 Accessed2 2 2022 https://citeseerx.ist.psu.edu/viewdoc/download?doi=10.1.1.639.269&rep=rep1&type=pdf .
- Kilinç, U. (2019). Export destination characteristics and markups: The role of country size[J]. Economica, 86(341), 116–138.
- Koenker, R., & Bassett, G., Jr. (1978). Regression quantiles[J]. Econometrica, 46(1), 33–50.
- Kong, D., Zhang, B., & Zhang, J. (2020). Higher education and corporate innovation[R] (SSRN Working Paper). Retrieved from https://papers.ssrn.com/sol3/papers.cfm?abstract_id=3636621
- Kugler, M., & Verhoogen, E. (2008). The quality-complementarity hypothesis: Theory and evidence from Colombia[R] (NBER Working Paper, 2008, No. w14418).
- Liu, Q., & Qiu, L. D. (2016). Intermediate input imports and innovations: Evidence from Chinese firms’ patent filings[J]. Journal of International Economics, 103, 166–183.
- Lu, Y., & Yu, L. (2015). Trade liberalization and markup dispersion: Evidence from China’s WTO accession[J]. American Economic Journal: Applied Economics, 7(4), 221–253.
- Madsen, J. B. (2014). Human capital and the world technology frontier[J]. Review of Economics and Statistics, 96(4), 676–692.
- Máñez, J. A., Rochina Barrachina, M. E., & Sanchis, J. A. (2020). The effects of export and R&D strategies on firms’ markups in downturns: The Spanish case[J]. Journal of Small Business Management 60 3 , 1–34.
- Manova, K., & Zhang, Z. (2009). Quality heterogeneity across firms and export destinations[R] (NBER Working Paper, No. 15342).
- Meinen, P. (2016, April). Markup responses to Chinese imports[J]. Economics Letters, 141, 122–124.
- Melitz, M. J. (2003). The impact of trade on intra-industry reallocations and aggregate industry productivity[J]. Econometrica, 71(6), 1695–1725.
- Melitz, M. J., & Ottaviano, G. I. P. (2008). Market size, trade, and productivity[J]. The Review of Economic Studies, 75(1), 295–316.
- Meng, X., Shen, K., & Xue, S. (2013). Economic reform, education expansion, and earnings inequality for urban males in China, 1988–2009[J]. Journal of Comparative Economics, 41(1), 227–244.
- Miller, S. M., & Upadhyay, M. P. (2000). The effects of openness, trade orientation, and human capital on total factor productivity[J]. Journal of Development Economics, 63(2), 399–423.
- Ouimet, P., & Zarutskie, R. (2014). Who works for startups? The relation between firm age, employee age, and growth[J]. Journal of Financial Economics, 112(3), 386–407.
- Peking University. (2011). Employment status of higher education graduates in China, 2009–2010 [J]. Beijing: Peking University Press.
- Romer, P. M. (1990). Endogenous technological change[J]. Journal of Political Economy, 98(5, Part 2), S71–S102.
- Rong, Z., & Wu, B. (2020). Scientific personnel reallocation and firm innovation: Evidence from ‘China’s college expansion[J]. Journal of Comparative Economics, 48(3), 709–728.
- Schott, P. K. (2004). Across-product versus within-product specialization in international trade[J]. The Quarterly Journal of Economics, 119(2), 647–678.
- Sun, X., Li, H., & Ghosal, V. (2020). Firm-level human capital and innovation: Evidence from China[J]. China Economic Review, 59, 101388.
- Weinberger, A. (2020). Markups and misallocation with evidence from exchange rate shocks[J]. Journal of Development Economics, 146, 102494. forthcoming.
- Xiang, X., Chen, F., Ho, C. Y., & Yue, W. (2017). Heterogeneous effects of trade liberalisation on firm‐level markups: Evidence from China[J]. The World Economy, 40(8), 1667–1686.
- Yu, M. (2015). Processing trade, tariff reductions and firm productivity: Evidence from Chinese firms[J]. The Economic Journal, 125(7), 943–988.
Appendix A.
The output elasticity of inputs
Table A1. Average output elasticity of inputs in each industry
Appendix B.
Additional estimation results of triple difference model
I also use the number of “985”Footnote4 universities and “211” universities owned by each province to measure prok to substantially measure the difference in human capital supply in each province after the university enrollment expansion policy. The reason for using this method is mainly based on two considerations. First, China has 39 “985” universities and 116 “211” universities, representing the highest levels of the country’s colleges and universities. Every year, graduates from “985” universities and “211” universities are considered the best among the university graduates of the year, and can better represent the supply of advanced human capital. If a province has more “985” universities and “211” universities, then the number of graduates from these universities will increase more with the implementation of the university enrollment expansion policy. Accordingly, the shock of advanced human capital supply induced by the policy will also be greater. Second, the “985” university and “211” university projects was started at the end of the 20th century, which was earlier than the implementation of the university enrollment expansion policy in 1999. Moreover, the list of “985” and “211” universities is relatively fixed. If universities enter the “985” or “211” list, they will hardly be removed from the list, thereby ensuring that the number of “985” and “211” universities in each province will be unaffected by the university enrollment expansion policy. Column (1) of shows the estimation results of EquationEquation (6)(6)
(6) when we use the number of “985” universities of each province to measure the difference in human capital supply in each province. The estimated coefficient of DDD_985 is significantly positive, indicating that the average difference in firms’ markup between high and low human capital-intensity industries before and after the expansion of human capital is greater in provinces with more “985” universities. Column (2) of presents the estimation results of EquationEquation (6)
(6)
(6) when we use the number of “211” universities of each province to measure the difference in human capital supply in each province. The estimated coefficient of DDD_211 is not significant but also positive.
Given the numerous types of universities in “985” and “211” universities, the impact of graduates from different types of universities on firm development varies. For example, science and engineering universities cultivate more college students in the natural science and engineering fields. After graduation, they are often the main force for firms to carry out R&D and innovation activities. They reduce the production cost of firms through R&D and innovation activities, which will have considerably direct impact on the firms’ markup. Finance and economics universities cultivate more human capital in economics, management, and law. These graduates may have an indirect impact on firms’ markup by improving the organizational management model and operational efficiency of these organizations. Therefore, I further use the number of science and engineering and comprehensive “985” and “211” universities of each province to measure the difference in human capital supply in each province after the university enrollment expansion policy. The results in columns (3) and (4) of show that the estimated coefficients of DDD_ 985_ SE and DDD_ 211_SE remain significantly positive, similar to previous estimation results.
Table B1. Results of the triple difference model
Appendix C.
Descriptive statistics of markups calculated based on the accounting method
Table C1. Average markups calculated by the accounting method for each industry
Appendix D.
More robustness tests
Using Chinese data to measure industry human capital intensity. The previous analysis is mainly based on the proportion of workers with at least four years of college education in various industries in the US in 1980 to measure the human capital intensity of different industries. In this section, as a robustness test, I also use the data from China Education Statistical Yearbook in 1995 and 2004 to re-measure the human capital intensity of various industries. The estimated results in columns (1) and (2) of show that the estimated coefficients of the interaction terms IHC_China1995 × post03 and IHC_China2004 × post03 remain significantly positive. This outcome indicates that human capital expansion significantly improves firms’ markup, which is similar to the previous benchmark regression results.
Controlling for industry time trend. Given that firms’ markup may be affected by some non-observing industry-specific factors, I follow Liu and Qiu (Citation2016) and further add the industry-specific linear time trend term to re-estimate EquationEquation (1)(1)
(1) to considerably control for the interference of non-observing industry-specific factors on previous regression results. The estimation results in column (3) of Table D1 show that the estimated coefficient of the interaction term
remains significantly positive. This result indicates that non-observing industry-specific factors does not have a substantial impact on previous estimation results.
Excluding firms in Shanghai and Beijing. Beijing and Shanghai have the best universities in China and also have the most number of graduates (Che & Zhang, Citation2018). Given their economic, political, and geographical advantages, Shanghai and Beijing can attract the most talented university graduates throughout the country. Therefore, an increase in high-quality human capital supply in these two cities may not be entirely the result of the implementation of the university enrollment expansion policy, which may interfere with previous estimation results. To address this concern, I exclude firms in Beijing and Shanghai and re-estimate EquationEquation (1)(1)
(1) . The estimation results in column (4) of show that human capital expansion improves firms’ markup, which is similar to previous regression results.
Controlling for the impact of firms’ entry and exit. During the sample period, many firms only appear one or two times in the sample. That is, firms have numerous entry and exit behaviors. Brandt, Van Biesebroeck, and Zhang (Citation2012) explained that the growth of TFP of Chinese manufacturing firms can be partially attributed to the entry and exit behaviors of firms. Given that the improvement of firm production efficiency is conducive to reducing the production cost, the entry and exit behaviors of firms likely have an impact on firms’ markup. To address this concern, I only retain firms that have existed for numerous years in the sample to construct the new sub-sample, and re-estimate EquationEquation (1)(1)
(1) . The estimation results in column (5) of are similar to previous benchmark regression results.
Table D1. Robustness test results