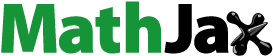
ABSTRACT
We estimate the impact of changes in non-performing loan (NPL) ratios on aggregate banking sector variables and the macroeconomy by estimating a panel Bayesian VAR model for twelve euro area countries. The main findings are as follows: i) An impulse response analysis shows that an exogenous increase in the change in NPL ratios tends to depress bank lending volumes, widens bank lending spreads and leads to a fall in real GDP growth and residential real estate prices; ii) A forecast error variance decomposition shows that shocks to the change in NPL ratios explain a relatively large share of the variance of the variables in the VAR, particularly for countries that experienced a large increase in NPL ratios during the recent crises; and iii) A three-year structural out-of-sample scenario analysis suggests that reducing banks’ NPL ratios can produce significant benefits in terms of improved macroeconomic and financial conditions.
1. Introduction
The non-performing loan (NPL) ratio in the euro area increased from around 3% at the onset of the global financial crisis in late 2008 to a peak of around 8% in 2014. A key driver of the substantial growth in NPL ratios was the severe and protracted recession in large parts of the euro area, which significantly reduced borrowers’ capacity to service their debt. At the same time, the fast increase in NPL ratios was also significantly influenced by other factors, such as banks’ lending and monitoring policies and limited capacity to work-out defaulted loans. More recently, the recovery of economic activity in the euro area and the development and implementation of policies to tackle non-performing loans by the Single Supervisory Mechanism (SSM) have led to a decline in the euro area NPL ratio, which reached around 6% at the end of 2017. The evolution of the NPL ratios has been rather heterogeneous across euro area countries reflecting the different macroeconomic conditions and diverse structural features (e.g., the efficiency of legal and judicial systems, insolvency frameworks, payment culture and the level of development of distressed debt markets, among others). At the end of 2017, the NPL ratio still remained above 10% in those euro area countries most affected by the recent economic and financial crisis, namely Cyprus, Greece, Ireland, Italy and Portugal, while it was below 5% in countries such as Austria, Belgium, Estonia, France, Lithuania and the Netherlands.
High NPL ratios in banks’ balance sheets can adversely affect the soundness of the banking system and its ability to lend to the real economy through three main channels. First, high non-performing loans reduce bank profits. They do so because they require higher provisions, they lead to lower interest income, generate higher expenses associated with their monitoring and management and lead to an increase in funding costs, as risk adverse investors are less willing to lend to institutions with a low credit quality.Footnote1 Second, non-performing loans feature higher risk weights, leading to higher capital needs. To maintain or boost capital adequacy, banks may thus deleverage, leading to a contraction in credit supply. Finally, the management of large NPL stocks can divert important managerial resources away from core and more profitable activities.Footnote2 Considering the importance of bank lending for the functioning of the euro area economy, there is a clear need to study the feedback loop between non-performing loans, bank credit and the real economy.
The empirical literature on NPLs features three main strands which investigates the determinants of NPLs, the impact of NPLs on the real economy and the feedback loops between NPLs and the macroeconomy, respectively.
The first strand of literature has identified three main groups of determinants of NPLs, namely bank level, industry-specific and macroeconomic. The first group includes: i) Exogenous factors, such as a sudden drop in economic activity (bad luck hypothesis); ii) Poor management, which can lead to bad credit risk decisions (bad management hypothesis); iii) Low capitalisation, which can make banks prone to risk taking (moral hazard hypothesis); and iv) Scarcity of resources allocated to underwriting and monitoring loans to boost short-term efficiency (skimping hypothesis). While the literature has found support for all these hypotheses, the bad management hypothesis is the most prominent one. Industry-specific drivers point mainly to the impact of competition on risk taking. On the one hand, stronger market power may drive lending rates higher, increasing the debt burden for borrowers and thus, their bankruptcy risk (competition-stability hypothesis). On the other hand, banks with more market power have higher franchise value and, therefore, more at stake in the event of defaults, making their underwriting more prudent (competition-fragility hypothesis). Overall, there seems to be no consensus in the literature on whether bank competition increases or decreases stability in the banking system (Beck, De Jonghe & Schepens, Citation2013; Goetz, Citation2018). Finally, regarding macroeconomic drivers, the literature has focused on various measures of economic activity, inflation, interest rates and the exchange rate as the most relevant drivers of NPLs (Anastasiou & Tsionas, Citation2016; Jiménez & Saurina, Citation2006; Louzis, Vouldis & Metaxas, Citation2012). Improved economic conditions, higher inflation and lower interest rates are found to strengthen the repayment capacity of borrowers, while exchange rate depreciations are shown to increase the debt burden of foreign-exchange denominated loans for unhedged borrowers.Footnote3
The second main strand of the literature studies the impact of non-performing loans on bank lending and economic activity. This literature relied both on bank-level and country-level data. For example, Balgova & Plekhanov (Citation2016), using data for a global sample of 100 countries, quantified the (positive) effects of policy-induced declines in NPLs on the real economy. The authors find that the foregone growth due to the overhang of NPLs can be large. Accornero, Alessandri, Carpinelli & Sorrentino (Citation2017), coupling bank-level data for Italy with borrower-based information for non-financial corporations, study the influence of NPLs on the supply of bank credit. They find that bank lending is impaired by the exogenous accumulation of new NPLs and the associated increase in provisions, but it is not causally affected by the level of NPL ratios.
The literature on the determinants of non-performing loans and on the impact of non-performing loans on bank lending and the real economy has traditionally relied on single equation estimation techniques, where either NPLs or macroeconomic variables are regressed against each other and other control variables. By modelling the dynamics of each variable separately, these studies neglect the dynamic interaction and feedbacks between the changes in non-performing loans, banking and macroeconomic variables. This is a major drawback, because an exogenous increase in NPLs is likely to impair economic activity, leading to a decline in the repayment capacity of borrowers and a further increase in NPLs. As a result, a third strand of literature has estimated the impact of shocks to NPLs using structural time series models where aggregate NPL ratios and economic activity are included in a VAR together with a broader set of banking and macroeconomic variables. For example, Espinoza & Prasad (Citation2010), ECB (Citation2011), De Bock &Demyanets (Citation2012) and Klein (Citation2013) estimate panel VAR models for various groups of countries and use country-level data to investigate the feedback interactions between NPLs and macroeconomic performance.Footnote4 In addition to the expected countercyclical behaviour of NPLs, all these studies find significant feedback effects from NPLs to the real economy.
We contribute to the empirical literature on the feedback effects between NPLs, the banking sector and the macroeconomy by estimating a panel Bayesian VAR model with hierarchical priors (Jarociński, Citation2010). The aim of our analysis is to estimate the impact of exogenous shocks to the change in NPL ratios on bank lending and the macroeconomy. Estimations are performed over the period from the first quarter of 2006 to the third quarter of 2017 for twelve euro area countries, namely Austria, Belgium, Cyprus, Estonia, France, Greece, Ireland, Italy, Lithuania, Netherlands, Portugal and Spain.
The variables included in the panel VAR are economic activity (which is a proxy for the repayment capacity of borrowers), inflation, the monetary policy rate, real estate prices, bank lending volumes both to non-financial corporations and to households for house purchase, bank lending spreads to these two sectors, the ratio of capital and reserves over total assets and the change in NPL ratios. In order to disentangle the exogenous shocks to the changes in the NPL ratio, we use the Choleski factorisation, a recursive technique largely disseminated by Christiano, Eichenbaum & Evans (Citation1999) and commonly adopted in the literature. Changes in NPL ratios which are unrelated to changes in the repayment capacity of borrowers (i.e., exogenous changes in NPL ratios) include, inter alia, sales of defaulted loans to investors, changes in banks’ own attitudes towards risk, write-offs, supervisory actions that incentivise banks to work out these loans (by offering restructuring solutions to clients) and other policy initiatives which deal with NPLs’ work-outs and defaults associated with poor enforcement mechanisms.
We find that an exogenous increase in the change in NPL ratios tends to depress bank lending, widens lending spreads and leads to a fall in real GDP growth and residential real estate prices. As a consequence, monetary policy rate is eased. While the responses of the capital and reserves-to-asset ratio vary across countries, a material increase is recorded in Cyprus, Spain, Ireland, Italy, Lithuania and Portugal, due to the increase in provisions for impairments recorded during the crisis. Interestingly, the results show that the decline in bank lending to non-financial corporations is generally more marked than that in mortgage loans. These results are robust to a change in the ordering of the variables in the Choleski factorisation and also when including in the VAR the annual rate of growth in NPL volumes rather annual changes in NPL ratios. The forecast error variance decomposition also shows that exogenous shocks to the change in NPL ratios explain a relatively large share of the variance of the variables in the VAR, particularly for countries that exhibited a large increase in NPL ratios during the crisis. Finally, a three-year structural out-of-sample scenario analysis assesses the impact of a decline in NPL ratios for Cyprus, Ireland, Spain, Italy, Greece and Portugal, i.e., the countries that exhibited the most sizable increase in NPL ratios during the crisis. More specifically, it quantifies the differential impact of a scenario where NPL ratios remain constant versus one where they are assumed to decline in line with observed recent developments. The exercise shows that reducing NPL ratios can produce non-negligible benefits in terms of improved macroeconomic and financial conditions.
Against this background, the contribution of this work to the literature is threefold. To our knowledge, this paper is the first which studies the impact of a shock to the change in NPL ratios using a panel Bayesian VAR model which allows for country-specific coefficients, hence capturing country-specific dynamics for a large group of euro area countries with a consistent approach. This is an important contribution, given that euro area countries experienced rather heterogeneous dynamics of the NPL ratios in the considered time period due to different economic developments as well as key structural and institutional features. At the same time, the model assumes that the parameters of the VAR for individual countries share a common component which is compatible with the fact that euro area countries are part of a common market and share a common monetary policy. This assumption ensures an efficient use of the data. Second, this empirical analysis, thanks to the use of a Bayesian approach which allows to estimate a large number of parameters despite the relatively short time-span of the available data series for NPL ratios, benefits from the inclusion in the VAR of a larger set of variables than those typically used in the literature. The use of a richer VAR allows to better characterize the feedback loop between non-performing loans, the real economy and the banking sector. In particular, the inclusion in the VAR of the capital and reserves to total asset ratio and lending spreads and the distinction between lending and spreads to non-financial corporations and to households for house purchases are a novelty. Including the capital and reserves to total assets ratio is important because shocks to NPL ratios affect capital and provisions for impairments. Also, having bank lending spreads among the endogenous variables is valuable because the exogenous shocks might lead to a re-pricing of bank loans, hence affecting the quantity of loans provided to the economy and, thus, macroeconomic conditions. Finally, this paper is the first study which constructs and relies on a balanced panel of quarterly time series of NPL ratios for almost 13 years and for a large number of euro area countries.
The rest of the paper is structured as follows. Section 2 presents the empirical methodology, including the econometric model and the priors adopted. The variables included in the panel VAR and the identification scheme are presented in Section 3 and 4, respectively. Section 5 presents three sets of results: the impulse response analysis, the forecast error variance decomposition, the robustness analysis and the out of sample structural counterfactual analysis. The last section concludes.
2. Empirical methodology
We estimate a panel VAR model for twelve euro area countries and ten variables for the period between the first quarter of 2006 and the third quarter of 2017.Footnote5 The twelve countries included in the analysis are Austria, Belgium, Cyprus, Estonia, France, Greece, Ireland, Italy, Lithuania, Spain, the Netherlands and Portugal. This is the largest number of countries for which we could construct quarterly data on non-performing loans (NPLs) over the longest possible period. The model allows for cross-subsectional heterogeneity, hence capturing country-specific dynamics. The following subsections describe in detail the methodology used to estimate the panel VAR, the variables included in the model and the adopted identification scheme.
2.1. Econometric model
We estimate the impact of shocks to the change in NPL ratios on bank lending and the economy based on the following panel model:
Where is an individual country (
),
is time (
),
is a column vector of
endogenous variables (10 endogenous variables at time
are included in the model),
is a vector of
constants which are country specific and
are matrices of coefficients for different order of lags until lag
. We employ two lags in the model, due to the short sample. Canova (Citation2005) employs only one lag when estimating a Bayesian VAR with slightly more than 10 years of quarterly data and five variables. Experimenting with a longer lag length resulted in a decline in the quality of the estimations. The model we estimate allows for country-specific coefficients, allowing us to capture the different impacts of the shocks across countries. Finally, it is assumed that the error term is normally distributed, as follows:
Transposing EquationEquation (1)(1)
(1) and expressing it in compact form, one obtains:
Stacking EquationEquation (3)(3)
(3) over the
periods it follows:
Which can be expressed as:
In turn, Equationequation (5)(5)
(5) can be vectorised as follows:
Calling and from EquationEquation (2)
(2)
(2) it follows that:
Moreover, calling , the random coefficient model estimated here assumes that
can be expressed as:
With a
vector of parameters and assuming that
, it follows that:
EquationEquations (8)(8)
(8) and (Equation9
(9)
(9) ) imply that the coefficients in the VAR will differ across countries while being drawn from the same distribution, centered around a common mean for the euro area (hence capturing similarity across country’s coefficients). This model is particularly appealing because it captures a common component across countries while allowing for cross-country heterogeneity in the response to shocks. This feature is useful in our context because of the different dynamics exhibited by the NPL ratios in the countries included in our sample. The next sub-section describes the priors used in the paper.
2.2. The priors
The hierarchical prior adopted in this paper follows Jarociński (Citation2010).Footnote6 The advantage of this prior is that it treats the set of vectors (
), the residual covariance matrices
(
), and the common mean and variance of the VAR coefficients
and
as random variables. In particular, the hyperparameters
and
will have a hyper-prior distribution.
The hyper-prior distribution proposed by Jarociński (Citation2010) is diffuse (improper) for , whereas for
the functional form relies on a diagonal matrix
of dimension
,
, which is inspired by the specification of the variance matrix of the Minnesota prior. In particular, for parameters
relating endogenous variables to their own lags, the variance is given by
, whereas for parameters related to cross-lag coefficients the variance is defined as
. Because some coefficients are large, while others are small, it is necessary to scale each coefficient’s variance by a factor which adjusts the size of the coefficients of variables
and
. The values for
and
are obtained by fitting autoregressive models by OLS for the
endogenous variables of the model (after pooling the data for all units) and then their standard deviation is computed. These standard errors capture the scale of unexpected movements in the variables. The full covariance matrix is then defined as
. The parameter
captures the overall tightness of the prior for
. Note that when
the prior variance is null and all the coefficients in
will take the value
(full pooling of the data across countries). By contrast, when
grows larger, coefficients differ more and more across the countries in the sample and become similar to the respective single country estimates. When
the coefficients for each country are their own individual estimates and there is no sharing of information across countries. Because the number of estimated coefficients in the dynamic equations differs substantially in the two cases (
in the pooled panel and
in the hierarchical model), it is desirable to assume an intermediate value for
to ensure a reasonable balance between fitting individual countries’ data, on the one hand, and constraining the specification to make the estimates tighter, on the other. Hence, in order for the model to allow some degree of information sharing, Jarociński (Citation2010) proposes as prior for
an inverse Gamma distribution with very small values for the shape and scale parameters. Small parameters for the inverse Gamma distribution make the prior weakly informative, letting the data talk about the posterior common mean and variance.Footnote7 The values considered for the other two hyperparameters are those typically assumed in the literature, namely
and
. Finally, the prior distribution for
is simply a diffuse prior. Combining the likelihood function with the priors mentioned in this sub-section one can obtain the full posterior distribution. However, this distribution does not allow for analytical derivation of the marginal posteriors, hence requiring the use of numerical methods.
3. Variables included in the panel VAR
The panel VAR includes 10 variables, which is a larger set than those typically used in the literature. This allows us to better characterise the dynamic interaction and feedback loops between non-performing loans, the real economy and the banking sector.Footnote8 In particular, the variables included are economic activity (which is a proxy for the repayment capacity of borrowers), inflation, the monetary policy rate, real estate prices, bank lending volumes both to non-financial corporations and to households for house purchase, bank lending spreads to these two sectors, the ratio of capital and reserves over total assets and the change in NPL ratios. and provide information on data sources and summary statistics, respectively.
Table 1. Data sources.
Table 2. Summary statistics.
Economic activity is measured by the annual rate of growth of real GDP (adjusted for calendar and seasonal effects). In the case of Ireland, economic growth is computed as the annual growth rate of the nominal modified Gross National Income (GNI*), deflated using the deflator of the modified domestic demand (MDD).Footnote9 Inflation is defined as the annual rate of growth in the Harmonised Index of Consumer Prices (HICP) (working day and seasonally adjusted). The source of these data is Eurostat and the Irish Central Statistics Office. The average over daily observations of the three-month Euribor rate is used as a proxy for the policy interest rate. The source of the data is the ECB Statistical Data Warehouse (SDW).
Bank lending is defined as the annual rate of growth in bank lending to non-financial corporations and to households for house purchase. Originally, these two variables are defined in terms of an index of notional stocks.Footnote10 The source of these series is the MFI Balance Sheet Statistics of the ECB.Footnote11
Including bank lending spreads among the endogenous variables in the VAR is important because the exogenous shocks might lead to a re-pricing of bank loans, hence affecting the quantity of loans provided to the economy and macroeconomic conditions. In the particular case of mortgage spreads, they may affect the business cycle via changes in house prices, housing wealth and collateral valuations (Walentin, Citation2014). The bank lending spreads are defined as the difference between bank lending rates (to households for house purchase and to non-financial corporations) and Euribor. The lending rates used to compute the spreads are the interest rates on new business loans granted in euros, all maturities combined.Footnote12 The source is the MFI Interest Rate Statistics of the ECB.
The series of residential real estate prices is included to account for the role that real estate markets play in business cycle fluctuations. This sector matters because it is a sizable sector of the real economy and firms and households own real estate properties, often used as collateral. Moreover, real estate transactions usually require credit, which is often provided by leveraged lenders. If borrowers default, the effects can be further amplified through a deleveraging process of the latter. As a consequence, changes in real estate prices can have large real effects and welfare implications (Hartmann, Citation2015). Other studies that have included house prices in a VAR framework similar to ours include Bjørnland & Jacobsen (Citation2010), Iacoviello (Citation2005) and Meeks (Citation2017). The residential real estate prices used in this analysis refer to new and existing dwellings for the whole country and are computed as the annual growth rate of the underlying index. The source of the data is the ECB SDW.
The ratio of bank capital and reserves over total assets is also included in the VAR. As with bank lending, this variable is defined in terms of an index of notional stocks and the source is MFI Balance Sheet Statistics of the ECB. Capital and reserves (the numerator) include total equity capital, non-distributed benefits or funds and specific or general provisions against loans, securities and other types of assets. The capital and reserves to assets ratio is then computed as the ratio between this series and total assets.Footnote13 Including the capital and reserves ratio is important because institutions with larger buffers are better prepared to support lending.
Finally, we include in the VAR the change in NPL ratios which is the most relevant variable in our analysis and is defined as the yearly difference in NPL ratios. NPL ratios are defined as non-performing loans divided by total gross loans and were computed relying on several sources. The main source was the IMF Financial Soundness Indicators (FSI) database. This database provides data on the financial health and soundness of member countries’ financial systems since 2001. The IMF has offered guidelines to the member countries in order to improve the cross-country comparability of the data. In particular, it recommends that loans have to be classified as non-performing especially when: i) Payments of the principal and interest are past due by one quarter (90 days) or more; or ii) The interest payments equal to one quarter (90 days) interest or more have been capitalized (reinvested into the principal amount), refinanced, or rolled over (that is, payment has been delayed by agreement). This guideline is based on the observation that 90 days is the horizon that is most widely used by countries to determine whether a loan is non-performing (IMF, Citation2006).
The effective period covered by the FSI database varies across variables and countries. For most of the countries, data on NPLs dates back to the start of the global financial crisis (2008 and 2009). In most cases, these series were extended backwards until the first quarter of 2006 by using bank-level information extracted from Bankscope. In particular, the weighted average of bank-specific NPL ratios (using banks’ assets as weights) was used to construct the system-wide figure for each quarter for Austria, Belgium, Estonia, Greece, Ireland, Lithuania and Portugal.
For Cyprus, France and Spain, data provided by the national central banks were used as well. In particular, the FSI NPL ratio data for Cyprus were extended backwards relying on data kindly provided by the Central Bank of Cyprus for the period between the fourth quarter of 2005 and the third quarter of 2011. For the first three quarters of 2005, bank-level information was used instead. In the case of France, the NPL ratio has been calculated by dividing the series of “Créances douteuses brutes” (available from the website of the Banque de France) and the stock of loans to the non-financial private sector (sourced from the ECB SDW). Finally, for Spain, data provided by the national central bank were used to extend the FSI series before the fourth quarter of 2013.
The series of NPL ratios per country are displayed in . As already mentioned, this is the largest number of countries for which we could construct quarterly data on non-performing loans (NPLs) over the longest possible period. It can be observed that the set of countries in the sample exhibits different dynamics in the evolution of the NPL ratios over time. In particular, there are countries where the NPL ratio increased during the crisis and decreased thereafter, but to different degrees and from different starting levels (Austria, Belgium and the Netherlands). In some cases, the NPL ratio increased significantly during the crisis and declined also substantially afterwards (Ireland and Spain), also to levels close to those prevalent before the crisis (Estonia and Lithuania). There are also countries where the NPL ratio increased, but did not decline so far (Greece), or did only very recently (Cyprus, Italy and Portugal). Finally, in France, the NPL ratio remained overall unchanged from the beginning to the end of the sample.
Figure 1. Non-performing loan ratios.

Focusing on the correlation between NPL ratios (our main variable of interest) and the remaining variables in the VAR (), we can observe that the change in NPL ratios appears to be negatively and significantly correlated with economic activity and bank lending. Also, an increase in the change in NPL ratios is significantly associated with a widening of bank lending spreads.
Table 3. Correlation matrix among the variables included in the panel VAR.
4. The identification scheme
The simple correlations between GDP growth, changes in the NPL ratios and the remaining macroeconomic and banking sector variables reported above do not allow to disentangle the source of variation of these variables. However, because the relation between these variables can run both ways, it is important to structurally identify the panel VAR.
We use Choleski decomposition in order to estimate the impact of changes in NPL ratios (De Bock & Demyanets, Citation2012; Espinoza & Prasad, Citation2010; Klein, Citation2013). This recursive identification approach implies that variables appearing earlier in the ordering are considered more exogenous than those appearing later. As such, variables that are ordered before a particular structural shock do not react to this shock on impact. Our identifying assumptions are as follows. First, according to the two-pillar monetary policy strategy of the ECB, the monetary policy rate is assumed to respond to a large number of indicators (Bernanke & Boivin, Citation2003; Ciccarelli, Maddaloni & Peydró, Citation2013; ECB, Citation2011). Hence, we rank the monetary policy rate last in the VAR. Second, bank lending and lending spreads affect the capital and reserves-to-asset ratio within the same quarter. This assumption reflects the impact of the profit and loss account (P&L) on capital in the same period when the result was generated. Hence, the capital and reserves-to-asset ratio is ranked before last in the system. Third, we assume that bank lending spreads move faster than macroeconomic variables (GDP and inflation). Hence, we rank spreads after macroeconomic variables but before the capital and reserves-to-asset ratio. Fourth, we follow Bjørnland & Jacobsen (Citation2010) and assume that real estate prices react to macroeconomic developments within the same quarter. Fifth, we assume that macroeconomic variables do not simultaneously react to the policy rate, while policy reacts to the macroeconomic environment simultaneously, as mentioned before. Also, we follow the standard literature on monetary policy and assume that inflation is impacted simultaneously by a shock to economic activity (Bernanke & Gertler, Citation1995; Christiano, Eichenbaum & Evans, Citation1996). Sixth, we assume that it takes time to obtain a loan but once the loan is granted, it affects macroeconomic variables instantaneously. Indeed, we place the macroeconomic variables (real GDP growth and inflation) after the lending variables and the change in the NPL ratio. Seventh, the change in the NPL ratio is placed after the loans because a shock to loans affects contemporaneously this ratio (through a change in its denominator). Lastly, we assume that changes in NPL ratios move slowly, meaning that GDP growth and inflation affect NPLs only with a lag. Indeed, accounting rules allow a loan to be classified as non-performing one quarter after the customer defaults. Hence, the change in the NPL ratio is placed before the macroeconomic variables. This ordering is similar to the ones used by (Hancock, Laing & Wilcox, Citation1995; Klein, Citation2013 and De Bock & Demyanets, Citation2012).
Overall, for our identification strategy, we use the following ordering: annual rate of growth in bank lending volumes to non-financial corporations, annual rate of growth in bank lending volumes to households for house purchase, annual change in the NPL ratio, real GDP growth, inflation rate, real estate prices, bank spreads on lending to non-financial corporations, bank spreads on lending to households for house purchase, bank capital and reserves to assets ratio and monetary policy interest rate.
4.1. Exogenous changes in NPL ratios
As mentioned in this study, we are interested in estimating the impact of exogenous changes in NPL ratios on bank lending and the macroeconomy. Against this background, while the accounting rule mentioned above serves to justify the ordering of the NPL ratio among the variables in the VAR, it is useful to clarify what an exogenous change in NPL ratios can be. Overall, there are several sources of exogenous variations in NPL ratios which are unrelated to changes in the repayment capacity of borrowers.
An exogenous change in NPL ratios can be related to the application of a new definition of non-performing exposures. In particular, while the IMF makes an effort to ensure the cross-country comparability of NPL ratios, it recognises that reporting practices differ among countries (IMF, Citation2006). For the countries in our sample, such reporting practices are affected not only by changes in the national definitions but also by the application of common reporting standards at the euro area level since 2014.Footnote14 In particular, the application of the “Final draft technical standards on NPLs and Forbearance” by the EBA (Citation2013) generally resulted in increases of recognised NPLs, which required banks to record additional provisions and in some cases also affected their capital positions.
Another possible exogenous change in NPL ratios is related to the transfer of non-performing loans from banks to Asset Management Companies (AMCs), which are dedicated entities that manage and workout distressed assets. The aim of these transfers is generally to cleanse banks’ balance sheets of bad loans, enabling banks to resume normal lending activities and to support a recovery in the economy. Various AMCs have been established in Europe after the financial crisis. The first was the National Asset Management Agency (NAMA) which was established in Ireland in 2009. The transfer of impaired assets to the National Asset Management Agency (NAMA) took place between 2009 and 2011. It took several months after it was announced by the authorities due to the necessary administrative arrangements and to the time needed to assess the value of the assets to be transferred. In November 2012, the Management Company for Assets Arising from the Banking Sector Reorganisation (SAREB) was created in Spain. In this case, the authorities had favoured alternative solutions before moving to the establishment of a system-wide AMC.Footnote15 Such AMCs resulted in significant reductions in the level of arrears in the banking system of these two countries, although borrower behaviour or repayment ability did not change as a result of the creation of these entities.
Furthermore, supervisory actions can also have an impact on non-performing loan ratios. For example, at a national level, the supervisory authorities in Cyprus and Ireland set NPL restructuring targets to incentivise banks to accelerate the resolution of bad loans and to encourage sustainable solutions, rather than forbearance.Footnote16 More specifically, the targets in Ireland referred to mortgage loans while in Cyprus they applied to the whole lending book. Also, the targets were not public in Ireland while banking system targets were published in Cyprus.
Actions by the supervisors at the euro area level also had an impact on the level of non-performing loan ratios in recent years. For example, the “Comprehensive Assessment” carried out by the ECB in 2014, which consisted of an Asset Quality Review (AQR) and a Stress Test (ST), resulted in a significant increase in the reported amount of NPLs in the euro area banking system and in the associated level of provisions for impairments (ECB, Citation2014). Furthermore, the NPL guidance provided by the ECB to banks in 2017 and 2018 strengthened banks’ incentives to reduce their NPLs by means of write-offs, restructurings or sales in secondary-markets (SSM, Citation2017; SSM Citation2018). For example, it recommended the timely write-off of uncollectable loans and set up expectations regarding the assessment of bank’s levels of prudential provisions for nonperforming loans. It also recommended the setup of dedicated management units to deal with high-risk clients and bad debt, which are separated from the banks’ sales units. The speed of implementation of such changes has varied from bank to bank and country to country and some countries had started to implement such recommendations beforehand. Importantly, sales of non-performing loans required the creation of secondary markets in the first place, which usually required the adoption of legal and judicial reforms. Taken together, it is estimated that transactions in secondary markets (sales) reduced the euro area NPL ratio by 1.7 p.p. while write-offs and restructurings led to a decline of 3.2 p.p. over the period between the fourth quarter of 2016 and 2018 (ECB, Citation2019).
Finally, another source of exogenous variation in non-performing loan ratios are the so-called “strategic defaults”. These are deliberate defaults which occur when solvent borrowers stop making repayments on a loan as a result of a rational financial strategy. Strategic defaulters are unwilling, rather than unable to pay back their loan. Strategic defaults tend to occur when borrowers see other borrowers defaulting on their obligations without any immediate implication for them. They are more frequent for household mortgages and commercial real estate loans. Such behaviour is usually the reflection of inefficient legal systems, weak enforcement rules and bankruptcy laws, the presence of borrower protection schemes and permissive bank’s attitudes towards risk, among others. While there is wide recognition that the number of “strategic defaulters” might be large in some countries, the size of the problem is difficult to quantify. However, an analysis conducted using data for corporate loans in Greece for the period 2008 to 2015 showed that one in six firms with a nonperforming loan were strategic defaulters (Asimakopoulos, Avramidis, Malliaropulos & Travlos, Citation2016). As a result, the authors highlight the importance of distinguishing the latter from financially distressed defaulters.
5. The results
Having presented the methodology to estimate the model and the assumptions regarding the identification strategy, we illustrate the impact of shocks to the change in NPL ratios in twelve euro area countries relying on three sets of results. First, we present the impulse response functions for this shock. We are especially interested in estimating the size and shape of the responses of the endogenous variables. Second, we report the share of the forecast error variance for each variable and country to assess the degree by which a variable is driven by this shock. Third, we perform additional estimations to assess the robustness of the results. Finally, we implement an out of sample structural conditional forecast analysis to assess and quantify the macroeconomic and financial benefits stemming from a decline in NPL ratios.
5.1. Impulse response analysis
Based on the estimated VAR model described in EquationEquation (1)(1)
(1) , we generate the impulse responses of the endogenous variables to the structural shock for each individual country. and (con’t) report the impulse responses to a one standard deviation shock to the change in the NPL ratio for two groups of countries, namely Austria, Belgium, Cyprus, Estonia, Spain and France in the first Figure, and Greece, Ireland, Italy, Lithuania, the Netherlands and Portugal in the second. The countries are reported in the columns, while the variables are displayed in the rows. The impulse responses are plotted over a four-year horizon (16 quarters) after the shock, which is assumed to take place at time 0. The median of the accepted draws is shown together with the 16% and 84% Bayesian credibility bands.
Figure 2a. Response to a shock to the change in the NPL ratio with new ordering.

shows that the size of the instantaneous shock to the change in NPL ratios is stronger for those countries where the NPL ratio increased the most over the sample period, namely Cyprus, Ireland, Lithuania, Portugal, Greece, Spain and Italy (third row). The impact for these countries ranges between 0.3 and 4.3 percentage points.
The shock to the change in NPL ratios leads to a decline in bank lending which is stronger for non-financial corporations than for households. Indeed, the annual growth of lending declines by up to 1.7 percentage points for non-financial corporations, while it decreases by up to 1 percentage point for households.Footnote17 The relative size of these responses suggests that following a shock to the change in the NPL ratio, banks materially deleverage their balance sheets. At the same time, the impulse responses show that there is more heterogeneity in the timing of the peak response for mortgages (between four and twelve quarters after the shock) rather than for non-financial corporations (between six and ten quarters after the shock). The shock also leads to a slight widening in both bank lending spreads (of up to about 0.3 percentage points) and to a decline in residential property prices (of up to 3.4 percentage points). For all these variables, the maximum impact is recorded for Cyprus, but strong effects can be seen also in Ireland, Lithuania and Estonia. While the responses of the capital and reserves to asset ratio vary across countries, a material increase is recorded in Cyprus, Spain, Ireland, Italy, Lithuania and Portugal, due to the recorded increase in provisions for impairments during the crisis.
The shock to the change in the NPL ratio also leads to a decline in real GDP growth in most of the countries (by between 0.07 and 1 percentage point), between two and seven quarters after the shock. As a result of the deterioration in economic activity, monetary policy is relaxed. The response of the inflation rate is rather heterogeneous across countries. These findings are in line with those of Klein (Citation2013) and Espinoza & Prasad (Citation2010). These authors estimate the impact of much larger shocks, but their relative impact is comparable to ours.Footnote18 Theoretical models also support our findings. For example, Curdia & Woodford (Citation2010) develop a dynamic stochastic general equilibrium model with credit frictions and find that an increase in the loss rate of loans (i.e., the equivalent to non-performing loans in our empirical model) leads to a widening in credit spreads, a contraction in credit and also to a substantial fall in real activity. All in all, it can be observed that the shape of the responses tends to vary widely across the countries in the sample, both in terms of size and shape. This finding may be attributed to the fact that the various banking sectors experienced different degrees of variations in NPL ratios and at different times, hence generating heterogeneous responses.
5.2. Forecast error variance decomposition
In this section, we present a forecast error variance decomposition to uncover further details on the relationship among the variables included in the model. The analysis shows the share of the forecast error variance of individual variables explained by exogenous shocks to other variables. In general, we expect that shocks to the change in NPL ratios are relatively more relevant drivers of the variables in the countries where NPL ratios increased the most because these countries have been impacted by stronger and more frequent shocks to NPLs. The results of this analysis are presented in . In the Table, we report the share of the variance for each variable and each country in the panel VAR up to a 16-quarter horizon.
Table 4. Forecast error variance decomposition (shock to the change in the NPL ratio).
The shock to the change in the NPL ratio explains a non-negligible share of the variance of the variables included in the VAR. In particular, these shocks are sizable drivers of real GDP growth, explaining between 10% and 33% of the variance in Lithuania, Estonia, Ireland and Cyprus. For the remaining countries, the NPL shock still explains between 2% and 5% of the variance of real GDP growth. Regarding bank lending, the share explained is larger for corporate lending than for mortgages. For NFC lending, the NPL shock explains between 5% and 17% of the variance for Cyprus, Ireland, Lithuania, Italy and Portugal. For mortgage lending, the share is large only for Cyprus, but smaller than 3% for the remaining countries.Footnote19 For spreads (both corporate and mortgage), the share is above 10% for Cyprus, Ireland and Lithuania, and for Italy is larger than 10% only for spreads on corporate lending. For residential real estate prices, the share is large for Cyprus, Ireland and Estonia (between 12% and 56%). Importantly, we observe that shocks to the change in the NPL ratio explain more than 50% of the variance in the same variable at the end of the horizon in countries like Cyprus, Greece, Ireland, Italy, Lithuania, Portugal and Spain. This finding suggests that exogenous factors have been key drivers of changes in NPL ratios.
These findings are broadly in line with the literature. Over long horizons (between 5 and 10 years), Espinoza & Prasad (Citation2010), De Bock & Demyanets (Citation2012) and Klein (Citation2013) find that shocks to the change in NPL ratios explain about 6%, 8% and 20% of the variance of GDP growth in their sample of countries, respectively. For the credit-to-GDP ratio (equivalent to bank lending in our model), the estimated share stands at 13% and 8% in De Bock & Demyanets (Citation2012) and Klein (Citation2013), respectively. For the change in the NPL ratio, Klein (Citation2013) and De Bock & Demyanets (Citation2012) find that 70% and 90% of the variance is explained by the same variable, respectively.
The shock to the change in the NPL ratio explains for most countries in our model a smaller share of the variance of our variables than the literature on credit supply shocks and shocks to bank capital would suggest.Footnote20 This is, however, not surprising, because the increase in NPLs has been only one of several disturbances that have in recent years affected our sample of countries.Footnote21 However, for some countries, particularly those hardly hit by the crisis, and some variables, the share is equally important and sometimes larger than that found in the literature.
5.3. Robustness analysis
This section implements two robustness checks of the impulse-response analysis performed before to assess the reliability of our results. First, we generate impulse responses to a shock in the NPL ratio relying on a different ordering of the variables in the Choleski factorisation. In this new ordering, loans and NPL ratios are included in the VAR after GDP and inflation and, thus, are affected by macroeconomic variables contemporaneously. Second, we replace the NPL ratios with the NPL volumes and order this variable first in the VAR.
In the first robustness check, we relax two of the identifying assumptions made in Sub-section 4. In particular, in that analysis, we assumed that i) It takes time to obtain a loan, but once the loan is granted, it instantaneously affects macroeconomic variables; and ii) Changes in NPL ratios move slowly, implying that GDP growth and inflation affect NPLs only with a lag. These assumptions led us to place the macroeconomic variables (real GDP growth and inflation) after the lending variables and the change in the NPL ratio. By contrast, in our first robustness check, we order loans and the NPL ratio after the two macroeconomic variables, implying a fast reply of these variables to changing macroeconomic conditions. The ordering used is as follows: real GDP growth, inflation rate, annual rate of growth in bank lending volumes to non-financial corporations, annual rate of growth in bank lending volumes to households for house purchase, annual change in the NPL ratio, annual rate of change in real estate prices, bank lending spreads on lending to non-financial corporations, bank lending spreads to households for house purchase, bank capital and reserves to assets ratio and monetary policy interest rate.
In the second robustness check, we replace the series of NPL ratios with a series of NPL volumes. The 90 days past due (DPD) rule discussed in Sub-section 3 applies to the volume of NPLs. Indeed, as banks are allowed to classify a loan as non-performing a quarter (90 days) after a loan defaulted, we rank the NPL volume first in the VAR, implying that it is not affected contemporaneously by the remaining variables in the VAR. The series of NPL volumes are computed by multiplying the NPL ratios presented in Sub-section 3 by the stock of loans at the country level from the MFI Balance Sheet Statistics of the ECB, which has data for 10 of the 12 countries in our sample.Footnote22 The NPL volumes enter the VAR as annual percentage changes. In this second robustness check, we use the following ordering: the annual rate of growth in NPL volumes, real GDP growth, the inflation rate, the annual rate of growth in bank lending volumes to non-financial corporations, annual rate of growth in bank lending volumes to households for house purchase, annual rate of growth of real estate prices, bank lending spreads to non-financial corporations, bank lending spreads to households for house purchase, bank capital and reserves to assets ratio and monetary policy rate.Footnote23
present the results for these two extensions. Most of the results are broadly in line with those presented before, although they are somewhat weaker when assuming that loans and NPLs reacts to macroeconomic variables instantaneously. In particular, both mortgage and corporate spreads are less significant across countries with the new ordering. Furthermore, for the impact on lending, it can be observed that there are changes in the significance of the responses across countries (some countries with previous significant responses are not insignificant anymore and vice versa), while the total number of significant responses is broadly unchanged. Finally, the impact on real estate prices and real GDP growth is also unchanged across countries. Regarding the responses of the endogenous variables to a shock in NPL volumes, the magnitudes are not comparable with those presented before (due to the different scale of the change in the NPL ratio and in the NPL volumes) but results are qualitatively similar. In particular, the number of significant responses of corporate and mortgage spreads increases while that one of real estate prices decreases. For real GDP growth and lending, the number of significant responses remains broadly unchanged, but with changes for specific countries.
Figure 3. Response to a shock to the annual rate of change in NPL volumes.

All in all, this robustness analysis confirms to a large extent the results presented in Sub-section 5.1. In particular, we continue to find that banks materially deleverage their balance sheets following a shock to NPLs and the impact is stronger for non-financial corporations than for households. The shock also leads to a decline in real GDP growth and residential property prices.
5.4. Structural out of sample scenario analysis
This section reports the results of a structural, out-of-sample scenario analysis which assesses the impact of two different paths of NPL ratios over the period 2017Q4 to 2020Q3. This exercise provides a quantitative illustration of the possible benefits associated with a further decline in NPL ratios in euro area countries. In this analysis, we focus on the six most relevant variables in the VAR and on the six countries that exhibited the most sizable increase in NPL ratios during the crisis, namely Cyprus, Ireland, Spain, Italy, Greece and Portugal. Under a baseline scenario, the out of sample change in the NPL ratio for each country is assumed to equal the average change recorded over the last four quarters of the historical data.Footnote24 Under an adverse scenario, the out of sample change in the NPL ratio is assumed to equal zero. In both cases, the remaining variables in the VAR are projected conditional on the assumed evolution of the change in the NPL ratio, following the methodology proposed by Antolin-Diaz, Petrella & Rubio-Ramírez (Citation2018). In particular, the forecasts are computed assuming that only the structural shock to the change in the NPL ratio adjusts to ensure the new path for the conditioning variable.Footnote25 This structural scenario is more meaningful than the one proposed by Waggoner & Zha (Citation1999), who assume that all the structural shocks adjust to ensure the evolution of the conditioning variable. Applied to our model, it would mean assessing the most likely set of circumstances under which the change in the NPL ratio evolves as prescribed. Instead, we are interested in computing the paths of the endogenous variables in the VAR which are consistent with a sequence of shocks to the change in the NPL ratio per se.
The observed and out of sample evolution of the change in NPL ratios for the two paths and the six countries are depicted in . By construction, the gap between the baseline and the adverse changes in the NPL ratio depends on how strongly the variable evolved in the last four quarters of our sample. This gap is the widest for Cyprus, followed by Ireland, Portugal, Italy and then Spain and Greece. These assumptions result in different levels of the NPL ratio at the end of the forecast horizon. Under the baseline, the level of the NPL ratio is expected to decline (with respect to the starting point) by 10.7 percentage points in Cyprus, 9.7 percentage points in Ireland, 5 percentage points in Portugal, 3.2 percentage points in Italy and 1.6 percentage points in both Spain and Greece.
Figure 4. Observed and assumed out-of-sample baseline and adverse change in NPL ratios for the structural scenario analysis.

The out of sample deviation between the baseline and adverse conditional forecasts of the variables is reported in . The countries are reported in the columns, while the variables are depicted in the rows. A positive value implies that the baseline forecast exhibits a higher value than the adverse one. The results show, as expected, that a further reduction in NPL ratios would have a positive impact on both the macroeconomic and the banking variables. At the end of the forecast horizon, the annual rate of growth of mortgage lending under the baseline scenario is between 1.4 (Italy) and 2.9 (Ireland) percentage points higher than under the adverse scenario, while the annual rate of growth of corporate lending increases faster by between 0.9 (Spain) and 4.4 (Ireland) percentage points. Bank lending spreads are narrower, by between 0.2 and 0.6 percentage points for mortgages and by between 0.2 and 0.8 for loans to non-financial corporations under the baseline scenario. Stronger lending and lower spreads lead to higher residential real estate prices, with annual rates of growth being between 1.6 (Italy) and 6.7 (Cyprus) percentage points higher under the baseline than the adverse. Finally, the rate of growth of real GDP is higher by between 0.5 (Italy) and 1.6 (Ireland) percentage points. Overall, this structural out-of-sample forecast illustrates that a further reduction in NPL ratios can generate significant medium-term economic benefits in euro area countries.
Figure 5. Difference in the structural scenario forecasts between the baseline and the adverse path for the main variables included in the panel VAR.

6. Conclusion
Non-performing loan (NPL) ratios increased substantially in many euro area countries since the onset of the global financial crisis. Despite a gradual decline from the peak in 2014, NPL ratios still remained a key problem in several euro area countries at the end of 2017. High NPL ratios can impair the stability of the banking system and its ability to lend to the real economy. Therefore, in particular, for highly bank-dependent economies such as the euro area, the necessity to deal with elevated NPL ratios is unquestionable.
Against this background, we quantify the impact of an exogenous increase in the change in NPL ratios on economic and banking sector developments in twelve euro area countries. Given the relatively short time series available for NPL ratios and the large number of parameters to be estimated, we estimate a panel Bayesian VAR model with hierarchical priors that allows for country-specific coefficients. The variables included in the panel VAR are economic activity (which is a proxy for the repayment capacity of borrowers), inflation, the monetary policy rate, real estate prices, bank lending volumes both to non-financial corporations and to households for house purchase, bank lending spreads to these two sectors, the ratio of capital and reserves over total assets and the change in NPL ratios. We estimate the impact of the shock using a Choleski factorisation approach.
We illustrate the impact of this shock relying on three sets of results. Looking first at the impulse response functions, we find that an exogenous increase in the change in NPL ratios depresses bank lending, widens lending spreads and leads to a fall in real GDP growth and residential real estate prices and an easing of monetary policy. The responses of inflation and capital and reserves over total assets vary across countries. However, the latter significantly increases in Cyprus, Spain, Ireland, Italy, Lithuania and Portugal. The reason is that this variable includes provisions for impairments which materially increased in these countries during the crisis. These results are robust to a change in the ordering of the variables in the Choleski factorisation (whereby bank loans and the NPL ratio are affected contemporaneously by macroeconomic variables) and also when including in the VAR the annual rate of growth in NPL volumes (instead of the NPL ratio) and order it first in the VAR. The forecast error variance decomposition also shows that shocks to the change in NPL ratios explain a large share of the variance of the variables in the VAR, particularly for those countries that experienced a large increase in NPL ratios during the crisis. Finally, a three-year structural out of sample forecast analysis provides quantitative evidence that a further reduction of NPL ratios can produce significant economic benefits in euro area countries.
Disclosure statement
No potential conflict of interest was reported by the author(s).
Additional information
Notes on contributors
Ivan Huljak
Ivan Huljak is Principal Advisor at the Croatian National Bank (HNB) in Zagreb, Croatia. His main duties are the coordination of financial stability risks analysis and related research, mostly covering the Non-financialCorporates and Banking sector but being also involved in various policy work. Between 2016 and 2019, he worked at the European Central Bank as Senior Financial Stability Expert in the Systemic Risk and FinancialInstitutions Division and earlier in the Stress Test Modelling Division. He also worked as a Consultant for the World Bank and was a fellow at the University of Zagreb, teaching Corporate Finance. He holds a Ph.D. inEconomics from the University of Split and a Master degree in Economics from the Universities of Zagreb.
Reiner Martin
Reiner Martin is Head of Research at the National Bank of Slovakia. Previously, he was Lead Economist at the Joint Vienna Institute (JVI) in Vienna, Austria, where he provided applied training courses for public sector officials on a range of financial and macroeconomic topics.He also conducts related research, mainly on non-performing loan resolution, macroprudential policy and stress testing. Until August 2018, he was Deputy Head of the European Central Bank’s Macroprudential Policy Division and, prior to that, Deputy Head of the ECB’s Stress Testing Division. Earlier than that, he was Research Advisor at the Oesterreichische Nationalbank (Austrian Central Bank) and Head of the ECB’s Convergence Section. He holds a Ph.D. in Economics from the University of Hamburg (Germany) and Master degrees in Economics and Political Science from the Universities of Sussex and Bristol (UK).
Diego Moccero
Diego Moccero is a Principal Financial Stability Expert at the Macroprudential Policies Division of the European Central Bank in Frankfurt, Germany. He conducts policy-oriented analysis on cyclical systemic risks and macro prudential policies in euro area countries. He also conducts related research, mainly on the impact of nonperforming loan on macroeconomic developments, the impact of bank capital buffers on bank lending and the economy and the measurement of financial cycles. Previously, he was Team Lead Expert at the EuropeanSystemic Risk Board (ESRB), coordinating a report covering macro-financial risks in EU countries. He also worked on stress testing as well as euro area macroeconomic and capital market developments. Before joining the ECB, he worked at the Organisation for Economic Cooperation and Development (OECD) in Paris, France. He holds a PhD in Economics from Paris School of Economics (PSE).
Cosimo Pancaro
Cosimo Pancaro is Team Lead at the European Central Bank working in the Systemic Risk and and Financial Institutions Division in the Macroprudential Policies and Financial Stability Department. Previously, he worked in the Stress Test Modelling Division. In the past, he was the lead of the top-down credit risk team. He has been involved both in country level stress tests carried over during the European sovereign crisis and in several EU-wide stress tests. Cosimo is the author of a number of academic and policy articles on different topics in the field of financial stability and stress-testing. Prior to joining the ECB, he worked as economist at the World Bank and as post-doctoral fellow at the University of Lausanne. He holds a PhD in Economics from the EuropeanUniversity Institute and a Master in Economics from the University of Essex.
Notes
1 For example, Arnould, Pancaro & Zochowski (Citation2019) find that lower credit quality is significantly associated with higher banks’ senior bond yields.
2 Grodzicki, Laliotis, Leber, Martin, O’Brien & Zboromirski (Citation2015), Fell, Grodzicki, Martin & O’Brien (Citation2016a), Fell, Grodzicki, Krušec, Martin & O’Brien (Citation2017) extensively elaborate on the challenges for the banking system stemming from the accumulation of non-performing exposures. Additionally, they illustrate macroeconomic and microeconomic policies which could be adopted to resolve this legacy issue.
3 The literature finds that inflation generally reduces the loan servicing burden. However, it also shows that, if wages are sticky, higher inflation might induce a fall in real income and, thus, cause an increase in the debt servicing burden.
4 These groups of countries include the Gulf Cooperative Council (GCC) countries, a group of 26 advanced economies, a large sample of emerging markets and Central, Eastern and South-Eastern Europe (CESEE), respectively.
5 The estimations in this paper were implemented relying on the BEAR toolbox and MATLAB codes developed by Dieppe, van Roye & Legrand (Citation2016).
6 The author compares impulse responses to monetary policy shocks in five euro area countries before the EMU and in four of the newer European Union member states from central–eastern Europe.
7 In particular, the values for the shape and scale parameters are given by and
, with
.
8 For example, Espinoza & Prasad (Citation2010) includes up to four variables, De Bock & Demyanets (Citation2012) and Klein (Citation2013) include five variables, while ECB (Citation2011) includes nine variables.
9 We use the modified Gross National Income (GNI*) instead of GDP because changes in the latter have become increasingly disconnected from actual trends in domestic living standards due to the sizeable distortion resulting from widespread activities of multinational companies. Instead, the GNI* attempts to control for (part of) the impact of globalisation on Irish macro-economic statistics. See Department of Finance (Citation2018) for more details.
10 Using notional stocks to compute the annual growth rates, rather than outstanding amounts, is important because the latter reflect not only the cumulative effect of financial transactions but also the impact of other non-transaction related changes (e.g., instrument reclassification, changes in exchange rates, price fluctuations and loan write-offs/write-downs, etc.). Excluding such non-transaction related changes is more meaningful for economic analysis.
11 Data for Estonia for loans to non-financial corporations before 2008 has been compiled by the Central Bank of Estonia and kindly shared with the authors.
12 The exception is lending rates to non-financial corporations in Greece, where the rates based on outstanding amounts have been used due to lack of data on new businesses.
13 Data for Estonia for capital and reserves and total assets before 2008 has been compiled by the Central Bank of Estonia and shared by the ECB.
14 Cyprus is a case where the NPL ratios increased substantially in end 2013 due to a new Directive by the Central Bank of Cyprus (CBC) which required credit institutions to classify a loan as non-performing if a loan fulfilled the following criteria: the loan was defaulted by more than 90 days, the loan was restructured, concessions have been granted to the borrower, and related account were defaulted. In several of these cases, the loans needed to be classified as NPLs (even if performing) for a period of one year. The change in the definition of NPLs made the country compliant with IFRS accounting standards and the result was a large increase in the NPL ratio and in provisions for impairment, which impaired bank’s capital positions.
15 For more details about AMCs in the euro area see Fell, Grodzicki, Martin & O’Brien (Citation2016b
16 The targets involved different steps in the restructuring process, for example, the number of proposed sustainable restructurings, the number of concluded restructurings, etc.
17 This result is consistent with Fell, Grodzicki, Metzler, & O’Brien (Citation2018). Using bank level data, they find that there is a negative significant relationship between the ratio of NPLs over tier 1 capital and loan origination. This relationship appears to be stronger for lending to non-financial corporations than for mortgages.
18 Klein (Citation2013), estimate that a 3 percentage point instantaneous shock to the change in the NPL ratio leads to a decline in real GDP growth of about 2 percentage point after one year. Espinoza & Prasad (Citation2010) find a relatively stronger impact. Indeed, they find that a 2.3 percentage point increase in the change in the NPL ratio leads to a decline in GDP growth of about 2 percentage points.
19 For comparison, Hristov, Hülsewig & Wollmershäuser (Citation2012) find that demand shocks explain 13% of the variance of the GDP deflator and 16% of lending volumes over a four year horizon in a sample of euro area countries.
20 For example, Mésonnier & Stevanovic (Citation2017) find that after one year, a bank capital shock accounts for some 4% of the variance of GDP growth and 11% of the variance of loan growth in the U.S. For the same country, Meeks (Citation2012) finds that credit market shocks account for 20% of the total mean square prediction error in industrial output at a three-year horizon. Bassett, Chosak, Driscoll & Zakrajšek (Citation2014) find the same share at a four year horizon for credit supply shocks. Hristov, Hülsewig & Wollmershäuser (Citation2012) find that credit supply shocks explain about 15% of the fluctuations recorded in real output, 12% of loan rates and 11% of loan volumes in a sample of euro area countries over a four year horizon. However, for some countries, particularly those hardly hit by the crisis, and some variables, the share is equally important and sometimes larger than that found in the literature on credit or bank capital shocks.
21 Other factors include, inter alia, liquidity and funding shocks, changes in bank competition, shocks to the perceived credit riskiness of borrowers and shocks to lending standards, among others.
22 The MFI Balance Sheet data for the loan volumes are not available for Cyprus and Estonia.
23 Once the variable of interest is ordered first in the VAR (i.e., it is assumed to be the most exogenous one), then the order of the remaining variables in the VAR is irrelevant for the calculation of response functions to a shock to that variable.
24 More specifically, it is assumed that the out of sample change in the NPL ratio is equal to −3.6% for Cyprus, −0.5% for Spain, −0.5% for Greece, −3.2% for Ireland, −1.1% for Italy and −1.7% for Portugal.
25 Restricting the value of a variable in the VAR over a certain period of time gives rise to a system of conditions that the structural disturbances should verify. Waggoner & Zha (Citation1999) show that the distribution of these restricted structural disturbances is normal. Jarocinski (Citation2010) proposed a numerically more efficient method that avoids drawing the shocks from that distribution. See Dieppe, van Roye & Legrand (Citation2016) for more details.
References
- Accornero, M., Alessandri, P., Carpinelli, L., & Sorrentino, A. M., “Non-performing loans and the supply of bank credit: Evidence from Italy,” Questioni di Economia e Finanza (Occasional Papers) 374, Bank of Italy, Economic Research and International Relations Area, March 2017.
- Anastasiou, L. H. D., & Tsionas, M. G., “Non-performing loans in the euro area: Are core-periphery banking markets fragmented?,” Working Papers 219, Bank of Greece, 2016.
- Antolin-Diaz, J., Petrella, I., & Rubio-Ramírez, J. F., “Structural scenario analysis with SVARs,” CEPR Discussion Papers 12579, C.E.P.R. Discussion Papers, January 2018.
- Arnould, G., Pancaro, C., & Zochowski, D., “Bank funding costs and solvency,” Working Paper 2356, European Central Bank January, 2019.
- Asimakopoulos, I., Avramidis, P. K., Malliaropulos, D., & Travlos, N. G., “Moral hazard and strategic default: Evidence from Greek corporate loans,” Working Papers 211, Bank of Greece, July 2016.
- Balgova, M., & Plekhanov, A., “The economic impact of reducing non-performing loans,” Working Paper Series 193, European Bank for Reconstruction and Development, June 2016.
- Bassett, W. F., Chosak, M. B., Driscoll, J. C., & Zakrajšek, E. (2014). Changes in bank lending standards and the macroeconomy. Journal of Monetary Economics, 62, 23–40.
- Beck, T., De Jonghe, O., & Schepens, G. (2013). Bank competition and stability: Cross-country heterogeneity. Journal of Financial Intermediation, 22(2), 218–244.
- Bernanke, B. S., & Boivin, J. (2003). Monetary policy in a data-rich environment. Journal of Monetary Economics, 50(April), 525–546.
- Bernanke, B. S., & Gertler, M. (1995). Inside the black box: The credit channel of monetary policy transmission. Journal of Economic Perspectives, 9(4), 27–48.
- Bjørnland, H. C., & Jacobsen, D. H. (2010). The role of house prices in the monetary policy transmission mechanism in small open economies. Journal of Financial Stability, 6(4), 218–229.
- Canova, F. (2005). The transmission of US shocks to Latin America. Journal of Applied Econometrics, 20(2), 229–251.
- Christiano, L. J., Eichenbaum, M., & Evans, C. (1996). The effects of monetary policy shocks: Evidence from the flow of funds. The Review of Economics and Statistics, 78(1), 16–34.
- Christiano, L. J., Eichenbaum, M., & Evans, C. L. (1999). Monetary policy shocks: What have we learned and to what end? In J. B. Taylor & M. Woodford (Eds.), Handbook of Macroeconomics 1 , chapter 2 65–148. North Holland: (Elsevier)
- Ciccarelli, M., Maddaloni, A., & Peydró, J.-L., “Heterogeneous transmission mechanism: Monetary policy and financial fragility in the euro area,” Working Paper Series 1527, European Central Bank, March 2013.
- Curdia, V., & Woodford, M. (2010). Credit spreads and monetary policy. Journal of Money, Credit and Banking, 42(September), 3–35.
- De Bock, R., & Demyanets, A., “Bank asset quality in emerging markets; determinants and spillovers,” IMF Working Papers 12/71, International Monetary Fund, March 2012.
- Department of Finance, “GDP and modified GNI – explanatory note,” Technical Report, Economic Division, Department of Finance, Ireland, May 2018.
- Dieppe, A., van Roye, B., & Legrand, R., “The BEAR toolbox,” Working Paper Series 1934, European Central Bank, July 2016.
- EBA, “Final draft technical standards on NPLs and Forbearance,” Technical Report, European Banking Authority, 2013.
- ECB, “The monetary policy of the ECB,” Technical Report, European Central Bank, 2011.
- ECB, “Aggregate report on the comprehensive assessment,” Technical Report, European Central Bank, 2014.
- ECB, “Financial stability review,” European Central Bank, 2019.
- Espinoza, R. A., & Prasad, A., “Nonperforming loans in the GCC banking system and their macroeconomic effects,” IMF Working Papers 10/224, International Monetary Fund, October 2010.
- Fell, J., Grodzicki, M., Krušec, D., Martin, R., & O’Brien, E. (2017). Overcoming non-performing loan market failures with transaction platforms. In Financial Stability Review, Special Features. Frankfurt am Main: European Central Bank, 130–144, November.
- Fell, J., Grodzicki, M., Martin, R., & O’Brien, E. 2016a. Financial Stability Review, November. European Central Bank.
- Fell, J., Grodzicki, M., Martin, R., & O’Brien, E. 2016b. Financial Stability Review, European Central Bank. European Central Bank .
- Fell, J., Grodzicki, M., Metzler, J., & O’Brien, E. (2018). In Financial Stability Review Madrid: Bank of Spain. November.
- Goetz, M. R. (2018). Competition and bank stability. Journal of Financial Intermediation, 35, 57–69.
- Grodzicki, M., Laliotis, D., Leber, M., Martin, R., O’Brien, E., & Zboromirski, P. 2015. Resolving the legacy of non-performing exposures in euro area banks,” Financial Stability Review , Special Feature European Central Bank. May.
- Hancock, D., Laing, A. J., & Wilcox, J. A. (1995). Bank capital shocks: Dynamic effects on securities, loans, and capital. Journal of Banking & Finance, 19(3–4), 661–677.
- Hartmann, P, “Real estate markets and macroprudential policy in Europe,” Working Paper Series 1796, European Central Bank, May 2015.
- Hristov, N., Hülsewig, O., & Wollmershäuser, T. (2012). Loan supply shocks during the financial crisis: Evidence for the euro area. Journal of International Money and Finance, 31(3), 569–592.
- Iacoviello, M. (2005). House prices, borrowing constraints, and monetary policy in the business cycle. American Economic Review, 95(3), 739–764.
- IMF, “Financial soundness indicators compilation guide (Guide),” Technical Report, International Monetary Fund, 2006.
- Jarocinski, M. (2010). Conditional forecasts and uncertainty about forecast revisions in vector autoregressions. Economics Letters, 108(3), 257–259.
- Jarociński, M. (2010). Responses to monetary policy shocks in the east and the west of Europe: A comparison. Journal of Applied Econometrics, 25(5), 833–868.
- Jiménez, G., & Saurina, J. (2006). Credit cycles, credit risk, and prudential regulation. International Journal of Central Banking 2 (2): 65–98.
- Klein, N, “Non-performing loans in CESEE; determinants and impact on macroeconomic performance,” IMF Working Papers 13/72, International Monetary Fund, March 2013.
- Louzis, D. P, Vouldis, A. T., & Metaxas, V. L. (2012). Macroeconomic and bank-specific determinants of non-performing loans in Greece: A comparative study of mortgage, business and consumer loan portfolios. Journal of Banking & Finance, 36(4), 1012–1027.
- Meeks, R. (2012). Do credit market shocks drive output fluctuations? Evidence from corporate spreads and defaults. Journal of Economic Dynamics and Control, 36(4), 568–584.
- Meeks, R. (2017). Do, “Capital regulation and the macroeconomy: Empirical evidence and macroprudential policy. European Economic Review, 95, 125–141.
- Mésonnier, J.-S., & Stevanovic, D. (2017). The macroeconomic effects of shocks to large banks’ capital. Oxford Bulletin of Economics and Statistics, 79(4), 546–569.
- SSM, “Addendum to the ECB Guidance to banks on nonperforming loans: Supervisory expectations for prudential provisioning of non-performing exposures.” Technical Report, Single Supervisory Mechanism, 2018.
- SSM, “Guidance to banks on non-performing loans,” Technical Report, Single Supervisory Mechanism, 2017.
- Waggoner, D. F., & Zha, T. (1999). Conditional forecasts in dynamic multivariate models. The Review of Economics and Statistics, 81(4), 639–651.
- Walentin, K. (2014). Business cycle implications of mortgage spreads. Journal of Monetary Economics, 67, 62–77.