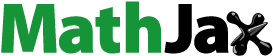
ABSTRACT
This paper analyzes the impact of high-speed train construction on enterprise innovation and its specific mechanisms by establishing high-speed train-city-firm level panel data and constructing a multi-period DID model in China. The results show that, firstly, the construction of a high-speed train significantly improves the innovation level of enterprises, and the construction of the first high-speed train can increase the number of patents of local listed enterprises by about 11.5%. Second, the impact of high-speed train construction on the innovation level of listed enterprises is mainly through the internal human capital and external marketization of enterprises. Specifically, the high-speed train construction affects innovation by weakening the transportation distance; on the other hand, it improves the external market environment and enhances the market competition, making firms seek innovation actively.
1. Introduction
Since 2007, China’s high-speed train construction has been developing rapidly, and by the end of 2020, China’s high-speed train will operate 36,000 km, occupying two-thirds of the world’s high-speed train running mileage and ranking first in the world (B, C, C, & B, Citation2013; Y Gao, Song, Sun, & Zang, Citation2020). The construction of high-speed trains breaks down the original urban boundaries by shortening travel time, breaking down the spatial barriers between regions, and diluting transportation distance’s impact (Castillo-Manzano, Pozo-Barajas, & Trapero, Citation2015; Kamga, Citation2015). Analyzed from the perspective of production factors, the construction of high-speed trains expands the options for the mobility of production factors in today’s increasingly inter-city connections. This is because high-speed train construction shortens transportation travel time and improves the accessibility of cities with high-speed train stations, thus reducing the cost of cross-city and cross-regional mobility of production factors such as people, capital, and information (Duan, Sun, & Zheng, Citation2020; D. Wang, Qian, Chen, Zhao, & Zhang, Citation2014; Willigers & Wee, Citation2011). For cities, the high-speed train construction makes cities more attractive. More scholars and experts are willing to come to third-tier second-and towns to live and work, thus bringing production factors such as knowledge, information, and technology (Dong, Zheng, & Kahn, Citation2019).
For companies, the rapid development of high-speed trains has several impacts. First, it expands the range of product sales reduces the cost of transporting goods and the cost of exchanging resources across regions, making it easier for firms to enter a market and increase their profits (Hof, Heyma, & Hoorn, Citation2012). Second, it brings foreign competitors to the local market, intensifying market competition and stimulating firms to innovate on their initiative (X. Hu, Huang, & Shi, Citation2019; Soetevent, Haan, & Heijnen, Citation2014). Finally, expanding the “talent pool” of enterprises, on the one hand, increases the attractiveness of enterprises in recruitment due to high-speed training and develops the recruitment scope to recruit high-quality talents; on the other hand, employees can improve their professional quality through on-the-job postgraduate studies or exchange and cooperation with universities and research institutes, thus, On the other hand, employees can improve their professional quality through doctoral studies or exchange and collaboration with universities and research institutes, thus enhancing the innovative strength of the company (X. Hu et al., Citation2019; Perl & Goetz, Citation2015).
In this paper, we analyze the impact of high-speed train construction on corporate innovation and broaden the scope of micromechanisms of high-speed train impact. With the exogenous event of the high-speed train construction, this paper analyzes the effects of the high-speed train on firms’ innovation by influencing the proportion of R&D staff internally, the level of regional marketization, and the increase of sales revenue faced externally by firms.
The primary purpose of this paper is to investigate the impact of high-speed train construction on the innovation capability of listed companies. The main question addressed in this paper is whether the construction of high-speed trains in China can enhance the innovation capability of firms? If it can, what is the specific mechanism of its impact? Firstly, based on the literature analysis method, the macro, regional and micro effects of high-speed train construction are summarized and analyzed. It is found that the existing literature mainly focuses on the macro level, with relatively little research on micro-enterprises and relatively little analysis on the mechanism of the effect of high-speed train construction on enterprise innovation. Based on this, this paper analyzes the mechanism of the development of high-speed railway construction on enterprise innovation from the internal and external influences of enterprises, among which the interior through the power of human capital level and sales revenue level, and the exterior through the power of marketization level. Second, this paper collects balanced panel data at the HSR-city-firm level, establishes a multi-period DID model of HSR construction, empirically tests the hypotheses proposed in the theoretical analysis and conducts robustness tests and heterogeneity analysis on the empirical results.
Based on the existing research on high-speed train construction and corporate innovation, we seek to make a marginal contribution to the existing literature. First, the current studies on high-speed train construction mainly focus on the impact on regional economic development and economical industrial structure, and most of the studies are based on provincial or prefecture-level city data, but relatively few studies on individual micro listed companies (Chen & Haynes, Citation2017; Jia, Shao, & Yang, Citation2021; D. Wang et al., Citation2014; Yao, Zhang, Wang, & Ou, Citation2019). In this paper, we mainly use the data at the level of listed companies and patents to portray the level of corporate innovation to enrich the research on the impact of high-speed train construction in micro aspects. Second, most existing literature mentions that high-speed training affects corporate innovation by affecting human capital, but less mentions how it affects it. In this paper, we first analyze the impact of high-speed train construction on enterprises and the mechanism of high-speed train construction on R&D personnel through high-speed train-transportation distance-R&D personnel-enterprise innovation. In addition to the internal mechanism of R&D personnel, this paper further analyzes the impact of the external marketization level on the creation of enterprises. Finally, this paper examines the effects of high-speed train construction through the mechanism of high-speed train construction-sales revenue-enterprise innovation. The existing studies on the impact of high-speed train construction on enterprise innovation have been conducted from the perspectives of alleviating financing constraints, influencing the distance from innovation center, and increasing the proportion of highly educated employees. And this paper tries to analyze the specific mechanism of high-speed train construction affecting the innovation level of enterprises from three perspectives: human capital and sales revenue of enterprises and external marketability level, which is the main innovation of this paper.
2. Literature review
2.1. Macro impact of high-speed train construction
The introduction of high-speed trains has dramatically changed the demand for different modes of transport (Coto-Millán, Inglada, & Rey, Citation2007). The most significant social benefit is the improved accessibility of the cities they serve (Kanafani, Rui, & Griffin, Citation2012). The development of high-speed train networks has improved the accessibility of central cities (Andersson, Shyr, & Fu, Citation2010), bringing the surrounding areas closer to the major cities (Gutiérrez, González, & Gómez, Citation1996). Studies based on prefecture-level city data have shown that the opening of high-speed trains promotes economic growth and has structural effects (Yang, Li, Jiao, Liu, & Zhang, Citation2020; Yao et al., Citation2019). Specifically, industrial development in developed cities relies on technological investment to promote industrial upgrading, and less developed cities receive industrial transfers from developed cities (B et al., Citation2013; X. Hu et al., Citation2019). In addition, the high-speed train network has significantly strengthened the role of east and central China in transportation (Chen & Haynes, Citation2017; Yu, Citation2021), illustrating from a geographic perspective that the construction of high-speed trains has led to an increasing intensity of urban economic ties between urban agglomerations.
The construction of high-speed trains works to improve regional economic development by driving the growth of high-speed train-related industries and improving transportation conditions (David, Andersson, ShyrAngel, & Lee, Citation2012). The impact of high-speed training on regional economic development is twofold: on the one hand, the positive impact is reflected in regional economic integration, co-location effect, buoyant industry, and diffusion effect; on the other hand, the negative impact is reflected in siphon effect (Y. Hu, Deng, & Zhang, Citation2020). In terms of the degree of influence, the lower the initial transport endowment of the city, the higher the capital or technological intensity of the industry, and the closer the distance from the high-speed train station to the city center, then the more robust the siphoning effect from the high-speed train will be (Coto-Millán et al., Citation2007). Railroad speed increases the GDP per capita in cities with stations. This effect is more pronounced in the middle and later stages of high-speed train speed increase, indicating that there is also a time lag in the impact of railroad speed increase (Y Gao et al., Citation2020). (Jia et al., Citation2021) reveals a double helix mechanism in the effects of high-speed train on industrial structure, and overall, high-speed train enhances the industrial structure height of cities. At the city level, the opening of high-speed trains increases the innovation level of towns along the route. This impact is mainly on the central and western regions and small and medium-sized cities, but not on the significant and large cities (Alexandersson et al., Citation2010). (S. Wang et al., Citation2019) analyzed the regional heterogeneity of the spatial spillover effect of high-speed train construction on actual economic development: the spillover effect on the east is small, the short-term impact on the central and western regions is insignificant, and the real economic growth in the northeast region has a sustained boost. This is because the degree of economic development varies widely among regions in China, among which there is a structural imbalance between the real economy and the virtual economy in the eastern part, a lower conversion rate of patent achievements in the central area, and a more profound restriction of resource factors in the western region.
3. micro impact of high-speed train construction
Railroad systems provide firms with opportunities for face-to-face knowledge exchange (Okumura & Kobayashi, Citation1997). The opening of high-speed trains also diminishes the stickiness of the number of human capital and increases the stickiness of firms’ unit cost of human capital (Vickerman, Citation2015). High-speed trains increase firms’ investment in innovation by alleviating financing constraints and promoting mechanisms such as information exchange and talent acquisition (Vickerman, Citation2015). The number of patent applications rose, and the quality improved for enterprises located in cities with high-speed trains. In terms of heterogeneity, firms further away from innovation centers, those closer to the technological frontier, and industries with more comparative advantages were more affected by the opening of the high-speed train (Clifton & Mulley, Citation2018). (Aldieri, Sena, & Vinci, Citation2018) The ability to obtain technology spillovers varies across regions by studying knowledge and technology spillovers in the U.S., E.U., and Japan. (Aldieri et al., Citation2018) found that substantial technology spillovers are not weakened by distance; in other words, greater distance between firms does not mean weaker technology spillovers. Moreover, there is heterogeneity in the impact of high-speed train on the total factor productivity of firms: it increases the total factor productivity of firms in the east and central regions and suppresses the total factor productivity of firms in the western areas with high-speed train; the total factor productivity increase brought by the opening of a high-speed train is more substantial for non-state firms than for state firms. The effect of a high-speed train is stronger for non-state enterprises than for state enterprises (Miller & Upadhyay, Citation2000).
High-speed trains have direct welfare benefits by reducing commuting and transportation costs and affecting urban environmental prices and land availability for agricultural production (Baum-Snow, Brandt, Henderson, Turner, & Zhang, Citation2017). Improving high-speed trains promotes labor and skill inflows and discourages capital outflows (Catherine, Ross, & Woo, Citation2018). Therefore, to enhance the development momentum of less developed regions, it can be achieved by improving the high-speed train in the area. The opening of high-speed trains allows local agglomeration economies to operate over longer distances, so many firms choose to relocate their companies to the suburbs (Bazin-Benoit, Beckerich, & Delaplace, Citation2016). (Ejaz, Arti, Goswami, & Kerr, Citation2015) empirically analyzes that the reduction in the distance can contribute to the increase in firms’ total factor productivity by comparing data before and after the construction of a high-speed train. (Lin, Citation2016) demonstrated that high-speed trains affect firm inventories through three paths: market expansion, market competition, and transportation costs, promoting economic growth.
4. Review of existing studies
The literature has shown that high-speed train construction has direct and indirect effects on enterprises. The indirect effect is to influence the development of enterprises by affecting regional economic growth and industrial structure upgrading. The immediate impact is to bring the inflow of people, capital, and other factors to promote the development of enterprises. However, the following aspects have been less studied in the literature: microscopic analysis of high-speed train construction on enterprise-level; specific mechanism analysis of high-speed train construction on enterprise innovation; how high-speed train construction affects R&D personnel of enterprises; the role played by market level in the impact of high-speed train construction. The role of marketization in the effects of high-speed train construction. This paper first analyzes the impact of high-speed train construction on enterprises and the mechanism of high-speed train construction on R&D personnel through high-speed train-transportation distance-R&D personnel-enterprise innovation. In addition to the internal mechanism of R&D personnel, this paper further analyzes the impact of the external marketization level on the creation of enterprises. Finally, this paper examines the effects of high-speed train construction through the mechanism of high-speed train construction-sales revenue-enterprise innovation. The existing studies on the impact of high-speed train construction on corporate innovation have been conducted from the perspectives of alleviating financing constraints (Y. Wang et al., Citation2019), affecting the distance to innovation centers (Gao & Zheng, Citation2017), and increasing the proportion of highly educated employees (S. Wang et al., Citation2019). And this paper tries to analyze the specific mechanism of high-speed train construction affecting the innovation level of enterprises from three perspectives: human capital and sales revenue of enterprises, and the external marketability level.
5. Method
5.1. Model construction
i. Baseline model
The basic assumption of traditional two-period DID is that all individuals are exposed to policy shocks at the same point in time. In contrast, multi-period DID applies to the case where the treatment periods of individuals cannot be the same. Considering the differences in the timing of high-speed train construction in different regions, this paper will refer to (Beck, Levine, & Levkov, Citation2010) and use multi-period DID for benchmark regression to analyze the impact of high-speed train construction on firm innovation.
Using the multi-period DID model assumes that both the experimental and control groups have the same trend in the absence of extraneous shocks. Therefore, this paper will perform a parallel trend test to verify the model’s validity before fulfilling the baseline regression. To test the similar trend hypothesis of the multi-period DID model and describe the dynamic relationship between the construction of high-speed train and the innovation level of listed companies, this paper adds a series of dummy variables before and after the occurrence of the policy in the multi-period DID model, excludes the dummy variables of the year of the construction of high-speed train and does not consider the relevant control variables at the level of listed companies and at the regional level The model is set up as follows to study the impact of the years before and after the construction of high-speed train on corporate innovation.
Refers to the total number of patents of the listed company in the current year; In the current year, when the share is j years before the construction of high-speed train,
is 1, when the claim is j years after the construction of high-speed train,
is 1, and the other policy dummy variables
are 0; µ is the time fixed effect; γ is the fixed effect of enterprises; ε is random standard error.
After passing the parallel trend test, this paper carries out multi-stage model regression. First, the primary form of the model is set as follows.
Refers to the total number of patents of the listed company in the current year;
is the construction of high-speed train in the city where the listed company is located in the sample. The high-speed train built in the prefecture-level town where the listed company is situated in that year is taken as 1, and the high-speed train not made in that year is taken as 0;
is the critical coefficient of this paper, which represents the impact of high-speed train construction on enterprise innovation.
Represents the control variables at the level of listed companies and regions. The control variables at the level of listed companies mainly include enterprise-scale, asset-liability ratio, Tobin Q value, enterprise revenue level, and the number of managers. The control variables at the regional level mainly include the regional economic level, regional human capital level, and total highway passenger transport.
ii. Robustness test
Because there is some variation in the degree of development of different firms, to exclude possible endogeneity problems, this paper will conduct a series of robustness tests on the model, including PSM-DID, placebo test, replacement of explanatory variables, and instrumental variables method.
To exclude the bias of sample selection, this paper will adopt the method of propensity score matching to match the control group with similar characteristics of listed companies in high-speed train construction cities to eliminate the problem of sample selection; the specific steps are: the sample is divided into two groups, one group is the experimental group (T), which represents the listed companies in high-speed train construction cities. First, the predicted scores of all sample listed companies are estimated by probability formula. Then companies with similar predicted scores are then matched using propensity score matching to obtain listed companies with similar characteristics to the experimental group, called the control group (C). After solving the selection bias by propensity score matching, the sample is then regressed using a multi-period DID model. The probability formula for a listed company is
.
Where: is the fraction of listed companies for matching; ф{.} is the probability calculation formula, normal cumulative distribution function;
includes firm size (Asset), gearing ratio (LEV), Tobin Q value (Tobin Q), the Enterprise revenue level (Operating profit), and several management (Manager) to match the experimental group sample.
The specific model for multi-period DID is set as follows.
Where: is the patent data of listed companies obtained by PSM matching;
is the control variable at the recorded company level and the regional level;
is the time fixed effect;
is the firm settled outcome;
is the random standard error.
(2) In analyzing the impact of high-speed train construction on firm innovation, a placebo test is needed to eliminate its systematic error because the model may omit relevant unobservable variables. Therefore, the placebo test is required to eliminate its systematic error, and the econometric model is as follows.
When the year is j (j takes 1,2,3) years before the construction of high-speed train, it is 1. Otherwise, it is 0;
it is the control variable at the listed company and regional levels.
(3) Firms’ innovation mainly includes innovation inputs and innovation outputs, the former representing firms’ innovation capability from the input side and the latter from the output side. To further verify the robustness of the benchmark regression results, this paper re-estimates the model using the share of enterprise R&D expenses instead of the number of patents. The model is set as follows.
Where: is the R&D cost share of the company;
is the control variable at the listed company level and the regional level.
(4) Although the previous series of robustness tests can alleviate the endogeneity problem between high-speed train construction and firm innovation, there is still a possibility of missing unobservable variables in the model. Therefore, this paper will further use instrumental variables to perform robustness tests on the model. In this paper, we borrow the idea of constructing instrumental variables from (Beck et al., Citation2010) and adopt the Ming dynasty post station as the instrumental variable for creating a high-speed train. Post stations were built to connect important cities and convey central government orders and military information in the Ming Dynasty. Therefore, the Ming dynasty post stations were similar to high-speed train stations in terms of function and satisfied the condition of instrumental relevance. In addition, the location of post-stations in the Ming Dynasty was chosen for political reasons at that time and did not affect the local economic development. Therefore, Ming Dynasty post stations are selected as the instrumental variable for constructing high-speed trains. The data of post stations in the Ming Dynasty are compiled from the “Examination of Post Stations in the Ming Dynasty (Updated Version),” in which the ancient and modern addresses of all post stations in the Ming Dynasty are compiled. In this paper, we take 1 for prefecture-level cities with post stations of the Ming Dynasty and 0 if not, and use them as the instrumental variables for regression. The model is set as follows.
Where: is the total number of patents of listed companies in the year;
is the instrumental variable of HSR construction;
is the control variable at recorded company level and regional level.
iii. Mechanism of action test
First, the mechanism of the role of HSR construction on firm innovation is analyzed based on the mediating effect model, which focuses on whether the mobility of R&D personnel plays a role between HSR construction and firm innovation. In this paper, we want to measure the high level of researchers by the percentage of corporate R&D workers (RDworkerR). The intermediate effect model is set as follows.
Where: is the total number of patents of listed companies in the current year;
represents the proportion of R&D personnel of enterprises;
is the control variable at recorded company level and regional level.
The intermediary effect is divided into three main steps, Path a, Path b, and Path c. The first step (Path a) tests the relationship between HSR construction and firm innovation and obtains α1, and proceeds to the next test. The second step (Path b) tests the relationship between the construction of HSR and the share of R&D personnel and obtains β1, significant then proceed to the next test. The third step (Path c): the mediating effect is analyzed, and the mediating variable is included in the regression model to obtain the coefficient
. The full mediating effect is established if the absolute value is small and insignificant.
Second, we test whether there is a mediating effect of the level of regional marketization on firm innovation. Since increased market competition will stimulate firms to initiate creation, the R&D expense ratio (RDexpenseR) represents innovation. The mediating effect model is also used here. The model is set up as follows.
Where: is the marketability level of the region where the company is located;
is the R&D cost share of the company.
Subsequently, the paper will analyze whether the level of marketization affects the factors, profit level, and the degree of competition of the firm with the following model setup.
Where: Y represents the current assets, return on assets (ROA), and competition in the market (HHI), respectively. Among them, current assets are used to illustrate the increase of existing elements of the enterprise, return on assets (ROA) describes the profit of the enterprise, and market competition (HHI) demonstrates the degree of competition faced by the enterprise.
6. Variable selection
The explanatory variables. Pat denotes the total number of patents of the firm in the year to measure the firm’s innovation output. rDexpenseR is the share of the firm’s R&D expenditure as a measure of the firm’s innovation investment. rDworkerR denotes the proportion of the firm’s R&D staff in the year.
The core explanatory variables. Train represents the construction of high-speed train construction dummy variable. 1 is taken if a high-speed train is formally constructed at the location of a listed company in a certain year; otherwise, 0. C_city represents the central city dummy variable. This paper refers to the document “National Urban System Planning (2010–2020)” issued by the Ministry of Housing and Urban-Rural Development in 2010, dividing all cities into national and non-national central cities. According to the relevant documents issued by the National Development and Reform Commission and the Ministry of Housing and Urban-Rural Development successively at a later stage, there are nine existing national central cities, namely Beijing, Tianjin, Shanghai, Guangzhou, Chongqing, Chengdu, Wuhan, Zhengzhou, and Xi’an. c_city = 1 represents central cities and c_city = 0 represents non-central cities.
The state represents the enterprise ownership dummy variable. In this paper, enterprises are divided into state-owned and non-state-owned enterprises, where state = 1 represents state-owned enterprises, and tech = 0 represents non-state-owned enterprises.
Marketization represents the marketization level of the region where the enterprise is located, and the marketization index is derived from the total marketization index score in the China Marketization Index Report by Province. The marketization index scores are mainly based on “the relationship between government and market,” “the development of the non-state economy,” “the degree of Frenchness of product market,” “the degree of development of the elemental market,” and “the degree of development of the market.” Therefore, the higher the score, the higher the degree of marketization of the city.
The control variable. Lev represents the asset-liability ratio of the enterprise, which is equal to the total liabilities divided by the total assets to evaluate the debt level of the enterprise. Tobin Q value represents the ratio of the enterprise’s market value to the replacement capital, which can determine the market expectation for the future profit of the enterprise. The asset represents the total asset level of an enterprise and is used to evaluate the size. Operating profit means the operating profit margin of an enterprise and considers the functional income level of the enterprise. The manager represents the number of managers in the enterprise. GDP represents the GDP level of the place where the enterprise is located in the current year and evaluates the market economic environment of the area where the enterprise is situated. Employee represents the total number of employees where the enterprise is located and evaluates the level of regional human capital. Highway means the full highway passenger transport in the yenterprise’s year City distance represents the transportation distance from the enterprise to the nearest city center.
7. Data sources
The primary data sources of this paper are CMSAR (China Stock Market & Accounting Research Database), Wind database, and statistical yearbooks of cities in various regions. Among them, the patent data of listed companies are from the CMSAR database. The data of listed companies are mainly from the CMSAR database, partly supplemented from the Wind database, and the data of regional characteristics are collected from city statistical yearbooks. The sample selection scope of this paper is listed companies in Shanghai, and Shenzhen A-shares, excluding S.T. enterprises, excluding insurance and financial enterprises, excluding listed companies with missing indicators, and finally, a total of 12,470 valid data are retained. The data of patent, enterprise, and regional characteristics are taken as logarithms.
8. Results and discussion
below lists the differences in the number of patents between enterprises without high-speed train construction and those in areas with high-speed train construction, mainly divided into differences in the three categories of a total number of patents, invention patent applications, and invention patent grants. In the sample, whether it is the total number of patents, the number of invention patent applications, or the number of invention patent licenses, there are significant differences between enterprises in regions where the high-speed train is built and those in areas where the high-speed train is not made, so it is initially assumed that the construction of high-speed train has a positive impact on enterprise innovation.
Table 1. Differences in the number of patents between enterprises in areas where high-speed railways are built and those in areas where they are not built.
9. Baseline regression results
In this paper, benchmark regressions are conducted based on satisfying parallel trends. reports the results of the benchmark regressions of high-speed train construction on firm innovation. regressions are fixed effects models with both firm and time set. Columns (1), (3), and (5) show the empirical results without introducing listed firm-level and regional-level control variables; columns (2), (4), and (6) show the observed results by introducing listed firm-level and regional-level control variables. In the empirical evidence without introducing control variables, the coefficients of the effect of high-speed train construction on the total number of patents, the number of invention patent applications, and the number of invention patents granted are 0.122, 0.0656, and 0.0667, respectively, and all of them are significant. The coefficients of the effect of high-speed train on the total number of patents, the number of invention patent applications, and the number of invention patent grants are 0.115, 0.0552, and 0.0623, respectively, in the empirical evidence of introducing the control variables of listed companies and regions. The effects on the number of patent applications were not significant. In general, the high-speed train will significantly increase the number of patents of enterprises.
Table 2. Baseline regression results.
The effect of enterprise size on patents is 0.112, which is significant at the 1% level, which indicates that the larger and stronger the enterprise is, the more resources it can invest in innovation R&D. The effect of the asset-liability ratio on patents is also positive. From the perspective of enterprise operation, the asset-liability ratio can represent the level of enterprise operation to a certain extent. Thus it also indicates that enterprises attach importance to the investment in innovation R&D in their daily operation. Tobin’s Q value represents the enterprise’s market value, and the higher the market value, the more the enterprise attaches importance to innovation, so its effect on patents is significantly positive. The impact of regional road passenger traffic on patents is −0.0167, which is significant at the 10% level. There is a substitution effect between road and rail in transportation, and road transport takes longer and increases the cost of factor flow, so its impact coefficient is negative.
10. Robustness test
(1) PSM-DID
(Shaw, Fang, Lu, & Tao, Citation2014) divided the high-speed train development into four phases, which are: the first phase (before August 2008) had no high-speed train service, the second phase (August 2008 to July 2011) built several high-speed train routes, the third phase (August 2011 to November 2012) the railroad slowdown policy was implemented due to the Wenzhou high-speed train accident, and the fourth phase (December 2012 to January 2013) new high-speed train routes were added, and fares were reduced. Therefore, this paper refers to this phase division basis, sets 2011 as the policy shock time point, and artificially divides the experimental and control groups.
Then, this paper uses the PSM method to test the uniform distribution of heterogeneous variables at the listed company level, and the results are shown in . The deviation of the control variables at the recorded company level after matching is substantially reduced. The t-value results indicate that the deviation after matching accepts the original hypothesis, which indicates that the experimental group’s mean value and control group’s mean value after checking are closer, and the heterogeneous variables at the listed company level pass the test of systematicity. The use of propensity score matching method for the matched control group for regression can eliminate sample selectivity bias to a large extent.
Table 3. Propensity score matching results.
By regressing the matched samples (), the results show that the coefficient of the impact of high-speed train construction on firm innovation is 0.115, which is consistent with the regression coefficient in , which indicates that the regression results in are robust.
Table 4. PSM-DID results.
(2) In this paper, a placebo test was conducted on the multi-period DID model, and the results are shown in . The coefficients of the constructed dummy variables are close to zero and insignificant, indicating that there is no interference of unobservable systematic errors on the estimation results and the baseline regression. Furthermore, the regression results passed the placebo test.
Table 5. Placebo test.
(3) Instrumental variable method
The results in show that high-speed train construction can still significantly increase the number of patents of firms using the instrumental variables method regression, which is the same as the results of the previous benchmark regression, so the robustness of the benchmark regression results can be verified.
Table 6. Instrumental variables regression.
11. Mechanism analysis
11.1. Human capital
reports the results of human capital mediated effects. First, the first column indicates that the impact of high-speed train construction on the number of firm patents is significantly positive, suggesting that high-speed train construction promotes firm innovation. Secondly, the second column regression results indicate that the high-speed train construction can make local firms recruit more R&D personnel and significantly increase the number of R&D personnel in listed firms. Finally, putting the dummy variable of high-speed train construction and the number of R&D personnel shared into the model simultaneously, the results show that the effects of both high-speed train construction and R&D personnel share are significantly positive. The coefficient of high-speed train construction development decreases from 0.115 to 0.107, which indicates the existence of the human capital mediating effect. This suggests that the mediating product of human capital exists, and it is a partial mediating effect.
Table 7. Regression of human capital mediating effects.
Further analysis is conducted based on the result that a partial mediating effect of human capital exists. The exchange and cooperation between enterprises and universities, and research institutes are conducive to enhancing the innovation level of enterprises while improving the transportation convenience helps enterprises find external partners and promote innovation. Therefore, the closer the transportation distance of enterprises from universities, the stronger their innovation ability is, and the further the transportation distance of enterprises from universities, the weaker their innovation ability is. The first column of of the regression results shows that the impact of the transportation distance from enterprises to colleges and universities on the innovation level of enterprises is significantly harmful. Since the transportation distance is mainly city-to-city, this paper’s regression adopts the LSDV method and controls for city fixed effects. The results show that the impact of space remains significant.
Table 8. Regression of transportation distance on firm innovation.
The regression results in the first and second columns of illustrate that the transportation distance from the firm to the university hurts firm innovation. Therefore, this paper adds the cross term of the high-speed train construction dummy variable and traffic distance to the model to illustrate the impact brought by high-speed trains. The results are shown in the third and fourth columns of , where the coefficients of the cross-sectional term between the dummy variable of high-speed train construction and traffic distance are positive and significant. This indicates that the negative effect of the traffic distance between the firm and the university is more negligible for firms that build high-speed train cities. This is because, after the construction of high-speed train, the communication travel is more convenient, the effect of traffic distance is weakened, and the enterprises have the incentive to recruit suitable employees or find partners in the broader area. The employees of the enterprises can also look for universities for further study in the broader circle, thus enhancing the innovation ability of the enterprises.
12. Level of marketization
reports the results of the mediating effects of the level of marketization. This is because, firstly, the first column indicates that the high-speed train construction significantly increases the share of R&D investment of enterprises, which suggests that the high-speed train construction promotes the motivation of enterprises to innovate. Secondly, the results in the second column indicate that the structure of high-speed trains significantly improves the marketization level of the region and optimizes the market environment. Finally, when the dummy variable of high-speed train construction and marketization level are put into the model simultaneously, the results show that the effects of both the dummy variable of high-speed train construction and marketization level are significantly positive. The impact of high-speed train construction decreases from 1.077 to 1.033, which indicates that the effect of marketization level is This suggests that there is a partial mediating effect of marketization level. This indicates that the high-speed train construction increases marketization, which promotes companies to actively seek innovation and thus increase their investment in innovation R&D.
Table 9. Intermediation effect of marketization level.
Based on the partial mediating effect of marketization level, this paper further analyzes the impact of marketization level on enterprise factors, profits, and peer competition. The results are listed in . The results in the first column of the table show that the level of marketization has a positive effect on the current asset ratio of enterprises, which indicates that the increase in the level of marketization in the region where the enterprise is located makes it easier for enterprises to obtain various factors needed for enterprise production, and mainly mobile production factors. The accumulation of elements in the enterprise makes it optimize the allocation of aspects to better develop itself and expand its scale. As a result, the local market increases the factor supply side of the enterprise and the market demand. This leads to an increase in the enterprise’s profitability, which brings about an increase in the return on assets. This is reflected in the results in the second column of , where the level of regional marketization raises the firm’s rate of return. The increase in the rate of return allows firms to invest more in innovation R&D and promote innovation. The results in the third column of show that marketization hurts the Herfindahl-Hirschman Index (HHI), indicating that competition in the local market has increased. This is because the construction of high-speed trains increases the city’s attractiveness, and potential competitors from outside the city will enter that market through the signal of high-speed train construction, thus increasing the market competition. The increase in the degree of market competition will inevitably make companies actively seek innovation to consolidate their position to invest more in innovation R&D.
Table 10. The impact of the level of marketization on the factors of the enterprise.
13. Conclusions and recommendations
13.1. Conclusion
This paper mainly analyzed the impact of high-speed railway construction on the innovation ability of listed companies. Firstly, based on the literature analysis method, the macro, regional and micro effects of high-speed railway construction are summarized and analyzed. It is found that the existing literature mainly focuses on the macro level, with relatively little research on micro-enterprises and relatively little analysis on the mechanism of the effect of high-speed railway construction on enterprise innovation. Based on this, this paper analyzes the mechanism of the development of high-speed railway construction on enterprise innovation from the internal and external influences of enterprises, among which the interior through the power of human capital level and sales revenue level, and the exterior through the impact of marketization level. Second, this paper collects balanced panel data at the HSR-city-firm level, establishes a multi-period DID model of HSR construction, empirically tests the hypotheses proposed in the theoretical analysis and conducts robustness tests and heterogeneity analysis on the empirical results. Based on the analysis of this paper, the following conclusions were obtained.
First, the construction of high-speed rail significantly enhances the innovation level of enterprises. From the results of the benchmark regression, the structure and opening of urban high-speed railways can make the number of patents of listed companies increase about 11.5%. The results of PSM-DID, placebo test, replacement of explanatory variables, and instrumental variable method show that the results obtained from the benchmark model are robust.
Second, HSR acts on the innovation level of listed companies mainly through human capital, marketability level, and sales revenue. Firstly, the construction of high-speed rail shortens the transportation distance from enterprises to universities, reducing the cost of finding partners and employees looking for universities for further education and improving the professional knowledge and skills of R&D personnel, thus significantly improving the innovation level of enterprises. Second, high-speed rail construction improves local marketability, mainly through increased factor supply, increased product demand, and increased peer competition affecting enterprises. Among them, the increase in factor supply and market demand will optimize the factor level as well as profit level of enterprises, which can invest more resources into innovation research and development; the increase in peer competition makes enterprises take the initiative to demand innovation due to external competition, and therefore increase their investment in innovation. Finally, the opening of high-speed railway construction promotes the flow of sales personnel, enhances the marketing ability of enterprises, and raises sales revenue, thus enhancing the motivation of innovation demand and motivating enterprises to take the initiative to innovate.
Third, there is heterogeneity in the impact of high-speed rail construction on different types of listed companies. For example, the effect on innovation output of listed companies is more significant after the construction of HSR without partnership relative to partners, non-central cities close to central cities, and non-state-owned enterprises relative to state-owned enterprises.
14. Recommendations
The construction of high-speed rail promotes national economic development and prosperity at the macro level and the opening up and development of a region at the regional level. At the micro-level, high-speed railway construction improves the number of patents and enhances the innovation level of listed companies along the route. However, there is heterogeneity in its impact. Based on the research results, this paper proposes the following insights.
First, the government should consider regional development when planning high-speed rail routes. From the results of heterogeneity analysis, it can be seen that
It can be seen from the results of the heterogeneity analysis that there are significant differences in the economic and social development of cities at different levels, and non-central towns have a more noticeable effect of high-speed rail construction on enterprise innovation compared to central cities, indicating that non-central cities need to build high-speed rail more. Therefore, relevant government departments should prioritize non-central cities when formulating future high-speed rail planning.
Second, the government should pay attention to transportation distance in economic development. According to the mechanism analysis, transportation distance can have an
enterprise innovation has a negative impact. Regional development should pay attention to the collocation of universities, research institutes, and enterprises, reasonably allocate the distance of the three, promote the integrated development of industry-university-research, maximize the advantages of the three, and realize the industrialization of the transformation of innovation achievements.
Third, regions should pay attention to the role of talents in innovation. From the regression results, we can see that human capital plays a vital role in enterprise
Human capital plays a vital role in enterprise innovation. Therefore, the government should continue to increase investment in education, cultivate innovative talents, and encourage introducing technology and outstanding skills to better play the role of talents and enhance the overall innovation level.
15. Research outlook
The rapid development of high-speed railroads in China makes the impact of high-speed railways on the economy and society a vital research topic in academia. However, most of the literature has analyzed whether high-speed railway construction promotes regional GDP, employment, and industrial development at the macro and regional levels. Only a small amount of literature has examined how high-speed railway construction improves the innovation level of enterprises at the micro-enterprise level. This paper analyzes the mechanism of the effect of high-speed rail on the innovation level of enterprises in terms of R&D personnel and marketability. However, there are still many areas for improvement in the theoretical and empirical analyses, and future research needs to be extended and further studied in the following aspects.
First, this paper analyzes the mechanism of high-speed rail on enterprise innovation from the perspective of human capital. As an essential tool of “passenger transportation,” whether high-speed rail drives the flow of other factors in addition to “people” needs to be further supplemented and improved in the future. Therefore, this paper only analyzes the mechanism from the perspective of human capital. Still, there is a lack of analysis from other perspectives, so we need to further expand the study of the corresponding mechanism in the future.
Second, the marketization level in this paper uses provincial-level data. In contrast, the control variables use prefecture-level city-data. Hence, the marketization level index of prefecture-level cities needs to be further measured in the future to achieve better matching of the data.
Third, the impact of sales revenue may have a certain two-sidedness. For example, for small and medium-sized enterprises, the construction of high-speed rail may lead to a loss of sales revenue and a negative impact; for listed enterprises, they are often the leading enterprises in the industry and have a specific monopoly position, leading to possible differences in the effect of different sectors and different sizes of enterprises, which requires further refinement in the future.
Fourth, the best travel time for high-speed rail travel is 3–4 hours, and people tend to choose air travel when the distance exceeds this time. Finally, the impact of the airline network on economic development and whether there is a compensating or substitution effect between airlines, high-speed rail, and highways need to be further analyzed.
Ethical statement
This article does not contain any studies with human participants or animals performed by any authors. I certify that this manuscript is original and has not been published, and will not be submitted elsewhere for publication. And the study is not split up into several parts to increase submissions and submitted to various journals or one journal over time.
Disclosure statement
No potential conflict of interest was reported by the author(s).
Data availability statement
The submitted article appears all data, models, and code generated or used during the study.
Additional information
Funding
References
- Aldieri, L., Sena, V., & Vinci, C. P. (2018, april). Domestic R&D spillovers and absorptive capacity: Some evidence for U.S., Europe, and Japan. International Journal of Production Economics, 198, 38–49.
- Alexandersson, G., Hulten, S., Fearnley, N., & Longva, F. (2010). Impact of regulation on the performances of long-distance transport services: A comparison of the different approaches in Sweden and Norway. Research in Transportation Economics, 29(1), 212–218.
- Andersson, D. E., Shyr, O. F., & Fu, J. (2010). Does high-speed rail accessibility influence residential property prices? Hedonic estimates from southern Taiwan. Journal of Transport Geography, 18(1), 166–174.
- B, J. C. A., C, X. C. L., C, Y. W., & B, Q. L. A. (2013). Accessibility impacts of China’s high-speed rail network - sciencedirect. Journal of Transport Geography, 28, 12–21.
- Baum-Snow, N., Brandt, L., Henderson, J. V., Turner, M. A., & Zhang, Q. (2017). Roads, railroads and decentralization of Chinese cities. Review of Economics & Statistics, 99(3), 435–448.
- Bazin-Benoit, S., Beckerich, C., & Delaplace, M. (2016). High-speed rail, corporate real estate and firm location: The results from two surveys (2008, 2014) in Reims. Open Transportation Journal, 10(Suppl–1, M2), 7–21.
- Beck, T., Levine, R., & Levkov, A. (2010). Big bad banks? The winners and losers from bank deregulation in the United States. The Journal of Finance, 65(5), 1637–1667.
- Castillo-Manzano, J. I., Pozo-Barajas, R., & Trapero, J. R. (2015, february). Measuring the substitution effects between high speed rail and air transport in Spain. Journal of Transport Geography, 43, 59–65.
- Catherine, L., Ross, M., & Woo. (2018). Identification and assessment of potential high-speed rail routes from megaregion perspective. Transportation Research Record, 2307(1), 31–42.
- Chen, Z., & Haynes, K. E. (2017, december). Impact of high-speed rail on regional economic disparity in China. Journal of Transport Geography, 65, 80–91.
- Clifton, G., & Mulley, C. (2018, September). Barriers and facilitators of integration between buses with a higher level of service and rail: An Australian case study. Research in Transportation Economics, 69, 39–50.
- Coto-Millán, P., Inglada, V., & Rey, B. (2007). Effects of network economies in high-speed rail: The Spanish case. The Annals of Regional Science, 41(4), 911–925.
- David, E., Andersson, O., ShyrAngel, F., & Lee. (2012). The successes and failures of a key transportation link: Accessibility effects of Taiwan’s high-speed rail. Annals of Regional Science, 49(2), 355–372.
- Dong, X., Zheng, S., & Kahn, M. E. (2019). The role of transportation speed in facilitating high skilled teamwork across cities. Journal of Urban Economics, 115, 103212.
- Duan, L., Sun, W., & Zheng, S. (2020). Transportation network and venture capital mobility: An analysis of air travel and high-speed rail in China. Journal of Transport Geography, 88, 102852.
- Ejaz, G., Arti, G., Goswami, W., & Kerr, R. (2015). Highway to success: The impact of the golden quadrilateral project for the location and performance of Indian manufacturing. The Economic Journal, 126(591), 317–357.
- Gao, Y., & Zheng, J. (2017). The impact of high-speed rail on innovation: An empirical test of the companion innovation hypothesis of transportation improvement with China’s manufacturing firms - ScienceDirect. World Development. 127.
- Gao, Y., Song, S., Sun, J., & Zang, L. (2020). Does high‐speed rail connection really promote local economy? Evidence from China’s Yangtze River Delta. Review of Development Economics, 24(1), 316–338.
- Gutiérrez, J., González, R., & Gómez, G. (1996). The European high-speed train network: Predicted effects on accessibility patterns. Journal of Transport Geography, 4(4), 227–238.
- Hof, B., Heyma, A., & Hoorn, T. (2012). Comparing the performance of models for wider economic benefits of transport infrastructure: Results of a Dutch case study. Transportation, 39(6), 1241–1258.
- Hu, X., Huang, J., & Shi, F. (2019, October). Circuity in China’s high-speed-rail network. Journal of Transport Geography, 80, 102504.1–102504.13.
- Hu, Y., Deng, T., & Zhang, J. (2020). Can commuting facilitation relieve spatial misallocation of labor? Habitat International, 106(2), 102136.
- Jia, R., Shao, S., & Yang, L. (2021). High-speed rail and CO2 emissions in urban China: A spatial difference-in-differences approach - ScienceDirect. Energy Economics, 99, 105271. Energy Economics
- Kamga, C. (2015, january). Emerging travel trends, high-speed rail, and the public reinvention of U.S. transportation. Transport Policy, 37, 111–120.
- Kanafani, A., Rui, W., & Griffin, A. (2012). The Economics of Speed – Assessing the performance of High Speed Rail in Intermodal Transportation. Procedia - Social and Behavioral Sciences, 43(none), 692–708.
- Lin, Y. (2016, March). Travel costs and urban specialization patterns: Evidence from China’s high speed railway system. Journal of Urban Economics, 98, 98–123.
- Miller, S. M., & Upadhyay, M. P. (2000). The effects of openness, trade orientation, and human capital on total factor productivity. Journal of Development Economics, 63(2), 399–423.
- Okumura, M., & Kobayashi, K. (1997). The growth of city systems with high-speed railway systems. The Annals of Regional Science, 31(1), 39–56.
- Perl, A. D., & Goetz, A. R. (2015, january). Corridors, hybrids and networks: Three global development strategies for high speed rail. Journal of Transport Geography, 42, 134–144.
- Shaw, S. L., Fang, Z., Lu, S., & Tao, R. (2014). Impacts of high speed rail on railroad network accessibility in China. Journal of Transport Geography, 40, 112–122.
- Soetevent, A. R., Haan, M. A., & Heijnen, P. (2014). Do auctions and forced divestitures increase competition? Evidence for retail gasoline markets. Journal of Industrial Economics, 62(3), 467–502.
- Vickerman, R. (2015). High-speed rail and regional development: The case of intermediate stations. Journal of Transport Geography, 42, 157–165.
- Wang, D., Qian, J., Chen, T., Zhao, M., & Zhang, Y. (2014). Influence of the high-speed rail on the spatial pattern of regional tourism – Taken Beijing–Shanghai high-speed rail of China as example. Asia Pacific Journal of Tourism Research, 19(8), 890–912.
- Wang, Y., Liang, S., Kong, D., & Wang, Q. (2019). High-speed rail, small city, and cost of debt: Firm-level evidence. Pacific-Basin Finance Journal, 57, 101194.
- Willigers, J., & Wee, B. V. (2011). High-speed rail and office location choices. A stated choice experiment for the Netherlands. Journal of Transport Geography, 19(4), 745–754.
- Yang, Z., Li, C., Jiao, J., Liu, W., & Zhang, F. (2020). On the joint impact of high-speed rail and megalopolis policy on regional economic growth in China. Transport Policy, 99, 20–30. Transport Policy
- Yao, S., Zhang, F., Wang, F., & Ou, J. (2019). High‐speed rail and urban economic growth in China after the global financial crisis. China & World Economy, 27(2), 44–65.
- Yu, L. (2021). Study on treatment effects and spatial spillover effects of Beijing–Shanghai HSR on the cities along the line. The Annals of Regional Science, 67(3), 671–695.